


What is the relationship between human neural network (brain) and artificial neural network (ANN)?
A teacher once compared it this way: It’s like the relationship between a mouse and Mickey Mouse.
Real-life neural networks are powerful, but completely different from the way humans perceive, learn and understand.
For example, ANNs exhibit vulnerabilities not usually found in human perception, and they are susceptible to adversarial perturbations.
An image may only need to modify the values of a few pixels, or add some noise data,
From a human perspective, observe There is no difference, and for the image classification network, it will be recognized as a completely unrelated category.
However, the latest research from Google DeepMind shows that our previous view may be wrong!
Even subtle changes in digital images can affect human perception.
In other words, human judgment can also be affected by such adversarial perturbations.
Paper address: https://www.nature.com/articles/s41467-023-40499-0
This article by Google DeepMind was published in Nature Communications.
The paper explores whether humans might also exhibit sensitivity to the same perturbations under controlled testing conditions.
Through a series of experiments, the researchers proved this.
At the same time, this also shows the similarities between human and machine vision.
Adversarial images
Adversarial images are subtle changes to an image that cause the AI model to misclassify the image content, - This type of deliberate deception is called an adversarial strike.
For example, an attack could be targeted to cause an AI model to classify a vase as a cat, or as anything other than a vase.
The above figure shows the process of adversarial attack (for the convenience of human observation, the random perturbations in the middle are exaggerated).
In digital images, each pixel in the RGB image has a value between 0-255 (at 8-bit depth), and the value represents the intensity of a single pixel.
For adversarial attacks, the attack effect may be achieved by changing the pixel value within a small range.
In the real world, adversarial attacks on physical objects may also be successful, such as causing a stop sign to be mistakenly recognized as a speed limit sign.
So, for security reasons, researchers are already working on ways to defend against adversarial attacks and reduce their risks.
Adversarial effects on human perception
Previous research has shown that people may be sensitive to large-amplitude image perturbations that provide clear shape cues.
However, what impact do more nuanced adversarial attacks have on humans? Do people perceive perturbations in images as harmless random image noise, and does it affect human perception?
To find out, researchers conducted controlled behavioral experiments.
First a series of raw images are taken and two adversarial attacks are performed on each image to produce multiple pairs of perturbed images.
In the animated example below, the original image is classified by the model as a "vase".
Due to the adversarial attack, the model misclassified the two disturbed images as "cat" and "truck" with high confidence.
Next, human participants were shown the two images and asked a targeted question: Which image was more like a cat? ?
Although neither photo looked like a cat, they had to make a choice.
Often, subjects believe that they have made random choices, but is this really the case?
If the brain was insensitive to subtle adversarial attacks, subjects would choose each picture 50% of the time.
However, experiments have found that the selection rate (that is, human perception bias) is actually higher than chance (50%), and in fact the adjustment of picture pixels is very small.
From the participant's perspective, it feels like they are being asked to differentiate between two nearly identical images. However, previous research has shown that people use weak perceptual signals when making choices - even though these signals are too weak to convey confidence or awareness.
In this example, we might see a vase, but some activity in the brain tells us that it has the shadow of a cat.
#The above figure shows pairs of adversarial images. The top pair of images are subtly perturbed, with a maximum amplitude of 2 pixels, causing the neural network to misclassify them as "truck" and "cat" respectively. (Volunteers were asked "Which one is more like a cat?")
The disturbance in the lower pair of images is more obvious, with a maximum amplitude of 16 pixels, and was incorrectly classified by the neural network as " "Chair" and "Sheep". (This time the question was "Which one is more like a sheep?")
In each experiment, participants reliably chose the answer to the target question more than half the time. Confrontation images. While human vision is not as susceptible to adversarial perturbations as machine vision, these perturbations can still bias humans in favor of decisions made by machines.
If human perception can be affected by adversarial images, then this will be a new but critical security issue.
This requires us to conduct in-depth research to explore the similarities and differences between the behavior of artificial intelligence visual systems and human perception, and to build safer artificial intelligence systems.
Paper details
The standard procedure for generating adversarial perturbations starts with a pre-trained ANN classifier that maps RGB images to A probability distribution over a fixed set of classes.
Any changes to the image (such as increasing the red intensity of a specific pixel) will produce a slight change in the output probability distribution.
Adversarial images are searched (gradient descent) to obtain a perturbation of the original image that causes the ANN to lower the probability of being assigned to the correct class (non-targeted attack) or assign a high probability Give certain designated alternative categories (targeted attacks).
To ensure that the perturbation does not deviate too far from the original image, the L (∞) norm constraint is often applied in the adversarial machine learning literature, specifying that no pixel can deviate from its original value by more than ±ε, ε is usually much smaller than the [0–255] pixel intensity range.
This constraint applies to pixels in each RGB color plane. Although this limitation does not prevent individuals from detecting changes in the image, by appropriately choosing ε, the main signal indicating the original image category remains mostly intact in the perturbed image.
Experiments
In the original experiment, the authors studied human response to brief, occluded adversarial images. Categorize responses.
By limiting exposure time to increase classification errors, the experiment was designed to increase an individual's sensitivity to aspects of a stimulus that might not otherwise influence classification decisions.
An adversarial perturbation is performed on the image of the real class T. By optimizing the perturbation, the ANN tends to misclassify the image as A. Participants were asked to make a forced choice between T and A.
The researchers also tested participants on control images, which were obtained in condition A by flipping them top-down. Adversarial perturbation image formation.
This simple transformation breaks the pixel-to-pixel correspondence between the adversarial perturbation and the image, largely eliminating the impact of the adversarial perturbation on the ANN, while retaining the perturbation specification and other Statistical data.
The results showed that participants were more likely to judge the perturbed image as category A compared to the control image.
Experiment 1 above used a brief masking demonstration to limit the influence of the original image category (primary signal) on the response, thereby revealing sensitivity to adversarial perturbations (subordinate signal) .
The researchers also designed three other experiments with the same goals, but avoided the need for large-scale perturbations and limited-exposure viewing.
In these experiments, the dominant signal in the image does not systematically guide response selection, allowing the influence of the subordinate signal to emerge.
In each experiment, a nearly identical pair of unmasked stimuli was presented and remained visible until a response was selected. The pair of stimuli have the same dominant signal, they are both modulations of the same underlying image, but have different slave signals. Participants were asked to select images that more closely resembled instances of the target category.
In Experiment 2, both stimuli were images belonging to the T category. One of them was perturbed, and the ANN predicted that it was more like the T category. The other was perturbed and was predicted to be Not even like a T category.
In Experiment 3, the stimulus is an image belonging to the real category T, one of which is perturbed to change the classification of the ANN, making it closer to the target adversarial category A, and the other One uses the same perturbation but flipped left and right as a control condition.
The effect of this control is to preserve the norm and other statistics of the perturbation, but it is more conservative than the control in Experiment 1, because the left and right sides of the image may have more characteristics than the upper and lower parts of the image. More similar statistics.
The pair of images in Experiment 4 are also modulations of the real category T, one is perturbed to be more like category A, and the other is more like category 3. Trials alternated between asking participants to choose an image that was more like Category A, or an image that was more like Category 3.
In Experiments 2-4, the human perceptual bias of each image was significantly positively correlated with the bias of the ANN. Perturbation amplitudes ranged from 2 to 16, which are smaller than perturbations previously studied on human participants and similar to those used in adversarial machine learning studies.
Surprisingly, perturbations of even 2 pixel intensity levels are enough to reliably affect human perception.
The strength of Experiment 2 is that it requires participants to make intuitive judgments (e.g., which of two perturbed cat images is more like a cat) ;
However, Experiment 2 allows adversarial perturbations to make images more or less cat-like simply by sharpening or blurring them.
The advantage of Experiment 3 is that all statistics of the perturbations compared are matched, not just the maximum amplitude of the perturbation.
However, matching perturbation statistics does not ensure that the perturbation is equally perceptible when added to the image, and therefore, participants may make choices based on image distortion.
The strength of Experiment 4 is that it demonstrates that participants are sensitive to the questions being asked, as the same image pairs produced systematically different responses depending on the question asked.
However, Experiment 4 asked participants to answer a seemingly absurd question (e.g., which of two omelet images looks more like a cat?), leading to question interpretation Variability of manner.
Taken together, Experiments 2-4 provide converging evidence that even very small perturbations with unlimited viewing time can have a strong impact on AI networks The subordinate confrontation signal will also affect human perception and judgment in the same direction.
Furthermore, extending the observation time (naturally perceived environment) is key for adversarial perturbations to have real consequences.
The above is the detailed content of Google DeepMind research found that adversarial attacks can affect the visual recognition of humans and AI, mistaking a vase for a cat!. For more information, please follow other related articles on the PHP Chinese website!
![Can't use ChatGPT! Explaining the causes and solutions that can be tested immediately [Latest 2025]](https://img.php.cn/upload/article/001/242/473/174717025174979.jpg?x-oss-process=image/resize,p_40)
ChatGPT is not accessible? This article provides a variety of practical solutions! Many users may encounter problems such as inaccessibility or slow response when using ChatGPT on a daily basis. This article will guide you to solve these problems step by step based on different situations. Causes of ChatGPT's inaccessibility and preliminary troubleshooting First, we need to determine whether the problem lies in the OpenAI server side, or the user's own network or device problems. Please follow the steps below to troubleshoot: Step 1: Check the official status of OpenAI Visit the OpenAI Status page (status.openai.com) to see if the ChatGPT service is running normally. If a red or yellow alarm is displayed, it means Open
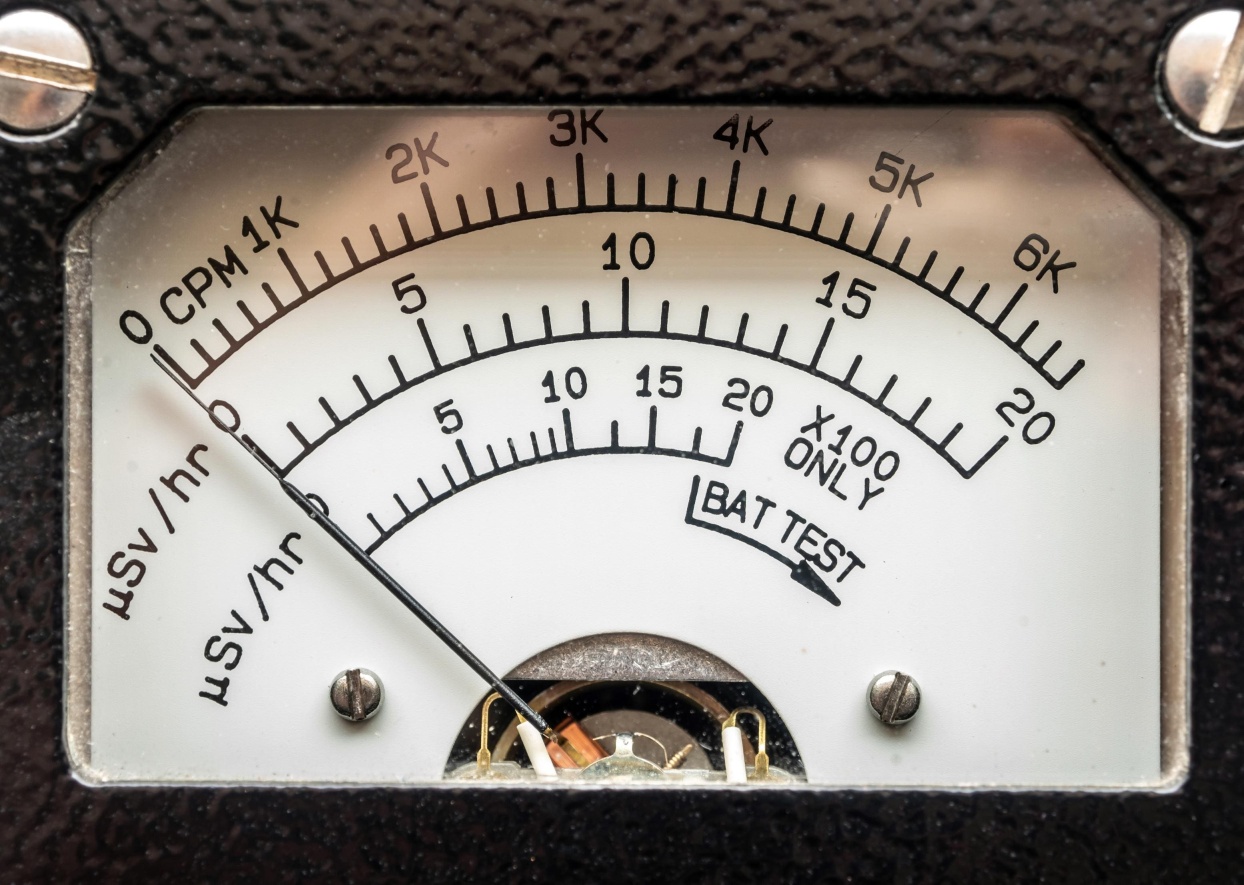
On 10 May 2025, MIT physicist Max Tegmark told The Guardian that AI labs should emulate Oppenheimer’s Trinity-test calculus before releasing Artificial Super-Intelligence. “My assessment is that the 'Compton constant', the probability that a race to
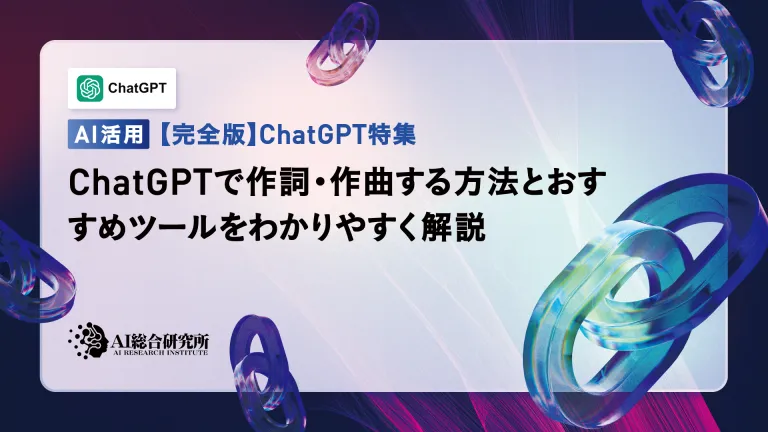
AI music creation technology is changing with each passing day. This article will use AI models such as ChatGPT as an example to explain in detail how to use AI to assist music creation, and explain it with actual cases. We will introduce how to create music through SunoAI, AI jukebox on Hugging Face, and Python's Music21 library. Through these technologies, everyone can easily create original music. However, it should be noted that the copyright issue of AI-generated content cannot be ignored, and you must be cautious when using it. Let’s explore the infinite possibilities of AI in the music field together! OpenAI's latest AI agent "OpenAI Deep Research" introduces: [ChatGPT]Ope
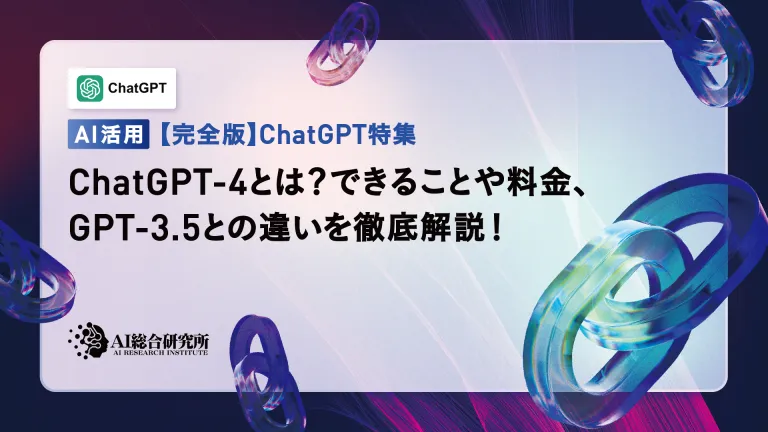
The emergence of ChatGPT-4 has greatly expanded the possibility of AI applications. Compared with GPT-3.5, ChatGPT-4 has significantly improved. It has powerful context comprehension capabilities and can also recognize and generate images. It is a universal AI assistant. It has shown great potential in many fields such as improving business efficiency and assisting creation. However, at the same time, we must also pay attention to the precautions in its use. This article will explain the characteristics of ChatGPT-4 in detail and introduce effective usage methods for different scenarios. The article contains skills to make full use of the latest AI technologies, please refer to it. OpenAI's latest AI agent, please click the link below for details of "OpenAI Deep Research"
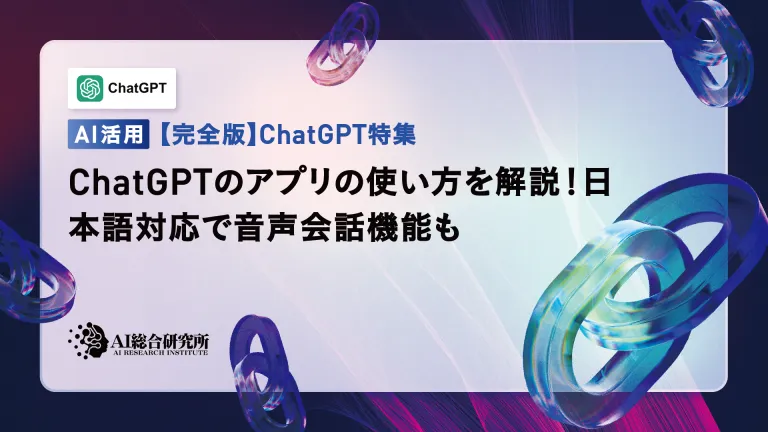
ChatGPT App: Unleash your creativity with the AI assistant! Beginner's Guide The ChatGPT app is an innovative AI assistant that handles a wide range of tasks, including writing, translation, and question answering. It is a tool with endless possibilities that is useful for creative activities and information gathering. In this article, we will explain in an easy-to-understand way for beginners, from how to install the ChatGPT smartphone app, to the features unique to apps such as voice input functions and plugins, as well as the points to keep in mind when using the app. We'll also be taking a closer look at plugin restrictions and device-to-device configuration synchronization
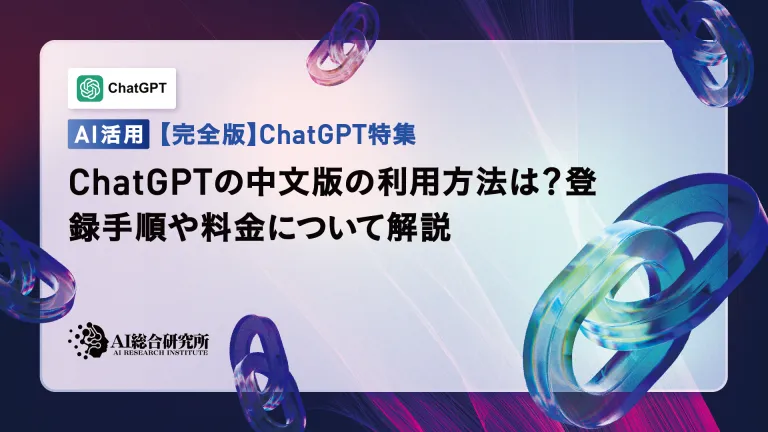
ChatGPT Chinese version: Unlock new experience of Chinese AI dialogue ChatGPT is popular all over the world, did you know it also offers a Chinese version? This powerful AI tool not only supports daily conversations, but also handles professional content and is compatible with Simplified and Traditional Chinese. Whether it is a user in China or a friend who is learning Chinese, you can benefit from it. This article will introduce in detail how to use ChatGPT Chinese version, including account settings, Chinese prompt word input, filter use, and selection of different packages, and analyze potential risks and response strategies. In addition, we will also compare ChatGPT Chinese version with other Chinese AI tools to help you better understand its advantages and application scenarios. OpenAI's latest AI intelligence
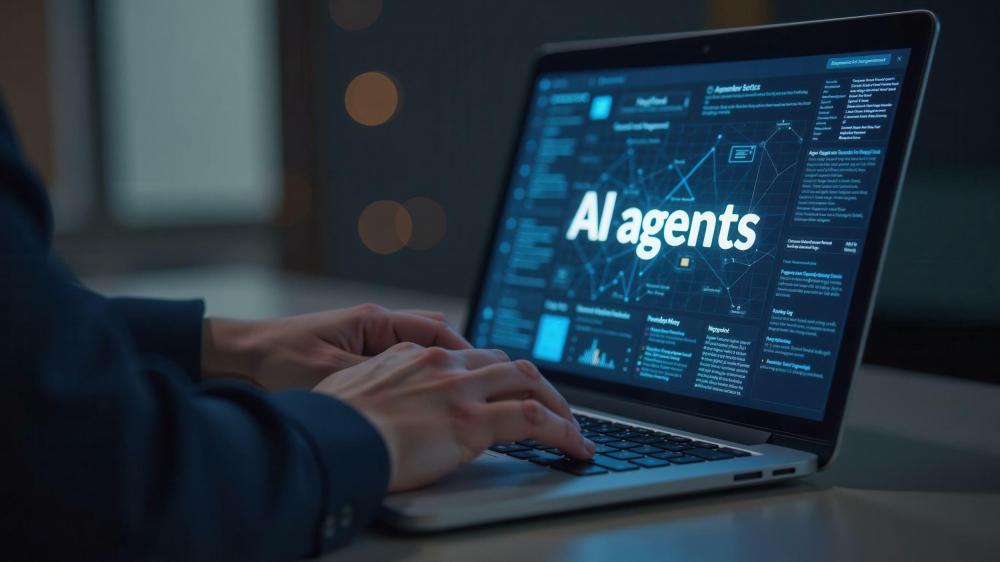
These can be thought of as the next leap forward in the field of generative AI, which gave us ChatGPT and other large-language-model chatbots. Rather than simply answering questions or generating information, they can take action on our behalf, inter
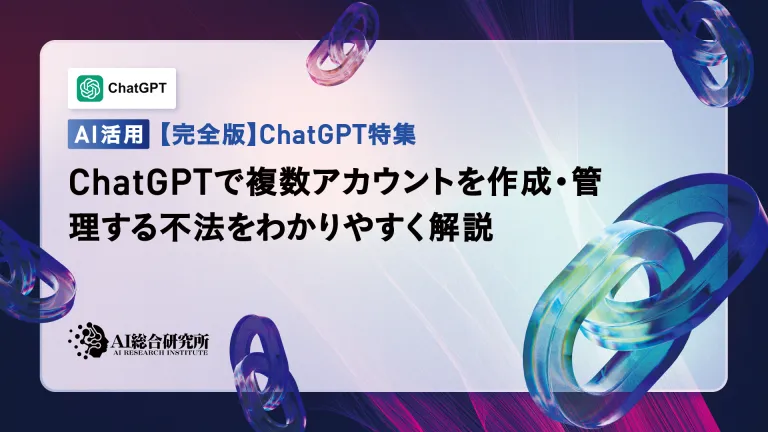
Efficient multiple account management techniques using ChatGPT | A thorough explanation of how to use business and private life! ChatGPT is used in a variety of situations, but some people may be worried about managing multiple accounts. This article will explain in detail how to create multiple accounts for ChatGPT, what to do when using it, and how to operate it safely and efficiently. We also cover important points such as the difference in business and private use, and complying with OpenAI's terms of use, and provide a guide to help you safely utilize multiple accounts. OpenAI


Hot AI Tools

Undresser.AI Undress
AI-powered app for creating realistic nude photos

AI Clothes Remover
Online AI tool for removing clothes from photos.

Undress AI Tool
Undress images for free

Clothoff.io
AI clothes remover

Video Face Swap
Swap faces in any video effortlessly with our completely free AI face swap tool!

Hot Article

Hot Tools

Notepad++7.3.1
Easy-to-use and free code editor

SecLists
SecLists is the ultimate security tester's companion. It is a collection of various types of lists that are frequently used during security assessments, all in one place. SecLists helps make security testing more efficient and productive by conveniently providing all the lists a security tester might need. List types include usernames, passwords, URLs, fuzzing payloads, sensitive data patterns, web shells, and more. The tester can simply pull this repository onto a new test machine and he will have access to every type of list he needs.

MantisBT
Mantis is an easy-to-deploy web-based defect tracking tool designed to aid in product defect tracking. It requires PHP, MySQL and a web server. Check out our demo and hosting services.
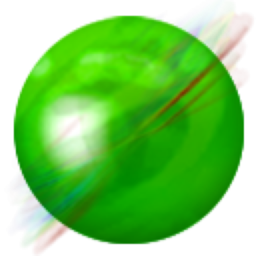
ZendStudio 13.5.1 Mac
Powerful PHP integrated development environment

SublimeText3 Chinese version
Chinese version, very easy to use
