The wave of large models coming to the mobile terminal is getting stronger and stronger, and finally someone has moved multi-modal large models to the mobile terminal. Recently, Meituan, Zhejiang University, etc. have launched multi-modal large models that can be deployed on the mobile terminal, including the entire process of LLM base training, SFT, and VLM. Perhaps in the near future, everyone will be able to own their own large model conveniently, quickly and at low cost.
MobileVLM is a fast, powerful and open visual language assistant designed specifically for mobile devices. It combines architectural design and technology for mobile devices, including 1.4B and 2.7B parameter language models trained from scratch, multi-modal vision models pre-trained in a CLIP manner, and efficient cross-modal interaction through projection. MobileVLM's performance is comparable to large models on various visual language benchmarks. Additionally, it demonstrates the fastest inference speeds on Qualcomm Snapdragon 888 CPU and NVIDIA Jeston Orin GPU. 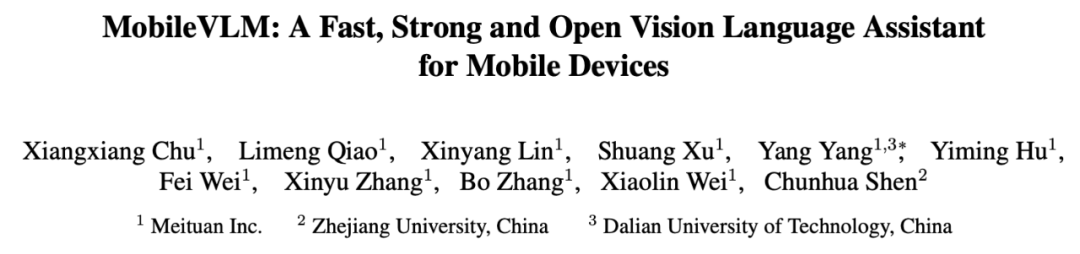
- Paper address: https://arxiv.org/pdf/2312.16886.pdf
- ##Code address: https://github.com/Meituan- AutoML/MobileVLM
Large-scale multimodality Models (LMMs), especially the family of visual language models (VLMs), have become a promising research direction for building universal assistants due to their greatly enhanced capabilities in perception and reasoning. However, how to connect the representations of pre-trained large language models (LLMs) and visual models, extract cross-modal features, and complete tasks such as visual question answering, image subtitles, visual knowledge reasoning, and dialogue has always been a problem.
The excellent performance of GPT-4V and Gemini on this task has been proven many times. However, the technical implementation details of these proprietary models are still poorly understood. At the same time, the research community has also proposed a series of language adjustment methods. For example, Flamingo leverages visual tokens to condition frozen language models through gated cross-attention layers. BLIP-2 considers this interaction insufficient and introduces a lightweight query transformer (called Q-Former) that extracts the most useful features from the frozen visual encoder and feeds them directly into the frozen of LLM. MiniGPT-4 pairs the frozen visual encoder in BLIP-2 with the frozen language model Vicuna via a projection layer. In addition, LLaVA applies a simple trainable mapping network to convert visual features into embedding tokens with the same dimensions as the word embeddings to be processed by the language model.
It is worth noting that training strategies are gradually changing to adapt to the diversity of large-scale multi-modal data. LLaVA may be the first attempt to replicate the instruction tuning paradigm of LLM to a multimodal scenario. To generate multimodal instruction trace data, LLaVA inputs textual information, such as the description sentence of the image and the bounding box coordinates of the image, to the pure language model GPT-4. MiniGPT-4 is first trained on a comprehensive dataset of image description sentences and then fine-tuned on a calibration dataset of [image-text] pairs. InstructBLIP performs visual language command tuning based on the pre-trained BLIP-2 model, and Q-Former is trained on various datasets organized in a command-tuned format. mPLUG-Owl introduces a two-stage training strategy: first pre-train the visual part, and then use LoRA to fine-tune the large language model LLaMA based on instruction data from different sources.
#Despite the above-mentioned progress in VLM, there is still a need to use cross-modal capabilities when computing resources are limited. Gemini surpasses sota on a range of multi-modal benchmarks and introduces mobile-grade VLM with 1.8B and 3.25B parameters for low-memory devices. And Gemini also uses common compression techniques such as distillation and quantization. The goal of this paper is to build the first open, mobile-grade VLM, trained using public datasets and available technologies for visual perception and reasoning, and tailored for resource-constrained platforms. The contributions of this article are as follows:
- This article proposes MobileVLM, which is a full-stack transformation of a multi-modal visual language model customized for mobile scenarios. . According to the authors, this is the first visual language model to deliver detailed, reproducible, and powerful performance from scratch. Through controlled and open source data sets, researchers have established a set of high-performance basic language models and multi-modal models.
- This paper conducts extensive ablation experiments on the design of visual encoders and systematically evaluates the performance sensitivity of VLMs to various training paradigms, input resolutions, and model sizes.
- This paper designs an efficient mapping network between visual features and text features, which can better align multi-modal features while reducing reasoning consumption.
- The model designed in this article can run efficiently on low-power mobile devices, with a measured speed of 21.5 tokens/s on Qualcomm’s mobile CPU and 65.5-inch processor.
- MobileVLM performs equally well on benchmarks as a large number of multi-modal large models, proving its application potential in many practical tasks. While this article focuses on edge scenarios, MobileVLM outperforms many state-of-the-art VLMs that can only be supported by powerful GPUs in the cloud.
##MobileVLM
Overall Architecture Design
Considering the main goal of achieving efficient visual perception and reasoning for resource-limited edge devices, the researchers designed the overall architecture of MobileVLM, as shown in Figure 1 , the model consists of three components: 1) a visual encoder, 2) a customized LLM edge device (MobileLLaMA), and 3) an efficient mapping network (referred to in the paper as "Lightweight Downsampling Mapping", LDP) for alignment Visual and textual space. Taking the image
as input, the visual encoder F_enc extracts the visual embedding
for image perception, where N_v = HW/P^2 represents the number of image blocks and D_v represents the hidden layer size of the visual embedding. In order to alleviate the efficiency problem of processing image tokens, the researchers designed a lightweight mapping network P for visual feature compression and visual-text modal alignment. It transforms f into the word embedding space and provides appropriate input dimensions for the subsequent language model, as follows:

In this way, the tokens of the image# are obtained ##And text tokens
, where N_t represents the number of text tokens, and D_t represents the size of the word embedding space. In the current MLLM design paradigm, LLM has the largest amount of calculation and memory consumption. In view of this, this article tailors a series of inference-friendly LLM for mobile applications, which has considerable advantages in speed and can perform autoregressive methods. Predict multi-modal input
, where L represents the length of the output tokens. This process can be expressed as
. 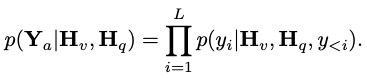
According to Section 5.1 of the original text For the empirical analysis, the researchers used the pre-trained CLIP ViT-L/14 with a resolution of 336×336 as the visual encoder F_enc. The Visual Transformer (ViT) divides the image into image blocks of uniform size and performs a linear embedding on each image block. After subsequent integration with the positional encoding, the resulting vector sequence is fed into the regular transform encoder. Typically, tokens used for classification will be added to the sequence for subsequent classification tasks.
For the language model, this article reduces the size of LLaMA to facilitate Deployment, that is, the model proposed in this paper can seamlessly support almost all popular inference frameworks. In addition, the researchers also evaluated the model latency on edge devices to select an appropriate model architecture. Neural Architecture Search (NAS) is a good choice, but currently researchers have not immediately applied it to current models. Table 2 shows the detailed settings of this paper’s architecture.
Specifically, this article uses the sentence piece tokenizer in LLaMA2 with a vocabulary size of 32000 and trains the embedding layer from scratch. This will facilitate subsequent distillation. Due to limited resources, the context length used by all models in the pre-training stage is 2k. However, as described in "Extending context window of large language models via positional interpolation", the context window during inference can be further extended to 8k. Detailed settings for other components are as follows.
- Apply RoPE to inject location information.
- Apply pre-normalization to stabilize training. Specifically, this paper uses RMSNorm instead of layer normalization, and the MLP expansion ratio uses 8/3 instead of 4.
- Use SwiGLU activation function instead of GELU.
Efficient mapping networkThe mapping network between the visual encoder and the language model is crucial for aligning multi-modal features. There are two existing modes: Q-Former and MLP projection. Q-Former explicitly controls the number of visual tokens included in each query to force the extraction of the most relevant visual information. However, this method inevitably loses the spatial location information of tokens and has a slow convergence speed. Additionally, it is not efficient for inference on edge devices. In contrast, MLP preserves spatial information but often contains useless tokens such as background. For an image with a patch size of P, N_v = HW/P^2 visual tokens need to be injected into the LLM, which greatly reduces the overall inference speed. Inspired by ViT’s conditional position coding algorithm CPVT, researchers use convolutions to enhance position information and encourage local interactions of visual encoders. Specifically, we investigated mobile-friendly operations based on deep convolutions (the simplest form of PEG) that are both efficient and well supported by a variety of edge devices. In order to preserve spatial information and minimize computational costs, this article uses convolution with a stride of 2, thereby reducing the number of visual tokens by 75%. This design greatly improves the overall inference speed. However, experimental results show that reducing the number of token samples will seriously reduce the performance of downstream tasks such as OCR. To mitigate this effect, the researchers designed a more powerful network to replace a single PEG. The detailed architecture of an efficient mapping network, called Lightweight Downsampling Mapping (LDP), is shown in Figure 2. Notably, this mapping network contains fewer than 20 million parameters and runs about 81 times faster than the visual encoder.
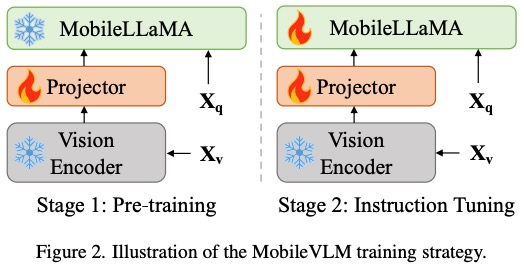
This article uses "Layer Normalization" instead of "Batch Normalization" so that training is not affected by batch size. Formally, LDP (denoted as P) takes as input a visual embedding
and outputs an efficiently extracted and aligned visual token
. The formula is as follows: 
Evaluation results of MobileLLaMAIn Table 3, the researcher evaluated the results proposed in this article on two standard natural language benchmarks. The model was extensively evaluated on two benchmarks targeting language understanding and commonsense reasoning respectively. In the evaluation of the former, this article uses the Language Model Evaluation Harness. Experimental results show that MobileLLaMA 1.4B is on par with the latest open source models such as TinyLLaMA 1.1B, Galactica 1.3B, OPT 1.3B and Pythia 1.4B. It is worth noting that MobileLLaMA 1.4B outperforms TinyLLaMA 1.1B, which is trained on 2T level tokens and is twice as fast as MobileLLaMA 1.4B. At the 3B level, MobileLLaMA 2.7B also shows comparable performance to INCITE 3B (V1) and OpenLLaMA 3B (V1), as shown in Table 5. On the Snapdragon 888 CPU, MobileLLaMA 2.7B is about 40% faster than OpenLLaMA 3B. 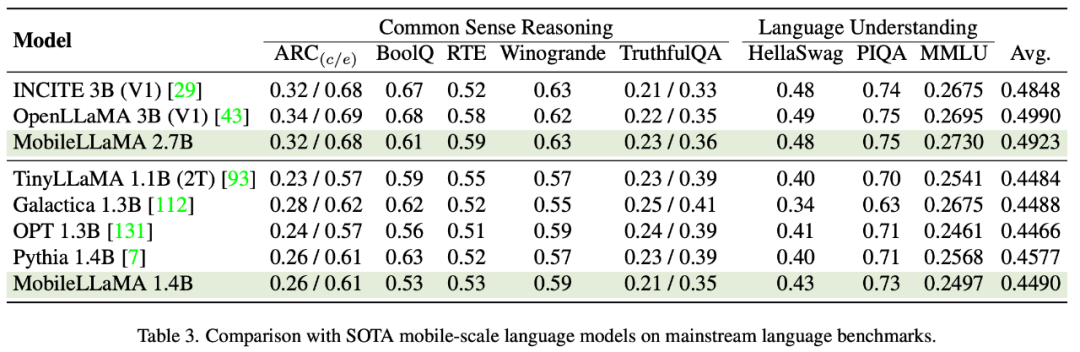
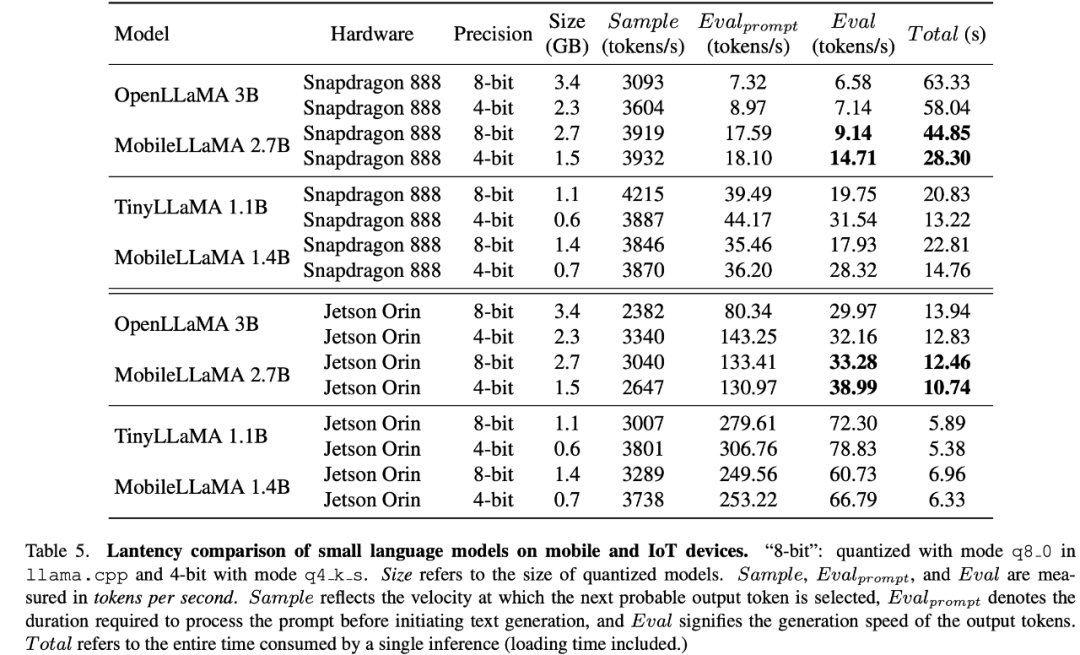
This paper evaluates the multi-modal performance of LLaVA on GQA, ScienceQA, TextVQA, POPE and MME. In addition, this paper also conducts a comprehensive comparison using MMBench. As shown in Table 4, MobileVLM achieves competitive performance despite reduced parameters and limited training data. In some cases, its metrics even outperform previous state-of-the-art multi-modal visual language models. 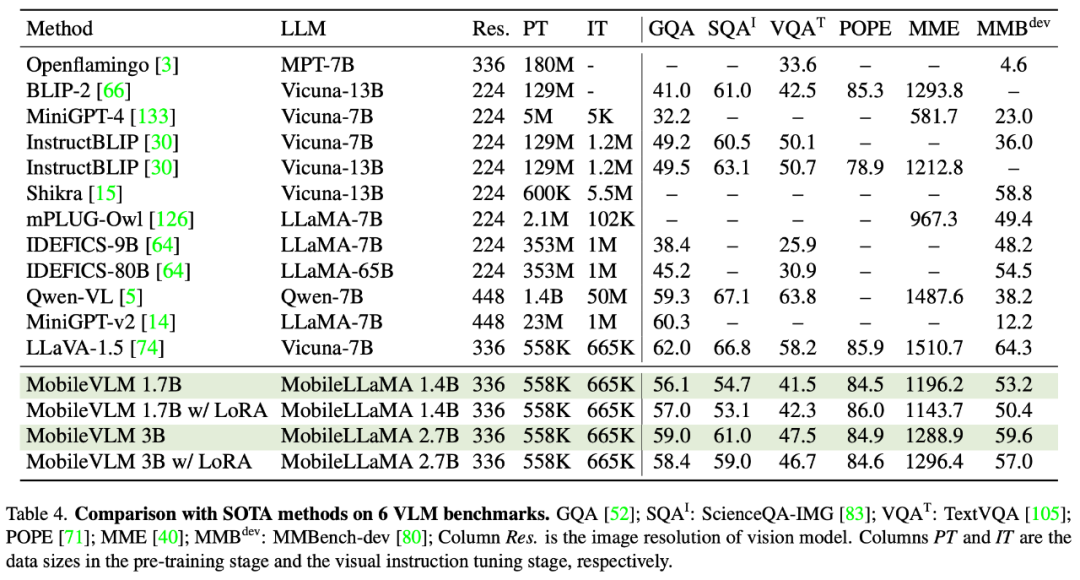
##Low-Rank Adaptation (LoRA) can be used with fewer trainable parameters Achieve the same or even better performance than fully fine-tuned LLM. This paper conducts an empirical study of this practice to validate its multimodal performance. Specifically, during the VLM visual instruction adjustment phase, this paper freezes all LLM parameters except the LoRA matrix. In MobileLLaMA 1.4B and MobileLLaMA 2.7B, the updated parameters are only 8.87% and 7.41% of the full LLM, respectively. For LoRA, this article sets lora_r to 128 and lora_α to 256. The results are shown in Table 4. It can be seen that MobileVLM with LoRA achieves comparable performance to full fine-tuning on 6 benchmarks, which is consistent with the results of LoRA.
Latency Testing on Mobile DevicesResearch The authors evaluated the inference latency of MobileLLaMA and MobileVLM on Realme GT mobile phones and NVIDIA Jetson AGX Orin platform. The phone is powered by Snapdragon 888 SoC and 8GB of RAM, which delivers 26 TOPS of computing power. Orin comes with 32GB of memory and delivers an astonishing 275 TOPS of computing power. It uses CUDA version 11.4 and supports the latest parallel computing technology for improved performance.
##Visual backbone network
In Table 7, the researchers compared the multi-modal performance at different scales and different numbers of visual tokens. All experiments used CLIP ViT as the visual encoder. 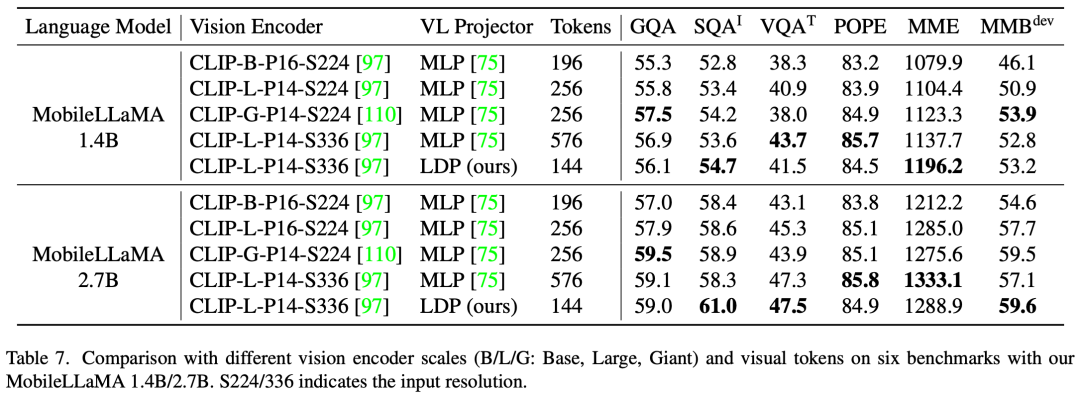
VL mapping network
Due to feature interaction and token Both interactions are beneficial. The researchers used depth convolution for the former and point convolution for the latter. Table 9 shows the performance of various VL mapped networks. Row 1 in Table 9 is the module used in LLaVA, which only transforms the feature space through two linear layers. Line 2 adds a DW (depthwise) convolution before each PW (pointwise) for token interaction, which uses 2x downsampling with a stride of 2. Adding two front-end PW layers will bring more feature-level interactions, thus making up for the performance loss caused by the reduction of tokens. Lines 4 and 5 show that adding more parameters does not achieve the desired effect. Lines 4 and 6 show that downsampling tokens at the end of the mapping network has a positive effect. 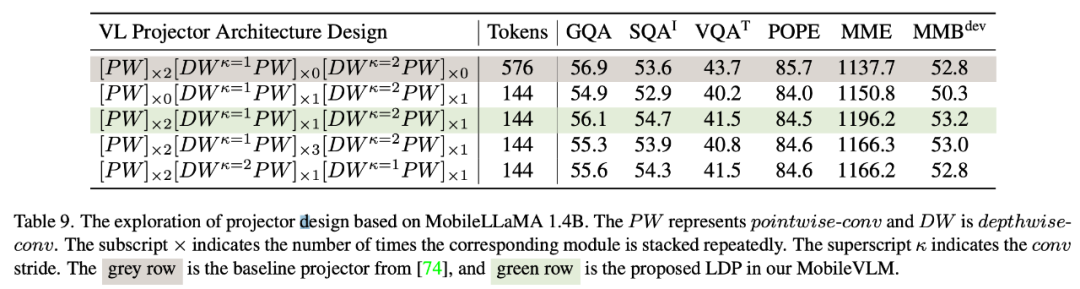
Visual resolution and number of tokens
Since the number of visual tokens directly affects the entire For modal model inference speed, this paper compares two design options: reducing the input resolution (RIR) and using a lightweight downsampling projector (LDP). Quantitative analysis of SFT
at Vicuna fine-tuned on LLaMA is widely used for large multimodal models. Table 10 compares two common SFT paradigms, Alpaca and Vicuna. The researchers found that the scores of SQA, VQA, MME, and MMBench all improved significantly. This shows that fine-tuning large language models using data from ShareGPT in Vicuna conversational mode ultimately yields the best performance. In order to better integrate SFT's prompt format with the training of downstream tasks, this paper removes the conversation mode on MobileVLM and finds that vicunav1 performs best. 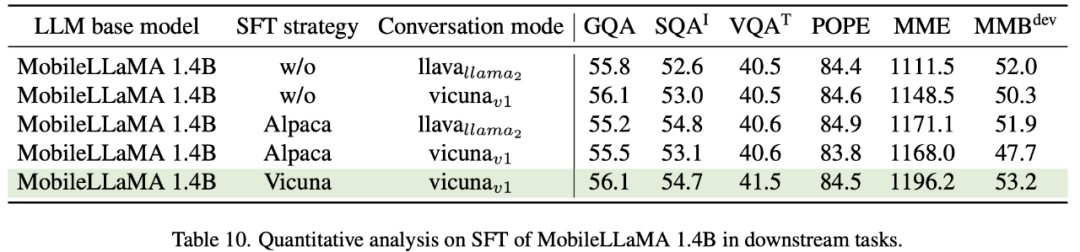
Conclusion
In short, MobileVLM is a suite of tools designed for mobile and An efficient and powerful mobile visual language model customized for IoT devices. This paper resets the language model and visual mapping network. The researchers conducted extensive experiments to select an appropriate visual backbone network, design an efficient mapping network, and enhance model capabilities through training solutions such as language model SFT (a two-stage training strategy including pre-training and instruction adjustment) and LoRA fine-tuning. . Researchers rigorously evaluated the performance of MobileVLM on mainstream VLM benchmarks. MobileVLM also shows unprecedented speeds on typical mobile and IoT devices. The researchers believe that MobileVLM will open up new possibilities for a wide range of applications such as multi-modal assistants deployed on mobile devices or autonomous vehicles, as well as broader artificial intelligence robots. The above is the detailed content of Meituan, Zhejiang University and others cooperate to create a full-process mobile multi-modal large model MobileVLM, which can run in real time and uses the Snapdragon 888 processor. For more information, please follow other related articles on the PHP Chinese website!