Ten indicators of machine learning model performance
Although large models are very powerful, solving practical problems does not necessarily rely entirely on large models. A less precise analogy to explain physical phenomena in reality without necessarily using quantum mechanics. For some relatively simple problems, perhaps a statistical distribution will suffice. For machine learning, it goes without saying that deep learning and neural networks are necessary. The key is to clarify the boundaries of the problem.
So how to evaluate the performance of a machine learning model when using ML to solve relatively simple problems? Here are 10 relatively commonly used evaluation indicators, hoping to be helpful to industry and research students.
1. Accuracy
Accuracy is a basic evaluation index in the field of machine learning and is usually used to quickly understand the performance of the model. Accuracy provides an intuitive way to measure a model's accuracy by simply calculating the ratio of the number of instances correctly predicted by the model to the total number of instances in the dataset.
Picture
However, accuracy, as an evaluation index, may be inadequate when dealing with imbalanced data sets. An imbalanced data set refers to a data set in which the number of instances of a certain category significantly exceeds that of other categories. In this case, the model may tend to predict a larger number of categories, resulting in falsely high accuracy.
Furthermore, accuracy provides no information about false positives and false negatives. A false positive is when the model incorrectly predicts a negative instance as a positive instance, while a false negative is when the model incorrectly predicts a positive instance as a negative instance. When evaluating model performance, it is important to distinguish between false positives and false negatives, as they have different effects on the performance of the model.
In summary, although accuracy is a simple and easy-to-understand evaluation metric, when dealing with imbalanced data sets, we need to be more careful in interpreting accuracy results.
2. Accuracy
Accuracy is an important evaluation index, which focuses on measuring the model's prediction accuracy for positive samples. Unlike accuracy, precision calculates the proportion of instances that are actually positive among the instances predicted by the model to be positive. In other words, accuracy answers the question: "When the model predicts an instance to be positive, what is the probability that this prediction is accurate?" A high-precision model means that when it predicts an instance to be positive, , this instance is very likely to be indeed a positive sample.
Picture
In some applications, such as medical diagnosis or fraud detection, the accuracy of the model is particularly important. In these scenarios, the consequences of false positives (i.e., incorrectly predicting negative samples as positive samples) can be very serious. For example, in medical diagnosis, a false-positive diagnosis may lead to unnecessary treatment or examination, causing unnecessary psychological and physical stress to the patient. In fraud detection, false positives can lead to innocent users being incorrectly labeled as fraudulent actors, impacting the user experience and the company's reputation.
Therefore, in these applications, it is crucial to ensure that the model has high accuracy. Only by improving accuracy can we reduce the risk of false positives and thus reduce the negative impact of false positives.
3. Recall rate
Recall rate is an important evaluation index, used to measure the model's ability to correctly predict all actual positive samples. Specifically, recall is calculated as the ratio of instances predicted by the model to be true positives to the total number of actual positive examples. This metric answers the question: "How many of the actual positive examples did the model correctly predict?"
Unlike precision, recall focuses on the model's ability to recall actual positive examples. . Even if the model has a low prediction probability for a certain positive sample, as long as the sample is actually a positive sample and is correctly predicted as a positive sample by the model, then this prediction will be included in the calculation of the recall rate. Therefore, recall is more concerned with whether the model is able to find as many positive samples as possible, not just those with higher predicted probabilities.
Picture
In some application scenarios, the importance of recall rate is particularly prominent. For example, in disease detection, if the model misses the actual sick patients, it may cause delays and deterioration of the disease, and bring serious consequences to the patients. For another example, in customer churn prediction, if the model does not correctly identify customers who are likely to churn, the company may lose the opportunity to take retention measures, thereby losing important customers.
Therefore, in these scenarios, recall rate becomes a crucial indicator. A model with high recall is better able to find actual positive samples, reducing the risk of omissions and thus avoiding possible serious consequences.
4. F1 score
F1 score is a comprehensive evaluation index that aims to find a balance between precision and recall. It is actually the harmonic mean of precision and recall, combining these two metrics into a single score, thus providing a way of evaluation that takes into account both false positives and false negatives.
Picture
In many practical applications, we often need to make a trade-off between precision and recall. Precision focuses on the correctness of the model's predictions, while recall focuses on whether the model is able to find all actual positive samples. However, overemphasizing one metric can often harm the performance of the other. For example, to improve recall, a model may increase predictions for positive samples, but this may also increase the number of false positives, thereby reducing accuracy.
F1 scoring is designed to solve this problem. It takes precision and recall into consideration, preventing us from sacrificing one metric in order to optimize another. By calculating the harmonic mean of precision and recall, the F1 score strikes a balance between the two, allowing us to evaluate a model's performance without favoring either side.
Therefore, the F1 score is a very useful tool when you need a metric that takes precision and recall into consideration, and don't want to favor one metric over the other. It provides a single score that simplifies the process of evaluating model performance and helps us better understand how the model performs in real-world applications.
5. ROC-AUC
ROC-AUC is a performance measurement method widely used in binary classification problems. It measures the area under the ROC curve, which depicts the relationship between the true positive rate (also called sensitivity or recall) and the false positive rate at different thresholds.
Picture
The ROC curve provides an intuitive way to observe the performance of the model under various threshold settings. By changing the threshold, we can adjust the true positive rate and false positive rate of the model to obtain different classification results. The closer the ROC curve is to the upper left corner, the better the model's performance in distinguishing positive and negative samples.
The AUC (area under the curve) provides a quantitative indicator to evaluate the discrimination ability of the model. The AUC value is between 0 and 1. The closer it is to 1, the stronger the discrimination ability of the model. A high AUC score means that the model can distinguish between positive samples and negative samples well, that is, the model's predicted probability for positive samples is higher than the predicted probability for negative samples.
Therefore, ROC-AUC is a very useful metric when we want to evaluate a model's ability to distinguish between classes. Compared with other indicators, ROC-AUC has some unique advantages. It is not affected by threshold selection and can comprehensively consider the performance of the model under various thresholds. In addition, ROC-AUC is relatively robust to class imbalance problems and can still give meaningful evaluation results even when the number of positive and negative samples is imbalanced.
ROC-AUC is a very valuable performance measure, especially suitable for binary classification problems. By observing and comparing the ROC-AUC scores of different models, we can gain a more comprehensive understanding of the model's performance and select the model with better discrimination ability.
6. PR-AUC
PR-AUC (area under the precision-recall curve) is a performance measurement method that is similar to ROC-AUC, but the focus is slightly different. PR-AUC measures the area under the precision-recall curve, which depicts the relationship between precision and recall at different thresholds.
Picture
Compared with ROC-AUC, PR-AUC pays more attention to the trade-off between precision and recall. Precision measures the proportion of instances that the model predicts to be positive that are actually positive, while recall measures the proportion of instances that the model correctly predicts to be positive among all instances that actually are positive. The trade-off between precision and recall is particularly important in imbalanced data sets, or when false positives are more of a concern than false negatives.
In an imbalanced data set, the number of samples in one category may far exceed the number of samples in another category. In this case, ROC-AUC may not accurately reflect the performance of the model because it mainly focuses on the relationship between the true positive rate and the false positive rate without directly considering the class imbalance. In contrast, PR-AUC more comprehensively evaluates the performance of the model through the trade-off between precision and recall, and can better reflect the effect of the model on imbalanced data sets.
Additionally, PR-AUC is also a more appropriate metric when false positives are more of a concern than false negatives. Because in some application scenarios, incorrectly predicting negative samples as positive samples (false positives) may bring greater losses or negative impacts. For example, in medical diagnosis, incorrectly diagnosing a healthy person as a diseased person can lead to unnecessary treatment and anxiety. In this case, we would prefer the model to have high accuracy to reduce the number of false positives.
To sum up, PR-AUC is a performance measurement method suitable for imbalanced data sets or scenarios where false positives are concerned. It can help us better understand the trade-off between precision and recall of models and choose an appropriate model to meet actual needs.
7. FPR/TNR
The False Positive Rate (FPR) is an important metric that measures the proportion of samples that the model incorrectly predicts as positive among all actual negative samples. It is a complementary indicator of specificity and corresponds to the true negative rate (TNR). FPR becomes a key element when we want to evaluate a model's ability to avoid false positives. False positives can lead to unnecessary worry or wasted resources, so understanding the FPR of a model is crucial to determine its reliability in real-world applications. By reducing the FPR, we can improve the precision and accuracy of the model, ensuring that positive predictions are only issued when positive samples actually exist.
Picture
On the other hand, the true negative rate (TNR), also known as specificity, is a measure of how well a model correctly identifies negative samples. index. It calculates the proportion of instances predicted by the model to be true negatives to the actual total negatives. When evaluating a model, we often focus on the model's ability to identify positive samples, but equally important is the model's performance in identifying negative samples. A high TNR means that the model can accurately identify negative samples, that is, among the instances that are actually negative samples, the model predicts a higher proportion of negative samples. This is crucial to avoid false positives and improve the overall performance of the model.
8. Matthews Correlation Coefficient (MCC)
MCC (Matthews Correlation Coefficient) is a measure used in binary classification problems, which provides us with a comprehensive consideration The relationship between true positives, true negatives, false positives and false negatives is evaluated. Compared with other measurement methods, the advantage of MCC is that it is a single value ranging from -1 to 1, where -1 means that the model's prediction is completely inconsistent with the actual result, and 1 means that the model's prediction is completely consistent with the actual result. .
Picture
More importantly, MCC provides a balanced way to measure the quality of binary classification. In binary classification problems, we usually focus on the model's ability to identify positive and negative samples, while MCC considers both aspects. It focuses not only on the model's ability to correctly predict positive samples (i.e., true positives), but also on the model's ability to correctly predict negative samples (i.e., true negatives). At the same time, MCC also takes false positives and false negatives into consideration to more comprehensively evaluate the performance of the model.
In practical applications, MCC is particularly suitable for handling imbalanced data sets. Because in an imbalanced data set, the number of samples in one category is much larger than that of another category, this often causes the model to be biased towards predicting the category with a larger number. However, MCC is able to consider all four metrics (true positives, true negatives, false positives, and false negatives) in a balanced manner, so it can generally provide a more accurate and comprehensive performance evaluation for imbalanced data sets.
In general, MCC is a powerful and comprehensive binary classification performance measurement tool. It not only takes into account all possible prediction results, but also provides an intuitive, well-defined numerical value to measure the consistency between predictions and actual results. Whether on balanced or unbalanced data sets, MCC is a useful metric that can help us understand the performance of the model more deeply.
9. Cross entropy loss
Cross entropy loss is a commonly used performance metric in classification problems, especially when the output of the model is a probability value. This loss function is used to quantify the difference between the probability distribution predicted by the model and the actual label distribution.
Picture
In classification problems, the goal of the model is usually to predict the probability that a sample belongs to different categories. Cross-entropy loss is used to evaluate the consistency between model predicted probabilities and actual binary results. It derives the loss value by taking the logarithm of the predicted probability and comparing it with the actual label. Therefore, cross-entropy loss is also called logarithmic loss.
The advantage of cross-entropy loss is that it can well measure the prediction accuracy of the model for the probability distribution. When the predicted probability distribution of the model is similar to the actual label distribution, the value of cross-entropy loss is low; conversely, when the predicted probability distribution is significantly different from the actual label distribution, the value of cross-entropy loss is high. Therefore, a lower cross-entropy loss value means that the model's predictions are more accurate, that is, the model has better calibration performance.
In practical applications, we usually pursue lower cross-entropy loss values, because this means that the model’s predictions for classification problems are more accurate and reliable. By optimizing the cross-entropy loss, we can improve the performance of the model and make it have better generalization ability in practical applications. Therefore, cross-entropy loss is one of the important indicators to evaluate the performance of a classification model. It can help us further understand the prediction accuracy of the model and whether further optimization of the parameters and structure of the model is needed.
10. Cohen's kappa coefficient
Cohen's kappa coefficient is a statistical tool used to measure the consistency between model predictions and actual labels. It is especially suitable for the evaluation of classification tasks. Compared with other measurement methods, it not only calculates the simple agreement between model predictions and actual labels, but also corrects for the agreement that may occur by chance, thus providing a more accurate and reliable evaluation result.
In practical applications, especially when multiple raters are involved in classifying the same set of samples, Cohen's kappa coefficient is very useful. In this case, we not only need to focus on the consistency of model predictions with actual labels, but also need to consider the consistency between different raters. Because if there is significant inconsistency between raters, the evaluation results of the model performance may be affected by the subjectivity of the raters, resulting in inaccurate evaluation results.
By using Cohen's kappa coefficient, this consistency that may occur by chance can be corrected for, allowing for a more accurate assessment of model performance. Specifically, it calculates a value between -1 and 1, where 1 represents perfect consistency, -1 represents complete inconsistency, and 0 represents random consistency. Therefore, a higher Kappa value means that the agreement between the model predictions and the actual labels exceeds the agreement expected by chance, which indicates that the model has better performance.
Picture
Cohen’s kappa coefficient can help us more accurately evaluate the gap between model predictions and actual labels in classification tasks consistency, while correcting for consistency that may occur by chance. It is especially important in scenarios involving multiple raters, as it can provide a more objective and accurate assessment.
Summary
There are many indicators for machine learning model evaluation. This article gives some of the main indicators:
- Accuracy: correctly predicted The ratio of the number of samples to the total number of samples.
- Precision: The proportion of True Positive (TP) samples to all predicted positive (TP and FP) samples, reflecting the model's ability to identify positive samples.
- Recall: The proportion of True Positive (TP) samples to all true positive (TP and FN) samples, reflecting the model's ability to discover positive samples.
- F1 value: The harmonic average of precision and recall, taking into account both precision and recall.
- ROC-AUC: The area under the ROC curve. The ROC curve is a function of the true positive rate (True Positive Rate, TPR) and the false positive rate (False Positive Rate, FPR). The larger the AUC, the better the classification performance of the model.
- PR-AUC: The area under the precision-recall curve, which focuses on the trade-off between precision and recall and is more suitable for imbalanced data sets.
- FPR/TNR: FPR measures the model’s ability to report false positives, and TNR measures the model’s ability to correctly identify negative samples.
- Cross entropy loss: used to evaluate the difference between the model's predicted probability and the actual label. Lower values indicate better model calibration and accuracy.
- Matthews Correlation Coefficient (MCC): A metric that takes into account the relationships between true positives, true negatives, false positives, and false negatives, providing a balanced measure of binary classification quality.
- Cohen's kappa: An important tool for evaluating model performance in classification tasks. It can accurately measure the consistency between predictions and labels and correct for accidental consistency, especially in multiple rater scenarios. Advantage.
Each of the above indicators has its own characteristics and is suitable for different problem scenarios. In practical applications, multiple indicators may need to be combined to comprehensively evaluate the performance of the model.
The above is the detailed content of Ten indicators of machine learning model performance. For more information, please follow other related articles on the PHP Chinese website!
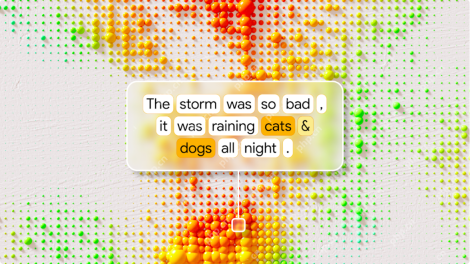
Exploring the Inner Workings of Language Models with Gemma Scope Understanding the complexities of AI language models is a significant challenge. Google's release of Gemma Scope, a comprehensive toolkit, offers researchers a powerful way to delve in
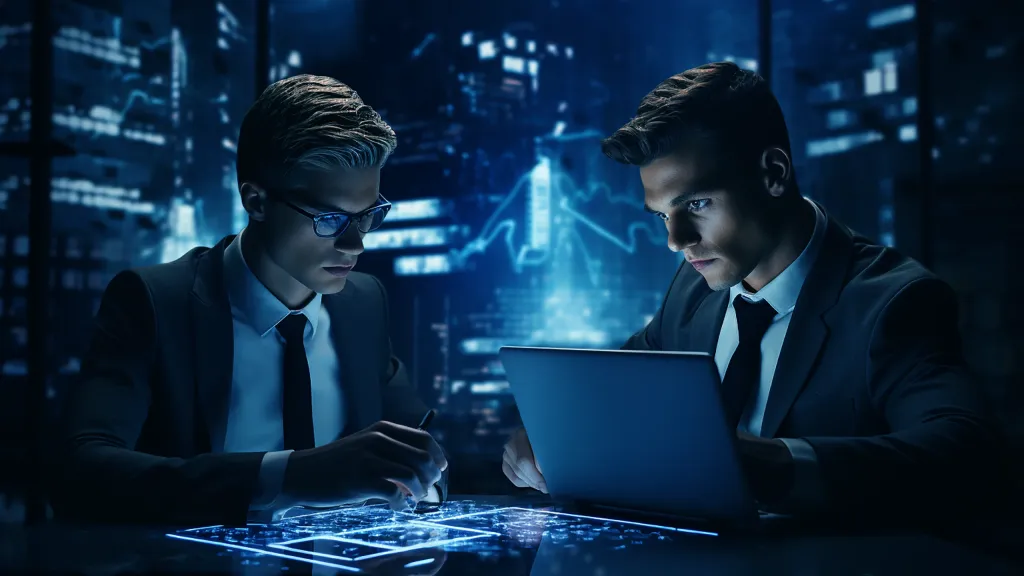
Unlocking Business Success: A Guide to Becoming a Business Intelligence Analyst Imagine transforming raw data into actionable insights that drive organizational growth. This is the power of a Business Intelligence (BI) Analyst – a crucial role in gu

SQL's ALTER TABLE Statement: Dynamically Adding Columns to Your Database In data management, SQL's adaptability is crucial. Need to adjust your database structure on the fly? The ALTER TABLE statement is your solution. This guide details adding colu
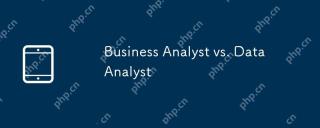
Introduction Imagine a bustling office where two professionals collaborate on a critical project. The business analyst focuses on the company's objectives, identifying areas for improvement, and ensuring strategic alignment with market trends. Simu
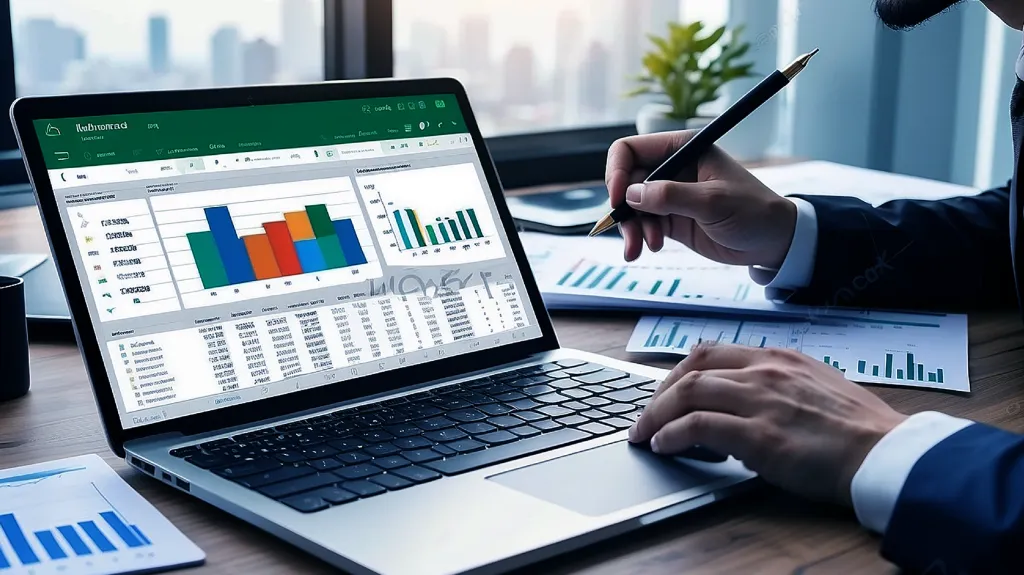
Excel data counting and analysis: detailed explanation of COUNT and COUNTA functions Accurate data counting and analysis are critical in Excel, especially when working with large data sets. Excel provides a variety of functions to achieve this, with the COUNT and COUNTA functions being key tools for counting the number of cells under different conditions. Although both functions are used to count cells, their design targets are targeted at different data types. Let's dig into the specific details of COUNT and COUNTA functions, highlight their unique features and differences, and learn how to apply them in data analysis. Overview of key points Understand COUNT and COU
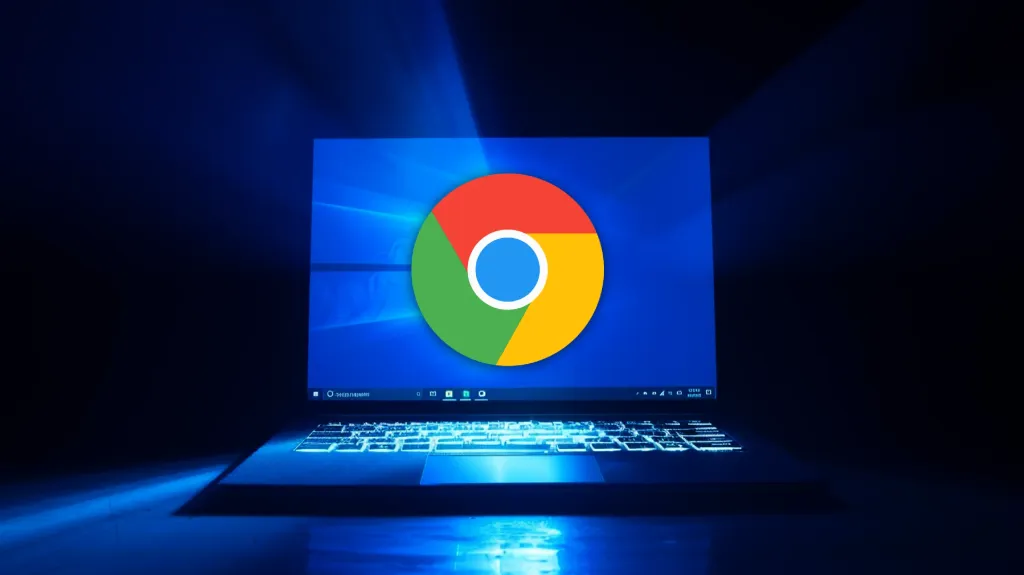
Google Chrome's AI Revolution: A Personalized and Efficient Browsing Experience Artificial Intelligence (AI) is rapidly transforming our daily lives, and Google Chrome is leading the charge in the web browsing arena. This article explores the exciti
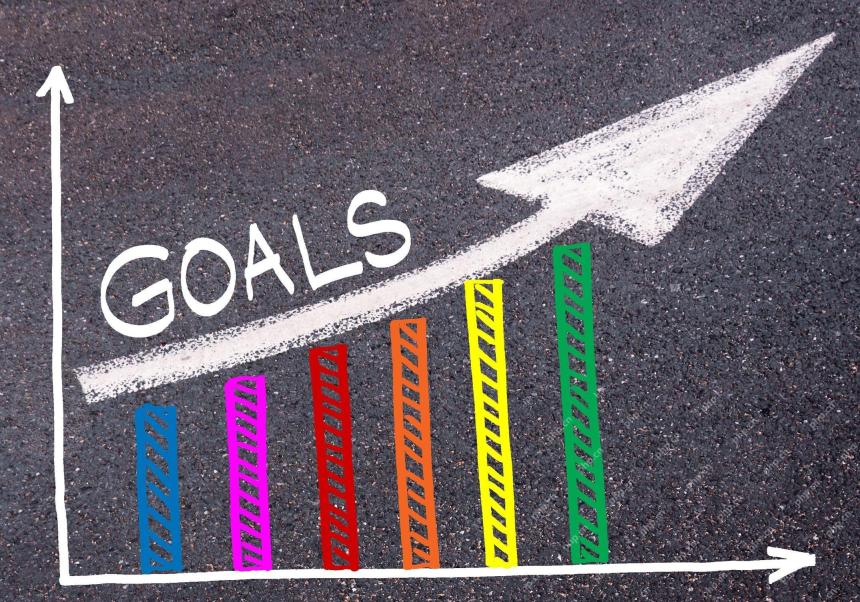
Reimagining Impact: The Quadruple Bottom Line For too long, the conversation has been dominated by a narrow view of AI’s impact, primarily focused on the bottom line of profit. However, a more holistic approach recognizes the interconnectedness of bu
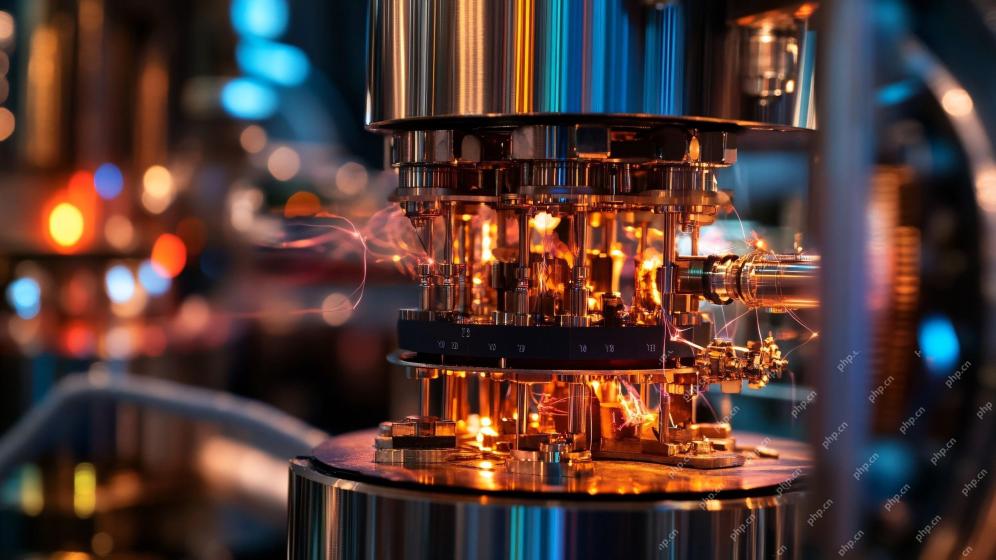
Things are moving steadily towards that point. The investment pouring into quantum service providers and startups shows that industry understands its significance. And a growing number of real-world use cases are emerging to demonstrate its value out


Hot AI Tools

Undresser.AI Undress
AI-powered app for creating realistic nude photos

AI Clothes Remover
Online AI tool for removing clothes from photos.

Undress AI Tool
Undress images for free

Clothoff.io
AI clothes remover

AI Hentai Generator
Generate AI Hentai for free.

Hot Article

Hot Tools

Zend Studio 13.0.1
Powerful PHP integrated development environment

SublimeText3 English version
Recommended: Win version, supports code prompts!
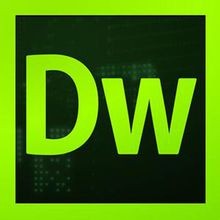
Dreamweaver CS6
Visual web development tools

MantisBT
Mantis is an easy-to-deploy web-based defect tracking tool designed to aid in product defect tracking. It requires PHP, MySQL and a web server. Check out our demo and hosting services.
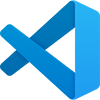
VSCode Windows 64-bit Download
A free and powerful IDE editor launched by Microsoft