


I recently read a recent study on pure visual surround perception on Arxiv. This research is based on the PETR series of methods and focuses on solving the pure visual perception problem of long-distance target detection, extending the perception range to 150 meters. The methods and results of this paper have great reference value for us, so I tried to interpret it
Original title: Far3D: Expanding the Horizon for Surround-view 3D Object Detection
Paper link: https://arxiv.org/abs/2308.09616
Author affiliation: Beijing Institute of Technology & Megvii Technology
Mission Background
Three-dimensional object detection plays an important role in understanding the three-dimensional scene of autonomous driving. Its purpose is to accurately locate and classify objects around the vehicle. Pure visual surround perception methods have the advantages of low cost and wide applicability, and have made significant progress. However, most of them focus on short-range sensing (for example, the sensing distance of nuScenes is about 50 meters), and the long-range detection field is less explored. Detecting distant objects is critical to maintaining a safe distance during actual driving, especially at high speeds or in complex road conditions.
Recently, significant progress has been made in 3D object detection from surround-view images, which can be deployed at low cost. However, most studies mainly focus on the short-range sensing range, and there are fewer studies on long-range detection. Directly extending existing methods to cover long distances will face challenges such as high computational cost and unstable convergence. To address these limitations, this paper proposes a new sparse query-based framework called Far3D.
Thesis Idea
According to the intermediate representation, existing look-around sensing methods can be roughly divided into two categories: methods based on BEV representation and methods based on sparse query representation. The method based on BEV representation requires a very large amount of calculation due to the need for intensive calculation of BEV features, making it difficult to extend to long-distance scenarios. The method based on sparse query representation will learn the global 3D query from the training data, the calculation amount is relatively small, and it has strong scalability. However, it also has some weaknesses. Although it can avoid the square growth of the number of queries, the global fixed query is not easy to adapt to dynamic scenarios, and targets are usually missed in long-distance detection
Figure 1: Performance comparison of 3D detection and 2D detection on the Argoverse 2 data set.
In long-range detection, methods based on sparse query representation have two main challenges.
- The first is poor recall performance. Due to the sparse distribution of queries in 3D space, only a small number of matching positive queries can be generated in long distance ranges. As shown in the figure above, the recall rate of 3D detection is lower, while the recall rate of existing 2D detection is much higher, leaving a clear performance gap between the two. Therefore, utilizing high-quality 2D object priors to improve 3D query is a promising method, which is beneficial to achieve precise positioning and comprehensive coverage of objects.
- Secondly, directly introducing 2D detection results to help 3D detection will face the problem of error propagation. As shown in the figure below, the two main sources are 1) object positioning error due to inaccurate depth prediction; 2) 3D position error in the frustum transformation increases with distance. These noisy queries will affect the stability of training and require effective denoising methods to optimize. Furthermore, during training, the model will show a tendency to overfit to densely packed close objects while ignoring sparsely distributed distant objects.
In order to deal with the above-mentioned problems, this article adopts the following design plan:
- In addition to the 3D global query learned from the data set, a 3D adaptive query generated from the 2D detection results is also introduced. Specifically, the 2D detector and depth prediction network are first used to obtain the 2D box and corresponding depth, and then projected into the 3D space through spatial transformation as the initialization of the 3D adaptive query.
- In order to adapt to the different scales of objects at different distances, Perspective-aware Aggergation is designed. It allows 3D query to interact with features of different scales, which is beneficial to feature capture of objects at different distances. For example, distant objects require large-resolution features, while close objects require different features. This design allows the model to adaptively interact with features.
- A strategy called Range-modulated 3D Denoising is designed to alleviate the problem of query error propagation and slow convergence. Considering that query regression difficulties at different distances are different, the noisy query is adjusted according to the distance and scale of the real box. Input multiple sets of noisy queries near GT into the decoder to reconstruct the 3D real box (for positive samples) and discard negative samples respectively.
Main contributions
- This paper proposes a new sparse query-based detection framework, which uses high-quality 2D object prior to generate 3D adaptive query, thereby expanding the perception range of 3D detection.
- This article designs a Perspective-aware Aggregation module, which aggregates visual features from different scales and perspectives, and a 3D Denoising strategy based on target distance to solve query error propagation and framework convergence problems.
- Experimental results on the long-range Argoverse 2 dataset show that Far3D surpasses previous look-around methods and outperforms several lidar-based methods. And its generality is verified on the nuScenes dataset.
Model design
Far3D process overview:
- Input the surround image into the backbone network and FPN layer, encode the 2D image features and encode them with camera parameters.
- Utilizes 2D detectors and depth prediction networks to generate reliable 2D object boxes and their corresponding depths, which are then projected into 3D space through camera transformations.
- The generated 3D adaptive query is combined with the initial 3D global query and iteratively regressed by the decoder layer to predict the 3D object frame. Furthermore, the model can implement time series modeling through long-term query propagation.
Perspective-aware Aggregation:
In order to introduce multi-scale features to the long-range detection model, this article applies 3D spatial deformable attention. It first performs offset sampling near the 3D position corresponding to the query, and then aggregates image features through 3D-2D view transformation. The advantage of this method instead of global attention in the PETR series is that the computational complexity can be significantly reduced. Specifically, for each query's reference point in 3D space, the model learns M sampling offsets around it and projects these offset points into different 2D view features.
Thereafter, the 3D query interacts with the projected sampled features. In this way, various features from different perspectives and scales will be brought together into a three-dimensional query by considering their relative importance.
Range-modulated 3D Denoising:
3D queries with different distances have different regression difficulties, which is different from existing 2D Denoising methods (such as DN-DETR, 2D queries that are usually treated equally). The difference in difficulty comes from query matching density and error propagation. On the one hand, the query matching degree corresponding to distant objects is lower than that of nearby objects. On the other hand, when introducing 2D priors in 3D adaptive query, small errors in 2D object boxes will be amplified, not to mention that this effect will increase as the object distance increases. Therefore, some queries near the GT box can be regarded as positive queries, while others with obvious deviations should be regarded as negative queries. This paper proposes a 3D Denoising method that aims to optimize those positive samples and directly discard negative samples.
Specifically, the authors build a GT-based noisy query by adding groups of positive and negative samples simultaneously. For both types, random noise is applied based on the location and size of the object to facilitate denoising learning in long-range perception. Specifically, positive samples are random points within the 3D box, while negative samples impose a larger offset on the GT, and the offset range changes with the distance of the object. This method can simulate noisy candidate positive samples and false positive samples during the training process
Experimental results
Far3D was achieved on Argoverse 2 with a 150m sensing range Highest performance. And after the model is scaled up, it can achieve the performance of several Lidar-based methods, demonstrating the potential of pure visual methods.
In order to verify the generalization performance, the author also conducted experiments on the nuScenes data set, showing that it achieved SoTA performance on both the validation set and the test set.
After ablation experiments, we came to the following conclusion: 3D adaptive query, perspective-aware aggregation and range-adjusted 3D noise reduction each have a certain gain
Thoughts on the paper
Q: What is the novelty of this article?
A: The main novelty is to solve the problem of perception of long-distance scenes . There are many problems in extending existing methods to long-distance scenarios, including computational costs and convergence difficulties. The authors of this article propose an efficient framework for this task. Although each module looks familiar individually, they all serve the detection of distant targets and have clear goals.
Q: Compared with BevFormer v2, what are the differences between MV2D?
A: MV2D mainly relies on 2D anchors to obtain corresponding features to bind 3D, but there is no explicit depth estimation, so the uncertainty will be relatively large for distant objects, and then it will be difficult to converge; BevFormer v2 mainly solves the domain gap between 2D backbone and 3D task scenes. Generally, the backbone pre-trained on 2D recognition tasks has insufficient ability to detect 3D scenes and does not explore problems in long-distance tasks.
Q: Can the timing be improved, such as query propagation plus feature propagation?
A: It is feasible in theory, but performance-efficiency tradeoff should be considered in practical applications.
Q: Are there any areas that need improvement?
A: Both long-tail issues and long-distance evaluation indicators deserve improvement. On a 26-class target like Argoverse 2, models do not perform well on long-tail classes and ultimately reduce average accuracy, which has not yet been explored. On the other hand, using unified metrics to evaluate distant and close objects may not be appropriate, which emphasizes the need for practical dynamic evaluation criteria that can be adapted to different scenarios in the real world.
Original link: https://mp.weixin.qq.com/s/xxaaYQsjuWzMI7PnSmuaWg
The above is the detailed content of AAAI2024: Far3D - Innovative idea of directly reaching 150m visual 3D target detection. For more information, please follow other related articles on the PHP Chinese website!
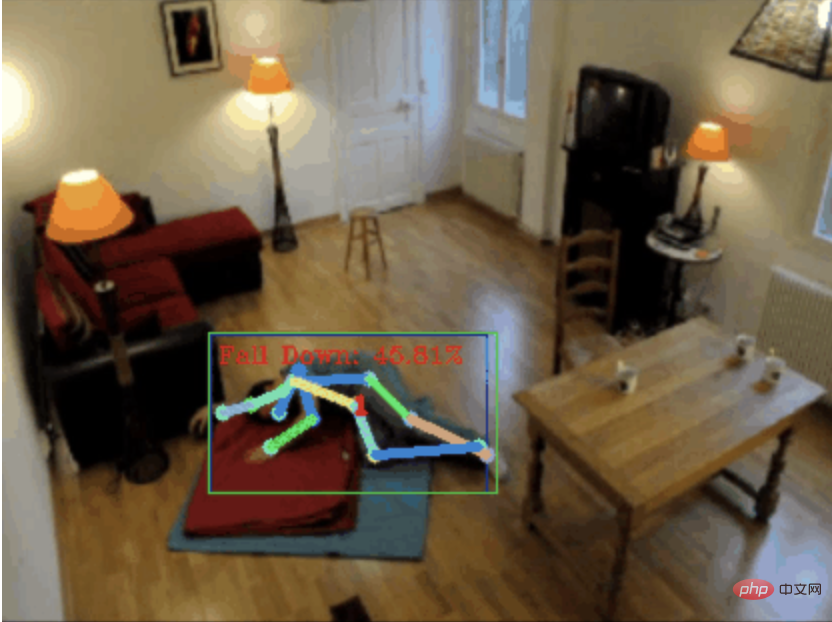
哈喽,大家好。今天给大家分享一个摔倒检测项目,准确地说是基于骨骼点的人体动作识别。大概分为三个步骤识别人体识别人体骨骼点动作分类项目源码已经打包好了,获取方式见文末。0. chatgpt首先,我们需要获取监控的视频流。这段代码比较固定,我们可以直接让chatgpt完成chatgpt写的这段代码是没有问题的,可以直接使用。但后面涉及到业务型任务,比如:用mediapipe识别人体骨骼点,chatgpt给出的代码是不对的。我觉得chatgpt可以作为一个工具箱,能独立于业务逻辑,都可以试着交给c
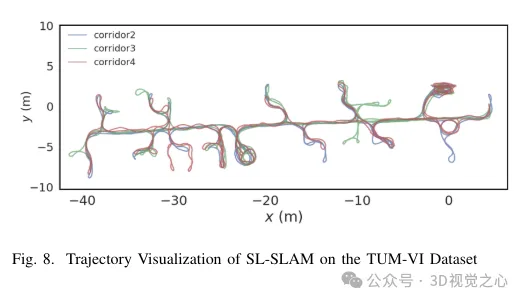
写在前面今天我们探讨下深度学习技术如何改善在复杂环境中基于视觉的SLAM(同时定位与地图构建)性能。通过将深度特征提取和深度匹配方法相结合,这里介绍了一种多功能的混合视觉SLAM系统,旨在提高在诸如低光条件、动态光照、弱纹理区域和严重抖动等挑战性场景中的适应性。我们的系统支持多种模式,包括拓展单目、立体、单目-惯性以及立体-惯性配置。除此之外,还分析了如何将视觉SLAM与深度学习方法相结合,以启发其他研究。通过在公共数据集和自采样数据上的广泛实验,展示了SL-SLAM在定位精度和跟踪鲁棒性方面优
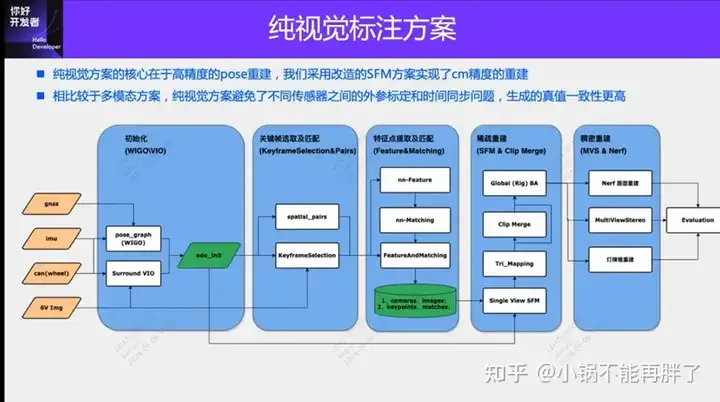
纯视觉的标注方案,主要是利用视觉加上一些GPS、IMU和轮速传感器的数据进行动态标注。当然面向量产场景的话,不一定非要是纯视觉,有一些量产的车辆里面,会有像固态雷达(AT128)这样的传感器。如果从量产的角度做数据闭环,把这些传感器都用上,可以有效地解决动态物体的标注问题。但是我们的方案里面,是没有固态雷达的。所以,我们就介绍这种最通用的量产标注方案。纯视觉的标注方案的核心在于高精度的pose重建。我们采用StructurefromMotion(SFM)的pose重建方案,来保证重建精度。但是传
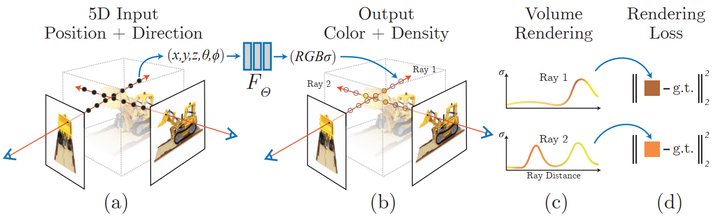
1介绍神经辐射场(NeRF)是深度学习和计算机视觉领域的一个相当新的范式。ECCV2020论文《NeRF:将场景表示为视图合成的神经辐射场》(该论文获得了最佳论文奖)中介绍了这项技术,该技术自此大受欢迎,迄今已获得近800次引用[1]。该方法标志着机器学习处理3D数据的传统方式发生了巨大变化。神经辐射场场景表示和可微分渲染过程:通过沿着相机射线采样5D坐标(位置和观看方向)来合成图像;将这些位置输入MLP以产生颜色和体积密度;并使用体积渲染技术将这些值合成图像;该渲染函数是可微分的,因此可以通过
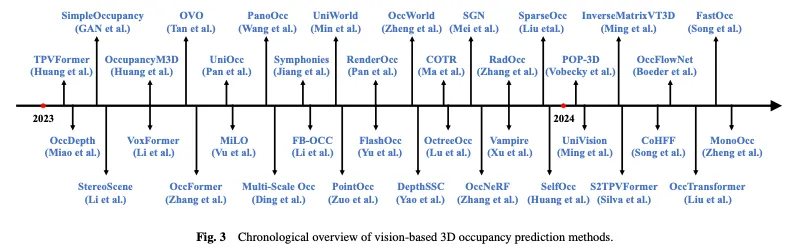
写在前面&笔者的个人理解近年来,自动驾驶因其在减轻驾驶员负担和提高驾驶安全方面的潜力而越来越受到关注。基于视觉的三维占用预测是一种新兴的感知任务,适用于具有成本效益且对自动驾驶安全全面调查的任务。尽管许多研究已经证明,与基于物体为中心的感知任务相比,3D占用预测工具具有更大的优势,但仍存在专门针对这一快速发展领域的综述。本文首先介绍了基于视觉的3D占用预测的背景,并讨论了这一任务中遇到的挑战。接下来,我们从特征增强、部署友好性和标签效率三个方面全面探讨了当前3D占用预测方法的现状和发展趋势。最后
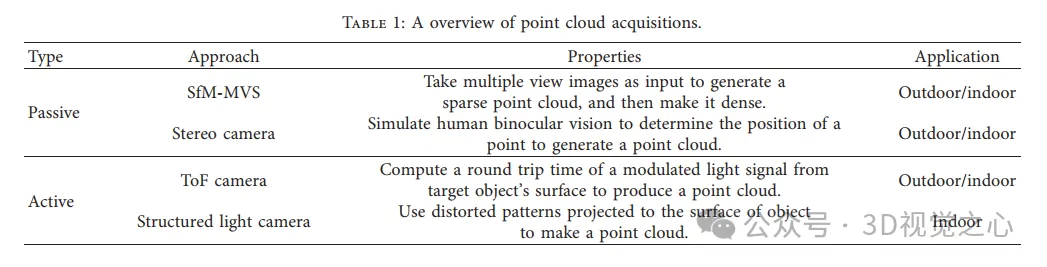
作为点集合的点云有望通过3D重建、工业检测和机器人操作中,在获取和生成物体的三维(3D)表面信息方面带来一场改变。最具挑战性但必不可少的过程是点云配准,即获得一个空间变换,该变换将在两个不同坐标中获得的两个点云对齐并匹配。这篇综述介绍了点云配准的概述和基本原理,对各种方法进行了系统的分类和比较,并解决了点云配准中存在的技术问题,试图为该领域以外的学术研究人员和工程师提供指导,并促进对点云配准统一愿景的讨论。点云获取的一般方式分为主动和被动方式,由传感器主动获取的点云为主动方式,后期通过重建的方式
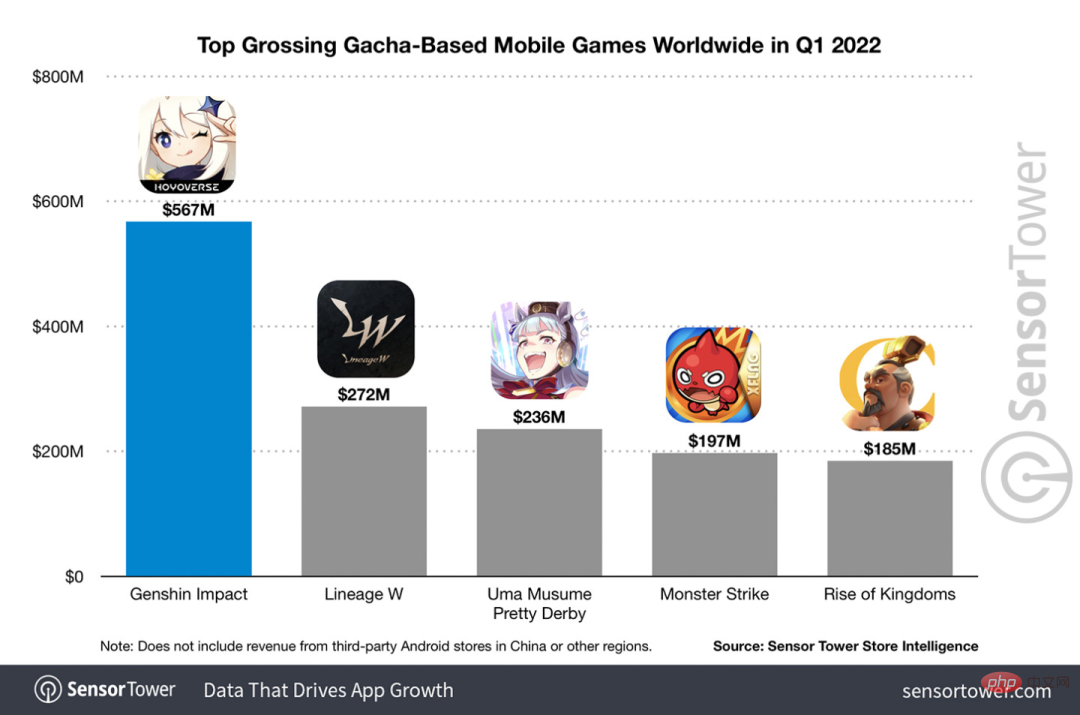
说到这两年风靡全球的国产游戏,原神肯定是当仁不让。根据5月公布的本年度Q1季度手游收入调查报告,在抽卡手游里《原神》以5.67亿美金的绝对优势稳稳拿下第一,这也宣告《原神》在上线短短18个月之后单在手机平台总收入就突破30亿美金(大约RM130亿)。如今,开放须弥前最后的2.8海岛版本姗姗来迟,在漫长的长草期后终于又有新的剧情和区域可以肝了。不过不知道有多少“肝帝”,现在海岛已经满探索,又开始长草了。宝箱总共182个+1个摩拉箱(不计入)长草期根本没在怕的,原神区从来不缺整活儿。这不,在长草期间

最近在Arxiv上阅读到一篇关于纯视觉环视感知的最新研究,该研究基于PETR系列方法,并专注于解决远距离目标检测的纯视觉感知问题,将感知范围扩大到150米。这篇论文的方法和结果对我们来说有很大的参考价值,所以我尝试着对其进行解读原标题:Far3D:ExpandingtheHorizonforSurround-view3DObjectDetection论文链接:https://arxiv.org/abs/2308.09616作者单位:北京理工大学&旷视科技任务背景三维物体检测在理解自动驾驶


Hot AI Tools

Undresser.AI Undress
AI-powered app for creating realistic nude photos

AI Clothes Remover
Online AI tool for removing clothes from photos.

Undress AI Tool
Undress images for free

Clothoff.io
AI clothes remover

AI Hentai Generator
Generate AI Hentai for free.

Hot Article

Hot Tools
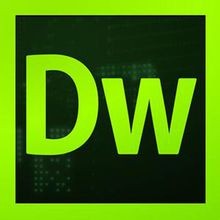
Dreamweaver CS6
Visual web development tools
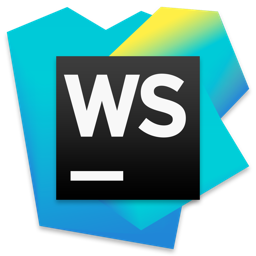
WebStorm Mac version
Useful JavaScript development tools

Zend Studio 13.0.1
Powerful PHP integrated development environment

SAP NetWeaver Server Adapter for Eclipse
Integrate Eclipse with SAP NetWeaver application server.

Safe Exam Browser
Safe Exam Browser is a secure browser environment for taking online exams securely. This software turns any computer into a secure workstation. It controls access to any utility and prevents students from using unauthorized resources.