As enterprises try to implement GenAI into their business, they face a lot of resistance and surprising change management issues. While senior leaders can support the move, middle managers and industry figures are resistant. Some may resist because they fear losing their jobs or need to rethink how to do their jobs better. In addition, enterprises also face the problem between random answers and deterministic answers when using AI tools, which further increases the existence of resistance
AI’s answers are probabilistic
GenAI provides gives a probabilistic answer, that is, it provides the most likely answer or next step, so when it comes to composing a letter or written work, it provides the most likely next word, phrase, or idea, however, the most likely The answer is different from the correct answer. Granted, this is usually a correct answer, and - given the vast amounts of training data available - it's very likely to be a good answer, but it's very different from the correct answer.
Further complicating this issue is the challenge of an audit trail of how the answer was arrived at. Many of the challenges faced in GenAI implementations arise from applications that require deterministic answers and the need to fully explain how the answer was derived. Where certainty in answers is required or perceived to be required, businesses and the people within them resist these applications because they create doubt about the usefulness or reliability of the results.
As it turns out, this is the case even when AI is offered as an assistant and humans are asked to be the final arbiter of answers, with a lack of explanation and uncertainty surrounding assistance creating distrust and resistance.
Machine intelligence provides probabilistic answers does not mean that it has no value, many examples show that it is valuable, however, if a company applies this answer to a question that requires a deterministic answer, then it Seems like a lie
Enterprises will encounter frustration when looking to apply GenAI to their business because when they need deterministic answers, they end up using probabilistic answers, so they need to start with probabilities Do machine learning (e.g. it is a tree because it has green leaves) and then need to test to see if there are issues that affect the validity of the answer (e.g. autumn).
Which areas are suitable for probabilistic models of AI?
How should enterprises introduce GenAI into programming? The technology industry has made some bold predictions that GenAI or AI can learn to program and significantly improve Programmer proficiency or efficiency productivity, that's interesting.
In the field of coding this is immediately effective, for example, the development of test scripts can itself be handled through probabilistic models, what is important here is that a business can test against many conditions and as broadly and as Generate scripts in depth. Creating effective tests for code and security vulnerabilities is an inherently probabilistic exercise, so GenAI shines and can be easily adopted by practitioners.
On the other hand, it is not conducive to the actual development of code. An enterprise wants the code to be 100% correct, which requires a more deterministic answer. However, it performs well in supporting knowledge management of code development. Highlights also allow you to create great starter sets, significantly increasing productivity.
Another advantage of GenAI is its ability to synthesize and summarize knowledge, so in the field of knowledge management, GenAI has made great achievements
In terms of customer relationship management functions, Salesforce has achieved great results through its Einstein product and other products, have made great progress successfully. These products are designed to synthesize or aggregate information from customer needs information to enable salespeople to conduct knowledge management more effectively. This is a very effective application scenario for GenAI
Marketing is a great field, for example: What might be the best next step to take? GenAI can have a huge immediate impact when it comes to sorting through customer data to determine the best solution, so it could become a powerful tool in processes such as claims processing where conclusive answers are needed. For example, for medical claims, we need to determine how to obtain the appropriate amount of compensation. In most cases, just getting it right is not enough, we need to be right all the time
The following questions can be helpful as an effective starting point for using GenAI to reduce frustration and resistance:
Where can we use it effectively right away?
- Where does it need to be combined with other technologies?
- Where should we not use it?
- What about the human factor?
Although probabilistic answers are a useful tool to solve problems, they generally do not exclude humans. Instead, it gives humans more sophisticated tools, especially when a business needs deterministic answers
If a business uses AI tools to help with business data and aggregate data, it can reduce friction, which is great Helps, but when using it to make decisions, people may feel uncomfortable with the decisions because they are not always correct, and since they are not sure how the AI tool got the answer, they don't know how to check it.
What is the way out of these dilemmas?
The solution focuses on fully understanding how automation works and its impact on other human tasks, and providing guidance and assistance for downstream work or other Possible Unintended Consequences
The application of this tool needs to be more mature, and users must be thoughtful when making decisions and fully consider its impact. When assessing a product's maturity, you need to consider individuals and businesses as well as the unintended consequences that may arise, lest they resist and stifle the tool's growth
Some questions that require this thought process include:
- Consider not only the tasks being automated, but also the impact of the human role being automated.
- Carefully consider the reality that any needed productivity increase will result in fewer people working on the task. How could you even take this into account?
- Think about how you can test this tool so people can trust it. The greater the uncertainty created by a disruptive new technology, the less willing people will be to trust it.
- Before moving to sweeping changes, think about how you can pilot AI tools to demonstrate their benefits.
Some Final Thoughts
After a year of frantic experimentation with GenAI, the industry successfully conducted thousands of pilots, yet the majority of these pilots All failed to reach production because they were hampered by myriad challenges in funding, change management, and adaptation.
However, GenAI is rapidly entering production and achieving impressive returns. The success of these production use cases seems to be more due to the use case being a perfect fit with GenAI's features rather than tool selection or other factors. Additionally, it seems that for most business functions, GenAI has a rich Effective roles are almost always focused on the correct sub-function. Given the huge investment in experimentation, perhaps the most effective way for most enterprises to discover where GenAI can work for them is to look at use cases where GenAI has successfully transitioned from pilot to production. Where this progress is already happening in large numbers, businesses can be confident that there is a good fit and significantly reduce the risk of wasted effort and money.
The above is the detailed content of Key issues affecting the effectiveness of GenAI. For more information, please follow other related articles on the PHP Chinese website!
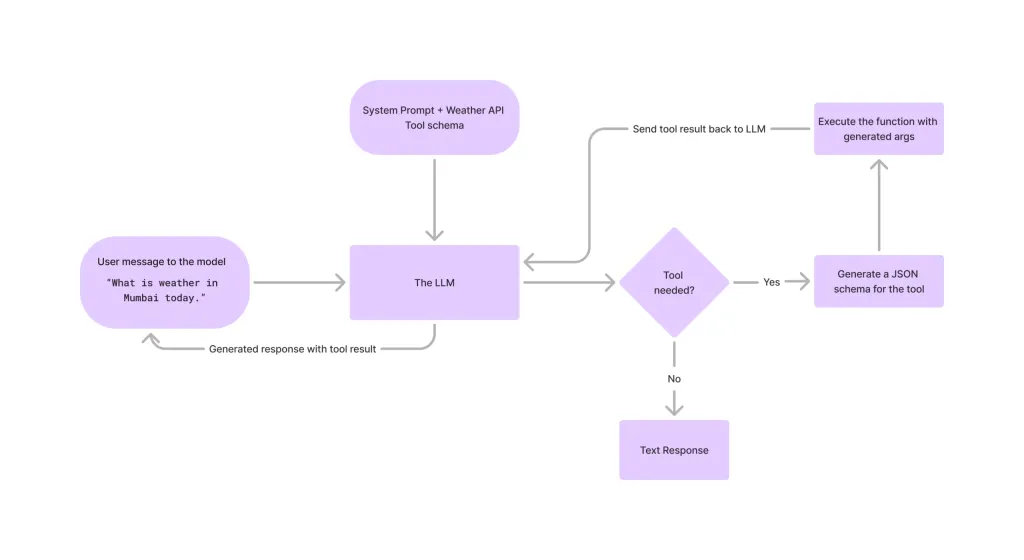
Large language models (LLMs) have surged in popularity, with the tool-calling feature dramatically expanding their capabilities beyond simple text generation. Now, LLMs can handle complex automation tasks such as dynamic UI creation and autonomous a
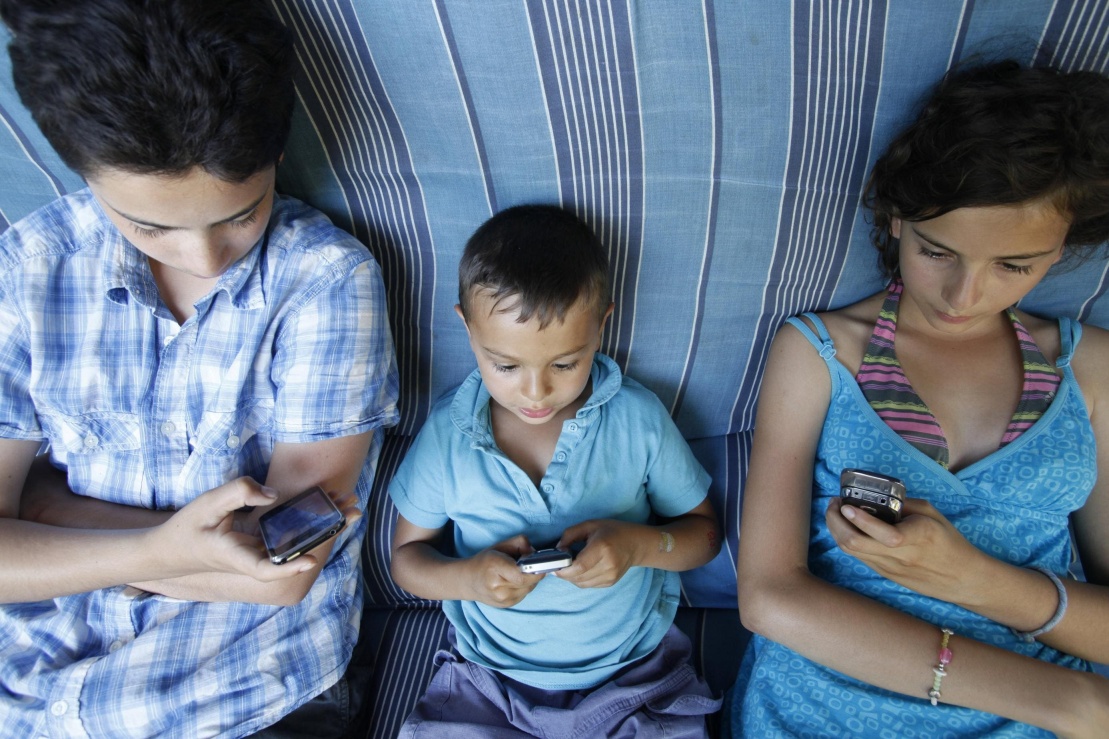
Can a video game ease anxiety, build focus, or support a child with ADHD? As healthcare challenges surge globally — especially among youth — innovators are turning to an unlikely tool: video games. Now one of the world’s largest entertainment indus
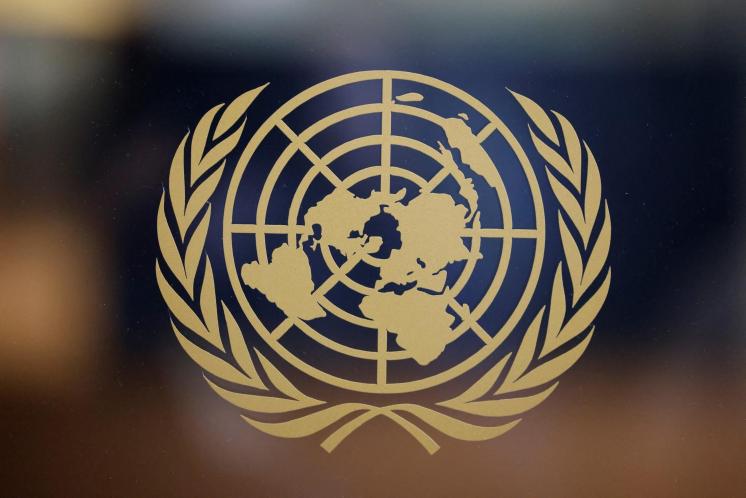
“History has shown that while technological progress drives economic growth, it does not on its own ensure equitable income distribution or promote inclusive human development,” writes Rebeca Grynspan, Secretary-General of UNCTAD, in the preamble.
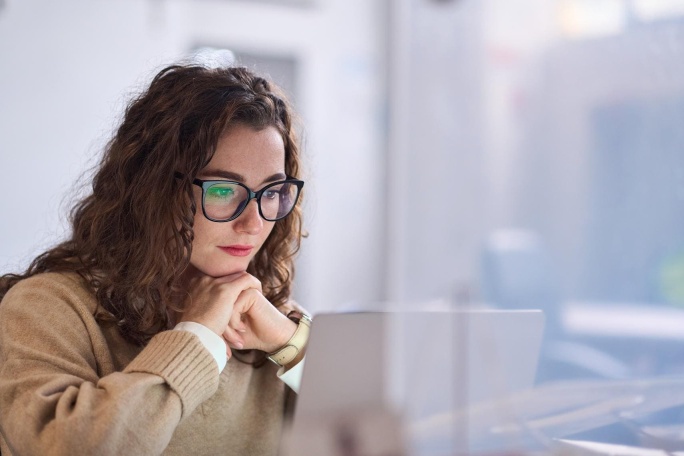
Easy-peasy, use generative AI as your negotiation tutor and sparring partner. Let’s talk about it. This analysis of an innovative AI breakthrough is part of my ongoing Forbes column coverage on the latest in AI, including identifying and explaining
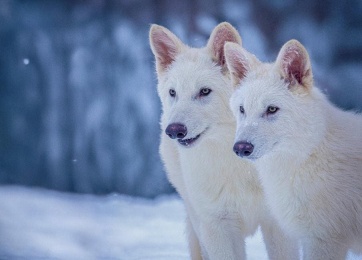
The TED2025 Conference, held in Vancouver, wrapped its 36th edition yesterday, April 11. It featured 80 speakers from more than 60 countries, including Sam Altman, Eric Schmidt, and Palmer Luckey. TED’s theme, “humanity reimagined,” was tailor made
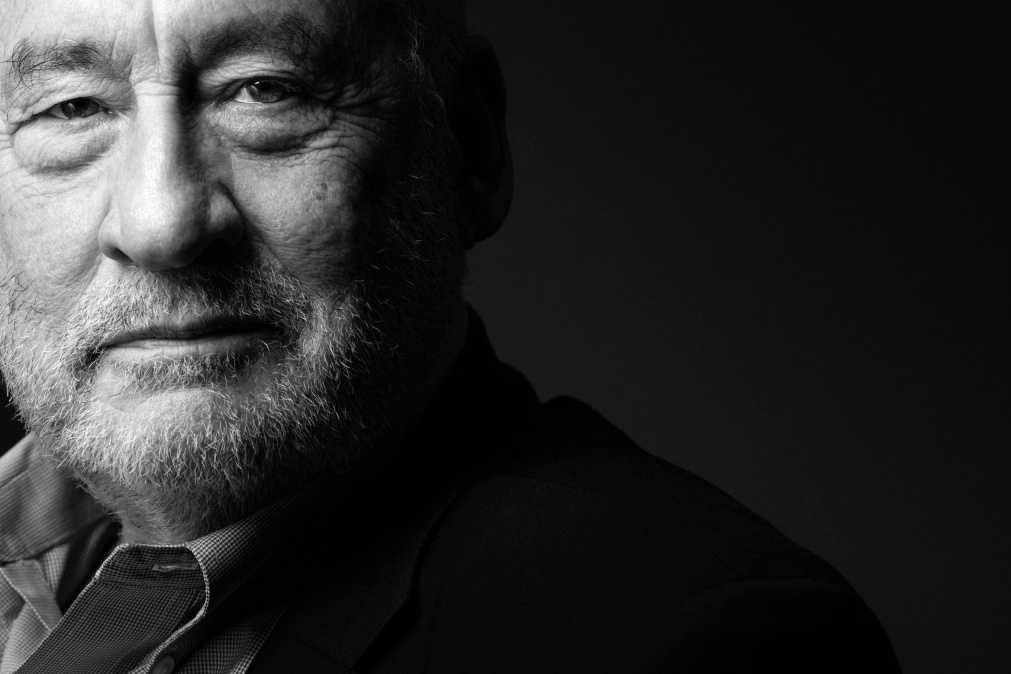
Joseph Stiglitz is renowned economist and recipient of the Nobel Prize in Economics in 2001. Stiglitz posits that AI can worsen existing inequalities and consolidated power in the hands of a few dominant corporations, ultimately undermining economic
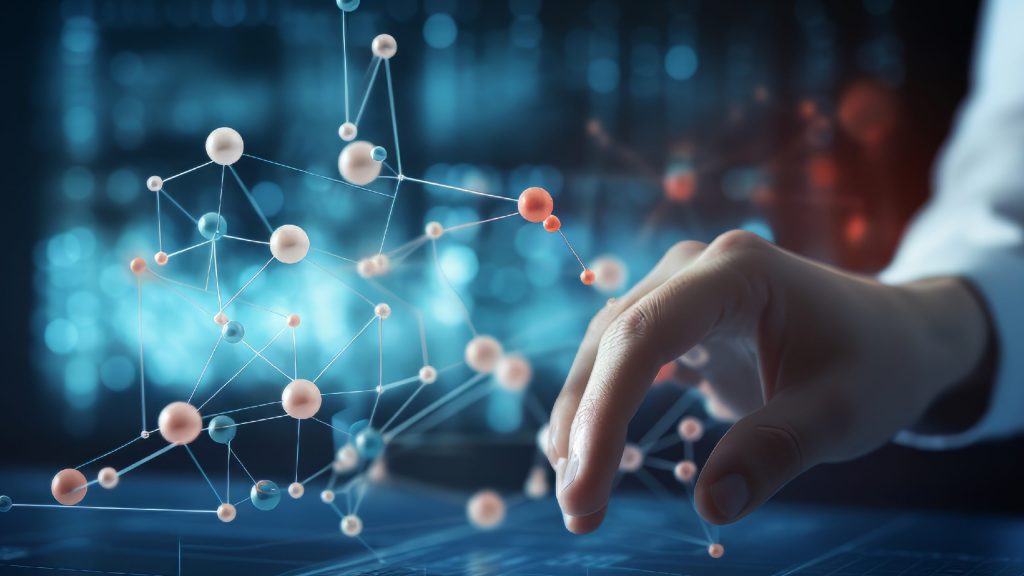
Graph Databases: Revolutionizing Data Management Through Relationships As data expands and its characteristics evolve across various fields, graph databases are emerging as transformative solutions for managing interconnected data. Unlike traditional
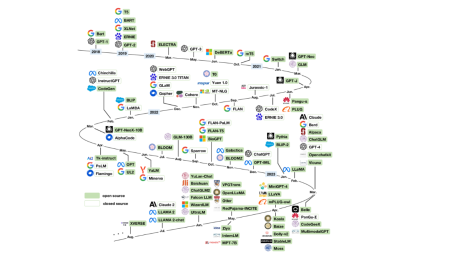
Large Language Model (LLM) Routing: Optimizing Performance Through Intelligent Task Distribution The rapidly evolving landscape of LLMs presents a diverse range of models, each with unique strengths and weaknesses. Some excel at creative content gen


Hot AI Tools

Undresser.AI Undress
AI-powered app for creating realistic nude photos

AI Clothes Remover
Online AI tool for removing clothes from photos.

Undress AI Tool
Undress images for free

Clothoff.io
AI clothes remover

AI Hentai Generator
Generate AI Hentai for free.

Hot Article

Hot Tools

SublimeText3 Linux new version
SublimeText3 Linux latest version

SAP NetWeaver Server Adapter for Eclipse
Integrate Eclipse with SAP NetWeaver application server.

SublimeText3 Chinese version
Chinese version, very easy to use
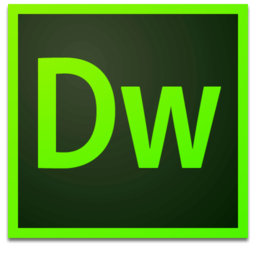
Dreamweaver Mac version
Visual web development tools

DVWA
Damn Vulnerable Web App (DVWA) is a PHP/MySQL web application that is very vulnerable. Its main goals are to be an aid for security professionals to test their skills and tools in a legal environment, to help web developers better understand the process of securing web applications, and to help teachers/students teach/learn in a classroom environment Web application security. The goal of DVWA is to practice some of the most common web vulnerabilities through a simple and straightforward interface, with varying degrees of difficulty. Please note that this software