


If they disagree, they will score points. Why are big domestic AI models addicted to 'swiping the rankings'?
I believe that friends who follow the mobile phone circle will not be unfamiliar with the phrase "get a score if you don't accept it". For example, theoretical performance testing software such as AnTuTu and GeekBench have attracted much attention from players because they can reflect the performance of mobile phones to a certain extent. Similarly, there are corresponding benchmarking software for PC processors and graphics cards to measure their performance
Since "everything can run", the most popular large AI models have also begun to participate in the running score competition. Especially after the "Hundred Model War" began, breakthroughs were made almost every day, and each company called itself "running" Score first"
Domestic AI large models have almost never lagged behind in terms of performance scores, but they have never been able to surpass GPT-4 in terms of user experience. This raises a question, that is, at major sales points, each mobile phone manufacturer can always claim that its products are "number one in sales". By constantly adding attributive terms, the market is subdivided and subdivided, so that everyone has the opportunity to become the number one. , but in the field of AI large models, the situation is different. After all, their evaluation criteria are basically unified, including MMLU (used to measure multi-task language understanding ability), Big-Bench (used to quantify and extrapolate the ability of LLMs), and AGIEval (used to evaluate the ability to deal with human-level problems). Task ability)
Currently, the large-scale model evaluation lists that are often cited in China include SuperCLUE, CMMLU and C-Eval. Among them, CMMLU and C-Eval are comprehensive examination evaluation sets jointly constructed by Tsinghua University, Shanghai Jiao Tong University and University of Edinburgh. CMMLU is jointly launched by MBZUAI, Shanghai Jiao Tong University and Microsoft Research Asia. As for SuperCLUE, it is co-written by artificial intelligence professionals from major universities
Take C-Eval as an example. On the list in early September, Yuntian Lifei's large model "Yuntian Shu" ranked first, 360 ranked eighth, but GPT-4 could only rank tenth. Since the standard is quantifiable, why are there counter-intuitive results? The reason why the large model running score list shows a scene of "devils dancing around" is actually because the current methods of evaluating the performance of large AI models have limitations. They use a "question solving" method to measure the ability of large models.
As we all know, in order to protect their lifespan, smartphone SoCs, computer CPUs and graphics cards will automatically reduce frequency under high temperatures, while low temperatures can improve chip performance. Therefore, some people will put their mobile phones in the refrigerator or equip their computers with more powerful cooling systems for performance testing, and can usually get higher scores than normal. In addition, major mobile phone manufacturers will also carry out "exclusive optimization" for various benchmarking software, which has become their standard operation
In the same way, the scoring of large artificial intelligence models is centered around question-taking, so there will naturally be a question bank. Yes, this is the reason why some large domestic models continue to be on the list. Due to various reasons, the question banks of major model lists are currently almost one-way transparent to manufacturers, which is what is called "benchmark leakage". For example, the C-Eval list had 13,948 questions when it was first launched, and due to the limited question bank, some unknown large models were allowed to "pass" by completing questions
You can imagine that before the exam, if you accidentally see the test paper and standard answers, and then memorize the questions suddenly, the exam scores will be greatly improved. Therefore, the question bank preset by the large model list is added to the training set, so that the large model becomes a model that fits the benchmark data. Moreover, the current LLM itself is known for its excellent memory, and reciting standard answers is simply a piece of cake
Through this method, small-size models can also have better results than large-size models in running scores. Some of the high scores achieved by large models are achieved through such "fine-tuning". In the paper "Don't Make Your LLM an Evaluation Benchmark Cheater", the Renmin University Hillhouse team bluntly pointed out this phenomenon, and this opportunistic approach is harmful to the performance of large models.
Researchers from the Hillhouse team found that benchmark leakage will cause large models to run exaggerated results. For example, a 1.3B model can surpass a model 10 times the size in some tasks, but the side effect is that these are specially designed for "test-taking" "The performance of large models designed on other normal testing tasks will be adversely affected. After all, if you think about it, you will know that the large AI model was originally supposed to be a "question maker", but it has become a "question memorizer". In order to get high scores on a certain list, it uses the specific knowledge and output style of the list. It will definitely mislead the large model.
The non-intersection of the training set, verification set, and test set is obviously only an ideal state. After all, the reality is very skinny, and the problem of data leakage is almost inevitable from the root. With the continuous advancement of related technologies, the memory and reception capabilities of the Transformer structure, which is the cornerstone of current large models, are constantly improving. This summer, Microsoft Research's General AI strategy has enabled the model to receive 100 million Tokens without causing unacceptable of forgetfulness. In other words, in the future, large AI models are likely to have the ability to read the entire Internet.
Even if technological progress is put aside, data pollution is actually difficult to avoid based on the current technical level, because high-quality data is always scarce and production capacity is limited. A paper published by the AI research team Epoch at the beginning of this year showed that AI will use up all high-quality human language data in less than 5 years, and this result is that it will increase the growth rate of human language data, that is, all human beings will publish in the next 5 years. Books written, papers written, and code written are all taken into account to predict the results.
If a data set is suitable for evaluation, then it will definitely play a better role in pre-training. For example, OpenAI's GPT-4 uses the authoritative inference evaluation data set GSM8K. Therefore, there is currently an embarrassing problem in the field of large-scale model evaluation. The demand for data from large-scale models seems to be endless, which leads to the evaluation agencies having to move faster and further than the manufacturers of artificial intelligence large-scale models. However, today’s assessment agencies seem to be simply incapable of doing this
As for why some manufacturers pay special attention to the running scores of large models and try to improve the rankings one after another? In fact, the logic behind this behavior is exactly the same as App developers injecting water into the number of users of their own Apps. After all, the user scale of an App is a key factor in measuring its value, and in the initial stage of the current large-scale AI model, the results on the evaluation list are almost the only relatively objective criterion. After all, in public perception, high scores mean It equals strong performance.
When brushing the rankings may bring a strong publicity effect and may even lay the foundation for financing, the addition of commercial interests will inevitably drive large AI model manufacturers to rush to brush the rankings.
The above is the detailed content of If they disagree, they will score points. Why are big domestic AI models addicted to 'swiping the rankings'?. For more information, please follow other related articles on the PHP Chinese website!
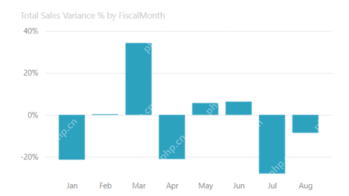
Harnessing the Power of Data Visualization with Microsoft Power BI Charts In today's data-driven world, effectively communicating complex information to non-technical audiences is crucial. Data visualization bridges this gap, transforming raw data i
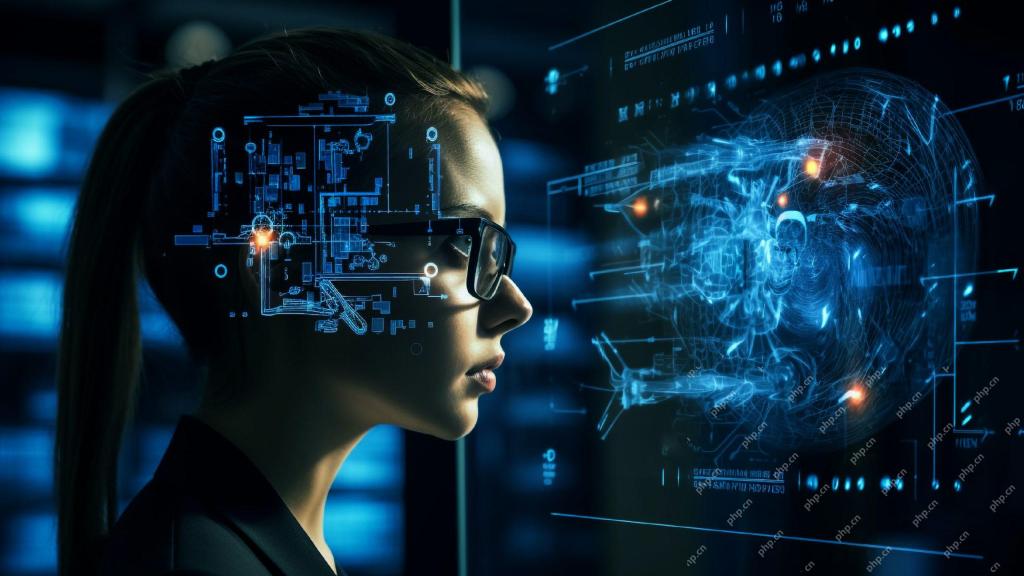
Expert Systems: A Deep Dive into AI's Decision-Making Power Imagine having access to expert advice on anything, from medical diagnoses to financial planning. That's the power of expert systems in artificial intelligence. These systems mimic the pro
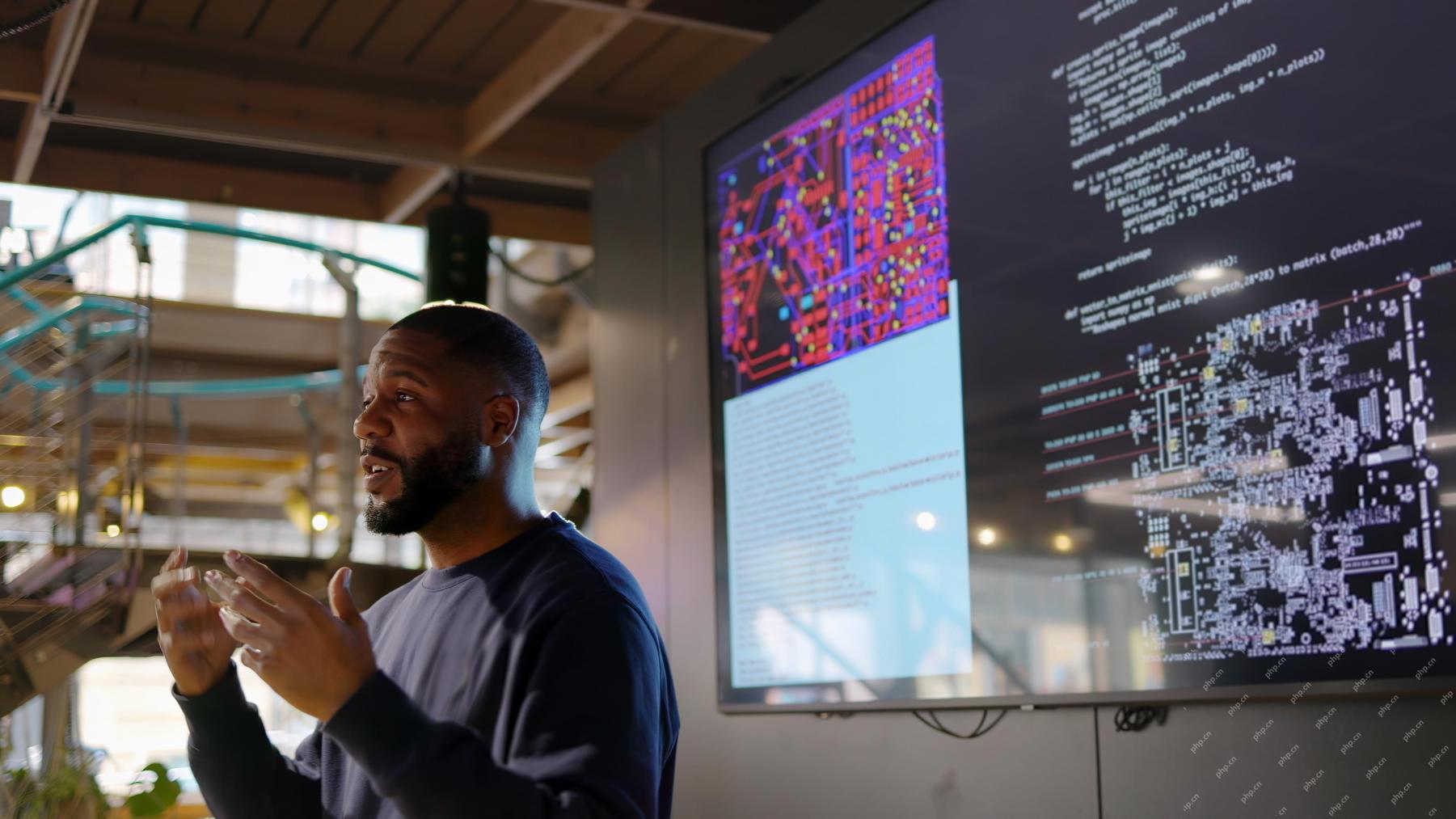
First of all, it’s apparent that this is happening quickly. Various companies are talking about the proportions of their code that are currently written by AI, and these are increasing at a rapid clip. There’s a lot of job displacement already around
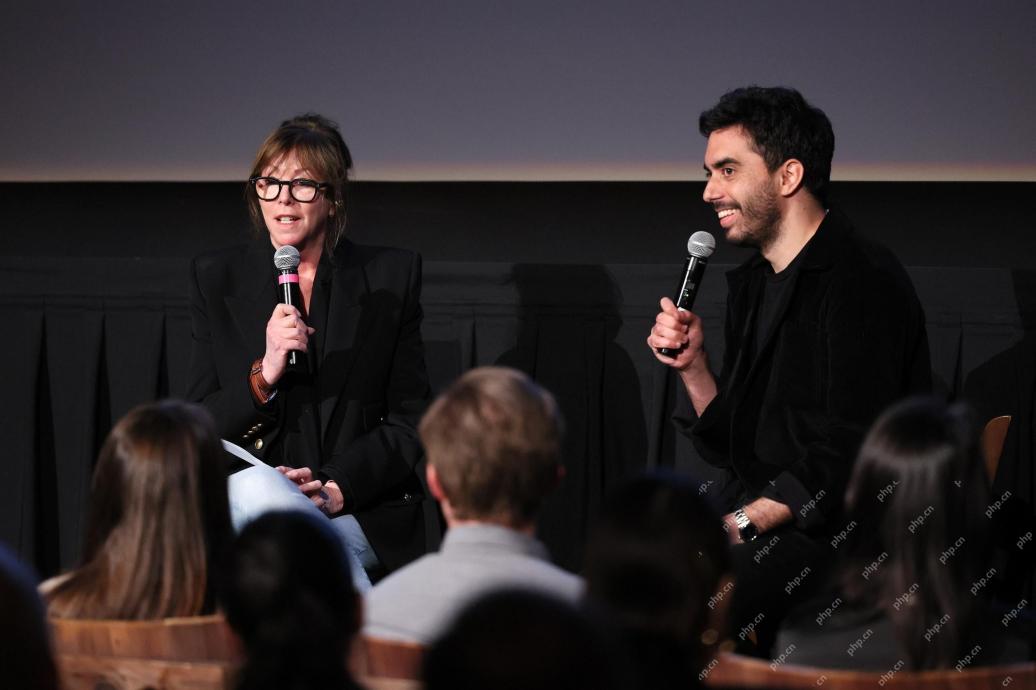
The film industry, alongside all creative sectors, from digital marketing to social media, stands at a technological crossroad. As artificial intelligence begins to reshape every aspect of visual storytelling and change the landscape of entertainment
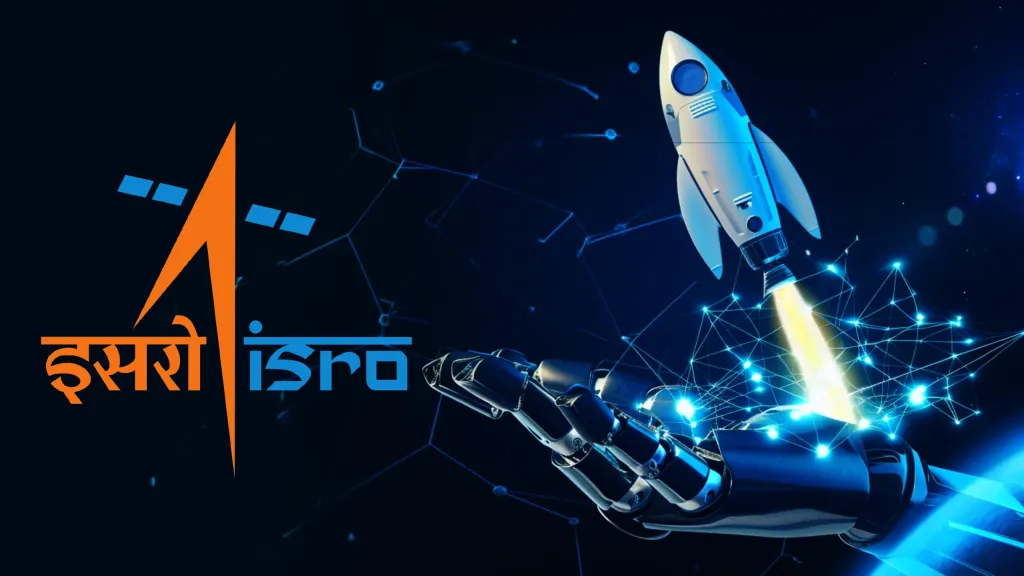
ISRO's Free AI/ML Online Course: A Gateway to Geospatial Technology Innovation The Indian Space Research Organisation (ISRO), through its Indian Institute of Remote Sensing (IIRS), is offering a fantastic opportunity for students and professionals to
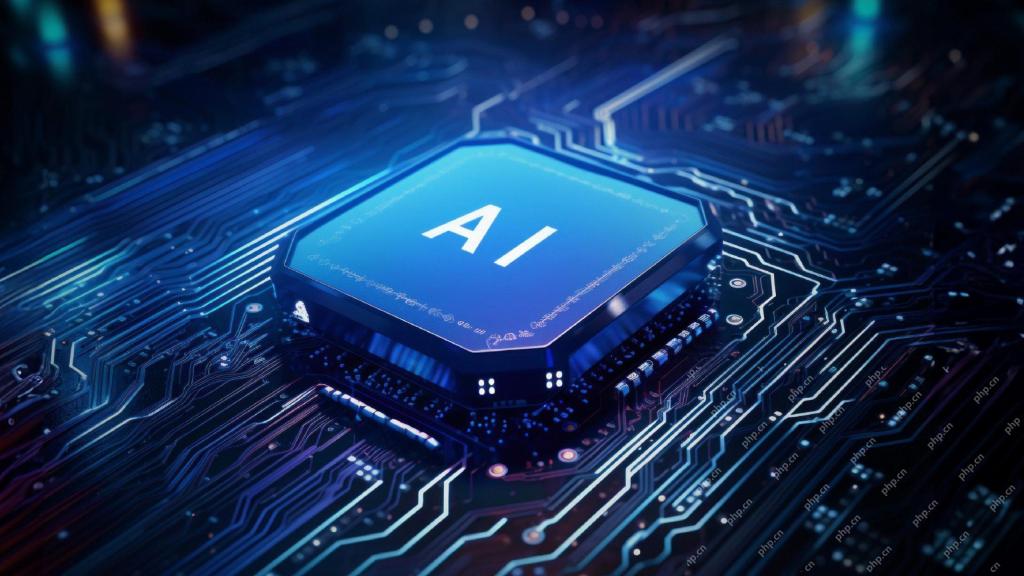
Local Search Algorithms: A Comprehensive Guide Planning a large-scale event requires efficient workload distribution. When traditional approaches fail, local search algorithms offer a powerful solution. This article explores hill climbing and simul
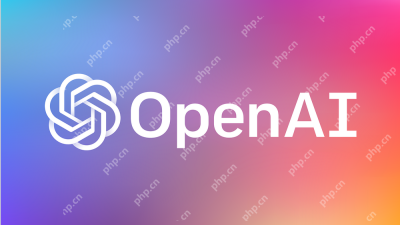
The release includes three distinct models, GPT-4.1, GPT-4.1 mini and GPT-4.1 nano, signaling a move toward task-specific optimizations within the large language model landscape. These models are not immediately replacing user-facing interfaces like
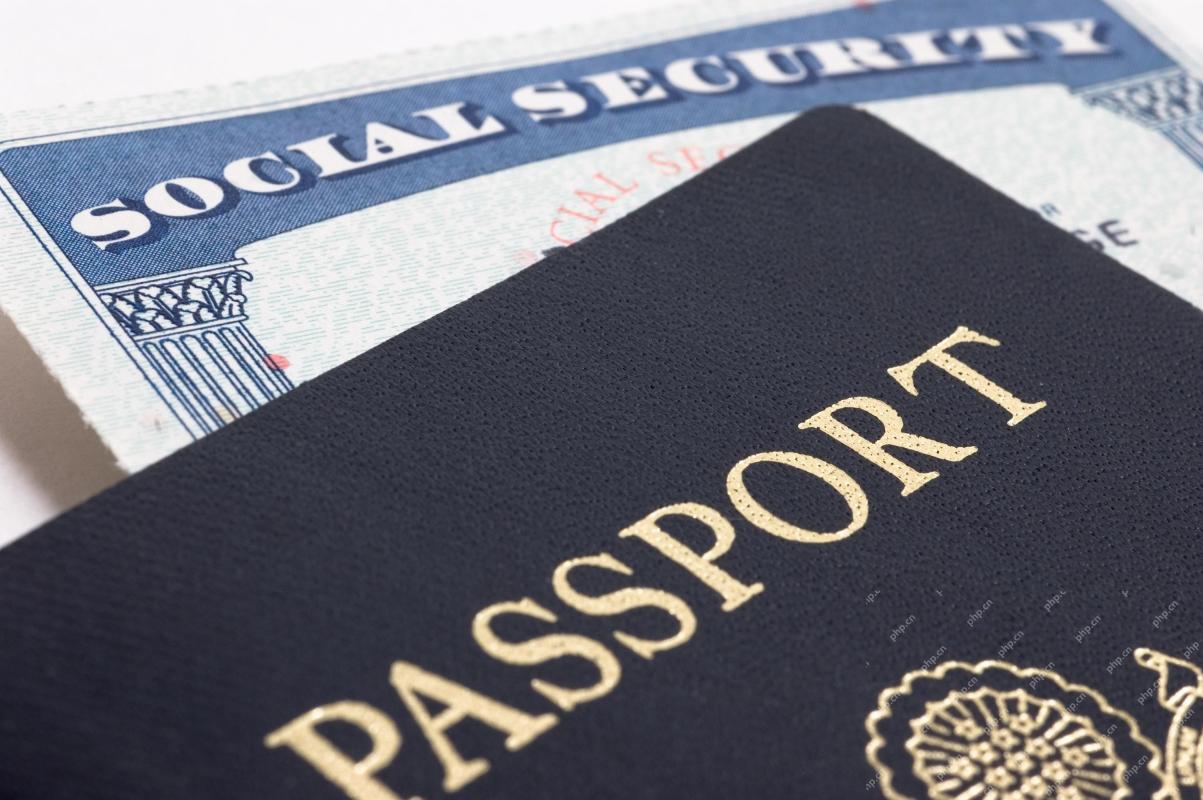
Chip giant Nvidia said on Monday it will start manufacturing AI supercomputers— machines that can process copious amounts of data and run complex algorithms— entirely within the U.S. for the first time. The announcement comes after President Trump si


Hot AI Tools

Undresser.AI Undress
AI-powered app for creating realistic nude photos

AI Clothes Remover
Online AI tool for removing clothes from photos.

Undress AI Tool
Undress images for free

Clothoff.io
AI clothes remover

AI Hentai Generator
Generate AI Hentai for free.

Hot Article

Hot Tools
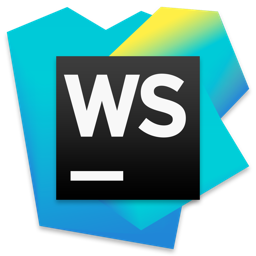
WebStorm Mac version
Useful JavaScript development tools
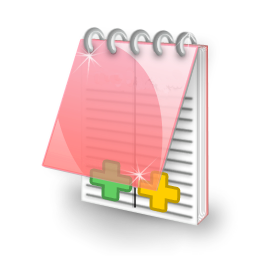
EditPlus Chinese cracked version
Small size, syntax highlighting, does not support code prompt function
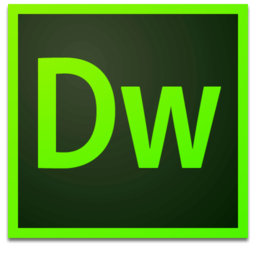
Dreamweaver Mac version
Visual web development tools

Zend Studio 13.0.1
Powerful PHP integrated development environment

SAP NetWeaver Server Adapter for Eclipse
Integrate Eclipse with SAP NetWeaver application server.