


Notes on Python development: Avoid common database operation problems
Introduction:
In Python development, database operations are very common tasks. However, due to developers' carelessness or lack of experience in database operations, a series of problems may occur, such as data inconsistency, performance degradation, security issues, etc. This article will introduce some common database operation problems and provide corresponding solutions to help developers avoid these problems.
1. The database connection problem is not handled correctly
When performing database operations, it is very important to handle the database connection correctly. Common problems include forgetting to close connections, connection leaks, connection pool full, etc. These issues can lead to performance degradation, wasted resources, or even system crashes.
Solution:
-
Use context to manage connections: Use the with statement to ensure that the connection is automatically closed when leaving the scope, as follows:
with connection.cursor() as cursor: # 执行数据库操作 pass
- Use connection pool: The connection pool can effectively manage connection resources and avoid the problems of connection leakage and full connection pool. It is recommended to use the connection pool function in open source libraries such as
DBUtils
,SQLAlchemy
,pymysql
, etc.
2. Forget to add transaction processing
When multiple database operations are involved, it is often necessary to maintain data consistency. If transactions are not used, data inconsistencies may arise, such as the inability to roll back when certain operations fail.
Solution:
-
Use transaction processing: For database operations that require consistency, transactions should be used. In Python, transaction processing can be implemented in the following ways:
with connection.cursor() as cursor: try: connection.begin() # 开启事务 # 执行数据库操作 connection.commit() # 提交事务 except: connection.rollback() # 回滚事务
- Add exception handling: When an exception is caught, the transaction should be rolled back in time to ensure data consistency.
3. Failure to parameterize SQL statements
When splicing SQL statements, if the user input parameters are not processed correctly, it may lead to SQL injection attacks, allowing malicious users to Perform illegal database operations, causing data leakage or damage.
Solution:
-
Use parameterized query: use parameter binding to pass the data entered by the user as a parameter into the database operation, instead of splicing it directly into in the SQL statement. For example:
sql = "SELECT * FROM users WHERE username = %s AND password = %s" cursor.execute(sql, (username, password))
- Input validation: Verify and filter user input to ensure that the entered data meets the requirements. Use Python's built-in regular expressions, string processing functions, etc. for security checks.
4. Failure to implement appropriate indexes
The index is a data structure provided in the database to speed up data retrieval. If indexes are not designed and used correctly, it may lead to inefficient queries or even full table scans.
Solution:
- Index design: When designing the database, set appropriate indexes reasonably according to the access mode and query requirements of the data. At the same time, the database should be optimized regularly, such as deleting useless indexes.
- Query optimization: When performing complex queries, analyze the execution plan to determine whether the query uses a suitable index. If not, you can consider optimizing the query.
5. Large batch operations are not processed in batches
When a large amount of data needs to be operated, such as inserting, updating, deleting, etc., one-time processing may cause memory overflow or performance decline.
Solution:
- Batch processing: Split large batch operations into multiple batch operations to reduce the amount of data in each operation and reduce memory pressure. Batch processing can be achieved by adding a
LIMIT
clause or using a cursor. - Batch submission: For insertion operations, data can be submitted to the database in batches instead of single insertion to reduce network communication overhead.
Summary:
In Python development, correctly handling database operations is a very important part. This article introduces some common database operation problems and provides corresponding solutions to help developers avoid these problems. By following these considerations, you can improve the performance, security, and maintainability of database operations, thereby better completing Python development tasks.
The above is the detailed content of Python Development Notes: Avoid Common Database Operation Problems. For more information, please follow other related articles on the PHP Chinese website!
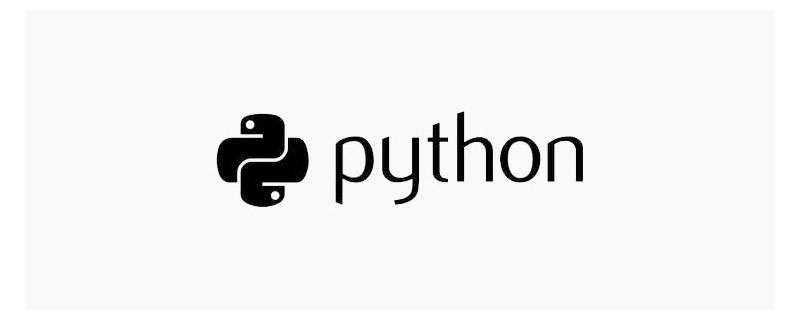
本篇文章给大家带来了关于Python的相关知识,其中主要介绍了关于Seaborn的相关问题,包括了数据可视化处理的散点图、折线图、条形图等等内容,下面一起来看一下,希望对大家有帮助。
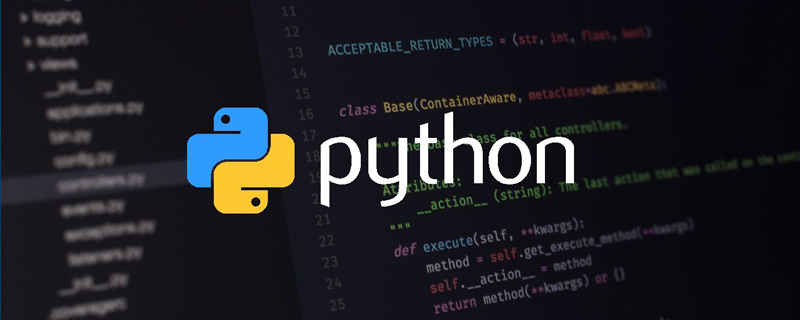
本篇文章给大家带来了关于Python的相关知识,其中主要介绍了关于进程池与进程锁的相关问题,包括进程池的创建模块,进程池函数等等内容,下面一起来看一下,希望对大家有帮助。
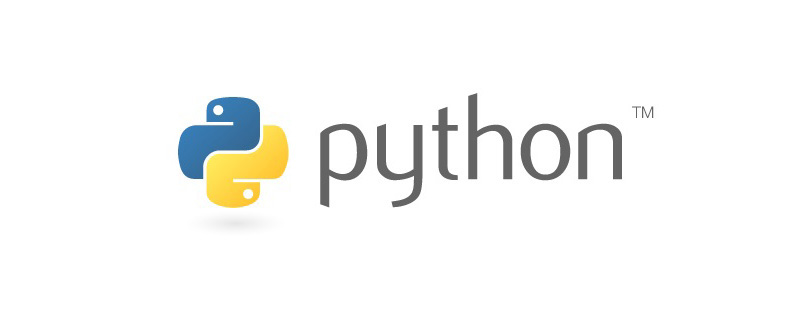
本篇文章给大家带来了关于Python的相关知识,其中主要介绍了关于简历筛选的相关问题,包括了定义 ReadDoc 类用以读取 word 文件以及定义 search_word 函数用以筛选的相关内容,下面一起来看一下,希望对大家有帮助。
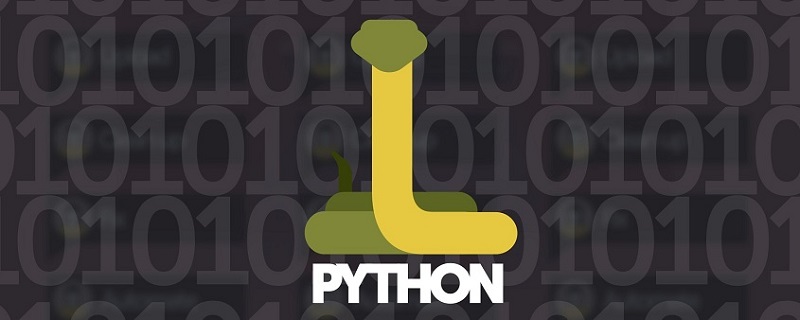
本篇文章给大家带来了关于Python的相关知识,其中主要介绍了关于数据类型之字符串、数字的相关问题,下面一起来看一下,希望对大家有帮助。
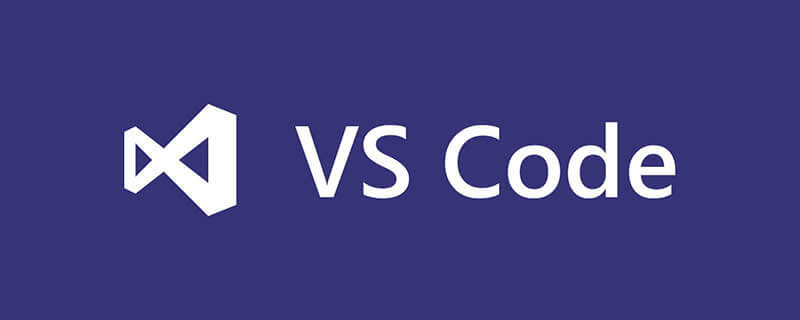
VS Code的确是一款非常热门、有强大用户基础的一款开发工具。本文给大家介绍一下10款高效、好用的插件,能够让原本单薄的VS Code如虎添翼,开发效率顿时提升到一个新的阶段。
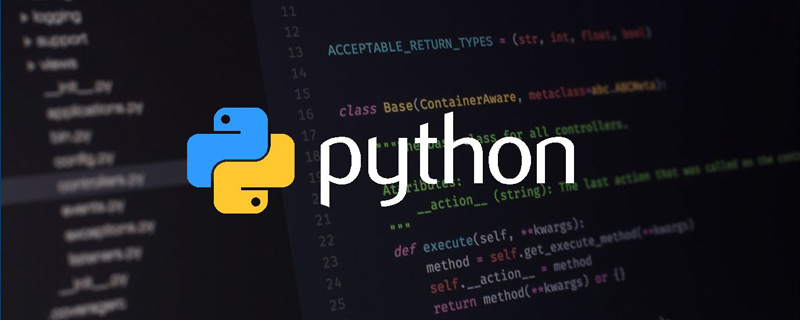
本篇文章给大家带来了关于Python的相关知识,其中主要介绍了关于numpy模块的相关问题,Numpy是Numerical Python extensions的缩写,字面意思是Python数值计算扩展,下面一起来看一下,希望对大家有帮助。
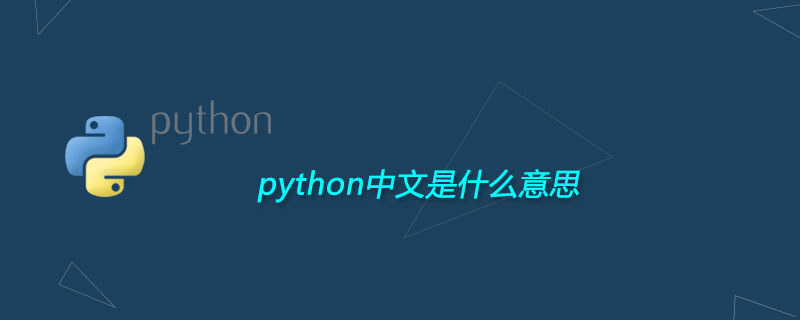
pythn的中文意思是巨蟒、蟒蛇。1989年圣诞节期间,Guido van Rossum在家闲的没事干,为了跟朋友庆祝圣诞节,决定发明一种全新的脚本语言。他很喜欢一个肥皂剧叫Monty Python,所以便把这门语言叫做python。


Hot AI Tools

Undresser.AI Undress
AI-powered app for creating realistic nude photos

AI Clothes Remover
Online AI tool for removing clothes from photos.

Undress AI Tool
Undress images for free

Clothoff.io
AI clothes remover

AI Hentai Generator
Generate AI Hentai for free.

Hot Article

Hot Tools
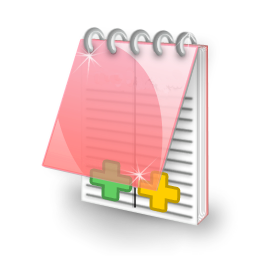
EditPlus Chinese cracked version
Small size, syntax highlighting, does not support code prompt function

SublimeText3 English version
Recommended: Win version, supports code prompts!

MinGW - Minimalist GNU for Windows
This project is in the process of being migrated to osdn.net/projects/mingw, you can continue to follow us there. MinGW: A native Windows port of the GNU Compiler Collection (GCC), freely distributable import libraries and header files for building native Windows applications; includes extensions to the MSVC runtime to support C99 functionality. All MinGW software can run on 64-bit Windows platforms.

SublimeText3 Linux new version
SublimeText3 Linux latest version

SAP NetWeaver Server Adapter for Eclipse
Integrate Eclipse with SAP NetWeaver application server.
