Steps for pandas to read excel files: 1. Make sure the Pandas library has been installed; 2. Import the Pandas library and other libraries that may be needed; 3. Use Pandas's "read_excel()" function to read Excel File; 4. Operate and analyze data, such as viewing the first few rows of data, viewing basic statistics of data, selecting specific columns, filtering, sorting data, grouping and aggregating data, and visualizing data etc.
Operating system for this tutorial: Windows 10 system, Python version 3.11.4, Dell G3 computer.
Pandas is a powerful data processing library that can be used to read, analyze and process various types of data, including Excel files. In this article, I will answer how to read Excel files using Pandas and explain the relevant code.
First, we need to make sure the Pandas library is installed. Pandas can be installed in a Python environment using the following command:
pip install pandas
Next, we need to import the Pandas library and other libraries that may be needed:
import pandas as pd
Now, we can use Pandas’ read_excel() function to read Excel files. The following is a sample code:
df = pd.read_excel('example.xlsx')
In the above code, the read_excel() function accepts one parameter, which is the path to the Excel file. This will return a Pandas DataFrame object named df containing the data from the Excel file.
In addition to the file path, the read_excel() function has other optional parameters, which can be used to specify the specific worksheet to be read, the number of rows to be skipped, the columns to be parsed, etc. For example:
df = pd.read_excel('example.xlsx', sheet_name='Sheet1', skiprows=2, usecols='A:C')
In the above code, the sheet_name parameter specifies the name of the worksheet to be read, the skiprows parameter specifies the number of rows to be skipped, and the usecols parameter specifies the column range to be parsed.
After reading the Excel file, we can use various functions and methods provided by Pandas to operate and analyze the data. Here are some common examples of operations:
View the first few rows of the data:
df.head()
View basic statistics of the data:
df.describe()
Select specific columns:
df['Column1']
Filter:
df[df['Column1'] > 10]
Sort your data:
df.sort_values('Column1', ascending=False)
Group and aggregate data:
df.groupby('Column1').mean()
Visualize data:
df.plot(x='Column1', y='Column2', kind='scatter')
Column1 and Column2 is the column name in the Excel file and can be replaced according to the actual situation.
To summarize, the basic steps for using Pandas to read Excel files include importing the library, using the read_excel() function to read the file, and operating and analyzing the data. Through these operations, we can easily read and process data in Excel files and perform further analysis and visualization.
The above is the detailed content of How to read excel files with pandas. For more information, please follow other related articles on the PHP Chinese website!
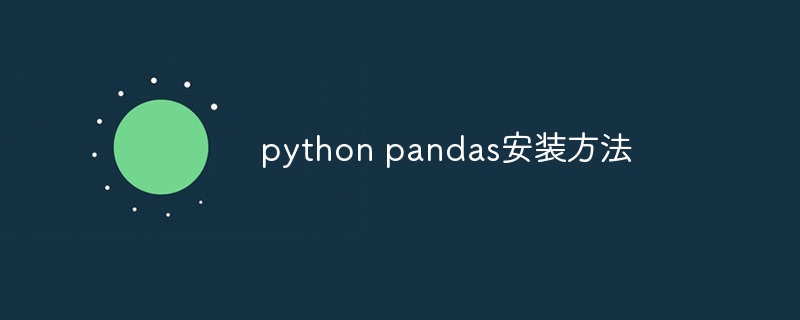
python可以通过使用pip、使用conda、从源代码、使用IDE集成的包管理工具来安装pandas。详细介绍:1、使用pip,在终端或命令提示符中运行pip install pandas命令即可安装pandas;2、使用conda,在终端或命令提示符中运行conda install pandas命令即可安装pandas;3、从源代码安装等等。
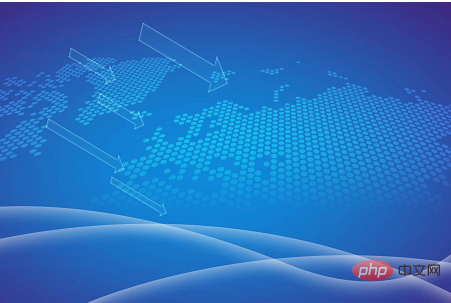
知乎上有个热门提问,日常工作中Python+Pandas是否能代替Excel+VBA?我的建议是,两者是互补关系,不存在谁替代谁。复杂数据分析挖掘用Python+Pandas,日常简单数据处理用Excel+VBA。从数据处理分析能力来看,Python+Pandas肯定是能取代Excel+VBA的,而且要远远比后者强大。但从便利性、传播性、市场认可度来看,Excel+VBA在职场工作上还是无法取代的。因为Excel符合绝大多数人的使用习惯,使用成本更低。就像Photoshop能修出更专业的照片,为
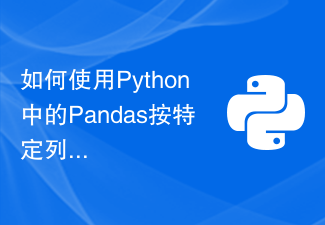
CSV(逗号分隔值)文件广泛用于以简单格式存储和交换数据。在许多数据处理任务中,需要基于特定列合并两个或多个CSV文件。幸运的是,这可以使用Python中的Pandas库轻松实现。在本文中,我们将学习如何使用Python中的Pandas按特定列合并两个CSV文件。什么是Pandas库?Pandas是一个用于Python信息控制和检查的开源库。它提供了用于处理结构化数据(例如表格、时间序列和多维数据)以及高性能数据结构的工具。Pandas广泛应用于金融、数据科学、机器学习和其他需要数据操作的领域。
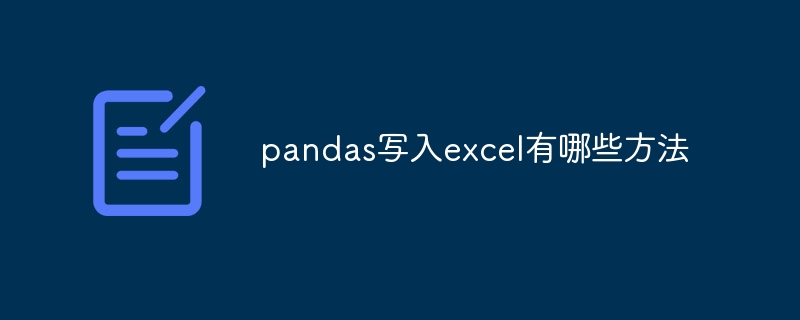
pandas写入excel的方法有:1、安装所需的库;2、读取数据集;3、写入Excel文件;4、指定工作表名称;5、格式化输出;6、自定义样式。Pandas是一个流行的Python数据分析库,提供了许多强大的数据清洗和分析功能,要将Pandas数据写入Excel文件,可以使用Pandas提供的“to_excel()”方法。
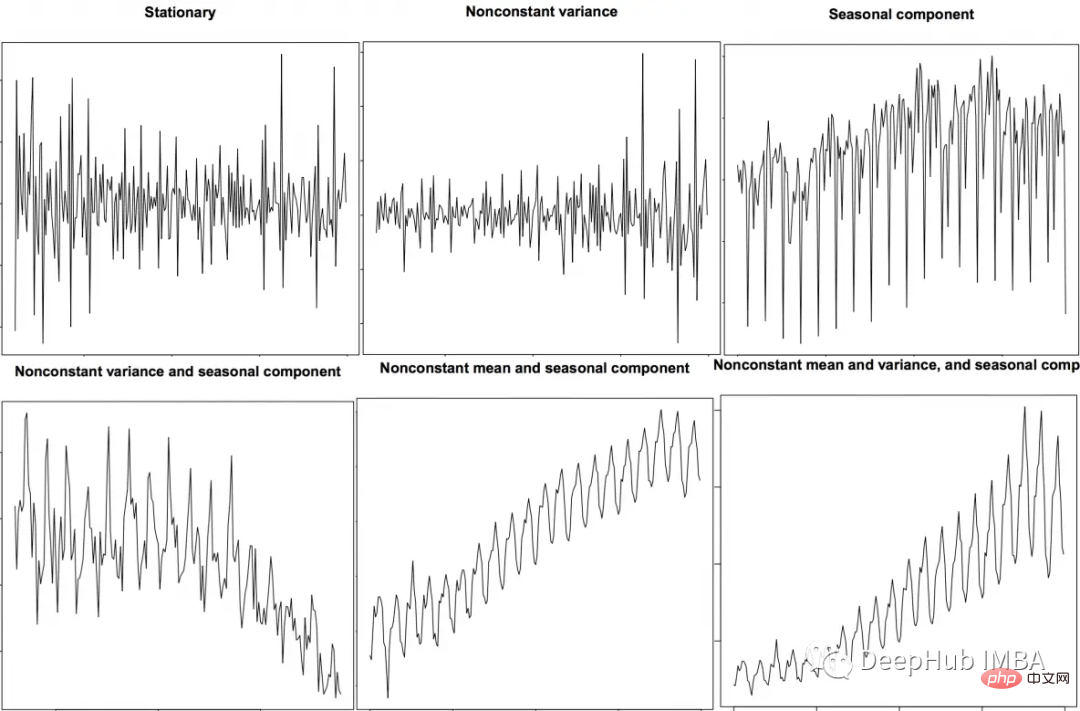
使用Pandas和Python从时间序列数据中提取有意义的特征,包括移动平均,自相关和傅里叶变换。前言时间序列分析是理解和预测各个行业(如金融、经济、医疗保健等)趋势的强大工具。特征提取是这一过程中的关键步骤,它涉及将原始数据转换为有意义的特征,可用于训练模型进行预测和分析。在本文中,我们将探索使用Python和Pandas的时间序列特征提取技术。在深入研究特征提取之前,让我们简要回顾一下时间序列数据。时间序列数据是按时间顺序索引的数据点序列。时间序列数据的例子包括股票价格、温度测量和交通数据。
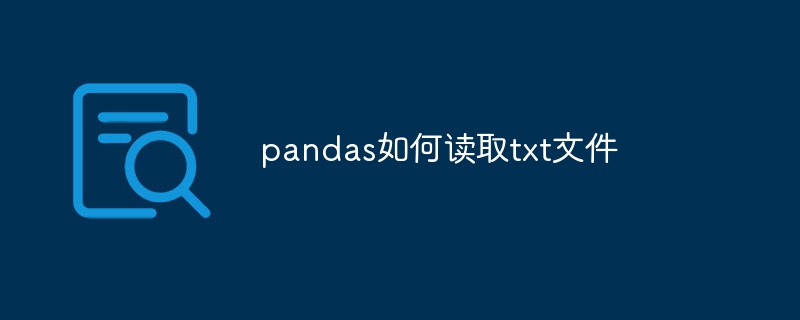
pandas读取txt文件的步骤:1、安装Pandas库;2、使用“read_csv”函数读取txt文件,并指定文件路径和文件分隔符;3、Pandas将数据读取为一个名为DataFrame的对象;4、如果第一行包含列名,则可以通过将header参数设置为0来指定,如果没有,则设置为None;5、如果txt文件中包含缺失值或空值,可以使用“na_values”指定这些缺失值。
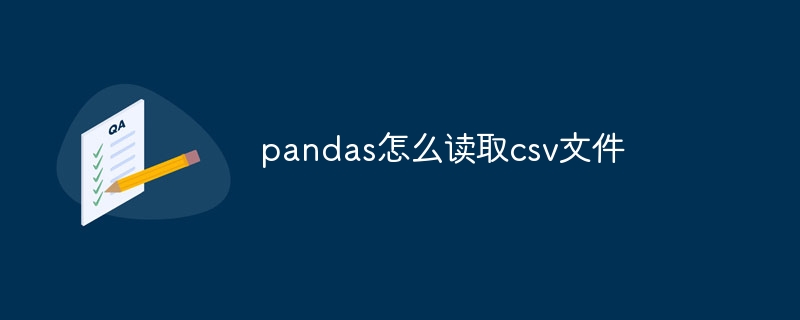
读取CSV文件的方法有使用read_csv()函数、指定分隔符、指定列名、跳过行、缺失值处理、自定义数据类型等。详细介绍:1、read_csv()函数是Pandas中最常用的读取CSV文件的方法。它可以从本地文件系统或远程URL加载CSV数据,并返回一个DataFrame对象;2、指定分隔符,默认情况下,read_csv()函数将使用逗号作为CSV文件的分隔符等等。
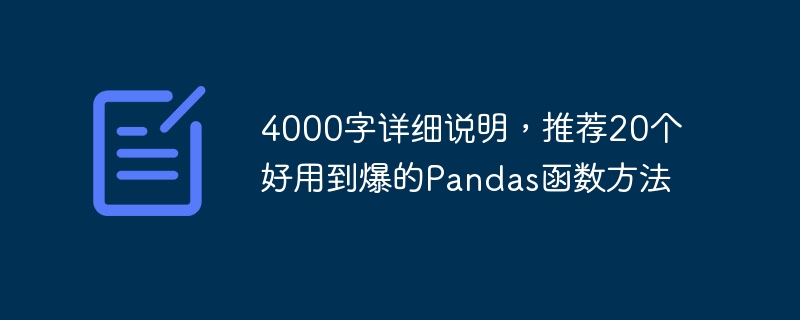
今天分享几个不为人知的pandas函数,大家可能平时看到的不多,但是使用起来倒是非常的方便,也能够帮助我们数据分析人员大幅度地提高工作效率,同时也希望大家看完之后能够有所收获。


Hot AI Tools

Undresser.AI Undress
AI-powered app for creating realistic nude photos

AI Clothes Remover
Online AI tool for removing clothes from photos.

Undress AI Tool
Undress images for free

Clothoff.io
AI clothes remover

AI Hentai Generator
Generate AI Hentai for free.

Hot Article

Hot Tools

Safe Exam Browser
Safe Exam Browser is a secure browser environment for taking online exams securely. This software turns any computer into a secure workstation. It controls access to any utility and prevents students from using unauthorized resources.
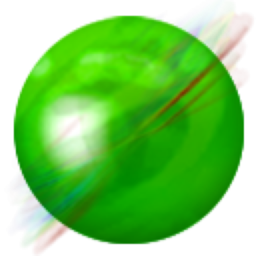
ZendStudio 13.5.1 Mac
Powerful PHP integrated development environment

SublimeText3 English version
Recommended: Win version, supports code prompts!

Zend Studio 13.0.1
Powerful PHP integrated development environment
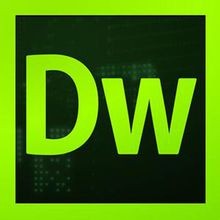
Dreamweaver CS6
Visual web development tools
