As we move deeper into the world of ML and GenAI, an emphasis on data quality becomes critical. John Jeske, CTO of KMS Technology's Advanced Technology Innovation Group, delves into data governance methods such as data lineage tracking and federated learning to ensure top model performance.
Data quality is key to model sustainability and stakeholder trust. During the modeling process, data quality makes long-term maintenance easier and enables you to build user confidence and confidence among your stakeholder community. The effects of ‘garbage in, garbage out’ are exacerbated in complex models, including large-scale languages and generative algorithms. "Jeske said.
Genetic AI Bias and Data Representative Issues
No matter which model you choose for your use case, poor data quality will inevitably lead to distortion of GenAI models. Pitfalls are often From training data that misrepresents the scope of the company, customer base, or application scope.
The real wealth is in the data itself, not the ephemeral model or modeling structure. Over the past few months , with the emergence of a large number of modeling frameworks, the value of data as a monetizable asset has become more prominent
Jeff Scott, senior vice president of software services at KMS Technology, further explained: "When the content generated by AI is consistent with the expected output When there is a bias, it is not an algorithm error, but a reflection of insufficient or distorted training data
Strict data integrity governance
Best practices for data governance include metadata management, data management and automation Quality inspection and other activities. For example, ensure the reliability of data sources, use certified datasets when acquiring data for training and modeling, and consider using automated data quality tools. Although this may add complexity, these tools are very helpful in ensuring data integrity
To improve data quality, we use tools that provide properties such as data validity, integrity checks, and time consistency, which Promotes reliable, consistent data, which is essential for robust AI models.
Accountability and continuous improvement in AI development
In everyone’s eyes, data is a problem. Within a company, assigning responsibility for data governance is an important task
The most important thing is to ensure that features work as designed and that the data being trained on makes sense from a potential customer's perspective. Feedback enhances learning, which is then taken into account the next time the model is trained, invoking continuous improvement until the point of trust.
In our workflow, AI and ML models undergo rigorous internal testing before being launched publicly. The data engineering team receives continuous feedback, allowing iterative improvements to the model to minimize biases and other anomalies
Risk Management and Customer Trust
Data governance needs to be implemented in relevant business areas Conduct data management and require ongoing involvement of subject matter experts to ensure data across teams and systems is appropriately curated and consistently accountable
Must understand the risks associated with receiving inaccurate results from technology, companies Transparency must be assessed, from data origins and handling of intellectual property to overall data quality and completeness.
Transparency is integral to customer trust, and data governance is not just a technical exercise, it can also impact a company’s reputation as risks are transferred from inaccurate AI predictions to end users.
With the continuous development of GenAI, mastering data governance has become increasingly important. This is not only to ensure data quality, but also to understand the complex relationship between data and AI models. This insight is critical to technological advancement, business health and maintaining the trust of stakeholders and the wider public
The above is the detailed content of Data governance blueprint in the GenAI era. For more information, please follow other related articles on the PHP Chinese website!
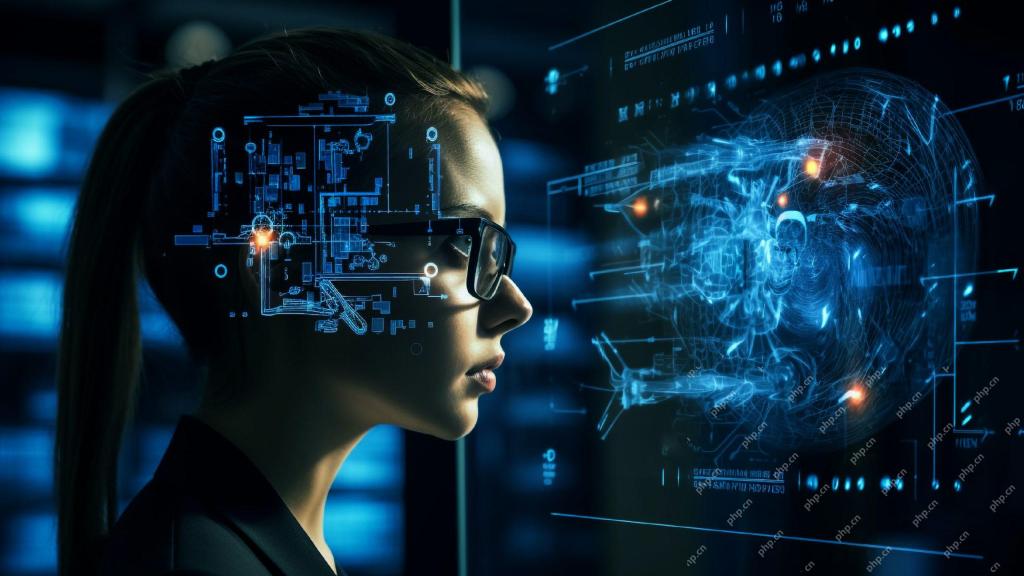
Expert Systems: A Deep Dive into AI's Decision-Making Power Imagine having access to expert advice on anything, from medical diagnoses to financial planning. That's the power of expert systems in artificial intelligence. These systems mimic the pro
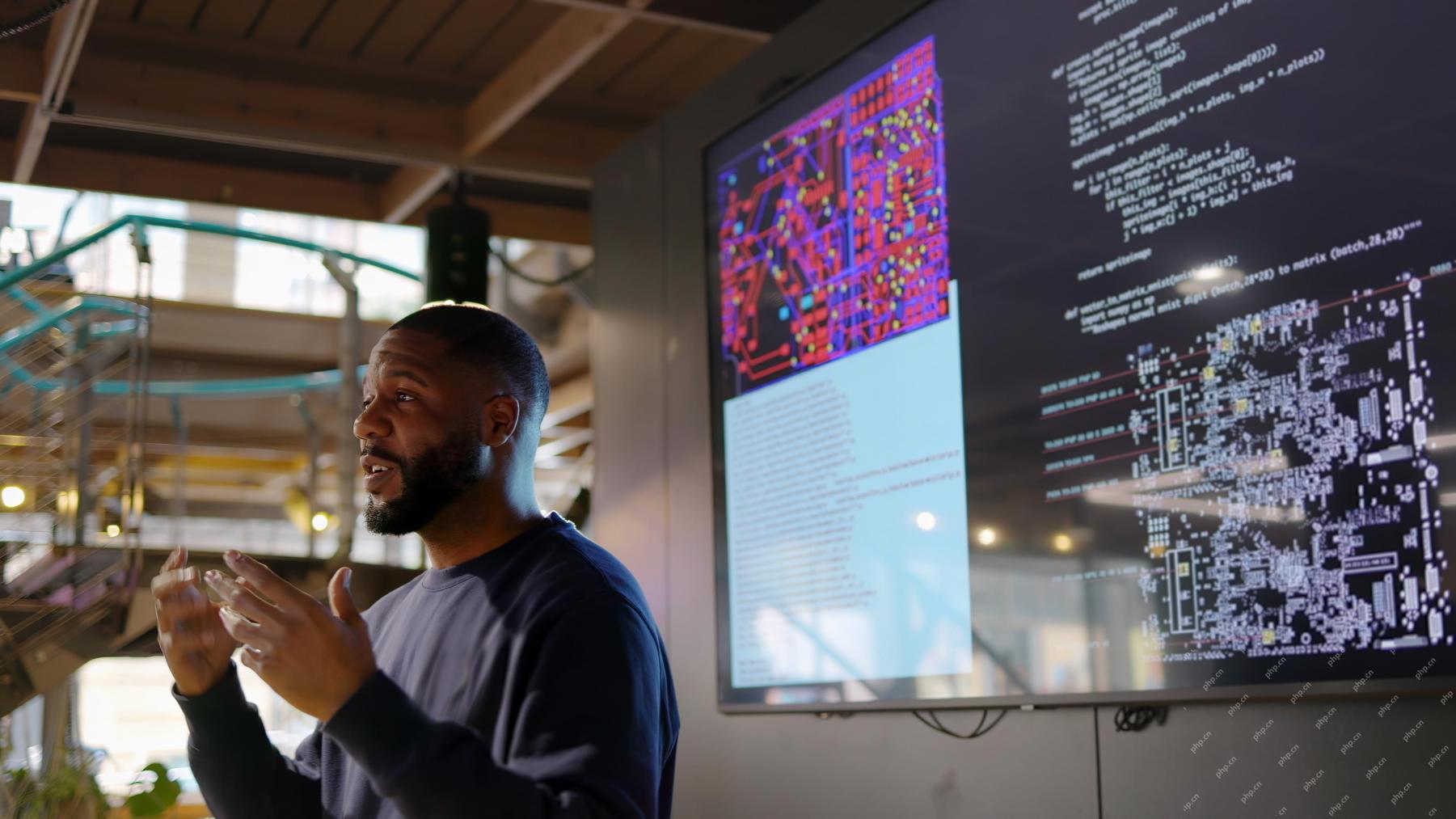
First of all, it’s apparent that this is happening quickly. Various companies are talking about the proportions of their code that are currently written by AI, and these are increasing at a rapid clip. There’s a lot of job displacement already around
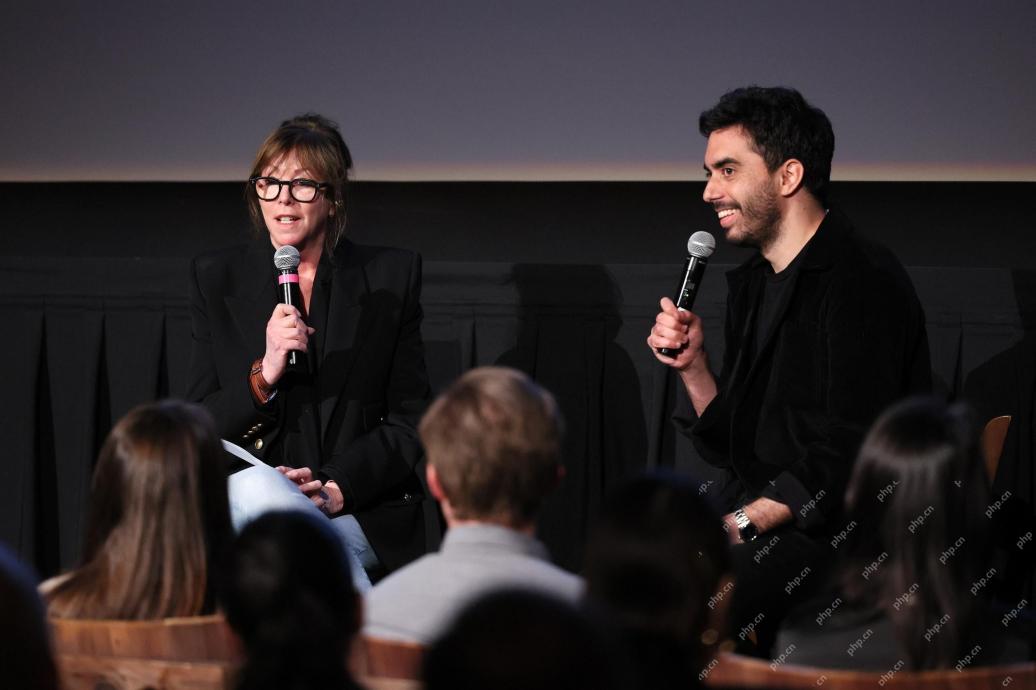
The film industry, alongside all creative sectors, from digital marketing to social media, stands at a technological crossroad. As artificial intelligence begins to reshape every aspect of visual storytelling and change the landscape of entertainment
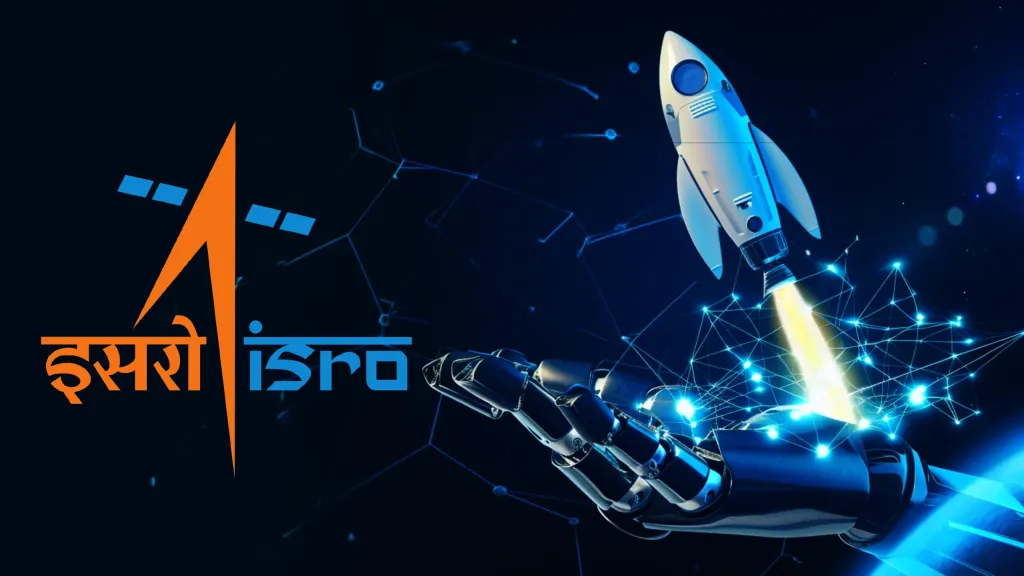
ISRO's Free AI/ML Online Course: A Gateway to Geospatial Technology Innovation The Indian Space Research Organisation (ISRO), through its Indian Institute of Remote Sensing (IIRS), is offering a fantastic opportunity for students and professionals to
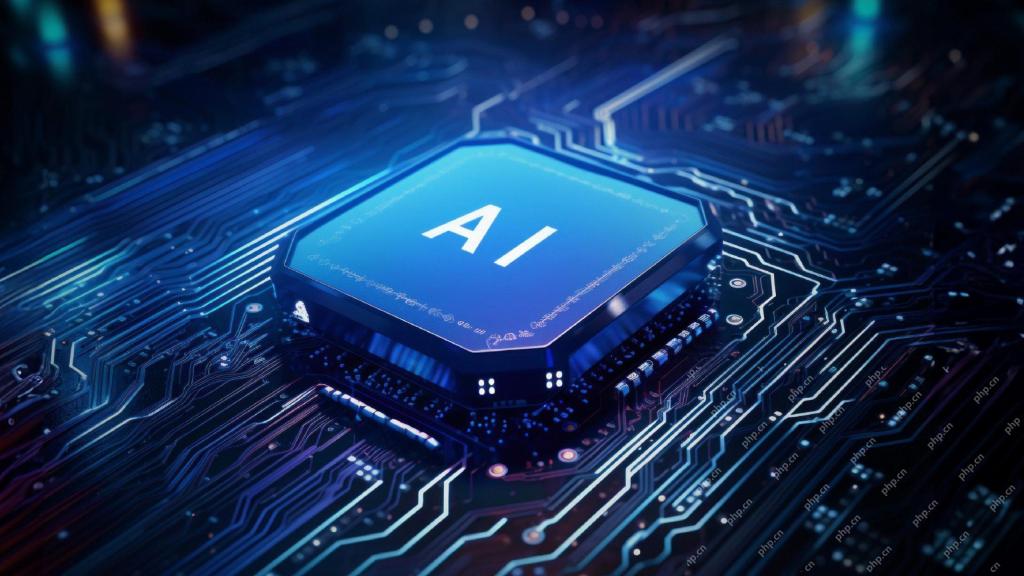
Local Search Algorithms: A Comprehensive Guide Planning a large-scale event requires efficient workload distribution. When traditional approaches fail, local search algorithms offer a powerful solution. This article explores hill climbing and simul
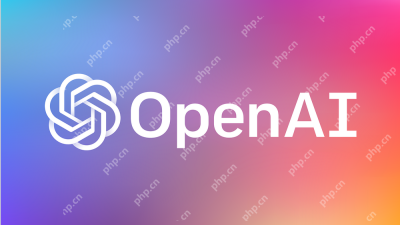
The release includes three distinct models, GPT-4.1, GPT-4.1 mini and GPT-4.1 nano, signaling a move toward task-specific optimizations within the large language model landscape. These models are not immediately replacing user-facing interfaces like
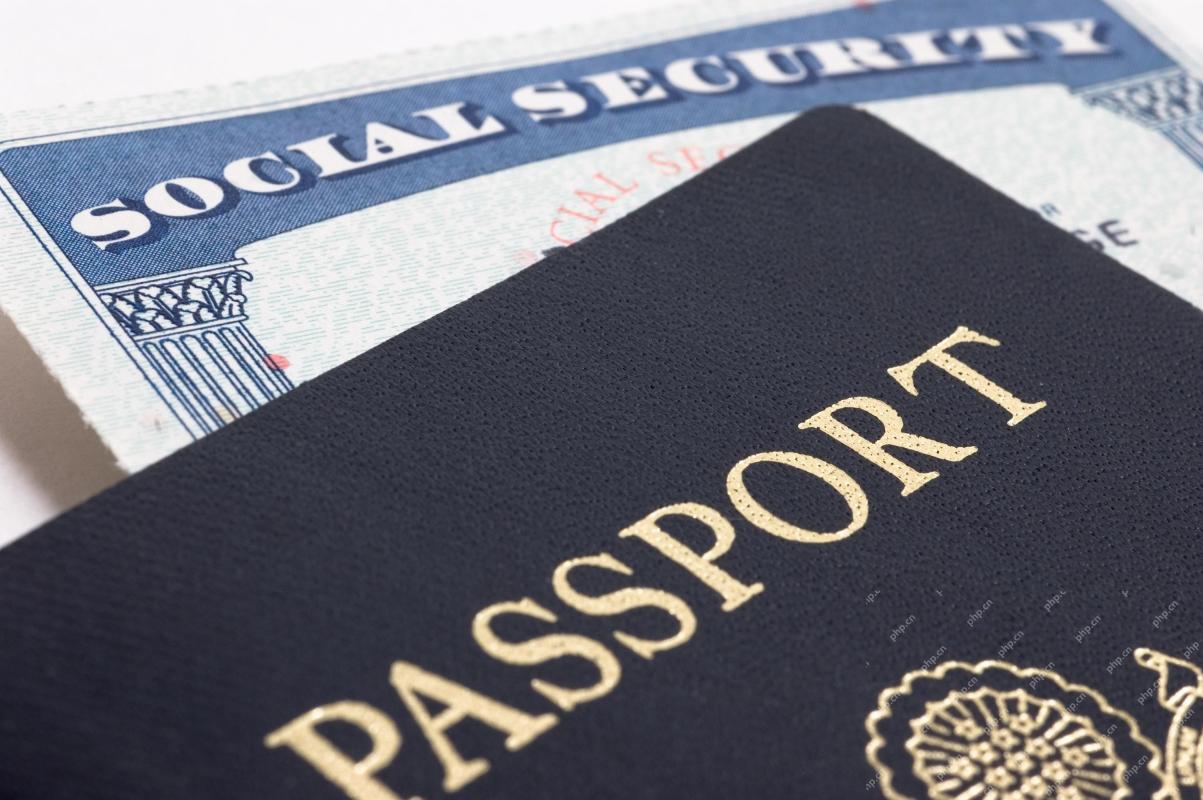
Chip giant Nvidia said on Monday it will start manufacturing AI supercomputers— machines that can process copious amounts of data and run complex algorithms— entirely within the U.S. for the first time. The announcement comes after President Trump si
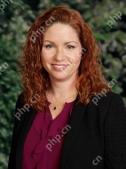
In a recent interview with Wendy Collins, chief AI officer at NTT DATA — a part of the global conglomerate NTT Group and innovator of IT and business services — she noted that this is a trend that we will begin to see more and more across the enterpr


Hot AI Tools

Undresser.AI Undress
AI-powered app for creating realistic nude photos

AI Clothes Remover
Online AI tool for removing clothes from photos.

Undress AI Tool
Undress images for free

Clothoff.io
AI clothes remover

AI Hentai Generator
Generate AI Hentai for free.

Hot Article

Hot Tools

SAP NetWeaver Server Adapter for Eclipse
Integrate Eclipse with SAP NetWeaver application server.
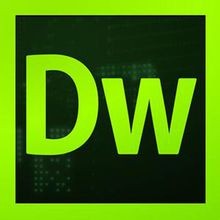
Dreamweaver CS6
Visual web development tools

Zend Studio 13.0.1
Powerful PHP integrated development environment
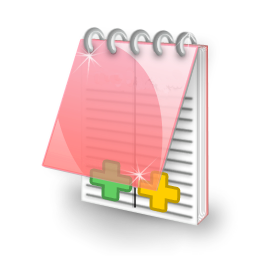
EditPlus Chinese cracked version
Small size, syntax highlighting, does not support code prompt function

MinGW - Minimalist GNU for Windows
This project is in the process of being migrated to osdn.net/projects/mingw, you can continue to follow us there. MinGW: A native Windows port of the GNU Compiler Collection (GCC), freely distributable import libraries and header files for building native Windows applications; includes extensions to the MSVC runtime to support C99 functionality. All MinGW software can run on 64-bit Windows platforms.