


As one of the most cutting-edge fields of technology, deep learning is often considered the key to technological progress. However, are there some cases where deep learning is not as effective as traditional methods? This article summarizes some high-quality answers from Zhihu to answer this question
Question link: https://www.zhihu.com/question/451498156
# Answer 1
Author: Jueleizai
Source link: https://www.zhihu.com/question /451498156/answer/1802577845
For fields that require interpretability, basic deep learning is incomparable with traditional methods. I have been working on risk control/anti-money laundering products for the past few years, but regulations require our decisions to be explainable. We have tried deep learning, but explainability is difficult to achieve, and the results are not very good. For risk control scenarios, data cleaning is very important, otherwise it will just be garbage in garbage out.
When writing the above content, I remembered an article I read two years ago: "You don’t need ML/AI, you need SQL"
https://www.php.cn/link/f0e1f0412f36e086dc5f596b84370e86
The author is Celestine Omin, a Nigerian software engineer, the largest e-commerce website in Nigeria One Konga works. We all know that precision marketing and personalized recommendations for old users are one of the most commonly used areas of AI. When others are using deep learning to make recommendations, his method seems extremely simple. He just ran through the database, screened out all users who had not logged in for three months, and pushed coupons to them. It also ran through the product list in the user's shopping cart and decided to recommend related products based on these popular products.
As a result, with his simple SQL-based personalized recommendations, the open rate of most marketing emails is between 7-10%, and when done well, the open rate is close to 25% -30%, three times the industry average open rate.
Of course, this example is not to tell you that the recommendation algorithm is useless and everyone should use SQL. It means that when applying deep learning, you need to consider constraints such as cost and application scenarios. . In my previous answer (What exactly does the implementation ability of an algorithm engineer refer to?), I mentioned that practical constraints need to be considered when implementing algorithms.
https://www.php.cn/link/f0e1f0412f36e086dc5f596b84370e86
And Nigeria’s e-commerce environment, It is still in a very backward state, and logistics cannot keep up. Even if the deep learning method is used to improve the effect, it will not actually have much impact on the company's overall profits.
Therefore, the algorithm must be "adapted to local conditions" when implemented. Otherwise, the situation of "the electric fan blowing the soap box" will occur again.
A large company introduced a soap packaging production line, but found that this production line had a flaw: there were often boxes without soap. They couldn't sell empty boxes to customers, so they had to hire a postdoc who studied automation to design a plan to sort empty soap boxes.
The postdoctoral fellow organized a scientific research team of more than a dozen people and used a combination of machinery, microelectronics, automation, X-ray detection and other technologies, spending 900,000 yuan to successfully solve the problem. Whenever an empty soap box passes through the production line, detectors on both sides will detect it and drive a robot to push the empty soap box away.
There was a township enterprise in southern China that also bought the same production line. When the boss found out about this problem, he got very angry and hired a small worker to say, "You can fix this for me, or else you can get away from me." The worker quickly figured out a way. He spent 190 yuan to place a high-power electric fan next to the production line and blew it hard, so that all the empty soap boxes were blown away.
(Although it’s just a joke)
Deep learning is a hammer, but not everything in the world is a nail.
# Answer 2
Author: Mo Xiao Fourier
Source link: https://www.zhihu.com/question/ 451498156/answer/1802730183
There are two common scenarios:
1. Scenarios that pursue explainability.
Deep learning is very good at solving classification and regression problems, but its explanation of what affects the results is very weak. In actual business scenarios, the requirements for interpretability are very high, such as In the following scenarios, deep learning is often overturned.
2. Many operations optimization scenarios
such as scheduling, planning, and allocation problems, often such The problem does not translate well into a supervised learning format, so optimization algorithms are often used. In current research, deep learning algorithms are often integrated into the solution process for better solutions, but in general, the model itself is not yet deep learning as the backbone.
Deep learning is a very good solution, but it is not the only one. Even when implemented, there are still big problems. If deep learning is integrated into the optimization algorithm, it can still be of great use as a component of the solution.
In short,
Answer 3
Author: LinT
Source link: https://www.zhihu.com/question/451498156/answer/1802516688
This question should be looked at based on scenarios. Although deep learning eliminates the trouble of feature engineering, it may be difficult to apply in some scenarios:
- Applications have high requirements on latency, but not so high accuracy. requirements, a simple model may be a better choice at this time;
- Some data types, such as tabular data, may be more suitable to use statistical learning models such as tree-based models instead of deep Learning model;
- Model decisions have a significant impact, such as safety-related and economic decision-making, and the model is required to be interpretable. Then linear models or tree-based models are more suitable than deep learning. Good choice;
- The application scenario determines the difficulty of data collection, and there is a risk of over-fitting when using deep learning
Real Applications are all based on demand. It is unscientific to talk about performance regardless of demand (accuracy, latency, computing power consumption). If the "dry translation" in the question is limited to a certain indicator, the scope of discussion may be narrowed.
Original link: https://mp.weixin.qq.com/s/tO2OD772qCntNytwqPjUsA
The above is the detailed content of What are some classic cases where deep learning is not as effective as traditional methods?. For more information, please follow other related articles on the PHP Chinese website!
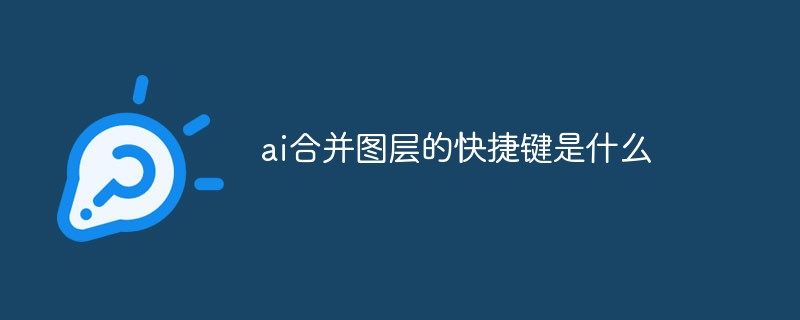
ai合并图层的快捷键是“Ctrl+Shift+E”,它的作用是把目前所有处在显示状态的图层合并,在隐藏状态的图层则不作变动。也可以选中要合并的图层,在菜单栏中依次点击“窗口”-“路径查找器”,点击“合并”按钮。
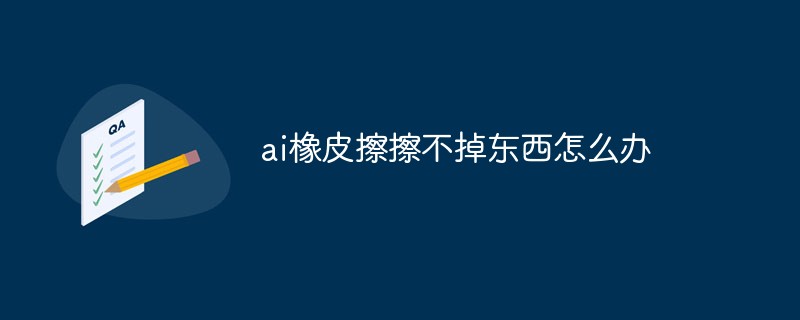
ai橡皮擦擦不掉东西是因为AI是矢量图软件,用橡皮擦不能擦位图的,其解决办法就是用蒙板工具以及钢笔勾好路径再建立蒙板即可实现擦掉东西。
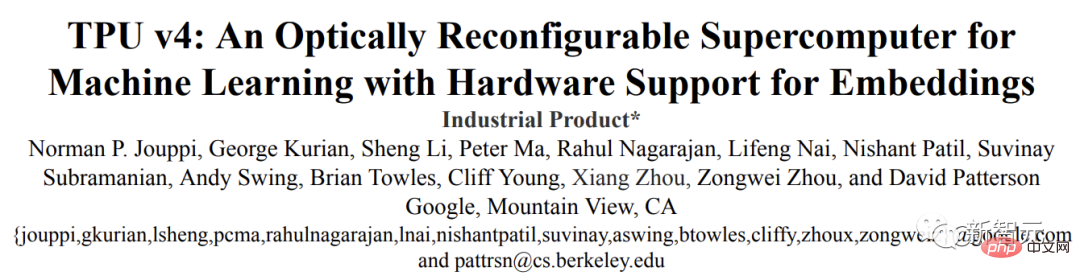
虽然谷歌早在2020年,就在自家的数据中心上部署了当时最强的AI芯片——TPU v4。但直到今年的4月4日,谷歌才首次公布了这台AI超算的技术细节。论文地址:https://arxiv.org/abs/2304.01433相比于TPU v3,TPU v4的性能要高出2.1倍,而在整合4096个芯片之后,超算的性能更是提升了10倍。另外,谷歌还声称,自家芯片要比英伟达A100更快、更节能。与A100对打,速度快1.7倍论文中,谷歌表示,对于规模相当的系统,TPU v4可以提供比英伟达A100强1.
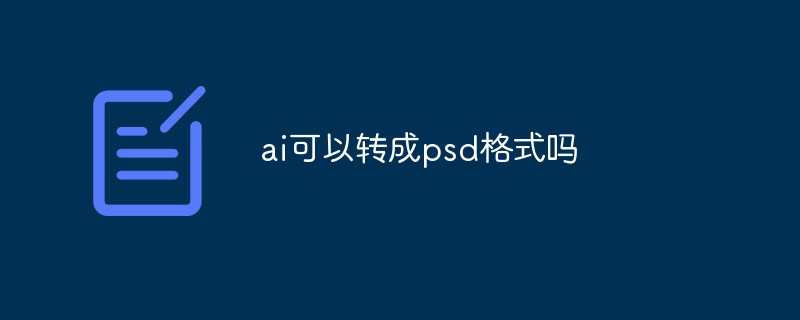
ai可以转成psd格式。转换方法:1、打开Adobe Illustrator软件,依次点击顶部菜单栏的“文件”-“打开”,选择所需的ai文件;2、点击右侧功能面板中的“图层”,点击三杠图标,在弹出的选项中选择“释放到图层(顺序)”;3、依次点击顶部菜单栏的“文件”-“导出”-“导出为”;4、在弹出的“导出”对话框中,将“保存类型”设置为“PSD格式”,点击“导出”即可;
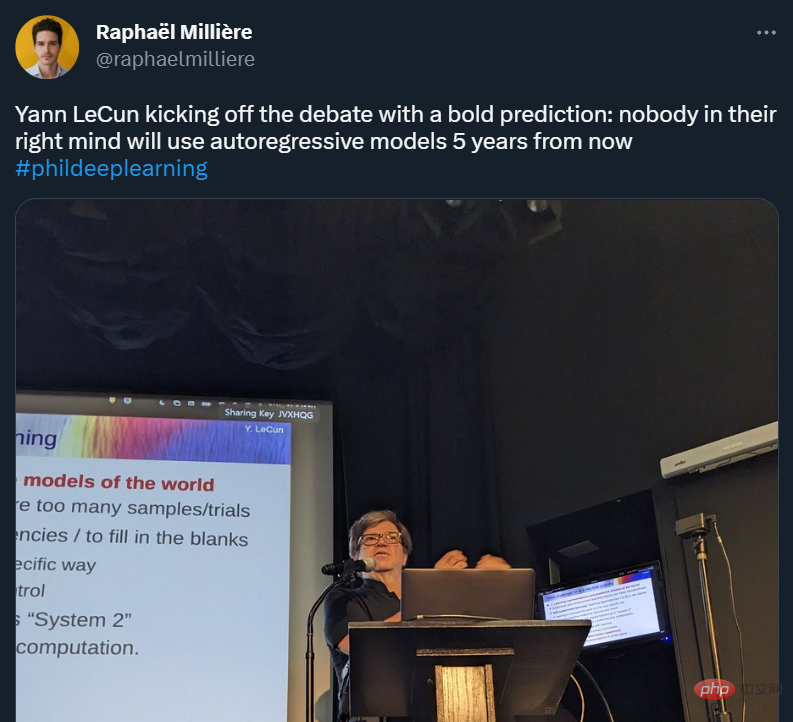
Yann LeCun 这个观点的确有些大胆。 「从现在起 5 年内,没有哪个头脑正常的人会使用自回归模型。」最近,图灵奖得主 Yann LeCun 给一场辩论做了个特别的开场。而他口中的自回归,正是当前爆红的 GPT 家族模型所依赖的学习范式。当然,被 Yann LeCun 指出问题的不只是自回归模型。在他看来,当前整个的机器学习领域都面临巨大挑战。这场辩论的主题为「Do large language models need sensory grounding for meaning and u
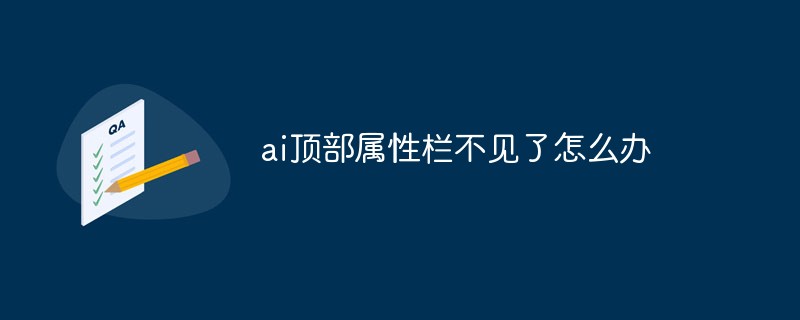
ai顶部属性栏不见了的解决办法:1、开启Ai新建画布,进入绘图页面;2、在Ai顶部菜单栏中点击“窗口”;3、在系统弹出的窗口菜单页面中点击“控制”,然后开启“控制”窗口即可显示出属性栏。
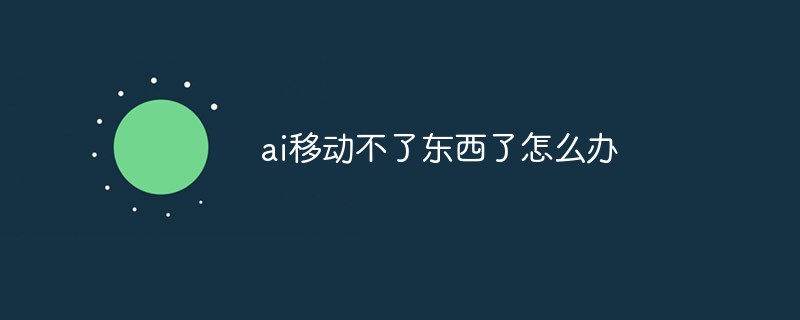
ai移动不了东西的解决办法:1、打开ai软件,打开空白文档;2、选择矩形工具,在文档中绘制矩形;3、点击选择工具,移动文档中的矩形;4、点击图层按钮,弹出图层面板对话框,解锁图层;5、点击选择工具,移动矩形即可。
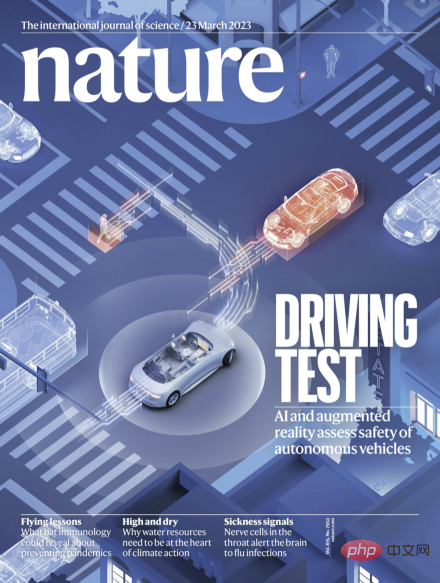
引入密集强化学习,用 AI 验证 AI。 自动驾驶汽车 (AV) 技术的快速发展,使得我们正处于交通革命的风口浪尖,其规模是自一个世纪前汽车问世以来从未见过的。自动驾驶技术具有显着提高交通安全性、机动性和可持续性的潜力,因此引起了工业界、政府机构、专业组织和学术机构的共同关注。过去 20 年里,自动驾驶汽车的发展取得了长足的进步,尤其是随着深度学习的出现更是如此。到 2015 年,开始有公司宣布他们将在 2020 之前量产 AV。不过到目前为止,并且没有 level 4 级别的 AV 可以在市场


Hot AI Tools

Undresser.AI Undress
AI-powered app for creating realistic nude photos

AI Clothes Remover
Online AI tool for removing clothes from photos.

Undress AI Tool
Undress images for free

Clothoff.io
AI clothes remover

AI Hentai Generator
Generate AI Hentai for free.

Hot Article

Hot Tools

SAP NetWeaver Server Adapter for Eclipse
Integrate Eclipse with SAP NetWeaver application server.
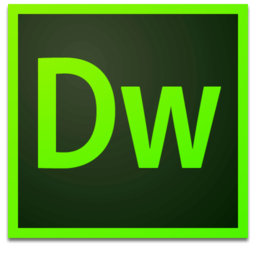
Dreamweaver Mac version
Visual web development tools
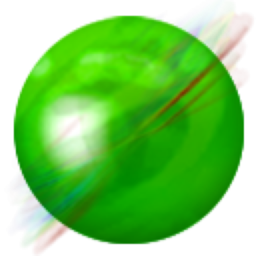
ZendStudio 13.5.1 Mac
Powerful PHP integrated development environment

Atom editor mac version download
The most popular open source editor

SublimeText3 Linux new version
SublimeText3 Linux latest version
