


Research on methods to solve stability problems encountered in MongoDB technology development
Research on methods to solve stability problems encountered in MongoDB technology development
Introduction:
With the advent of the big data era, the evolution of data storage and processing Demand is also growing. As a high-performance, scalable, non-relational database, MongoDB has demonstrated strong advantages in many application scenarios. However, when using MongoDB for technical development, stability issues often become a headache for developers. Therefore, this article will explore ways to resolve common stability issues in MongoDB technology development and provide specific code examples.
- Connection management issues
Since MongoDB is a distributed database system, connection management has become a common stability issue. During the development process, we often encounter problems such as connection pool exhaustion, connection timeout, and connection disconnection caused by too many connections. In order to solve these problems, we can consider the following aspects:
1.1 Set the connection pool parameters reasonably:
When using the MongoDB client driver, you can set the connection pool according to actual needs parameters, such as the maximum number of connections, the minimum number of connections, connection timeout, etc. A reasonable connection pool configuration can help us better manage connections and avoid stability problems caused by too many connections.
Code example:
from pymongo import MongoClient def connect_mongodb(): client = MongoClient("mongodb://localhost:27017") # 设置最大连接数为100,最小连接数为10,连接超时时间为5秒 client.max_pool_size = 100 client.min_pool_size = 10 client.server_selection_timeout = 5000 return client
1.2 Regularly releasing connection resources:
After using the database connection, timely release of connection resources is an effective management method. We can realize the function of automatically releasing connection resources by writing connection pool code to ensure the stability of the database connection.
Code example:
from pymongo import MongoClient from pymongo.pool import Pool class MyConnectionPool(Pool): def __init__(self, max_connections=100, *args, **kwargs): super().__init__(max_connections, *args, **kwargs) self.connections = [] def create_connection(self): client = MongoClient("mongodb://localhost:27017") # 设置连接的超时时间 client.server_selection_timeout = 5000 self.connections.append(client) return client def get_connection(self): if self.connections: return self.connections.pop() return self.create_connection() def release_connection(self, connection): self.connections.append(connection) def close(self): for connection in self.connections: connection.close() self.connections = [] pool = MyConnectionPool(max_connections=10)
- Writing operation problems
During the writing operation of MongoDB, problems such as data loss and writing delay are often encountered. In order to solve these problems, we need to pay attention to the following points:
2.1 Set the write concern level appropriately:
MongoDB provides a variety of write concern levels, such as majority, acknowledged, etc. We can choose the appropriate write attention level based on actual needs to ensure write stability. It is worth noting that the write concern level will have a certain impact on the performance of write operations, so the choice needs to be weighed.
Code example:
from pymongo import MongoClient def write_to_mongodb(): client = MongoClient("mongodb://localhost:27017") # 设置写入关注级别为 majority client.write_concern = {'w': 'majority'} db = client['mydb'] collection = db['mycollection'] collection.insert_one({'name': 'Alice'})
2.2 Batch writing data:
In order to improve the efficiency of writing operations, we can consider using batch writing. By packaging multiple write operations into one request, network overhead and write latency can be reduced, and write stability can be improved.
Code sample:
from pymongo import MongoClient def bulk_write_to_mongodb(): client = MongoClient("mongodb://localhost:27017") db = client['mydb'] collection = db['mycollection'] # 批量写入数据 requests = [InsertOne({'name': 'Alice'}), InsertOne({'name': 'Bob'})] collection.bulk_write(requests)
Conclusion:
By setting the connection pool parameters appropriately, releasing connection resources regularly, setting the write attention level reasonably, and using batch writing data, we can Solve common stability problems in MongoDB technology development. Of course, specific solutions need to be customized according to specific business scenarios and needs. With the continuous deepening and accumulation of MongoDB practice, we can deepen our understanding of MongoDB stability issues and provide more effective solutions.
Note: The above code examples are for reference only, please adjust and optimize according to the actual situation.
The above is the detailed content of Research on methods to solve stability problems encountered in MongoDB technology development. For more information, please follow other related articles on the PHP Chinese website!
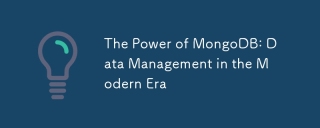
MongoDB is a NoSQL database because of its flexibility and scalability are very important in modern data management. It uses document storage, is suitable for processing large-scale, variable data, and provides powerful query and indexing capabilities.
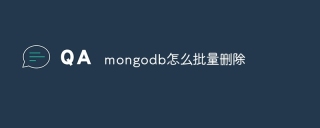
You can use the following methods to delete documents in MongoDB: 1. The $in operator specifies the list of documents to be deleted; 2. The regular expression matches documents that meet the criteria; 3. The $exists operator deletes documents with the specified fields; 4. The find() and remove() methods first get and then delete the document. Please note that these operations cannot use transactions and may delete all matching documents, so be careful when using them.
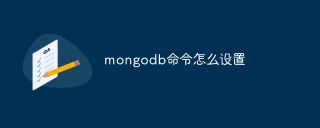
To set up a MongoDB database, you can use the command line (use and db.createCollection()) or the mongo shell (mongo, use and db.createCollection()). Other setting options include viewing database (show dbs), viewing collections (show collections), deleting database (db.dropDatabase()), deleting collections (db.<collection_name>.drop()), inserting documents (db.<collecti
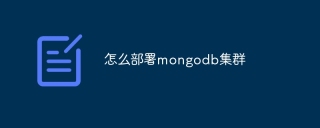
Deploying a MongoDB cluster is divided into five steps: deploying the primary node, deploying the secondary node, adding the secondary node, configuring replication, and verifying the cluster. Including installing MongoDB software, creating data directories, starting MongoDB instances, initializing replication sets, adding secondary nodes, enabling replica set features, configuring voting rights, and verifying cluster status and data replication.
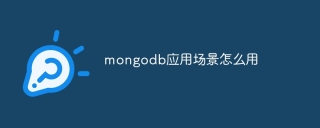
MongoDB is widely used in the following scenarios: Document storage: manages structured and unstructured data such as user information, content, product catalogs, etc. Real-time analysis: Quickly query and analyze real-time data such as logs, monitoring dashboard displays, etc. Social Media: Manage user relationship maps, activity streams, and messaging. Internet of Things: Process massive time series data such as device monitoring, data collection and remote management. Mobile applications: As a backend database, synchronize mobile device data, provide offline storage, etc. Other areas: diversified scenarios such as e-commerce, healthcare, financial services and game development.
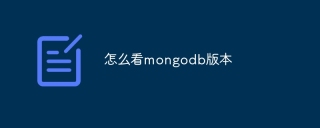
How to view MongoDB version: Command line: Use the db.version() command. Programming language driver: Python: print(client.server_info()["version"])Node.js: db.command({ version: 1 }, (err, result) => { console.log(result.version); });
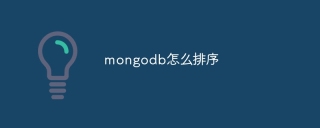
MongoDB provides a sorting mechanism to sort collections by specific fields, using the syntax db.collection.find().sort({ field: order }) ascending/descending order, supports compound sorting by multiple fields, and recommends creating indexes to improve sorting performance.
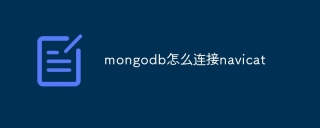
To connect to MongoDB with Navicat: Install Navicat and create a MongoDB connection; enter the server address in the host, enter the port number in the port, and enter the MongoDB authentication information in the user name and password; test the connection and save; Navicat will connect to the MongoDB server.


Hot AI Tools

Undresser.AI Undress
AI-powered app for creating realistic nude photos

AI Clothes Remover
Online AI tool for removing clothes from photos.

Undress AI Tool
Undress images for free

Clothoff.io
AI clothes remover

AI Hentai Generator
Generate AI Hentai for free.

Hot Article

Hot Tools

DVWA
Damn Vulnerable Web App (DVWA) is a PHP/MySQL web application that is very vulnerable. Its main goals are to be an aid for security professionals to test their skills and tools in a legal environment, to help web developers better understand the process of securing web applications, and to help teachers/students teach/learn in a classroom environment Web application security. The goal of DVWA is to practice some of the most common web vulnerabilities through a simple and straightforward interface, with varying degrees of difficulty. Please note that this software
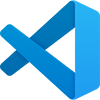
VSCode Windows 64-bit Download
A free and powerful IDE editor launched by Microsoft

MinGW - Minimalist GNU for Windows
This project is in the process of being migrated to osdn.net/projects/mingw, you can continue to follow us there. MinGW: A native Windows port of the GNU Compiler Collection (GCC), freely distributable import libraries and header files for building native Windows applications; includes extensions to the MSVC runtime to support C99 functionality. All MinGW software can run on 64-bit Windows platforms.
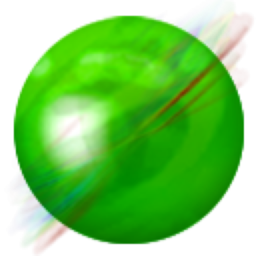
ZendStudio 13.5.1 Mac
Powerful PHP integrated development environment
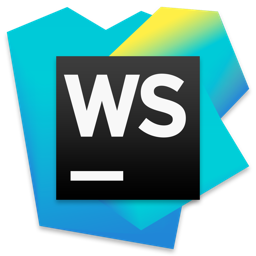
WebStorm Mac version
Useful JavaScript development tools