The impact of data scarcity on model training
The impact of data scarcity on model training requires specific code examples
In the fields of machine learning and artificial intelligence, data is one of the core elements of training models. However, a problem we often face in reality is data scarcity. Data scarcity refers to the insufficient amount of training data or the lack of annotated data. In this case, it will have a certain impact on model training.
The problem of data scarcity is mainly reflected in the following aspects:
- Overfitting: When the amount of training data is insufficient, the model is prone to overfitting. Overfitting means that the model overly adapts to the training data and cannot generalize well to new data. This is because the model does not have enough data samples to learn the distribution and characteristics of the data, causing the model to produce inaccurate prediction results.
- Underfitting: Compared with overfitting, underfitting means that the model cannot fit the training data well. This is because the amount of training data is insufficient to cover the diversity of the data, resulting in the model being unable to capture the complexity of the data. Underfitted models often fail to provide accurate predictions.
How to solve the problem of data scarcity and improve the performance of the model? The following are some commonly used methods and code examples:
- Data augmentation (Data Augmentation) is a common method to increase the number of training samples by transforming or expanding existing data. Common data enhancement methods include image rotation, flipping, scaling, cropping, etc. The following is a simple image rotation code example:
from PIL import Image def rotate_image(image, angle): rotated_image = image.rotate(angle) return rotated_image image = Image.open('image.jpg') rotated_image = rotate_image(image, 90) rotated_image.save('rotated_image.jpg')
- Transfer learning (Transfer Learning) uses already trained models to solve new problems. By using already learned features from existing models, better training can be performed on scarce data sets. The following is a code example of transfer learning:
from keras.applications import VGG16 from keras.models import Model base_model = VGG16(weights='imagenet', include_top=False, input_shape=(224, 224, 3)) x = base_model.output x = GlobalAveragePooling2D()(x) x = Dense(1024, activation='relu')(x) predictions = Dense(num_classes, activation='softmax')(x) model = Model(inputs=base_model.input, outputs=predictions) model.compile(optimizer='adam', loss='categorical_crossentropy', metrics=['accuracy'])
- Domain Adaptation (Domain Adaptation) is a method of transferring knowledge from the source domain to the target domain. Better generalization capabilities can be obtained by using some domain-adaptive techniques, such as self-supervised learning, domain adversarial networks, etc. The following is a code example of domain adaptation:
import torch import torchvision import torch.nn as nn source_model = torchvision.models.resnet50(pretrained=True) target_model = torchvision.models.resnet50(pretrained=False) for param in source_model.parameters(): param.requires_grad = False source_features = source_model.features(x) target_features = target_model.features(x) class DANNClassifier(nn.Module): def __init__(self, num_classes): super(DANNClassifier, self).__init__() self.fc = nn.Linear(2048, num_classes) def forward(self, x): x = self.fc(x) return x source_classifier = DANNClassifier(num_classes) target_classifier = DANNClassifier(num_classes) source_outputs = source_classifier(source_features) target_outputs = target_classifier(target_features)
Data scarcity has a non-negligible impact on model training. Through methods such as data augmentation, transfer learning, and domain adaptation, we can effectively solve the problem of data scarcity and improve the performance and generalization ability of the model. In practical applications, we should choose appropriate methods based on specific problems and data characteristics to obtain better results.
The above is the detailed content of The impact of data scarcity on model training. For more information, please follow other related articles on the PHP Chinese website!
![[Ghibli-style images with AI] Introducing how to create free images with ChatGPT and copyright](https://img.php.cn/upload/article/001/242/473/174707263295098.jpg?x-oss-process=image/resize,p_40)
The latest model GPT-4o released by OpenAI not only can generate text, but also has image generation functions, which has attracted widespread attention. The most eye-catching feature is the generation of "Ghibli-style illustrations". Simply upload the photo to ChatGPT and give simple instructions to generate a dreamy image like a work in Studio Ghibli. This article will explain in detail the actual operation process, the effect experience, as well as the errors and copyright issues that need to be paid attention to. For details of the latest model "o3" released by OpenAI, please click here⬇️ Detailed explanation of OpenAI o3 (ChatGPT o3): Features, pricing system and o4-mini introduction Please click here for the English version of Ghibli-style article⬇️ Create Ji with ChatGPT
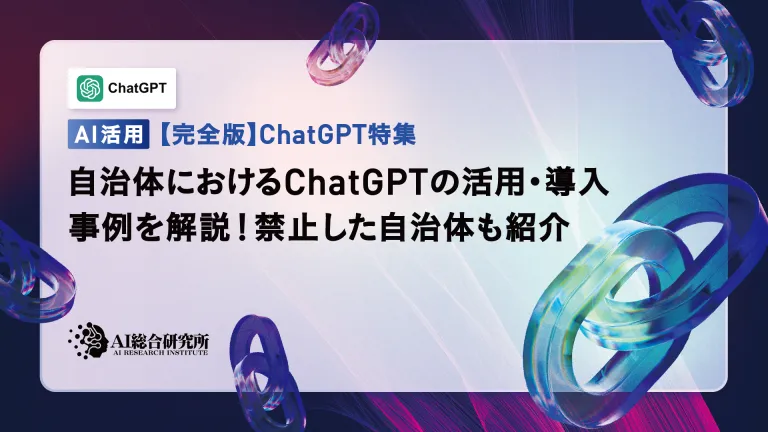
As a new communication method, the use and introduction of ChatGPT in local governments is attracting attention. While this trend is progressing in a wide range of areas, some local governments have declined to use ChatGPT. In this article, we will introduce examples of ChatGPT implementation in local governments. We will explore how we are achieving quality and efficiency improvements in local government services through a variety of reform examples, including supporting document creation and dialogue with citizens. Not only local government officials who aim to reduce staff workload and improve convenience for citizens, but also all interested in advanced use cases.
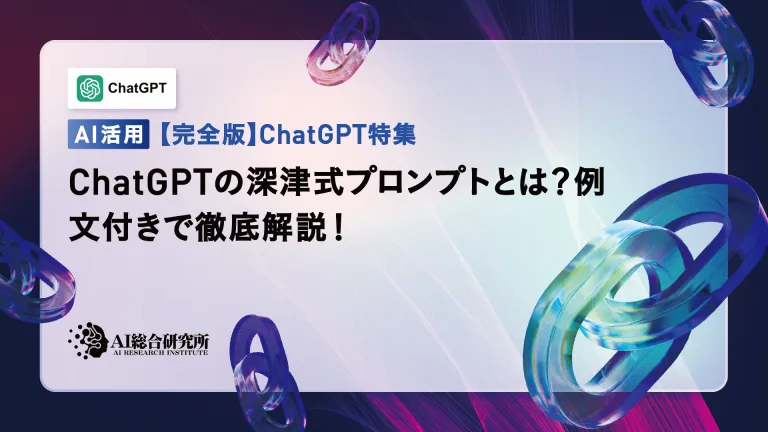
Have you heard of a framework called the "Fukatsu Prompt System"? Language models such as ChatGPT are extremely excellent, but appropriate prompts are essential to maximize their potential. Fukatsu prompts are one of the most popular prompt techniques designed to improve output accuracy. This article explains the principles and characteristics of Fukatsu-style prompts, including specific usage methods and examples. Furthermore, we have introduced other well-known prompt templates and useful techniques for prompt design, so based on these, we will introduce C.
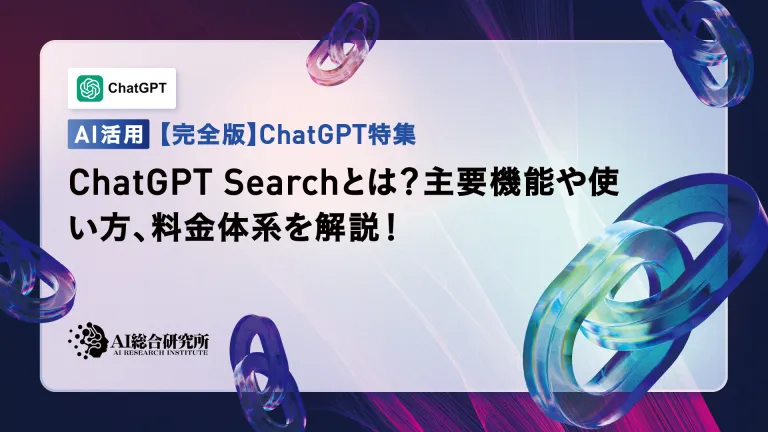
ChatGPT Search: Get the latest information efficiently with an innovative AI search engine! In this article, we will thoroughly explain the new ChatGPT feature "ChatGPT Search," provided by OpenAI. Let's take a closer look at the features, usage, and how this tool can help you improve your information collection efficiency with reliable answers based on real-time web information and intuitive ease of use. ChatGPT Search provides a conversational interactive search experience that answers user questions in a comfortable, hidden environment that hides advertisements
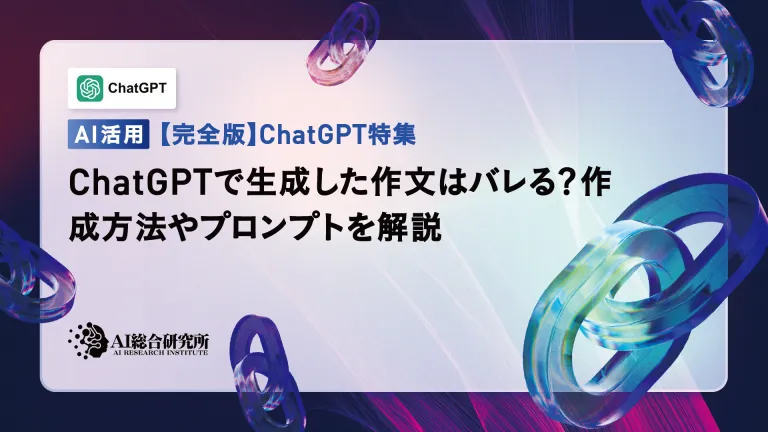
In a modern society with information explosion, it is not easy to create compelling articles. How to use creativity to write articles that attract readers within a limited time and energy requires superb skills and rich experience. At this time, as a revolutionary writing aid, ChatGPT attracted much attention. ChatGPT uses huge data to train language generation models to generate natural, smooth and refined articles. This article will introduce how to effectively use ChatGPT and efficiently create high-quality articles. We will gradually explain the writing process of using ChatGPT, and combine specific cases to elaborate on its advantages and disadvantages, applicable scenarios, and safe use precautions. ChatGPT will be a writer to overcome various obstacles,
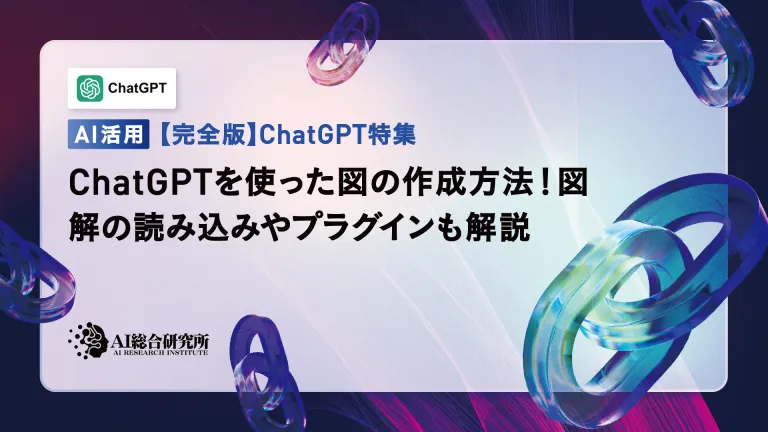
An efficient guide to creating charts using AI Visual materials are essential to effectively conveying information, but creating it takes a lot of time and effort. However, the chart creation process is changing dramatically due to the rise of AI technologies such as ChatGPT and DALL-E 3. This article provides detailed explanations on efficient and attractive diagram creation methods using these cutting-edge tools. It covers everything from ideas to completion, and includes a wealth of information useful for creating diagrams, from specific steps, tips, plugins and APIs that can be used, and how to use the image generation AI "DALL-E 3."
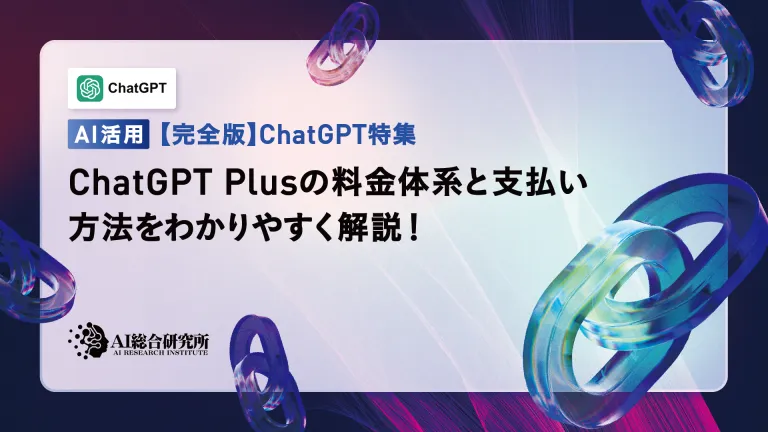
Unlock ChatGPT Plus: Fees, Payment Methods and Upgrade Guide ChatGPT, a world-renowned generative AI, has been widely used in daily life and business fields. Although ChatGPT is basically free, the paid version of ChatGPT Plus provides a variety of value-added services, such as plug-ins, image recognition, etc., which significantly improves work efficiency. This article will explain in detail the charging standards, payment methods and upgrade processes of ChatGPT Plus. For details of OpenAI's latest image generation technology "GPT-4o image generation" please click: Detailed explanation of GPT-4o image generation: usage methods, prompt word examples, commercial applications and differences from other AIs Table of contents ChatGPT Plus Fees Ch
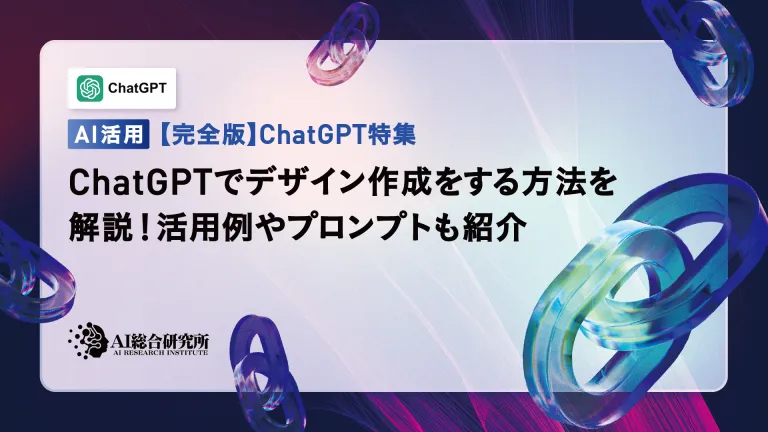
How to use ChatGPT to streamline your design work and increase creativity This article will explain in detail how to create a design using ChatGPT. We will introduce examples of using ChatGPT in various design fields, such as ideas, text generation, and web design. We will also introduce points that will help you improve the efficiency and quality of a variety of creative work, such as graphic design, illustration, and logo design. Please take a look at how AI can greatly expand your design possibilities. table of contents ChatGPT: A powerful tool for design creation


Hot AI Tools

Undresser.AI Undress
AI-powered app for creating realistic nude photos

AI Clothes Remover
Online AI tool for removing clothes from photos.

Undress AI Tool
Undress images for free

Clothoff.io
AI clothes remover

Video Face Swap
Swap faces in any video effortlessly with our completely free AI face swap tool!

Hot Article

Hot Tools
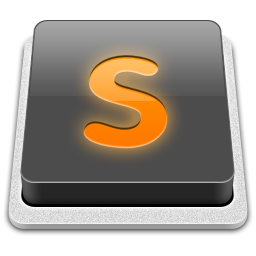
SublimeText3 Mac version
God-level code editing software (SublimeText3)
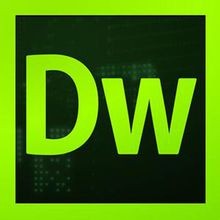
Dreamweaver CS6
Visual web development tools
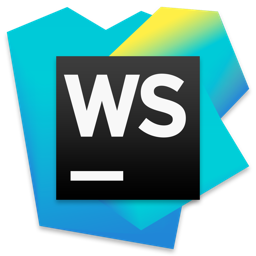
WebStorm Mac version
Useful JavaScript development tools

PhpStorm Mac version
The latest (2018.2.1) professional PHP integrated development tool

mPDF
mPDF is a PHP library that can generate PDF files from UTF-8 encoded HTML. The original author, Ian Back, wrote mPDF to output PDF files "on the fly" from his website and handle different languages. It is slower than original scripts like HTML2FPDF and produces larger files when using Unicode fonts, but supports CSS styles etc. and has a lot of enhancements. Supports almost all languages, including RTL (Arabic and Hebrew) and CJK (Chinese, Japanese and Korean). Supports nested block-level elements (such as P, DIV),
