


Why medical data quality is critical in the age of artificial intelligence
Effective medical data analysis requires taking into account the subjectivity of data quality. The quality of data will directly affect the accuracy, reliability and validity of the information obtained from the data. If data quality is poor, it can lead to incorrect diagnoses, ineffective treatments, and increased risks to patients and providers. Therefore, for healthcare managers looking to improve healthcare outcomes and performance through data analytics, it is critical to identify and address critical data quality issues
Data quality is key
Identification The first step in critical data quality issues is to determine what data quality means for the specific context and goals. Data quality can be assessed along dimensions such as accuracy, completeness, consistency, relevance, and completeness. Depending on the type and purpose of data analysis, some dimensions may be more important than others.
A growing number of healthcare innovations are enabling doctors to systematically provide better care to their patients. As doctors learn from the experiences of other doctors, we, as patients, realize that health care is complex and not always effective. Individual doctors learn from treating patients, but this information is rarely further used by other doctors to improve care.
However, if healthcare does not adopt routine care for learning, what data will doctors rely on to make important decisions?
The main approach to health care is to use clear methods. Randomized trials span several years, and the results are analyzed and gradually applied to clinical practice. While the safety and effectiveness of treatments can be determined, there is not enough information to compare different treatment options and find out which treatment works best
In short, while the data captured in such trials The information is good, but not enough. Healthcare doesn’t have enough data to tailor treatments or learn quickly.
Data Quality in Healthcare
Data quality in healthcare helps determine the cost of payment for medical services. With the increasing popularity of artificial intelligence (AI), data analytics, Internet of Medical Things (IoMT), and data visualization tools, the importance of data quality in healthcare cannot be underestimated.
In the healthcare industry, data quality refers to the following characteristics of data collected by healthcare organizations:
- Accuracy: Only when every detailed entry of the information is correct and correctly presented , the data is considered accurate.
- Integrity: Integrity means that all information collected by the provider is logged and easily accessible.
- Relevance: The relevance factor is satisfied when the data collected are used in a medical setting and for medical purposes.
- Legality: Demonstrate that data collection, processing, storage and use comply with all legal requirements and standards.
- Consistency: Data is considered consistent only if it is continually updated and reflects the patient's health status and medical interventions.
- Accessibility: Accessibility standards are met when medical personnel have full access to the details they need and can use to perform their duties.
The quality of data accumulated from various solutions can impact decision-making processes at both individual and global levels. If the data collected lacks any of the above attributes or is of poor quality, it means that the use of such erroneous data may have negative consequences for patients, hospitals and researchers
Healthcare Technology and Innovation
Healthcare as an industry is starting to learn from real-world nursing. While the infrastructure has always been in place, the recent convergence of data—technologies such as electronic health records, artificial intelligence, and computing power—has created an environment in which learning healthcare systems can be realized and anticipated.
Healthcare can turn knowledge learned from daily care into data. This knowledge can further help us better understand each person’s unique characteristics. It helps recognize how unique characteristics impact the effectiveness of available treatment options and provide tailored care to individuals
In healthcare, IT solutions are being adopted at an incredible rate. This has resulted in the emergence of many ever-changing trends and prompted continuous progress and improvements. However, these trends may have implications for data quality management
Learning the wrong lessons from bad data, however, is not only a problem, but a serious one that deserves attention. Industry makes decisions based on these recommendations. This could cause serious harm to patients, whose confidence in the validity of the evidence could be shaken.
The lesson here is clear: If healthcare is to learn from routine care, they must protect patients by ensuring data quality is high enough to explain recommendations.
New IT solutions that assist in the collection and processing of high-quality medical data have made significant advances in medical data management. Combining insights with responsibilities helps protect patients. In the process, they can define data quality standards and real-world evidence that are sufficient for their use. These standards can encourage key decision-makers, including doctors, insurers and regulators, to decide whether real-world evidence is trustworthy enough to influence standard procedures in health care. Improve healthcare providers’ predictive capabilities and avoid situations that could lead to poor patient outcomes. At the same time, this also helps improve hospital management and personnel management. The quality of data standards will further help measure accuracy, completeness and traceability
Summary
In the current learning healthcare system, few treatment decisions are based on reality Guided by the evidence of the world. Every treatment decision is influenced by past practice. Significant risks may exist if accuracy, completeness and traceability are not strictly emphasized. Not all companies that generate healthcare evidence use high-quality data or measure data quality. Relying on low-quality, evidence-based data can have disastrous consequences
But there is hope for a bright future in healthcare.
Health care organizations are embracing modern technology to learn from the most reliable medical data. However, in this case, data quality must be critical.
For the healthcare industry, the shift to a learning healthcare system has become more important than ever. The availability of electronic health data, computing power and artificial intelligence will bring about innovation. However, it is equally important for professionals in the healthcare industry to learn to differentiate between high-quality data and low-quality data and ensure they learn the right lessons from it
The above is the detailed content of Why medical data quality is critical in the age of artificial intelligence. For more information, please follow other related articles on the PHP Chinese website!
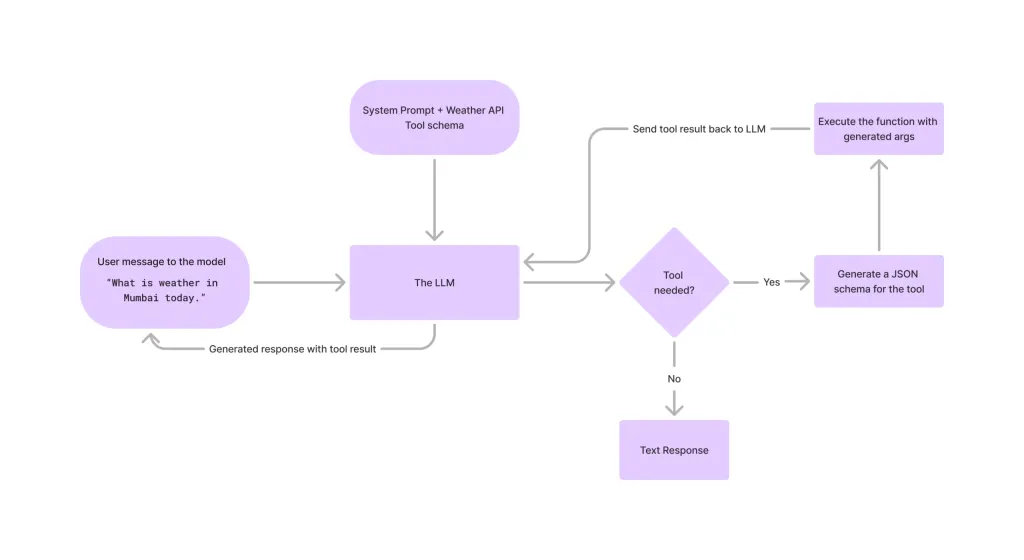
Large language models (LLMs) have surged in popularity, with the tool-calling feature dramatically expanding their capabilities beyond simple text generation. Now, LLMs can handle complex automation tasks such as dynamic UI creation and autonomous a
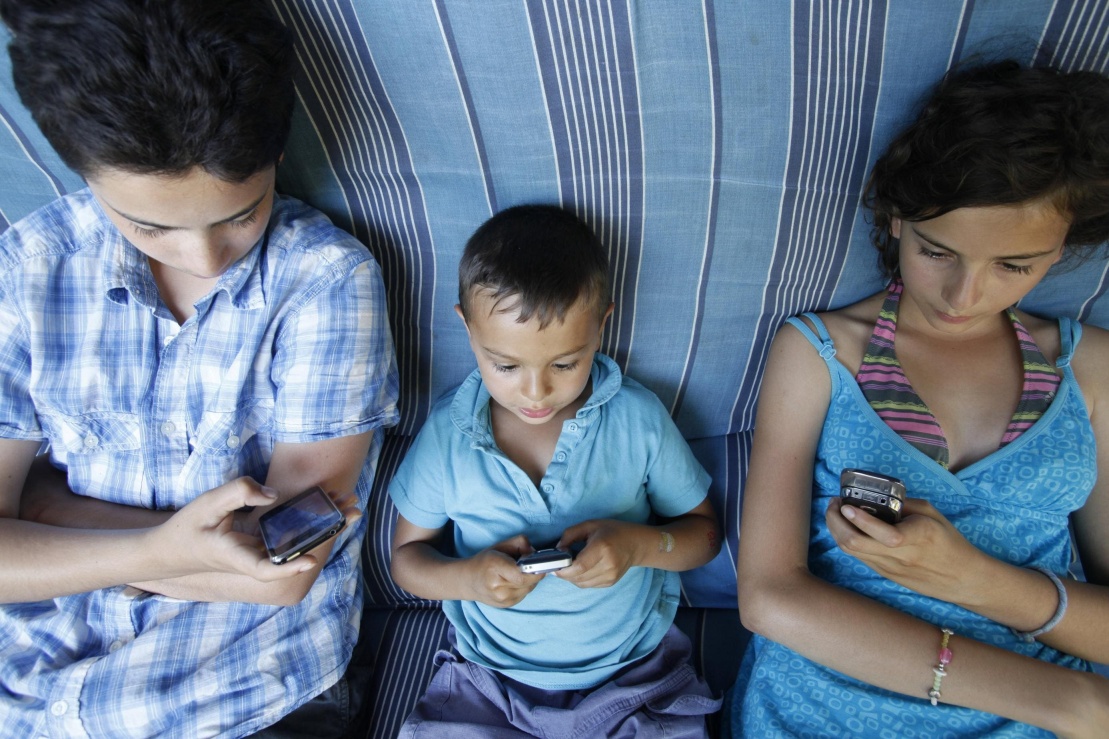
Can a video game ease anxiety, build focus, or support a child with ADHD? As healthcare challenges surge globally — especially among youth — innovators are turning to an unlikely tool: video games. Now one of the world’s largest entertainment indus
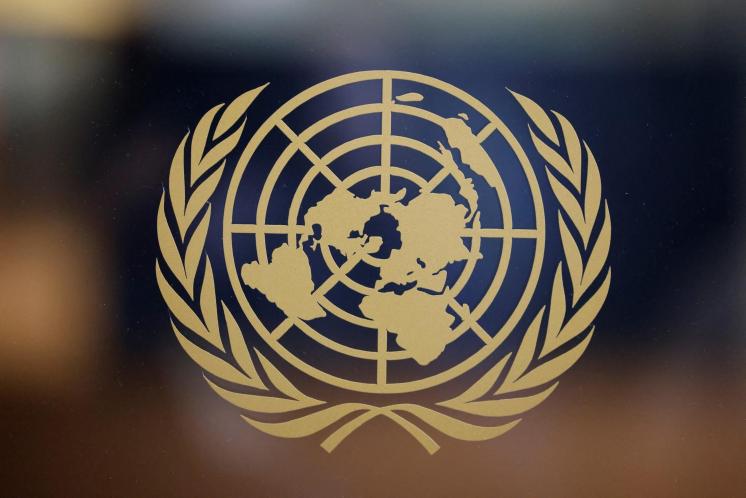
“History has shown that while technological progress drives economic growth, it does not on its own ensure equitable income distribution or promote inclusive human development,” writes Rebeca Grynspan, Secretary-General of UNCTAD, in the preamble.
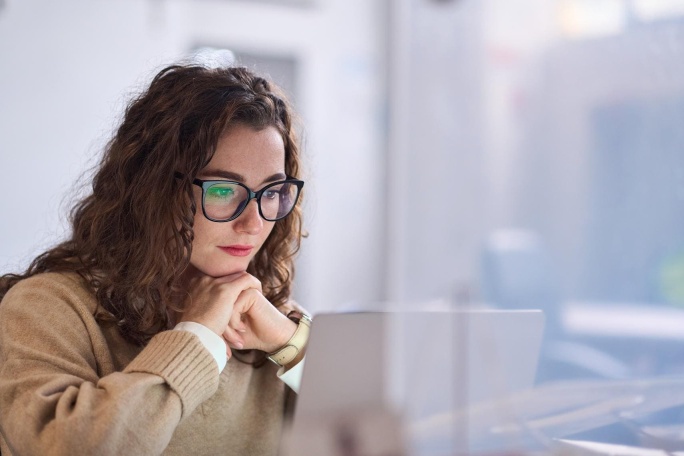
Easy-peasy, use generative AI as your negotiation tutor and sparring partner. Let’s talk about it. This analysis of an innovative AI breakthrough is part of my ongoing Forbes column coverage on the latest in AI, including identifying and explaining
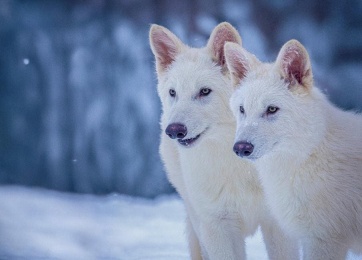
The TED2025 Conference, held in Vancouver, wrapped its 36th edition yesterday, April 11. It featured 80 speakers from more than 60 countries, including Sam Altman, Eric Schmidt, and Palmer Luckey. TED’s theme, “humanity reimagined,” was tailor made
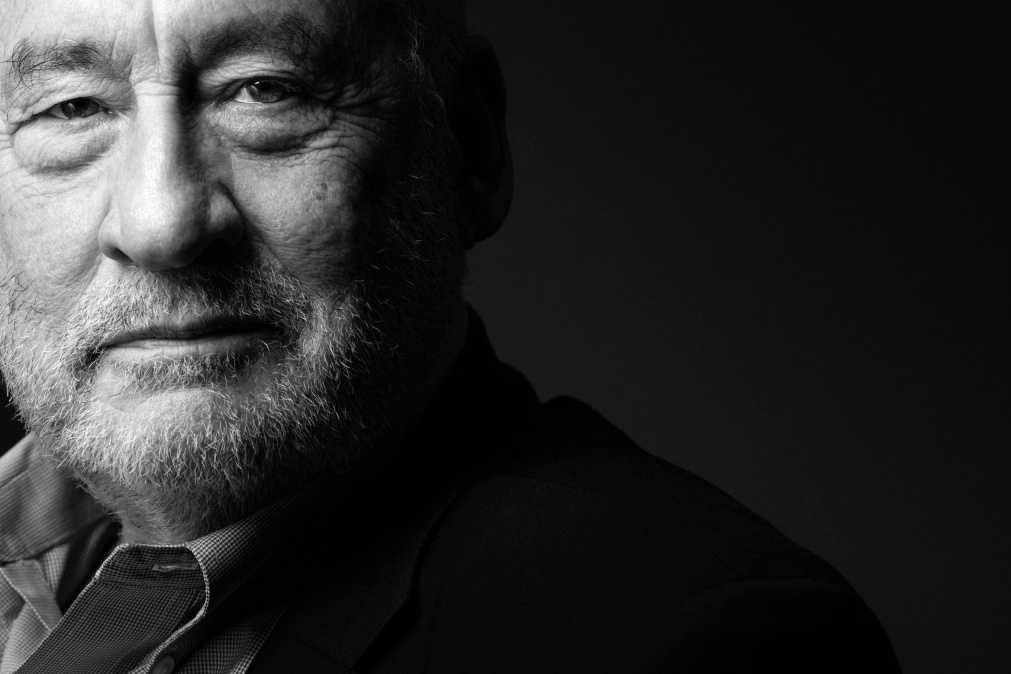
Joseph Stiglitz is renowned economist and recipient of the Nobel Prize in Economics in 2001. Stiglitz posits that AI can worsen existing inequalities and consolidated power in the hands of a few dominant corporations, ultimately undermining economic
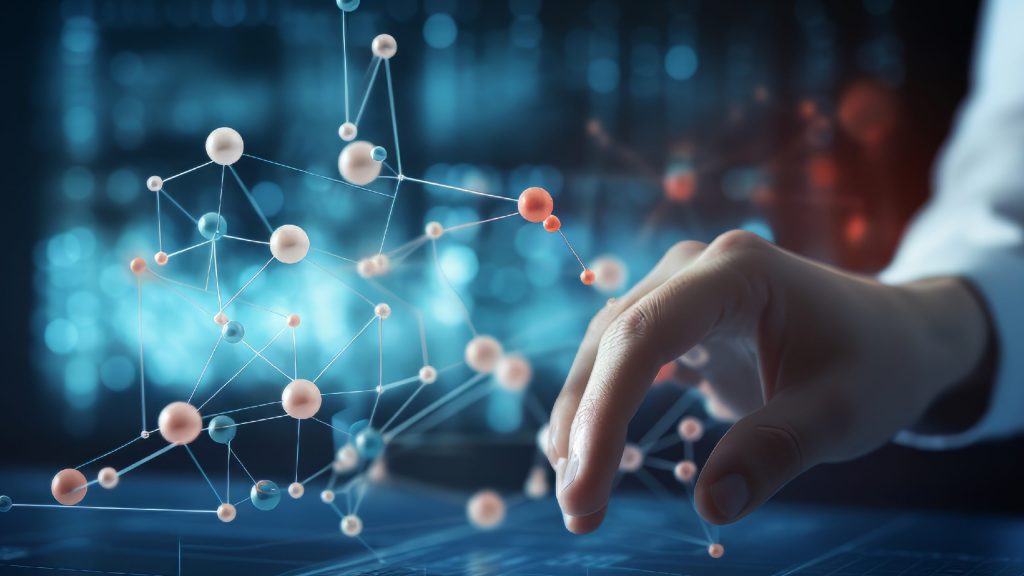
Graph Databases: Revolutionizing Data Management Through Relationships As data expands and its characteristics evolve across various fields, graph databases are emerging as transformative solutions for managing interconnected data. Unlike traditional
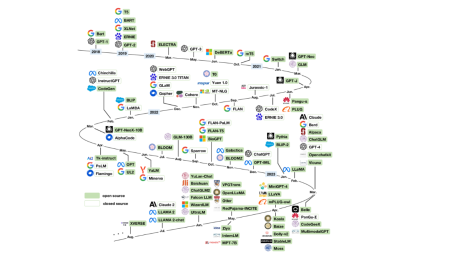
Large Language Model (LLM) Routing: Optimizing Performance Through Intelligent Task Distribution The rapidly evolving landscape of LLMs presents a diverse range of models, each with unique strengths and weaknesses. Some excel at creative content gen


Hot AI Tools

Undresser.AI Undress
AI-powered app for creating realistic nude photos

AI Clothes Remover
Online AI tool for removing clothes from photos.

Undress AI Tool
Undress images for free

Clothoff.io
AI clothes remover

AI Hentai Generator
Generate AI Hentai for free.

Hot Article

Hot Tools
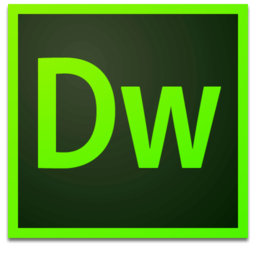
Dreamweaver Mac version
Visual web development tools

SublimeText3 English version
Recommended: Win version, supports code prompts!

Notepad++7.3.1
Easy-to-use and free code editor

Atom editor mac version download
The most popular open source editor

SAP NetWeaver Server Adapter for Eclipse
Integrate Eclipse with SAP NetWeaver application server.