How to implement Dijkstra's algorithm using Python?
How to implement Dijkstra's algorithm using Python?
Introduction:
Dijkstra's algorithm is a commonly used single-source shortest path algorithm that can be used to solve the shortest path problem between two vertices in a weighted graph. This article will introduce in detail how to use Python to implement Dijkstra's algorithm, including algorithm principles and specific code examples.
- Algorithm Principle
The core idea of Dijkstra's algorithm is to gradually determine the shortest path from the source point to other vertices by continuously selecting the vertex closest to the source point. The algorithm is mainly divided into the following steps:
(1) Initialization: Set the distance from the source point to other vertices to infinity, and the distance from the source point to itself to 0. At the same time, create a dictionary that records the shortest path and a collection that records the vertices that have been visited.
(2) Select the unvisited vertex currently closest to the source point, mark it as visited, and update the distance from the source point to its adjacent vertices.
(3) Repeat the above steps until all vertices have been visited or there are currently no selectable vertices. - Code implementation
The following is a code example using Python to implement Dijkstra's algorithm:
import sys def dijkstra(graph, start): # 初始化 distances = {vertex: sys.maxsize for vertex in graph} # 记录源点到各顶点的距离 distances[start] = 0 visited = set() previous_vertices = {vertex: None for vertex in graph} # 记录最短路径的前驱结点 while graph: # 选择当前距离源点最近的未访问顶点 current_vertex = min( {vertex: distances[vertex] for vertex in graph if vertex not in visited}, key=distances.get ) # 标记为已访问 visited.add(current_vertex) # 更新当前顶点的相邻顶点的距离 for neighbor in graph[current_vertex]: distance = distances[current_vertex] + graph[current_vertex][neighbor] if distance < distances[neighbor]: distances[neighbor] = distance previous_vertices[neighbor] = current_vertex # 当前顶点从图中移除 graph.pop(current_vertex) return distances, previous_vertices # 示例使用 if __name__ == '__main__': # 定义图结构(字典表示) graph = { 'A': {'B': 5, 'C': 1}, 'B': {'A': 5, 'C': 2, 'D': 1}, 'C': {'A': 1, 'B': 2, 'D': 4, 'E': 8}, 'D': {'B': 1, 'C': 4, 'E': 3, 'F': 6}, 'E': {'C': 8, 'D': 3}, 'F': {'D': 6} } start_vertex = 'A' distances, previous_vertices = dijkstra(graph, start_vertex) # 打印结果 for vertex in distances: path = [] current_vertex = vertex while current_vertex is not None: path.insert(0, current_vertex) current_vertex = previous_vertices[current_vertex] print(f'最短路径: {path}, 最短距离: {distances[vertex]}')
The above code example shows how to use Dijkstra's algorithm to solve a given graph structure from the source point The shortest path and shortest distance to each vertex.
Conclusion:
This article introduces the principle of Dijkstra algorithm in detail and gives a code example of using Python to implement Dijkstra algorithm. Readers can modify and expand the sample code to apply to more complex scenarios. By mastering this algorithm, readers can better solve the problem of shortest paths in weighted graphs.
The above is the detailed content of How to implement Dijkstra's algorithm using Python?. For more information, please follow other related articles on the PHP Chinese website!
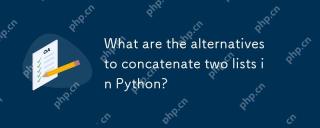
There are many methods to connect two lists in Python: 1. Use operators, which are simple but inefficient in large lists; 2. Use extend method, which is efficient but will modify the original list; 3. Use the = operator, which is both efficient and readable; 4. Use itertools.chain function, which is memory efficient but requires additional import; 5. Use list parsing, which is elegant but may be too complex. The selection method should be based on the code context and requirements.
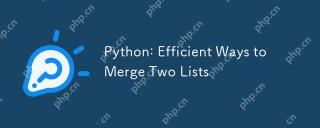
There are many ways to merge Python lists: 1. Use operators, which are simple but not memory efficient for large lists; 2. Use extend method, which is efficient but will modify the original list; 3. Use itertools.chain, which is suitable for large data sets; 4. Use * operator, merge small to medium-sized lists in one line of code; 5. Use numpy.concatenate, which is suitable for large data sets and scenarios with high performance requirements; 6. Use append method, which is suitable for small lists but is inefficient. When selecting a method, you need to consider the list size and application scenarios.
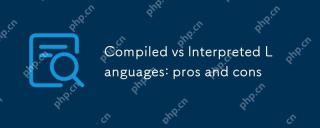
Compiledlanguagesofferspeedandsecurity,whileinterpretedlanguagesprovideeaseofuseandportability.1)CompiledlanguageslikeC arefasterandsecurebuthavelongerdevelopmentcyclesandplatformdependency.2)InterpretedlanguageslikePythonareeasiertouseandmoreportab
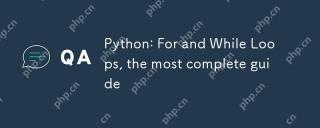
In Python, a for loop is used to traverse iterable objects, and a while loop is used to perform operations repeatedly when the condition is satisfied. 1) For loop example: traverse the list and print the elements. 2) While loop example: guess the number game until you guess it right. Mastering cycle principles and optimization techniques can improve code efficiency and reliability.
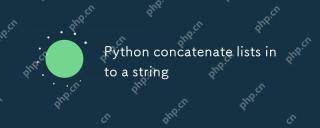
To concatenate a list into a string, using the join() method in Python is the best choice. 1) Use the join() method to concatenate the list elements into a string, such as ''.join(my_list). 2) For a list containing numbers, convert map(str, numbers) into a string before concatenating. 3) You can use generator expressions for complex formatting, such as ','.join(f'({fruit})'forfruitinfruits). 4) When processing mixed data types, use map(str, mixed_list) to ensure that all elements can be converted into strings. 5) For large lists, use ''.join(large_li
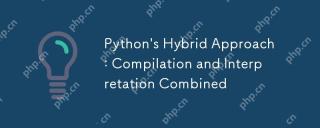
Pythonusesahybridapproach,combiningcompilationtobytecodeandinterpretation.1)Codeiscompiledtoplatform-independentbytecode.2)BytecodeisinterpretedbythePythonVirtualMachine,enhancingefficiencyandportability.
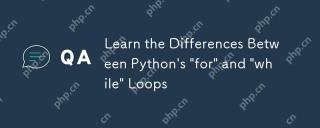
ThekeydifferencesbetweenPython's"for"and"while"loopsare:1)"For"loopsareidealforiteratingoversequencesorknowniterations,while2)"while"loopsarebetterforcontinuinguntilaconditionismetwithoutpredefinediterations.Un
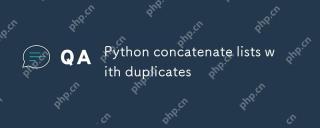
In Python, you can connect lists and manage duplicate elements through a variety of methods: 1) Use operators or extend() to retain all duplicate elements; 2) Convert to sets and then return to lists to remove all duplicate elements, but the original order will be lost; 3) Use loops or list comprehensions to combine sets to remove duplicate elements and maintain the original order.


Hot AI Tools

Undresser.AI Undress
AI-powered app for creating realistic nude photos

AI Clothes Remover
Online AI tool for removing clothes from photos.

Undress AI Tool
Undress images for free

Clothoff.io
AI clothes remover

Video Face Swap
Swap faces in any video effortlessly with our completely free AI face swap tool!

Hot Article

Hot Tools

Safe Exam Browser
Safe Exam Browser is a secure browser environment for taking online exams securely. This software turns any computer into a secure workstation. It controls access to any utility and prevents students from using unauthorized resources.
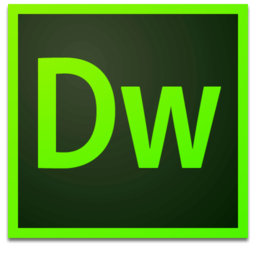
Dreamweaver Mac version
Visual web development tools

SecLists
SecLists is the ultimate security tester's companion. It is a collection of various types of lists that are frequently used during security assessments, all in one place. SecLists helps make security testing more efficient and productive by conveniently providing all the lists a security tester might need. List types include usernames, passwords, URLs, fuzzing payloads, sensitive data patterns, web shells, and more. The tester can simply pull this repository onto a new test machine and he will have access to every type of list he needs.
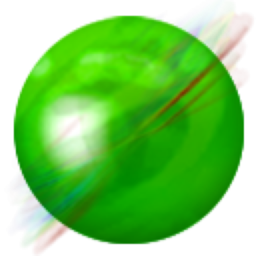
ZendStudio 13.5.1 Mac
Powerful PHP integrated development environment
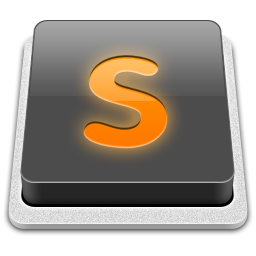
SublimeText3 Mac version
God-level code editing software (SublimeText3)
