Large language models (LLMs) including decoder-only structures (such as GPT and LLAMA series models), encoder-only structures (such as BERT), and encoder-decoder structures (such as T5) and their variant models ) has achieved remarkable success and has been widely used in various language processing and multi-modal tasks. Despite this success, training LLM is so expensive that only a few companies can afford it. In addition, current trends indicate that larger training data will be used in the future, which will further increase the development cost of large models. For example, LLAMA-1 training uses 1-1.4 TB tokens, while Llama 2 reaches 2 TB. Another key challenge in developing an LLM is evaluation. Mainstream evaluation methods are divided into two categories: knowledge evaluation (MMLU and C-Eval) and NLP task evaluation. These evaluation methods may not truly reflect the capabilities of the model because there may be data leakage issues, i.e. some parts of the evaluation data set may have been used during the model training process. Furthermore, knowledge-oriented assessment methods may not be adequate for assessing intelligence levels. A more fair and objective assessment method is to measure the intelligence quotient (IQ) of the LLM, which is to generalize the LLM to conditions and contexts not seen in the training data. Growth strategy. In order to solve the training cost problem, many institutions such as Beijing Zhiyuan Artificial Intelligence Research Institute and the Institute of Computing Technology of the Chinese Academy of Sciences have recently made some attempts - training a 100 billion parameter level LLM through a growth strategy for the first time. Growth means that the number of parameters during training is not fixed, but expands from smaller models to larger models. 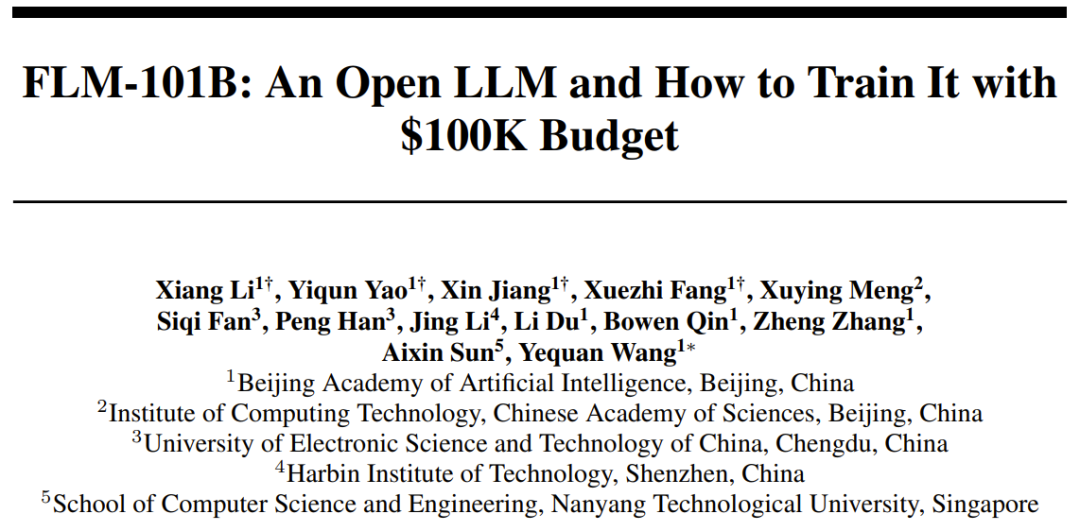
Figure 1 shows three typical scenarios of growth strategies. Since the FLOPs of an LLM are roughly proportional to the number of its parameters, the area between the change curve of the model parameters and the X-axis can represent the computational cost of training. 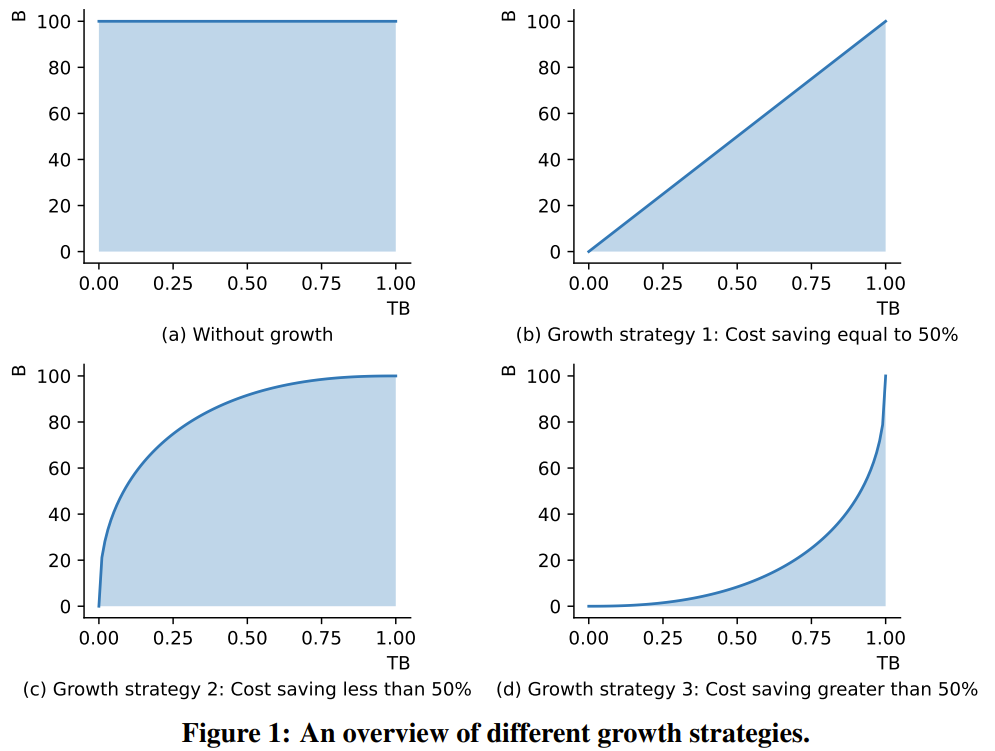
Figure 1 (a) shows the standard training strategy without model growth; 1 (b) is a linear growth strategy, which can save 50% of the cost; 1 (c) It is a moderate growth strategy, which can save less than 50% of the cost; 1 (d) is a radical growth strategy, which can save more than 50% of the cost. This analysis illustrates that in order to save as much computing cost as possible, an aggressive growth strategy should be adopted. The design of the growth operator of this new study is inspired by the MSG in the paper "2x faster language model pre-training via masked structural growth", which is a complete A set of operations covering all four growth dimensions of the Transformer structure. More importantly, MSG can grow while tightly preserving functionality. Therefore, although a small model can learn quickly with a smaller parameter search space, its knowledge can be inherited by subsequent larger models. This makes it possible for growth strategies to achieve better performance using the same or less computational cost. Open source FLM-101B model. Researchers at Zhiyuan Research Institute trained an LLM model with 101 billion parameters through gradual growth, and they also stated that they would release the model as open source. The architecture of this model is an evolution of FreeLM. Therefore, the researchers named it FLM-101B, where F stands for Free. #The FreeLM framework has two pre-training objectives, which are guided by language signals and teacher signals respectively. In this new research, these two goals are unified into a common language modeling paradigm. IQ Assessment Benchmark. In addition to the low-cost training paradigm, the team also made another contribution by proposing a systematic set of benchmarks for LLM's intelligence quotient (IQ) assessment. Previous research has shown that although the perplexity level (PPL) indicator can reflect the quality of generated text to a certain extent, it is not reliable. On the other hand, the scale of LLM training data is so large that it is difficult for us to distinguish whether the model is just quoting knowledge data, or whether it is really achieving human-like reasoning, analysis, and generalization capabilities, which are what this study defines IQ Foundation. Some commonly used evaluation metrics (MMLU for English and C-Eval for Chinese) are obviously knowledge-oriented and cannot fully reflect the intelligence level of the model. For a sanity check, the team conducted a test: five computer science researchers from world-renowned universities took an exam using C-Eval’s chemistry test questions . It turned out that their accuracy was almost as good as random guessing because most of the volunteers had forgotten what they had learned about chemistry. Therefore, evaluation benchmarks that emphasize knowledge of expertise are not adequate measures of a model's IQ. To comprehensively measure LLM's IQ, the team developed an IQ assessment benchmark that takes into account four key aspects of IQ: symbol mapping, rule understanding, pattern mining, and Anti-interference.
Language is symbolic in nature. There have been some studies using symbols rather than category labels to assess the intelligence level of LLMs. Similarly, the team used a symbolic mapping approach to test the LLM's ability to generalize to unseen contexts.
An important ability of human intelligence is to understand given rules and take corresponding actions. This testing method has been widely used in various levels of testing. Therefore, rule understanding becomes the second test here.
Rewritten content: Pattern mining is an important part of intelligence, which involves induction and deduction. In the history of scientific development, this method plays a crucial role. In addition, test questions in various competitions often require this ability to answer. For these reasons, we chose pattern mining as the third evaluation indicator
The last and very important indicator is the anti-interference ability, which is also one of the core capabilities of intelligence. Studies have pointed out that both language and images are easily disturbed by noise. With this in mind, the team used interference immunity as a final evaluation metric.
Of course, these four indicators are by no means the final word in LLM IQ assessment, but they can serve as a starting point to stimulate subsequent research and development, and are expected to eventually lead to a comprehensive set of LLM IQ assessment framework. The main contributions of this study include:
The researchers stated that this is a study using growth strategies to train more than 1,000 people from scratch. LLM research attempt on billion parameters. At the same time, this is also the lowest cost 100 billion parameter model currently, costing only 100,000 US dollars
By improving FreeLM training objectives, potential hyperparameter search methods and function-preserving growth, This study addresses the issue of instability. The researchers believe this method can also help the broader scientific research community.
The researchers also conducted experimental comparisons of the new model with previously powerful models, including using knowledge-oriented benchmarks and a newly proposed systematic IQ assessment benchmark. Experimental results show that the FLM-101B model is competitive and robust
The team will release model checkpoints, code, related tools, etc. to promote the research and development of bilingual LLM in Chinese and English with a scale of 100 billion parameters.
Architecturally, FLM-101B uses FreeLM as its backbone network, and integrates xPos. In terms of model size, thanks to the new growth strategy, researchers can obtain models of three sizes: 16B, 51B, and 101B in one training. As for the pre-training settings, FLM-101B inherits the training strategy of FreeLM. In terms of growth strategy, instead of the common practice of training models of different sizes independently, the team can sequentially train three models with 16B, 51B, and 101B parameters. Each of these models inherits the knowledge of the smaller model before it. As for the training hardware, a cluster of 24 DGX-A800 GPU (8×80G) servers is used; the training time of FLM-101B is less than 26 days. See Tables 1 and 2 below for multi-parallel strategy and model configurations. 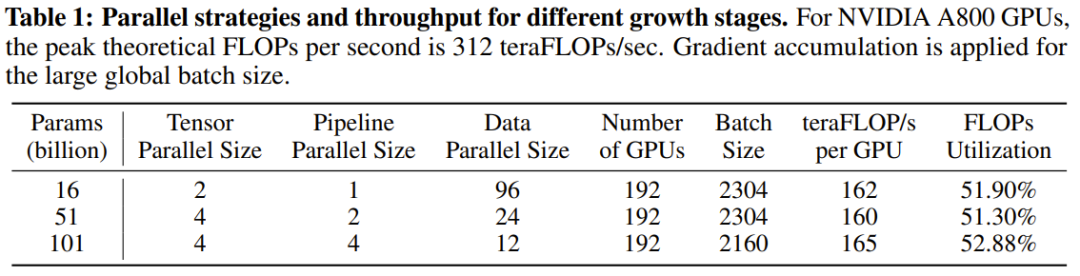
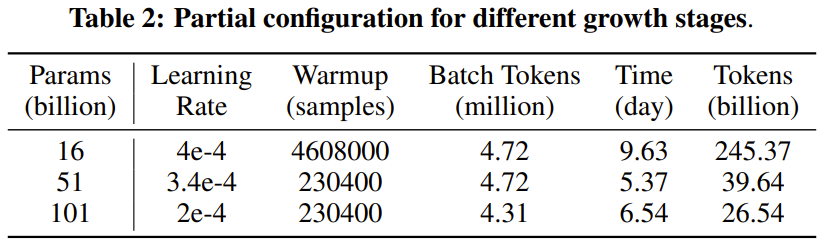
Training Stability of FLM-101BIn order to solve unstable problems such as loss divergence and gradient explosion, researchers have proposed a promising solution, which is briefly described as follows. Loss prediction. The newly proposed method to achieve training stability is as follows: First, determine the distribution of the data before starting FLM-16B training. Next, perform a grid search on three hyperparameters, including the learning rate, initialization standard deviation, and softmax temperature of the output layer. The grid search is performed by running a surrogate model with a hidden state dimension (i.e., model width) of 256, a head count of 2, and a parameter count of 40 million. All other structural hyperparameters and training data of this surrogate model are the same as FLM-16B. Using data parallelism on 6 nodes, a grid search run took 24.6 hours, which roughly translates into 6 hours using a 24-node configuration. Through this grid search, the researchers found the optimal hyperparameters: learning rate = 4e-4, standard deviation = 1.6e-2, softmax temperature = 2.0. Then they migrate these hyperparameters through µP to achieve a seamless training experience that avoids instability problems. When MSG is used in combination, LM-51B and FLM-101B do not have subsequent growth divergence problems. Figure 2 shows the complete training loss curve. 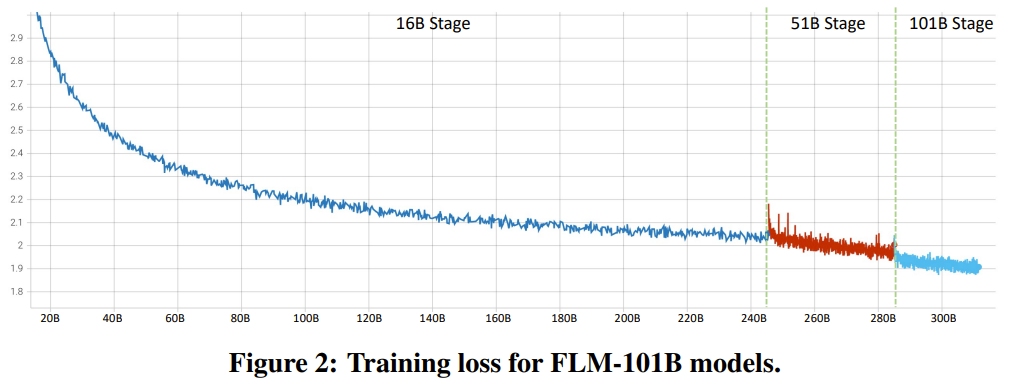
Mixed precision via Bfloat16. The purpose of using mixed precision is to save memory and time costs during runtime. Here they chose Bfloat16. Table 3 compares FLM-101B with others Performance of powerful baseline models (LLAMA series models and GLM-130B). 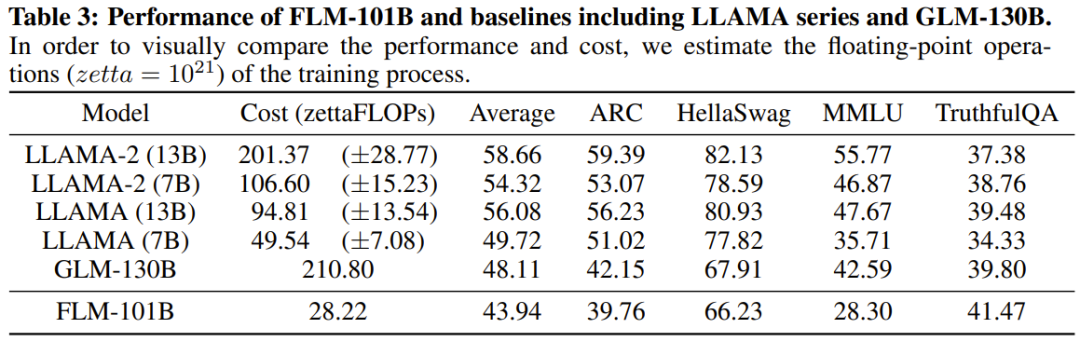
The researchers stated that these results indicate that FLM-101B does not have any advantage in factual knowledge, and its performance will continue if more training data can be used. promote. Table 4 shows the results of eFLM-16B versus the baseline model in terms of expertise assessment. 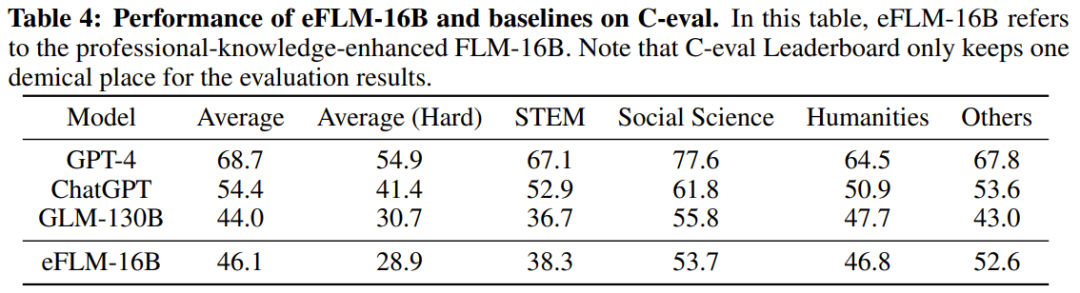
It turns out that scores on datasets that emphasize expertise do not reflect the level of intelligence of LLM, as some specific training data may have an overwhelming contribution. Table 5 shows the performance of each stage of the FLM model. 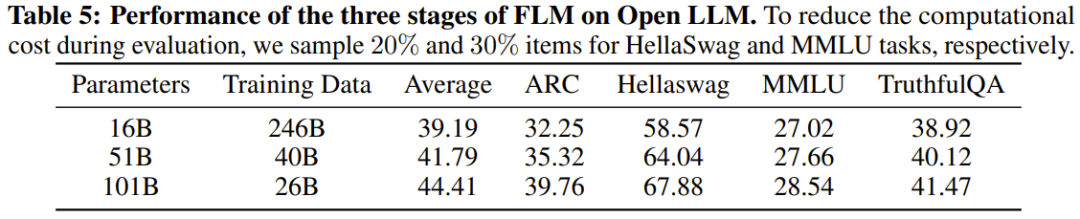
As expected, the performance of FLM will improve as the model increases. The FLM-101B performed best on almost every mission. This means that each time the model grows, it inherits the knowledge from the previous stage. In the experiment, in order to test the IQ of LLM For a more systematic evaluation, the team from the Intellectual Property Research Institute used existing IQ-related data sets and made some necessary modifications. They also generated some new synthetic data. Specifically, the IQ assessment they proposed mainly considers four aspects: symbol mapping, rule understanding, pattern mining, and anti-interference. These tasks have one key thing in common: they all rely on reasoning and generalization in new contexts. The following tables show the results of the IQ experiment: 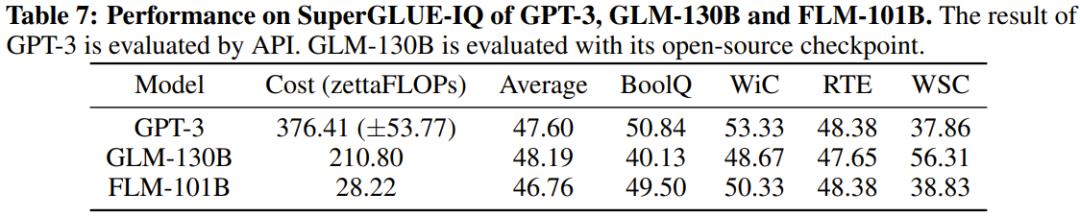


##From these tables, on these four IQ evaluation benchmarks, FLM-101B achieves results comparable to GPT-3 and better than GLM-130B at a much lower computational cost.
In addition to the impact of training data, the researchers speculate that this advantage may be due to the small model in the early stages refining the smaller search space, and when the model becomes more This advantage continues to play out when larger and wider, and generalization capabilities are enhanced.
The above is the detailed content of With 100,000 US dollars + 26 days, a low-cost LLM with 100 billion parameters was born. For more information, please follow other related articles on the PHP Chinese website!