How to implement data filtering function in MongoDB
MongoDB is one of the most popular NoSQL databases in the world, which is widely known for its high scalability and flexibility. Popular with developers. When using MongoDB, we often need to retrieve data from the database based on specific conditions. To this end, MongoDB provides rich query and filtering functions. This article will introduce how to implement data filtering in MongoDB and provide some specific code examples.
1. Basic filtering operation example
First, we need to establish a client connected to the MongoDB database. In Python, we can use PyMongo to achieve this functionality. The following is a simple sample code:
from pymongo import MongoClient client = MongoClient("mongodb://localhost:27017/") db = client["mydatabase"] collection = db["mycollection"]
Next, we can use the find()
method to retrieve data from the collection and filter the data by specifying query conditions. The following is a sample code that demonstrates how to retrieve users whose age is greater than 25:
users = collection.find({"age": {"$gt": 25}}) for user in users: print(user)
In the above example, we use the find()
method to get all documents that satisfy the specified query criteria. The query condition uses MongoDB’s query operator $gt
, which means greater than. Running the above code will output information for all users whose age is greater than 25.
2. Examples of advanced filtering operations
MongoDB provides a rich set of query operators, allowing us to implement more complex data filtering requirements. The following are some commonly used query operators and their sample codes:
-
$eq
: equalsusers = collection.find({"age": {"$eq": 30}})
-
$ne
: Not equal tousers = collection.find({"age": {"$ne": 30}})
-
$gt
: Greater thanusers = collection.find({"age": {"$gt": 25}})
-
$gte
: Greater than or equal tousers = collection.find({"age": {"$gte": 25}})
-
$lt
: Less thanusers = collection.find({"age": {"$lt": 30}})
-
$lte
: Less than or equal tousers = collection.find({"age": {"$lte": 30}})
-
$in
: Matches any one condition in the given listusers = collection.find({"age": {"$in": [25, 30, 35]}})
-
$ nin
: Does not meet any condition in the given listusers = collection.find({"age": {"$nin": [25, 30, 35]}})
The above example shows how to use common query operators. We can perform flexible data filtering according to actual needs. .
3. Examples of complex filtering operations
In addition to basic query operators, MongoDB also provides support for logical operators and regular expressions, allowing us to implement more complex data filtering and queries. . Here is some sample code:
-
$and
: multiple conditions are met simultaneouslyusers = collection.find({"$and": [{"age": {"$gt": 25}}, {"age": {"$lt": 30}}]})
-
$or
: Any one of multiple conditions is metusers = collection.find({"$or": [{"age": {"$lt": 25}}, {"age": {"$gt": 30}}]})
-
$not
: The specified condition is not metusers = collection.find({"age": {"$not": {"$eq": 30}}})
-
Regular expression matching
users = collection.find({"name": {"$regex": "^J"}})
By combining these operators, we can achieve more complex data filtering and query functions.
Summary:
This article introduces how to implement the data filtering function in MongoDB, and provides some specific code examples, including basic filtering operations and advanced filtering operations. Through these examples, we can use MongoDB more flexibly for data filtering and querying to meet actual needs. I hope this article will be helpful to you in using MongoDB.
The above is the detailed content of How to implement data filtering function in MongoDB. For more information, please follow other related articles on the PHP Chinese website!
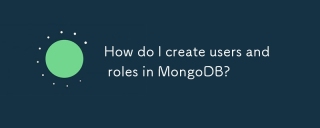
The article discusses creating users and roles in MongoDB, managing permissions, ensuring security, and automating these processes. It emphasizes best practices like least privilege and role-based access control.
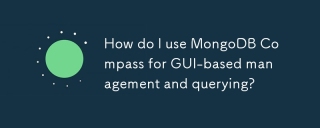
MongoDB Compass is a GUI tool for managing and querying MongoDB databases. It offers features for data exploration, complex query execution, and data visualization.

The article discusses selecting a shard key in MongoDB, emphasizing its impact on performance and scalability. Key considerations include high cardinality, query patterns, and avoiding monotonic growth.
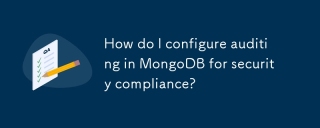
The article discusses configuring MongoDB auditing for security compliance, detailing steps to enable auditing, set up audit filters, and ensure logs meet regulatory standards. Main issue: proper configuration and analysis of audit logs for security
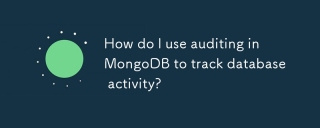
This article details how to implement auditing in MongoDB using change streams, aggregation pipelines, and various storage options (other MongoDB collections, external databases, message queues). It emphasizes performance optimization (filtering, as
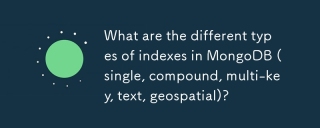
The article discusses various MongoDB index types (single, compound, multi-key, text, geospatial) and their impact on query performance. It also covers considerations for choosing the right index based on data structure and query needs.
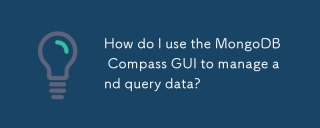
This article explains how to use MongoDB Compass, a GUI for managing and querying MongoDB databases. It covers connecting, navigating databases, querying with a visual builder, data manipulation, and import/export. While efficient for smaller datas
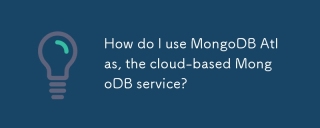
This article guides users through MongoDB Atlas, a cloud-based NoSQL database. It covers setup, cluster management, data handling, scaling, security, and optimization strategies, highlighting key differences from self-hosted MongoDB and emphasizing


Hot AI Tools

Undresser.AI Undress
AI-powered app for creating realistic nude photos

AI Clothes Remover
Online AI tool for removing clothes from photos.

Undress AI Tool
Undress images for free

Clothoff.io
AI clothes remover

AI Hentai Generator
Generate AI Hentai for free.

Hot Article

Hot Tools

SublimeText3 Linux new version
SublimeText3 Linux latest version
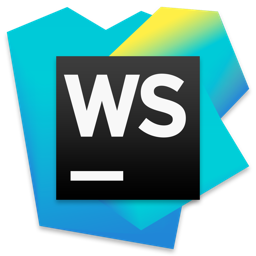
WebStorm Mac version
Useful JavaScript development tools
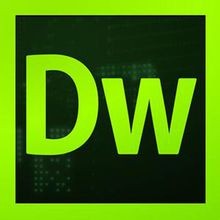
Dreamweaver CS6
Visual web development tools

SAP NetWeaver Server Adapter for Eclipse
Integrate Eclipse with SAP NetWeaver application server.

SublimeText3 Chinese version
Chinese version, very easy to use
