How to develop a simple machine learning system using MongoDB
How to use MongoDB to develop a simple machine learning system
With the development of artificial intelligence and machine learning, more and more developers are beginning to use MongoDB as their database selection. MongoDB is a popular NoSQL document database that provides powerful data management and query capabilities and is ideal for storing and processing machine learning data sets. This article will introduce how to use MongoDB to develop a simple machine learning system and give specific code examples.
- Install and configure MongoDB
First, we need to install and configure MongoDB. You can download the latest version from the official website (https://www.mongodb.com/) and follow the instructions to install it. After the installation is complete, you need to start the MongoDB service and create a database.
The method of starting the MongoDB service varies depending on the operating system. In most Linux systems, you can start the service with the following command:
sudo service mongodb start
In Windows systems, you can enter the following command in the command line:
mongod
To create a database, you can use MongoDB The command line tool mongo. Enter the following command at the command line:
mongo use mydb
- Import and process the data set
To develop a machine learning system, you first need to have a data set. MongoDB can store and process many types of data, including structured and unstructured data. Here, we take a simple iris dataset as an example.
We first save the iris data set as a csv file, and then use MongoDB's import tool mongodump to import the data. Enter the following command at the command line:
mongoimport --db mydb --collection flowers --type csv --headerline --file iris.csv
This will create a collection named flowers and import the iris dataset into it.
Now, we can use MongoDB’s query language to process the dataset. The following are some commonly used query operations:
- Query all data:
db.flowers.find()
- Query the value of a specific attribute:
db.flowers.find({ species: "setosa" })
- Query a certain range of attribute values:
db.flowers.find({ sepal_length: { $gt: 5.0, $lt: 6.0 } })
- Build a machine learning model
MongoDB provides many tools and APIs for operating data. We can use these tools and APIs to build our machine learning models. Here we will develop our machine learning system using the Python programming language and pymongo, the Python driver for MongoDB.
We first need to install pymongo. You can use the pip command to install:
pip install pymongo
Then, we can write Python code to connect to MongoDB and perform related operations. The following is a simple code example:
from pymongo import MongoClient # 连接MongoDB数据库 client = MongoClient() db = client.mydb # 查询数据集 flowers = db.flowers.find() # 打印结果 for flower in flowers: print(flower)
This code will connect to the database named mydb and query the data set as flowers. Then, print the query results.
- Data preprocessing and feature extraction
In machine learning, it is usually necessary to preprocess data and extract features. MongoDB can provide us with some functions to assist in these operations.
For example, we can use MongoDB's aggregation operation to calculate the statistical characteristics of the data. The following is a sample code:
from pymongo import MongoClient # 连接MongoDB数据库 client = MongoClient() db = client.mydb # 计算数据集的平均值 average_sepal_length = db.flowers.aggregate([ { "$group": { "_id": None, "avg_sepal_length": { "$avg": "$sepal_length" } }} ]) # 打印平均值 for result in average_sepal_length: print(result["avg_sepal_length"])
This code will calculate the average of the sepal_length attribute in the data set and print the result.
- Training and evaluating machine learning models
Finally, we can use MongoDB to save and load machine learning models for training and evaluation.
The following is a sample code:
from pymongo import MongoClient from sklearn.linear_model import LogisticRegression import pickle # 连接MongoDB数据库 client = MongoClient() db = client.mydb # 查询数据集 flowers = db.flowers.find() # 准备数据集 X = [] y = [] for flower in flowers: X.append([flower["sepal_length"], flower["sepal_width"], flower["petal_length"], flower["petal_width"]]) y.append(flower["species"]) # 训练模型 model = LogisticRegression() model.fit(X, y) # 保存模型 pickle.dump(model, open("model.pkl", "wb")) # 加载模型 loaded_model = pickle.load(open("model.pkl", "rb")) # 评估模型 accuracy = loaded_model.score(X, y) print(accuracy)
This code will load the data set from MongoDB and prepare training data. Then, use the logistic regression model to train and save the model locally. Finally, the model is loaded and evaluated using the dataset.
Summary:
This article introduces how to use MongoDB to develop a simple machine learning system and gives specific code examples. By combining the power of MongoDB with machine learning technology, we can develop more powerful and intelligent systems more efficiently. Hope this article helps you!
The above is the detailed content of How to develop a simple machine learning system using MongoDB. For more information, please follow other related articles on the PHP Chinese website!
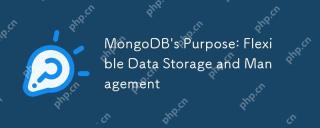
MongoDB's flexibility is reflected in: 1) able to store data in any structure, 2) use BSON format, and 3) support complex query and aggregation operations. This flexibility makes it perform well when dealing with variable data structures and is a powerful tool for modern application development.
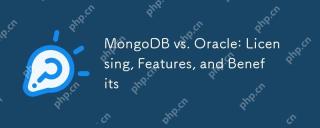
MongoDB is suitable for processing large-scale unstructured data and adopts an open source license; Oracle is suitable for complex commercial transactions and adopts a commercial license. 1.MongoDB provides flexible document models and scalability across the board, suitable for big data processing. 2. Oracle provides powerful ACID transaction support and enterprise-level capabilities, suitable for complex analytical workloads. Data type, budget and technical resources need to be considered when choosing.
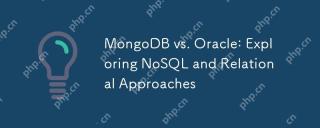
In different application scenarios, choosing MongoDB or Oracle depends on specific needs: 1) If you need to process a large amount of unstructured data and do not have high requirements for data consistency, choose MongoDB; 2) If you need strict data consistency and complex queries, choose Oracle.
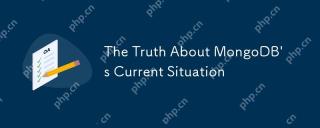
MongoDB's current performance depends on the specific usage scenario and requirements. 1) In e-commerce platforms, MongoDB is suitable for storing product information and user data, but may face consistency problems when processing orders. 2) In the content management system, MongoDB is convenient for storing articles and comments, but it requires sharding technology when processing large amounts of data.
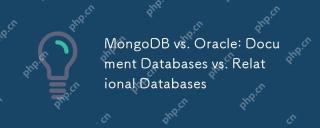
Introduction In the modern world of data management, choosing the right database system is crucial for any project. We often face a choice: should we choose a document-based database like MongoDB, or a relational database like Oracle? Today I will take you into the depth of the differences between MongoDB and Oracle, help you understand their pros and cons, and share my experience using them in real projects. This article will take you to start with basic knowledge and gradually deepen the core features, usage scenarios and performance performance of these two types of databases. Whether you are a new data manager or an experienced database administrator, after reading this article, you will be on how to choose and use MongoDB or Ora in your project
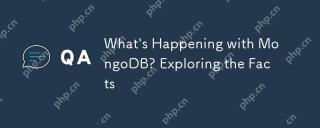
MongoDB is still a powerful database solution. 1) It is known for its flexibility and scalability and is suitable for storing complex data structures. 2) Through reasonable indexing and query optimization, its performance can be improved. 3) Using aggregation framework and sharding technology, MongoDB applications can be further optimized and extended.
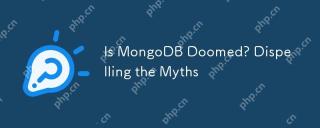
MongoDB is not destined to decline. 1) Its advantage lies in its flexibility and scalability, which is suitable for processing complex data structures and large-scale data. 2) Disadvantages include high memory usage and late introduction of ACID transaction support. 3) Despite doubts about performance and transaction support, MongoDB is still a powerful database solution driven by technological improvements and market demand.
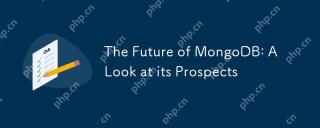
MongoDB'sfutureispromisingwithgrowthincloudintegration,real-timedataprocessing,andAI/MLapplications,thoughitfaceschallengesincompetition,performance,security,andeaseofuse.1)CloudintegrationviaMongoDBAtlaswillseeenhancementslikeserverlessinstancesandm


Hot AI Tools

Undresser.AI Undress
AI-powered app for creating realistic nude photos

AI Clothes Remover
Online AI tool for removing clothes from photos.

Undress AI Tool
Undress images for free

Clothoff.io
AI clothes remover

Video Face Swap
Swap faces in any video effortlessly with our completely free AI face swap tool!

Hot Article

Hot Tools

Safe Exam Browser
Safe Exam Browser is a secure browser environment for taking online exams securely. This software turns any computer into a secure workstation. It controls access to any utility and prevents students from using unauthorized resources.

SublimeText3 Linux new version
SublimeText3 Linux latest version

DVWA
Damn Vulnerable Web App (DVWA) is a PHP/MySQL web application that is very vulnerable. Its main goals are to be an aid for security professionals to test their skills and tools in a legal environment, to help web developers better understand the process of securing web applications, and to help teachers/students teach/learn in a classroom environment Web application security. The goal of DVWA is to practice some of the most common web vulnerabilities through a simple and straightforward interface, with varying degrees of difficulty. Please note that this software

SublimeText3 English version
Recommended: Win version, supports code prompts!
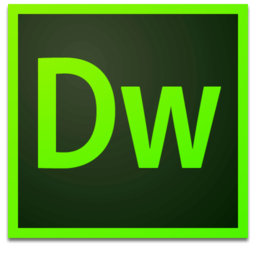
Dreamweaver Mac version
Visual web development tools
