Breadth First, Look (BFS) is a graph traversal calculation used to study the center of breadth movement in a graph. Normal use of BFS utilizes the line information structure to keep track of incoming hubs. Regardless, it is conceivable to leverage other information structures to perform BFS without using explicit wires.
An alternative way to implement BFS without wires is to utilize two clusters or records: one for the hub at the current level being investigated, and one for the next level hub to be investigated. Initially, the current level list contains the source center.
Calculation starts by highlighting the current level list and going to each hub. For each hub passed through, its adjacent hubs are inspected. If an adjacent hub is not visited, it is marked as visited and added to the other level list. The handle will continue until all hubs in the current level list have been passed.
Once the current level list is fully traversed, the calculation continues to another level list and re-hashes the method to the hub and inspects the next level list. This preparation continues until there are no more unvisited nodes.
usage instructions
Breadth-first method
Breadth-first method
The BFS algorithm starts at the source hub, investigates its neighbors, and most recently moved to neighbors at another level. Use the line information structure to keep track of the hubs you visit. In each cycle, the computation visits a hub, marks it as completed, and queues unvisited adjacent hubs. This preparation will continue until all accessible centers have been visited.
The code initializes a vector adj to represent the infectious list of the chart. Each file of vectors is compared to a center, and each recorded value contains adjacent centers. A BFS traversal is performed by a BFS job, which takes the source hub, the number of hubs N, the vector vis passing through the hub, a separate dp, and the vector v used to keep track of the hubs to be visited. The bfsTraversal job initializes the vanished hub and deletes the vectors, then calls the BFS job to perform the traversal.
algorithm
Create an infection list representation of the graph.
Initialize a line to store the hub to be accessed.
Initialize the disappearing cluster to track disappearing nodes.
Initialize the delete cluster to store on each hub what was deleted from the source hub. Set the source hub's delimiter to 0.
Enqueue the source hub and check if it has been accessed.
Although the pipeline cannot be purged, please do the following:
Delete the hub at the head of the queue. For each neighbor hub that has been dequeued and not yet traversed, do the following: Queue the neighbor hub. Mark adjacent hubs as visited. Updated neighbor hub deletion to dequeue hub deletion (also 1).
Repeat step 6 until the row is empty.
After the BFS traversal is complete, a separate cluster will contain the intervals from the source node to all other centers in the graph.
(Optional) You can also keep track of each hub's parent hub in a BFS traversal to get from the source hub to all other hubs in the simplest way.
Example
#include <iostream> #include <queue> #include <unordered_set> using namespace std; void bfsTraversal(int adjacencyList[][2], int numVertices, int source) { bool visited[numVertices + 1] = {false}; int distances[numVertices + 1] = {0}; queue<int> vertices; vertices.push(source); visited[source] = true; while (!vertices.empty()) { int node = vertices.front(); cout << node << ", "; vertices.pop(); for (int i = 0; i < 2; i++) { int next = adjacencyList[node][i]; if (!visited[next]) { vertices.push(next); distances[next] = distances[node] + 1; visited[next] = true; } } } } int main() { int adjacencyList[][2] = {{0, 0}, {1, 2}, {3, 4}, {0, 0}, {0, 0}}; int numVertices = 4; int source = 2; bfsTraversal(adjacencyList, numVertices, source); return 0; }
Output
2,3,4,0
Example
#include <iostream> #include <vector> using namespace std; void bfsTraversal(vector<vector<int>>& adjacencyList, int N, int source) { vector<bool> visited(N + 1, false); vector<int> distances(N + 1, 0); vector<int> vertices; vertices.push_back(source); visited[source] = true; int curr = 0; while (curr < vertices.size()) { int node = vertices[curr]; cout << node << ", "; for (int i = 0; i < adjacencyList[node].size(); i++) { int next = adjacencyList[node][i]; if (!visited[next]) { vertices.push_back(next); distances[next] = distances[node] + 1; visited[next] = true; } } curr++; } cout << "\nDistances from source " << source << ":\n"; for (int i = 1; i <= N; i++) { cout << "Node " << i << ": " << distances[i] << endl; } } int main() { int N = 8; vector<vector<int>> adjacencyList(N + 1); adjacencyList[0] = {1, 2}; adjacencyList[1] = {2}; adjacencyList[2] = {0, 3}; adjacencyList[3] = {3}; adjacencyList[4] = {5}; adjacencyList[5] = {6, 7}; adjacencyList[6] = {}; adjacencyList[7] = {}; adjacencyList[8] = {}; int source = 5; bfsTraversal(adjacencyList, N, source); return 0; }
Output
5, 6, 7, Distances from source 5: Node 1: 0 Node 2: 0 Node 3: 0 Node 4: 0 Node 5: 0 Node 6: 1 Node 7: 1 Node 8: 0
in conclusion
This article describes breadth-first search (BFS) calculations that do not use row information structures. BFS calculations are typically used to navigate a chart in a step-by-step fashion starting from a given source center. Typically, a route is used to store hubs to travel to. Regardless, this article examines an alternative approach that utilizes a basic list or clustering to store the next level of hubs.
This selective use completes the breadth-first study of graphs. This article traces the steps of BFS computation, such as initializing infectious records, maintaining go-to and separation clusters, and using circles to emphasize central levels. It also provides C code instructions illustrating BFS traversal without using a single line. The code accurately studies the graph, prints the BFS traversal permutation, and calculates the distance from the source hub to all other nodes. Overall, this article provides a clear explanation and feasible usage of BFS calculations without the use of lines, demonstrating an alternative approach to navigating graphs in a breadth-first manner.
The above is the detailed content of Breadth-first search does not use queues. For more information, please follow other related articles on the PHP Chinese website!
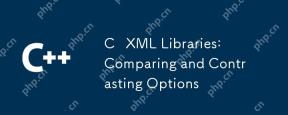
There are four commonly used XML libraries in C: TinyXML-2, PugiXML, Xerces-C, and RapidXML. 1.TinyXML-2 is suitable for environments with limited resources, lightweight but limited functions. 2. PugiXML is fast and supports XPath query, suitable for complex XML structures. 3.Xerces-C is powerful, supports DOM and SAX resolution, and is suitable for complex processing. 4. RapidXML focuses on performance and parses extremely fast, but does not support XPath queries.
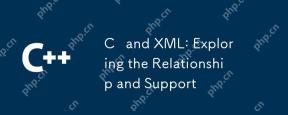
C interacts with XML through third-party libraries (such as TinyXML, Pugixml, Xerces-C). 1) Use the library to parse XML files and convert them into C-processable data structures. 2) When generating XML, convert the C data structure to XML format. 3) In practical applications, XML is often used for configuration files and data exchange to improve development efficiency.
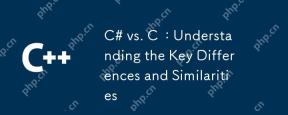
The main differences between C# and C are syntax, performance and application scenarios. 1) The C# syntax is more concise, supports garbage collection, and is suitable for .NET framework development. 2) C has higher performance and requires manual memory management, which is often used in system programming and game development.
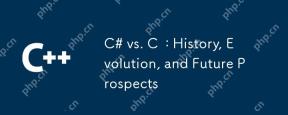
The history and evolution of C# and C are unique, and the future prospects are also different. 1.C was invented by BjarneStroustrup in 1983 to introduce object-oriented programming into the C language. Its evolution process includes multiple standardizations, such as C 11 introducing auto keywords and lambda expressions, C 20 introducing concepts and coroutines, and will focus on performance and system-level programming in the future. 2.C# was released by Microsoft in 2000. Combining the advantages of C and Java, its evolution focuses on simplicity and productivity. For example, C#2.0 introduced generics and C#5.0 introduced asynchronous programming, which will focus on developers' productivity and cloud computing in the future.
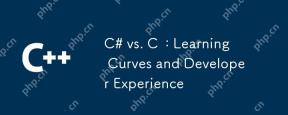
There are significant differences in the learning curves of C# and C and developer experience. 1) The learning curve of C# is relatively flat and is suitable for rapid development and enterprise-level applications. 2) The learning curve of C is steep and is suitable for high-performance and low-level control scenarios.
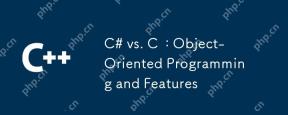
There are significant differences in how C# and C implement and features in object-oriented programming (OOP). 1) The class definition and syntax of C# are more concise and support advanced features such as LINQ. 2) C provides finer granular control, suitable for system programming and high performance needs. Both have their own advantages, and the choice should be based on the specific application scenario.
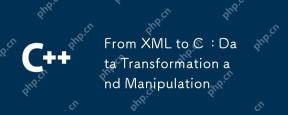
Converting from XML to C and performing data operations can be achieved through the following steps: 1) parsing XML files using tinyxml2 library, 2) mapping data into C's data structure, 3) using C standard library such as std::vector for data operations. Through these steps, data converted from XML can be processed and manipulated efficiently.
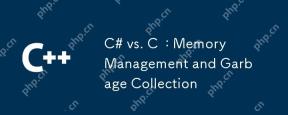
C# uses automatic garbage collection mechanism, while C uses manual memory management. 1. C#'s garbage collector automatically manages memory to reduce the risk of memory leakage, but may lead to performance degradation. 2.C provides flexible memory control, suitable for applications that require fine management, but should be handled with caution to avoid memory leakage.


Hot AI Tools

Undresser.AI Undress
AI-powered app for creating realistic nude photos

AI Clothes Remover
Online AI tool for removing clothes from photos.

Undress AI Tool
Undress images for free

Clothoff.io
AI clothes remover

Video Face Swap
Swap faces in any video effortlessly with our completely free AI face swap tool!

Hot Article

Hot Tools
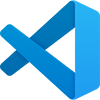
VSCode Windows 64-bit Download
A free and powerful IDE editor launched by Microsoft

DVWA
Damn Vulnerable Web App (DVWA) is a PHP/MySQL web application that is very vulnerable. Its main goals are to be an aid for security professionals to test their skills and tools in a legal environment, to help web developers better understand the process of securing web applications, and to help teachers/students teach/learn in a classroom environment Web application security. The goal of DVWA is to practice some of the most common web vulnerabilities through a simple and straightforward interface, with varying degrees of difficulty. Please note that this software

PhpStorm Mac version
The latest (2018.2.1) professional PHP integrated development tool

SublimeText3 English version
Recommended: Win version, supports code prompts!

Atom editor mac version download
The most popular open source editor