


The basic visual model based on Transformer has shown very powerful performance in various downstream tasks, such as segmentation and detection, and models such as DINO have emerged with semantic segmentation attributes after self-supervised training.
It is strange that the visual Transformer model does not have similar emergent capabilities after being trained for supervised classification
Recently, Professor Ma Yi's team studied models based on the Transformer architecture to explore whether the emergent segmentation ability is simply the result of a complex self-supervised learning mechanism, or whether the same performance can be achieved under more general conditions by appropriately designing the model architecture. Emergence
Code link: https://github.com/Ma-Lab-Berkeley/CRATE
Please click the following link to view the paper: https://arxiv.org/abs/2308.16271
After a large number of experiments, the researchers proved that when using the white-box Transformer model CRATE, its design Explicitly model and pursue low-dimensional structure in data distributions to emerge whole- and part-level segmentation properties with minimally supervised training recipes
Through hierarchical fine-grained analysis, we get An important conclusion is drawn: emergent properties strongly confirm the design mathematical function of white-box networks. Based on this result, we proposed a method to design a white-box basic model that is not only high-performance but also fully mathematically interpretable
Professor Ma Yi also said, Research on deep learning will gradually shift from empirical design to theoretical guidance.
The emergent properties of white-box CRATE
DINO’s segmentation-emergent ability refers to the DINO model’s ability to process language tasks At this time, the input sentence can be divided into smaller fragments and each fragment can be processed independently. This ability enables the DINO model to better understand complex sentence structures and semantic information, thereby improving its performance in the field of natural language processing
Representation learning in intelligent systems It aims to convert the world's high-dimensional, multi-modal sensory data (images, language, speech) into a more compact form while retaining its basic low-dimensional structure to achieve efficient recognition (such as classification) and grouping (such as segmentation) and tracking.
The training of deep learning models usually adopts a data-driven approach, by inputting large-scale data and learning in a self-supervised manner
Among the basic vision models, the DINO model shows surprising emergence capabilities. ViTs can recognize explicit semantic segmentation information even without supervised segmentation training. The DINO model with self-supervised Transformer architecture performs well in this regard
Follow-up work has studied how to utilize this segmentation information in the DINO model and perform it in downstream tasks such as segmentation and detection. etc. have achieved state-of-the-art performance, and some work has also proven that the penultimate layer features in ViTs trained with DINO are strongly related to the salience information in the visual input, such as distinguishing foreground, background and object boundaries, thereby improving image segmentation and other task performance.
In order to highlight the segmentation attributes, DINO needs to skillfully combine self-supervised learning, knowledge distillation and weight averaging methods during the training process
It is unclear whether each component introduced in DINO is essential for the emergence of segmentation masks. Although DINO also adopts the ViT architecture as its backbone, in the ordinary supervised ViT model trained on the classification task, and No segmentation emergent behavior was observed.
The emergence of CRATE
Based on the successful case of DINO, researchers want to explore complex self-supervised learning Is the pipeline necessary to obtain emergent properties in Transformer-like visual models?
The researchers believe that a promising way to promote segmentation properties in Transformer models is to design the Transformer model architecture taking into account the input data structure, which also represents representation learning. A combination of classic methods and modern data-driven deep learning frameworks.
Compared with the current mainstream Transformer model, this design method can also be called a white-box Transformer model.
Based on the previous work of Professor Ma Yi’s group, researchers conducted extensive experiments on the CRATE model with a white-box architecture, proving that CRATE’s white-box design is the reason for the emergence of segmentation attributes in self-attention graphs.
What needs to be rephrased is: Qualitative assessment
The researcher uses the [CLS] token-based attention Strive to explain and visualize the model using the graph method and find that the query-key-value matrices in CRATE are all the same
The CRATE model can be observed The self-attention map can correspond to the semantics of the input image. The internal network of the model performs clear semantic segmentation on each image, achieving an effect similar to the DINO model.
Ordinary ViT does not show similar segmentation properties when trained on supervised classification tasks
Based on previous research on visual image learning of block-by-block deep features, researchers conducted principal component analysis (PCA) research on deep token representations of CRATE and ViT models
It can be found that CRATE can still capture the boundaries of objects in the image without segmentation supervision training.
Moreover, the principal components also indicate the feature alignment of similar parts between the token and the object, such as the red channel corresponding to the horse’s leg
The degree of PCA visualization structure of the supervised ViT model is quite low.
Quantitative Evaluation
The researchers used existing segmentations to evaluate the CRATE emergent segmentation properties. and object detection technology
As can be seen from the self-attention map, CRATE explicitly captures object-level semantics with clear boundaries. In order to quantitatively measure the quality of segmentation, the researchers used self-attention to The attention map generates a segmentation mask and compares the standard mIoU (mean intersection-over-union ratio) with the real mask.
It can be seen from the experimental results that CRATE is significantly better than ViT in terms of visual and mIOU scores, which shows that the internal representation of CRATE is useful for generating segmentation masks. Mask tasks more efficiently
Object detection and fine-grained segmentation
To further validate and evaluate the rich semantics captured by CRATE Information, the researchers adopted MaskCut, an efficient object detection and segmentation method, to obtain an automated evaluation model without manual annotation, and can extract more fine-grained segmentation from images based on the token representation learned by CRATE.
As you can see in the segmentation results on COCO val2017, there is an internal representation of CRATE in the detection and The segmentation index is better than that of supervised ViT. MaskCut with supervised ViT features cannot even produce segmentation masks at all in some cases.
White box analysis of CRATE’s segmentation capabilities
The role of depth in CRATE
The design of each layer of CRATE follows the same conceptual purpose: to optimize sparse rate reduction and transform token distribution into a compact and structured form. After rewriting: The design of each level of CRATE follows the same philosophy: optimize the reduction of sparse rate, and transform the distribution of tokens into a compact and structured form
Assume CRATE The emergence of semantic segmentation capabilities is similar to "representing clusters of tokens belonging to similar semantic categories in Z". It is expected that CRATE's segmentation performance can improve with increasing depth.
To test this, the researchers used the MaskCut pipeline to quantitatively evaluate segmentation performance across internal representations across different layers; and also applied PCA visualization to understand how segmentation emerges with depth. .
It can be observed from the experimental results that the segmentation score improves when using representations from deeper layers, which is very consistent with CRATE’s incremental optimization design.
In contrast, even though the performance of ViT-B/8 improves slightly in later layers, its segmentation scores are significantly lower than CRATE. The PCA results show that deep extraction from CRATE The representation will gradually pay more attention to foreground objects and be able to capture texture-level details.
melting experiment in CRATE
Attention block (MSSA) in CRATE and The MLP block (ISTA) is different from the attention block in ViT
To study the impact of each component on the emergent segmentation properties of CRATE, the researchers selected three CRATE variants: CRATE, CRATE-MHSA, CRATE-MLP. These variants represent the attention block (MHSA) and the MLP block in ViT respectively
The researchers applied the same pre-training settings on the ImageNet-21k dataset and then applied coarse segmentation evaluation and mask segmentation evaluation to quantitatively compare the performance of different models.
According to experimental results, CRATE significantly outperforms other model architectures in all tasks. It is worth noting that although the architectural difference between MHSA and MSSA is small, simply replacing MHSA in ViT with MSSA in CRATE can significantly improve ViT's coarse segmentation performance (i.e. VOC Seg). Performance. This further proves the effectiveness of white-box design
The content that needs to be rewritten is: pay attention to the identification of the semantic attributes of the header
[CLS] The self-attention map between the token and the image block token can see a clear segmentation mask. According to intuition, each attention head should be able to capture some features of the data.
The researchers first input the image into the CRATE model, and then had a human inspect and select four attention heads that seemed to have semantic meaning; then they used these attention heads on other input images. Self-attention map visualization on the head.
Observation can be found that each attention head can capture different parts of the object, and even different semantics. For example, the attention head in the first column can capture the legs of different animals, while the attention head in the last column can capture the ears and head
Since it is deformable This ability to parse visual input into part-whole hierarchies has been a goal of recognition architecture since the release of deformable part models and capsule networks, and the CRATE model of white-box design also has this ability.
The above is the detailed content of Professor Ma Yi's new work: White-box ViT successfully achieves 'partitioned emergence', is the era of empirical deep learning coming to an end?. For more information, please follow other related articles on the PHP Chinese website!
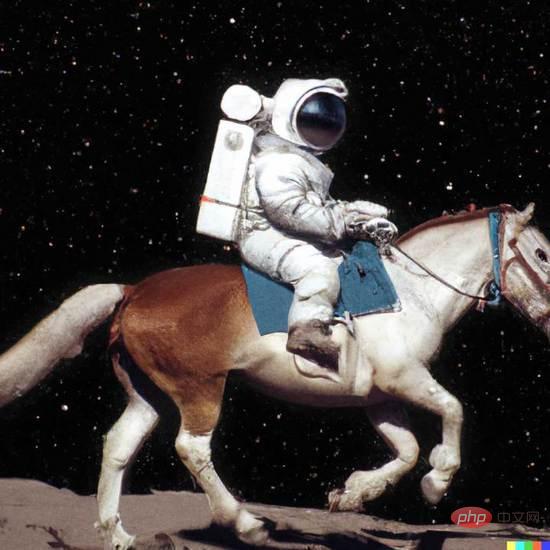
1 前言在发布DALL·E的15个月后,OpenAI在今年春天带了续作DALL·E 2,以其更加惊艳的效果和丰富的可玩性迅速占领了各大AI社区的头条。近年来,随着生成对抗网络(GAN)、变分自编码器(VAE)、扩散模型(Diffusion models)的出现,深度学习已向世人展现其强大的图像生成能力;加上GPT-3、BERT等NLP模型的成功,人类正逐步打破文本和图像的信息界限。在DALL·E 2中,只需输入简单的文本(prompt),它就可以生成多张1024*1024的高清图像。这些图像甚至
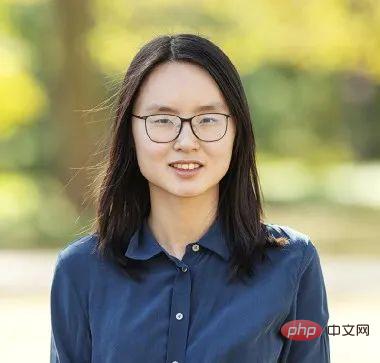
“Making large models smaller”这是很多语言模型研究人员的学术追求,针对大模型昂贵的环境和训练成本,陈丹琦在智源大会青源学术年会上做了题为“Making large models smaller”的特邀报告。报告中重点提及了基于记忆增强的TRIME算法和基于粗细粒度联合剪枝和逐层蒸馏的CofiPruning算法。前者能够在不改变模型结构的基础上兼顾语言模型困惑度和检索速度方面的优势;而后者可以在保证下游任务准确度的同时实现更快的处理速度,具有更小的模型结构。陈丹琦 普
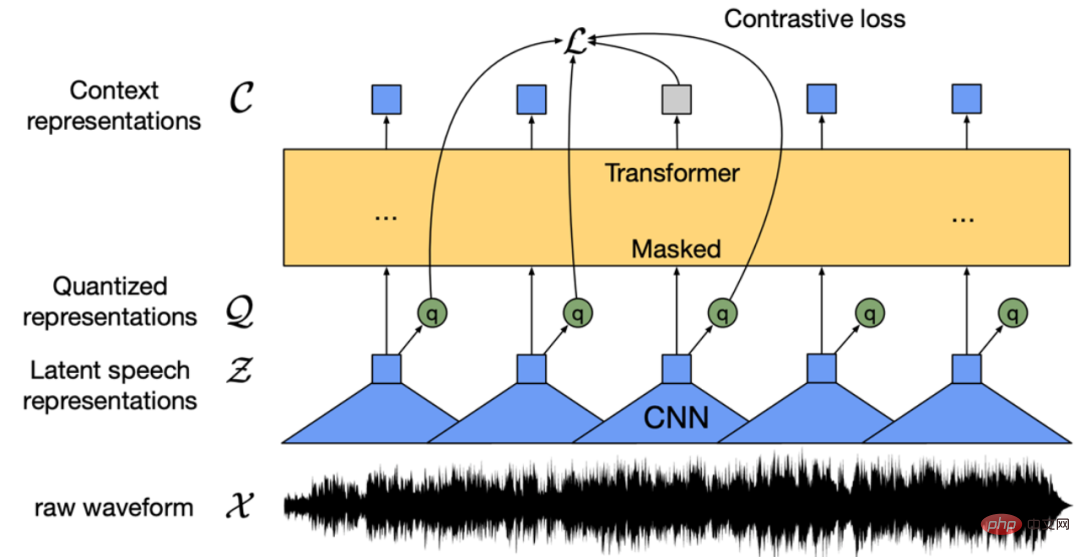
Wav2vec 2.0 [1],HuBERT [2] 和 WavLM [3] 等语音预训练模型,通过在多达上万小时的无标注语音数据(如 Libri-light )上的自监督学习,显著提升了自动语音识别(Automatic Speech Recognition, ASR),语音合成(Text-to-speech, TTS)和语音转换(Voice Conversation,VC)等语音下游任务的性能。然而这些模型都没有公开的中文版本,不便于应用在中文语音研究场景。 WenetSpeech [4] 是
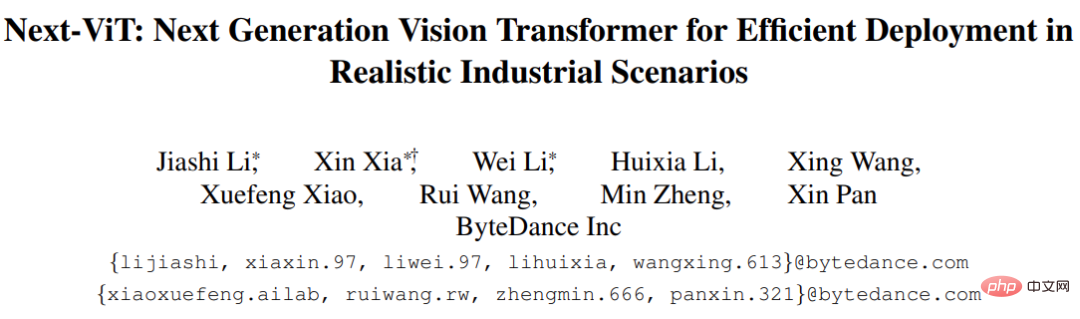
由于复杂的注意力机制和模型设计,大多数现有的视觉 Transformer(ViT)在现实的工业部署场景中不能像卷积神经网络(CNN)那样高效地执行。这就带来了一个问题:视觉神经网络能否像 CNN 一样快速推断并像 ViT 一样强大?近期一些工作试图设计 CNN-Transformer 混合架构来解决这个问题,但这些工作的整体性能远不能令人满意。基于此,来自字节跳动的研究者提出了一种能在现实工业场景中有效部署的下一代视觉 Transformer——Next-ViT。从延迟 / 准确性权衡的角度看,
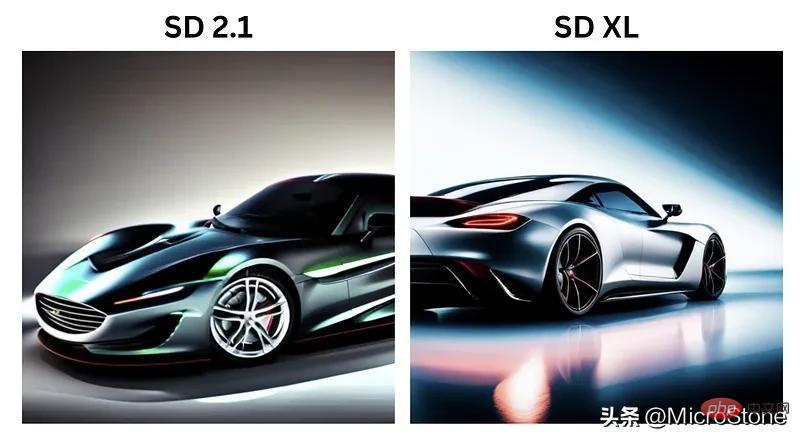
3月27号,Stability AI的创始人兼首席执行官Emad Mostaque在一条推文中宣布,Stable Diffusion XL 现已可用于公开测试。以下是一些事项:“XL”不是这个新的AI模型的官方名称。一旦发布稳定性AI公司的官方公告,名称将会更改。与先前版本相比,图像质量有所提高与先前版本相比,图像生成速度大大加快。示例图像让我们看看新旧AI模型在结果上的差异。Prompt: Luxury sports car with aerodynamic curves, shot in a
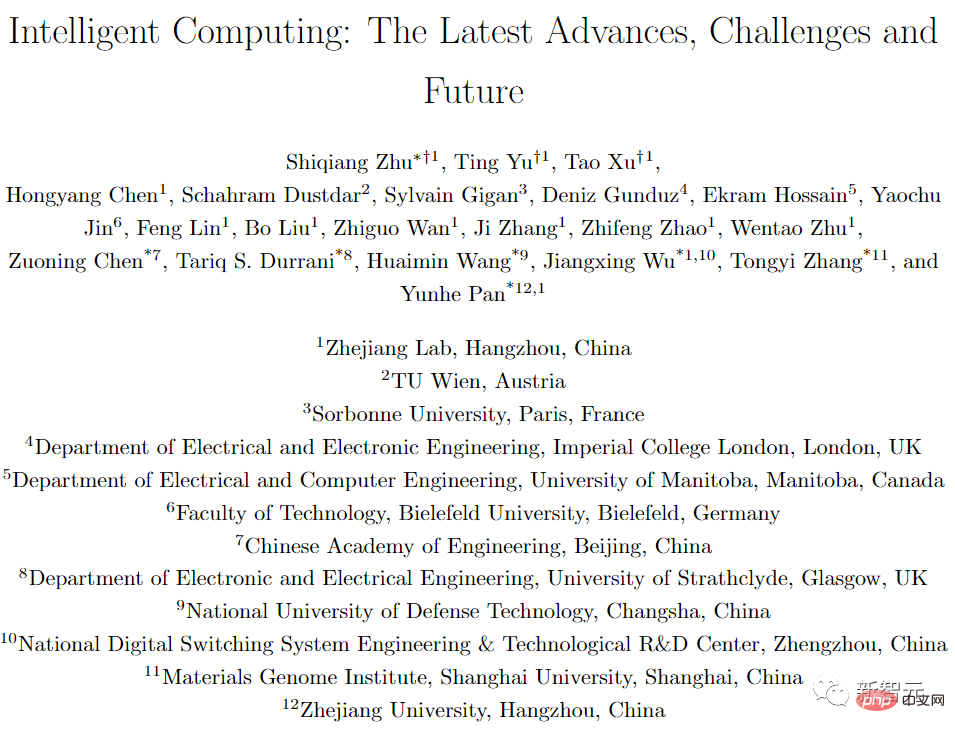
人工智能就是一个「拼财力」的行业,如果没有高性能计算设备,别说开发基础模型,就连微调模型都做不到。但如果只靠拼硬件,单靠当前计算性能的发展速度,迟早有一天无法满足日益膨胀的需求,所以还需要配套的软件来协调统筹计算能力,这时候就需要用到「智能计算」技术。最近,来自之江实验室、中国工程院、国防科技大学、浙江大学等多达十二个国内外研究机构共同发表了一篇论文,首次对智能计算领域进行了全面的调研,涵盖了理论基础、智能与计算的技术融合、重要应用、挑战和未来前景。论文链接:https://spj.scien
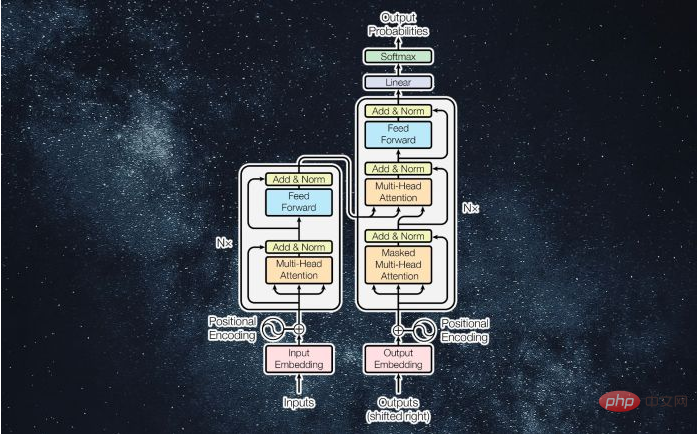
译者 | 李睿审校 | 孙淑娟近年来, Transformer 机器学习模型已经成为深度学习和深度神经网络技术进步的主要亮点之一。它主要用于自然语言处理中的高级应用。谷歌正在使用它来增强其搜索引擎结果。OpenAI 使用 Transformer 创建了著名的 GPT-2和 GPT-3模型。自从2017年首次亮相以来,Transformer 架构不断发展并扩展到多种不同的变体,从语言任务扩展到其他领域。它们已被用于时间序列预测。它们是 DeepMind 的蛋白质结构预测模型 AlphaFold
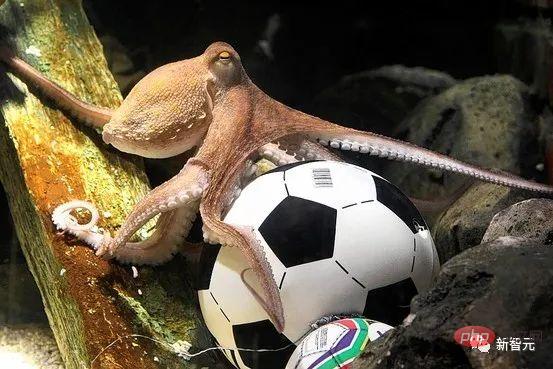
说起2010年南非世界杯的最大网红,一定非「章鱼保罗」莫属!这只位于德国海洋生物中心的神奇章鱼,不仅成功预测了德国队全部七场比赛的结果,还顺利地选出了最终的总冠军西班牙队。不幸的是,保罗已经永远地离开了我们,但它的「遗产」却在人们预测足球比赛结果的尝试中持续存在。在艾伦图灵研究所(The Alan Turing Institute),随着2022年卡塔尔世界杯的持续进行,三位研究员Nick Barlow、Jack Roberts和Ryan Chan决定用一种AI算法预测今年的冠军归属。预测模型图


Hot AI Tools

Undresser.AI Undress
AI-powered app for creating realistic nude photos

AI Clothes Remover
Online AI tool for removing clothes from photos.

Undress AI Tool
Undress images for free

Clothoff.io
AI clothes remover

AI Hentai Generator
Generate AI Hentai for free.

Hot Article

Hot Tools

Zend Studio 13.0.1
Powerful PHP integrated development environment

Notepad++7.3.1
Easy-to-use and free code editor

SecLists
SecLists is the ultimate security tester's companion. It is a collection of various types of lists that are frequently used during security assessments, all in one place. SecLists helps make security testing more efficient and productive by conveniently providing all the lists a security tester might need. List types include usernames, passwords, URLs, fuzzing payloads, sensitive data patterns, web shells, and more. The tester can simply pull this repository onto a new test machine and he will have access to every type of list he needs.
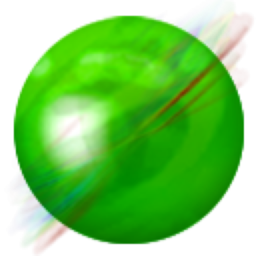
ZendStudio 13.5.1 Mac
Powerful PHP integrated development environment
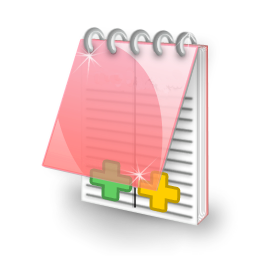
EditPlus Chinese cracked version
Small size, syntax highlighting, does not support code prompt function
