In coordination diagrams, identifying hubs that do not belong to any cycle is crucial for different applications. These centers form the basis of acyclic subgraphs and play an important role in understanding the general graph structure. By using efficient graph intersection calculations, such as Profundity First Hunt (DFS) or Tarjan's calculation of closely related parts, we can effortlessly decide and print hubs that do not participate in any loops. These methods ensure the characterization of centers without circular collaboration, provide important knowledge for the non-circular parts of diagrams, and support different critical thinking situations related to diagrams.
usage instructions
Depth-first search (DFS) with loop detection
Tarjan’s strongly connected component algorithm
Depth-first search (DFS) with loop detection
In this approach, we use depth-first tracking (DFS) to navigate the coordination chart and distinguish cycles on the way. We mark visited centers and keep a list so that centers can be tracked in an ongoing DFS manner. If we hit a trailing edge (reaching the edge of the hub in a sustained DFS manner), we differentiate a cycle. At the end of DFS, the center in the ongoing DFS way will be important for a cycle. Hubs that do not use persistent DFS are not part of any loop and can be printed.
algorithm
Perform a Deep First Hunt (DFS) from each unvisited center on the chart.
During DFS, visited hubs are marked and added to the ongoing DFS path list.
If we encounter a trailing edge (an edge to a hub in the current DFS mode), we distinguish a cycle and mark all hubs in the current DFS mode as part of the cycle.
When DFS for a hub is complete, remove it from the list of in-progress DFS paths.
After completing the DFS of all hubs, the hubs that do not belong to any cycle will remain unchanged and we can print them.
Example
#include <iostream> #include <vector> class Graph { public: Graph(int numVertices); void addEdge(int src, int dest); void DFS(); private: void DFSUtil(int v, std::vector<bool>& visited, std::vector<int>& dfsPath); int numVertices; std::vector<std::vector<int>> adjList; }; Graph::Graph(int numVertices) : numVertices(numVertices) { adjList.resize(numVertices); } void Graph::addEdge(int src, int dest) { adjList[src].push_back(dest); } void Graph::DFSUtil(int v, std::vector<bool>& visited, std::vector<int>& dfsPath) { visited[v] = true; dfsPath.push_back(v); for (int neighbor : adjList[v]) { if (!visited[neighbor]) { DFSUtil(neighbor, visited, dfsPath); } else { std::cout << "Cycle found: "; for (size_t i = 0; i < dfsPath.size(); ++i) { if (dfsPath[i] == neighbor) { while (i < dfsPath.size()) { std::cout << dfsPath[i] << " "; ++i; } break; } } std::cout << std::endl; } } dfsPath.pop_back(); } void Graph::DFS() { std::vector<bool> visited(numVertices, false); std::vector<int> dfsPath; for (int i = 0; i < numVertices; ++i) { if (!visited[i]) { DFSUtil(i, visited, dfsPath); } } } int main() { Graph graph(6); graph.addEdge(0, 1); graph.addEdge(1, 2); graph.addEdge(2, 3); graph.addEdge(3, 4); graph.addEdge(4, 1); graph.addEdge(4, 5); std::cout << "DFS traversal with cycle detection:\n"; graph.DFS(); return 0; }
Output
DFS traversal with cycle detection: Cycle found: 1 2 3 4
Tarjan’s strongly connected component algorithm
Tarjan's calculation is a powerful calculation used to track all key related parts of the coordination diagram. Explicitly related parts are subsets of hubs for which coordination exists between any two hubs in the subset. A hub that is not part of any closely related component is not part of any cycle. By finding key associated parts we can identify hubs that do not belong to any cycle and print them\
algorithm
Apply Tarjan's calculations to the bootstrapping diagram to track all key relevant parts.
After tracing all the important related parts, distinguish the centers that are crucial for the closely related parts.
Hubs that do not belong to any explicitly associated parts do not belong to any loop and can be printed.
Both methods do differentiate and print centers that do not belong to any cycle in the coordination chart. The DFS method provides a simpler and more straightforward implementation, while Tarjan's calculations are more complex but provide additional data on focused correlation parts, which can be helpful for specific chart-related tasks. The decision on approach depends on the specific needs and the context of the main pressing issues.
Example
#include <iostream> #include <vector> #include <stack> #include <algorithm> using namespace std; class Graph { int V; vector<vector<int>> adj; vector<bool> visited; vector<int> disc, low; stack<int> st; vector<vector<int>> SCCs; vector<bool> essentialNodes; public: Graph(int V) : V(V) { adj.resize(V); visited.resize(V, false); disc.resize(V, -1); low.resize(V, -1); essentialNodes.resize(V, true); } void addEdge(int u, int v) { adj[u].push_back(v); } void tarjanDFS(int u) { static int time = 0; disc[u] = low[u] = ++time; st.push(u); visited[u] = true; for (int v : adj[u]) { if (disc[v] == -1) { tarjanDFS(v); low[u] = min(low[u], low[v]); } else if (visited[v]) { low[u] = min(low[u], disc[v]); } } if (low[u] == disc[u]) { vector<int> SCC; int v; do { v = st.top(); st.pop(); SCC.push_back(v); visited[v] = false; } while (v != u); SCCs.push_back(SCC); } } void tarjan() { for (int i = 0; i < V; ++i) { if (disc[i] == -1) { tarjanDFS(i); } } } void identifyEssentialNodes() { for (const vector<int>& SCC : SCCs) { for (int v : SCC) { for (int u : adj[v]) { if (find(SCC.begin(), SCC.end(), u) == SCC.end()) { essentialNodes[u] = false; } } } } } void printEssentialNodes() { cout << "Essential Nodes for Each SCC:\n"; for (int i = 0; i < V; ++i) { if (essentialNodes[i]) { cout << i << " "; } } cout << endl; } }; int main() { Graph g(6); g.addEdge(0, 1); g.addEdge(1, 2); g.addEdge(2, 0); g.addEdge(1, 3); g.addEdge(3, 4); g.addEdge(4, 5); g.addEdge(5, 3); g.tarjan(); g.identifyEssentialNodes(); g.printEssentialNodes(); return 0; }
Output
Essential Nodes for Each SCC: 0 1 2 4 5
in conclusion
These two methods do solve the problem of identifying centers that do not belong to any cycle in the coordination chart. The DFS method is easy to implement and does not require many additional information structures. Tarjan's calculations, on the other hand, provide additional data on key correlation components, which may be helpful in certain situations.
The decision between the two methods depends on the specific prerequisites of the problem and the requirements for additional data passing through period-independent differentiation centers. In general, if the only goal is to find hubs that do not belong to any cycle, the DFS approach may be favored for its simplicity. Nonetheless, Tarjan's calculations may be an important tool if further examination of key relevant parts is required. Both methods provide proficient arrangements and can be adapted to the properties of the coordination chart and the desired outcome of the exam
The above is the detailed content of Print nodes in a directed graph that do not belong to any cycle. For more information, please follow other related articles on the PHP Chinese website!
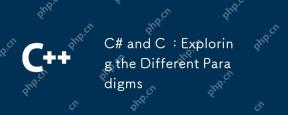
The main differences between C# and C are memory management, polymorphism implementation and performance optimization. 1) C# uses a garbage collector to automatically manage memory, while C needs to be managed manually. 2) C# realizes polymorphism through interfaces and virtual methods, and C uses virtual functions and pure virtual functions. 3) The performance optimization of C# depends on structure and parallel programming, while C is implemented through inline functions and multithreading.
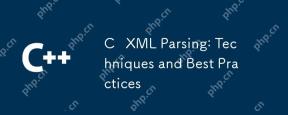
The DOM and SAX methods can be used to parse XML data in C. 1) DOM parsing loads XML into memory, suitable for small files, but may take up a lot of memory. 2) SAX parsing is event-driven and is suitable for large files, but cannot be accessed randomly. Choosing the right method and optimizing the code can improve efficiency.
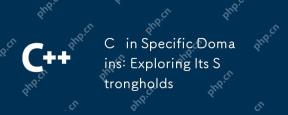
C is widely used in the fields of game development, embedded systems, financial transactions and scientific computing, due to its high performance and flexibility. 1) In game development, C is used for efficient graphics rendering and real-time computing. 2) In embedded systems, C's memory management and hardware control capabilities make it the first choice. 3) In the field of financial transactions, C's high performance meets the needs of real-time computing. 4) In scientific computing, C's efficient algorithm implementation and data processing capabilities are fully reflected.
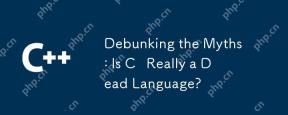
C is not dead, but has flourished in many key areas: 1) game development, 2) system programming, 3) high-performance computing, 4) browsers and network applications, C is still the mainstream choice, showing its strong vitality and application scenarios.
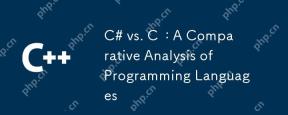
The main differences between C# and C are syntax, memory management and performance: 1) C# syntax is modern, supports lambda and LINQ, and C retains C features and supports templates. 2) C# automatically manages memory, C needs to be managed manually. 3) C performance is better than C#, but C# performance is also being optimized.
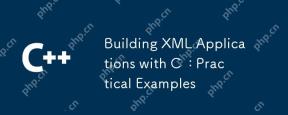
You can use the TinyXML, Pugixml, or libxml2 libraries to process XML data in C. 1) Parse XML files: Use DOM or SAX methods, DOM is suitable for small files, and SAX is suitable for large files. 2) Generate XML file: convert the data structure into XML format and write to the file. Through these steps, XML data can be effectively managed and manipulated.
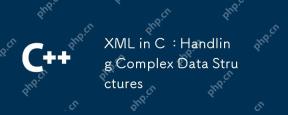
Working with XML data structures in C can use the TinyXML or pugixml library. 1) Use the pugixml library to parse and generate XML files. 2) Handle complex nested XML elements, such as book information. 3) Optimize XML processing code, and it is recommended to use efficient libraries and streaming parsing. Through these steps, XML data can be processed efficiently.
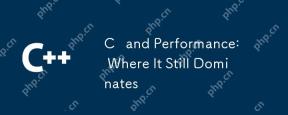
C still dominates performance optimization because its low-level memory management and efficient execution capabilities make it indispensable in game development, financial transaction systems and embedded systems. Specifically, it is manifested as: 1) In game development, C's low-level memory management and efficient execution capabilities make it the preferred language for game engine development; 2) In financial transaction systems, C's performance advantages ensure extremely low latency and high throughput; 3) In embedded systems, C's low-level memory management and efficient execution capabilities make it very popular in resource-constrained environments.


Hot AI Tools

Undresser.AI Undress
AI-powered app for creating realistic nude photos

AI Clothes Remover
Online AI tool for removing clothes from photos.

Undress AI Tool
Undress images for free

Clothoff.io
AI clothes remover

Video Face Swap
Swap faces in any video effortlessly with our completely free AI face swap tool!

Hot Article

Hot Tools
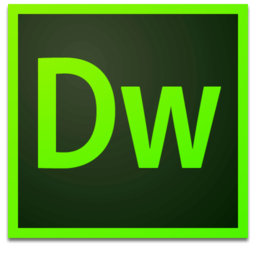
Dreamweaver Mac version
Visual web development tools

SublimeText3 Linux new version
SublimeText3 Linux latest version

mPDF
mPDF is a PHP library that can generate PDF files from UTF-8 encoded HTML. The original author, Ian Back, wrote mPDF to output PDF files "on the fly" from his website and handle different languages. It is slower than original scripts like HTML2FPDF and produces larger files when using Unicode fonts, but supports CSS styles etc. and has a lot of enhancements. Supports almost all languages, including RTL (Arabic and Hebrew) and CJK (Chinese, Japanese and Korean). Supports nested block-level elements (such as P, DIV),

DVWA
Damn Vulnerable Web App (DVWA) is a PHP/MySQL web application that is very vulnerable. Its main goals are to be an aid for security professionals to test their skills and tools in a legal environment, to help web developers better understand the process of securing web applications, and to help teachers/students teach/learn in a classroom environment Web application security. The goal of DVWA is to practice some of the most common web vulnerabilities through a simple and straightforward interface, with varying degrees of difficulty. Please note that this software

Atom editor mac version download
The most popular open source editor
