Good problem solving in computer science relies heavily on efficient algorithms such as Greedy Best First Search (GBFS). GBFS has established credibility as the best solution to pathfinding or optimization problems. Therefore, in this article we discuss GBFS in depth while exploring its implementation in C.
grammar
void greedyBestFirstSearch(Graph graph, Node startNode, Node goalNode);
algorithm
The greedy best first search algorithm aims to find the path from a given start node to a target node in the graph. The following are the general steps of the algorithm -
Initialize an empty priority queue.
Put the starting node into the priority queue.
Create an empty set to track visited nodes.
When the priority queue is not empty -
Dequeue the node with the highest priority from the queue.
If the dequeued node is the target node, the algorithm terminates and the path is found.
Otherwise, mark the dequeue node as visited.
Put all unvisited neighbor nodes of the dequeued node into the priority queue.
If the priority queue becomes empty before reaching the destination node, no path exists.
Method 1: Heuristic function based on Euclidean distance
Example
#include <iostream> #include <queue> #include <cmath> #include <vector> #include <unordered_set> using namespace std; // Structure to represent a node in the graph struct Node { int x, y; // Coordinates of the node int cost; // Cost to reach this node }; // Euclidean distance heuristic function double euclideanDistance(int x1, int y1, int x2, int y2) { return sqrt(pow((x1 - x2), 2) + pow((y1 - y2), 2)); } // Custom comparison function for nodes in the priority queue struct NodeCompare { bool operator()(const Node& node1, const Node& node2) const { return node1.cost > node2.cost; } }; // Greedy Best-First Search function void greedyBestFirstSearch(vector<vector<int>>& graph, Node start, Node goal) { int rows = graph.size(); int cols = graph[0].size(); // Priority queue for nodes to be explored priority_queue<Node, vector<Node>, NodeCompare> pq; // Visited nodes set unordered_set<int> visited; // Add the start node to the priority queue pq.push(start); while (!pq.empty()) { // Get the node with the lowest cost Node current = pq.top(); pq.pop(); // Check if the current node is the goal node if (current.x == goal.x && current.y == goal.y) { cout << "Goal node reached!" << endl; return; } // Mark the current node as visited int nodeId = current.x * cols + current.y; visited.insert(nodeId); // Explore the neighboring nodes int dx[] = {-1, 1, 0, 0}; // Possible x-direction movements int dy[] = {0, 0, -1, 1}; // Possible y-direction movements for (int i = 0; i < 4; i++) { int newX = current.x + dx[i]; int newY = current.y + dy[i]; // Check if the neighboring node is within the graph boundaries if (newX >= 0 && newX < rows && newY >= 0 && newY < cols) { // Calculate the heuristic value for the neighboring node double heuristicValue = euclideanDistance(newX, newY, goal.x, goal.y); // Check if the neighboring node has not been visited if (visited.find(newX * cols + newY) == visited.end()) { // Create a new node for the neighboring position Node neighbor; neighbor.x = newX; neighbor.y = newY; neighbor.cost = current.cost + graph[newX][newY]; // Add the neighboring node to the priority queue pq.push(neighbor); } } } } cout << "Goal node not reachable!" << endl; } int main() { // Example graph represented as a 2D vector vector<vector<int>> graph = { {3, 5, 1, 2}, {1, 3, 2, 4}, {5, 2, 6, 7}, {4, 3, 1, 2} }; Node start; start.x = 0; // Starting x-coordinate start.y = 0; // Starting y-coordinate start.cost = 0; // Cost to reach the starting node Node goal; goal.x = 3; // Goal x-coordinate goal.y = 3; // Goal y-coordinate // Run Greedy Best-First Search algorithm greedyBestFirstSearch(graph, start, goal); return 0; }
Output
Goal node reached!
illustrate
This code contains two key elements. First, it contains the definition of the Graph class, which represents a graph structure using adjacency lists.
Secondly, it introduces CompareEuclideanDistance - a custom comparator for evaluating nodes by estimating their distance from the target node using the Euclidean distance formula.
greedyBestFirstSearch function implements the greedy best first search algorithm. It uses a priority queue to store nodes based on their heuristic values.
This algorithm first puts the starting node into the priority queue.
In each iteration, it dequeues the highest priority node and checks if it is the target node.
If the target node is found, the "Path Found!" message will be printed. Otherwise, the algorithm marks the dequeued node as visited and enqueues its unvisited neighboring nodes.
If the priority queue becomes empty and no target node is found, a "Path does not exist!" message is printed.
The main function demonstrates the usage of the algorithm by creating a graph, defining the starting node and target node, and calling the greedyBestFirstSearch function.
Method 2: Heuristic function based on Manhattan distance
Our strategy for solving this problem requires the use of a heuristic function that relies on the concept of Manhattan distance. This distance measure, sometimes called taxi distance, involves adding the horizontal and vertical distances between nodes.
Example
#include <iostream> #include <queue> #include <cmath> #include <vector> #include <unordered_set> using namespace std; // Structure to represent a node in the graph struct Node { int x, y; // Coordinates of the node int cost; // Cost to reach this node }; // Manhattan distance heuristic function int manhattanDistance(int x1, int y1, int x2, int y2) { return abs(x1 - x2) + abs(y1 - y2); } // Custom comparison function for nodes in the priority queue struct NodeCompare { bool operator()(const Node& node1, const Node& node2) const { return node1.cost > node2.cost; } }; // Greedy Best-First Search function void greedyBestFirstSearch(vector<vector<int>>& graph, Node start, Node goal) { int rows = graph.size(); int cols = graph[0].size(); // Priority queue for nodes to be explored priority_queue<Node, vector<Node>, NodeCompare> pq; // Visited nodes set unordered_set<int> visited; // Add the start node to the priority queue pq.push(start); while (!pq.empty()) { // Get the node with the lowest cost Node current = pq.top(); pq.pop(); // Check if the current node is the goal node if (current.x == goal.x && current.y == goal.y) { cout << "Goal node reached!" << endl; return; } // Mark the current node as visited int nodeId = current.x * cols + current.y; visited.insert(nodeId); // Explore the neighboring nodes int dx[] = {-1, 1, 0, 0}; // Possible x-direction movements int dy[] = {0, 0, -1, 1}; // Possible y-direction movements for (int i = 0; i < 4; i++) { int newX = current.x + dx[i]; int newY = current.y + dy[i]; // Check if the neighboring node is within the graph boundaries if (newX >= 0 && newX < rows && newY >= 0 && newY < cols) { // Calculate the heuristic value for the neighboring node int heuristicValue = manhattanDistance(newX, newY, goal.x, goal.y); // Check if the neighboring node has not been visited if (visited.find(newX * cols + newY) == visited.end()) { // Create a new node for the neighboring position Node neighbor; neighbor.x = newX; neighbor.y = newY; neighbor.cost = current.cost + graph[newX][newY]; // Add the neighboring node to the priority queue pq.push(neighbor); } } } } cout << "Goal node not reachable!" << endl; } int main() { // Example graph represented as a 2D vector vector<vector<int>> graph = { {3, 5, 1, 2}, {1, 3, 2, 4}, {5, 2, 6, 7}, {4, 3, 1, 2} }; Node start; start.x = 0; // Starting x-coordinate start.y = 0; // Starting y-coordinate start.cost = 0; // Cost to reach the starting node Node goal; goal.x = 3; // Goal x-coordinate goal.y = 3; // Goal y-coordinate // Run Greedy Best-First Search algorithm greedyBestFirstSearch(graph, start, goal); return 0; }
Output
Goal node reached!
illustrate
This code follows a similar structure to Method 1, but uses a custom comparator, CompareManhattanDistance, which uses the Manhattan distance formula to compare nodes based on their estimated distance to the target node.
greedyBestFirstSearch function implements the greedy best first search algorithm using the Manhattan distance heuristic.
The main function demonstrates the use of the algorithm, creates a graph, defines the starting node and target node, and calls the greedyBestFirstSearch function.
in conclusion
In this article, we explore the greedy best-first search algorithm and its implementation in C. By employing these methods, programmers can efficiently find paths in graphs and solve optimization problems. The choice of heuristic function, such as Euclidean distance or Manhattan distance, can significantly affect the performance of the algorithm in different scenarios.
The above is the detailed content of Implementation of Greedy Best-First Search Algorithm in C++. For more information, please follow other related articles on the PHP Chinese website!
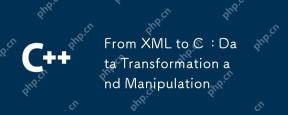
Converting from XML to C and performing data operations can be achieved through the following steps: 1) parsing XML files using tinyxml2 library, 2) mapping data into C's data structure, 3) using C standard library such as std::vector for data operations. Through these steps, data converted from XML can be processed and manipulated efficiently.
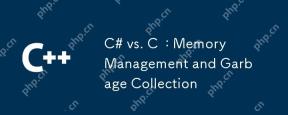
C# uses automatic garbage collection mechanism, while C uses manual memory management. 1. C#'s garbage collector automatically manages memory to reduce the risk of memory leakage, but may lead to performance degradation. 2.C provides flexible memory control, suitable for applications that require fine management, but should be handled with caution to avoid memory leakage.

C still has important relevance in modern programming. 1) High performance and direct hardware operation capabilities make it the first choice in the fields of game development, embedded systems and high-performance computing. 2) Rich programming paradigms and modern features such as smart pointers and template programming enhance its flexibility and efficiency. Although the learning curve is steep, its powerful capabilities make it still important in today's programming ecosystem.
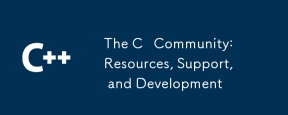
C Learners and developers can get resources and support from StackOverflow, Reddit's r/cpp community, Coursera and edX courses, open source projects on GitHub, professional consulting services, and CppCon. 1. StackOverflow provides answers to technical questions; 2. Reddit's r/cpp community shares the latest news; 3. Coursera and edX provide formal C courses; 4. Open source projects on GitHub such as LLVM and Boost improve skills; 5. Professional consulting services such as JetBrains and Perforce provide technical support; 6. CppCon and other conferences help careers

C# is suitable for projects that require high development efficiency and cross-platform support, while C is suitable for applications that require high performance and underlying control. 1) C# simplifies development, provides garbage collection and rich class libraries, suitable for enterprise-level applications. 2)C allows direct memory operation, suitable for game development and high-performance computing.
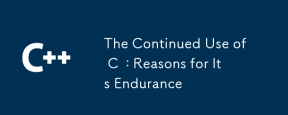
C Reasons for continuous use include its high performance, wide application and evolving characteristics. 1) High-efficiency performance: C performs excellently in system programming and high-performance computing by directly manipulating memory and hardware. 2) Widely used: shine in the fields of game development, embedded systems, etc. 3) Continuous evolution: Since its release in 1983, C has continued to add new features to maintain its competitiveness.
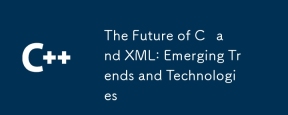
The future development trends of C and XML are: 1) C will introduce new features such as modules, concepts and coroutines through the C 20 and C 23 standards to improve programming efficiency and security; 2) XML will continue to occupy an important position in data exchange and configuration files, but will face the challenges of JSON and YAML, and will develop in a more concise and easy-to-parse direction, such as the improvements of XMLSchema1.1 and XPath3.1.
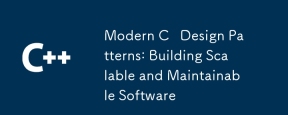
The modern C design model uses new features of C 11 and beyond to help build more flexible and efficient software. 1) Use lambda expressions and std::function to simplify observer pattern. 2) Optimize performance through mobile semantics and perfect forwarding. 3) Intelligent pointers ensure type safety and resource management.


Hot AI Tools

Undresser.AI Undress
AI-powered app for creating realistic nude photos

AI Clothes Remover
Online AI tool for removing clothes from photos.

Undress AI Tool
Undress images for free

Clothoff.io
AI clothes remover

AI Hentai Generator
Generate AI Hentai for free.

Hot Article

Hot Tools

mPDF
mPDF is a PHP library that can generate PDF files from UTF-8 encoded HTML. The original author, Ian Back, wrote mPDF to output PDF files "on the fly" from his website and handle different languages. It is slower than original scripts like HTML2FPDF and produces larger files when using Unicode fonts, but supports CSS styles etc. and has a lot of enhancements. Supports almost all languages, including RTL (Arabic and Hebrew) and CJK (Chinese, Japanese and Korean). Supports nested block-level elements (such as P, DIV),
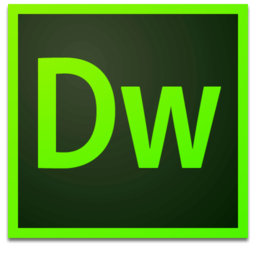
Dreamweaver Mac version
Visual web development tools

Safe Exam Browser
Safe Exam Browser is a secure browser environment for taking online exams securely. This software turns any computer into a secure workstation. It controls access to any utility and prevents students from using unauthorized resources.

SublimeText3 Chinese version
Chinese version, very easy to use

PhpStorm Mac version
The latest (2018.2.1) professional PHP integrated development tool