


Interspeech 2023 | Volcano Engine Streaming Audio Technology Speech Enhancement and AI Audio Coding
Background introduction
In order to deal with various complex audio and video communication scenarios, such as multi-device, multi-person, and multi-noise scenarios, streaming media communication technology has gradually become a part of people’s lives. indispensable technology. In order to achieve a better subjective experience and enable users to hear clearly and truly, the streaming audio technology solution combines traditional machine learning and AI-based voice enhancement solutions, using deep neural network technology solutions to achieve voice noise reduction and echo cancellation. , interfering voice elimination and audio encoding and decoding, etc., to protect the audio quality in real-time communication.
As the flagship international conference in the field of speech signal processing research, Interspeech has always represented the most cutting-edge research direction in the field of acoustics. Interspeech 2023 includes a number of articles related to audio signal speech enhancement algorithms, among which, ## A total of 4 research papers from the #volcanoengine streaming audio team were accepted by the conference, including speech enhancement, AI-based encoding and decoding , echo cancellation, and unsupervised adaptive speech enhancement.
It is worth mentioning that in the field of unsupervised adaptive speech enhancement, the joint team of ByteDance and NPU successfully completed the subtask of unsupervised domain adaptive conversational speech in this year’s CHiME (Computational Hearing in Multisource Environments) Challenge. Enhancement (Unsupervised domain adaptation for conversational speech enhancement, UDASE) won the championship (https://www.chimechallenge.org/current/task2/results). The CHiME Challenge is an important international competition launched in 2011 by well-known research institutions such as the French Institute of Computer Science and Automation, the University of Sheffield in the UK, and the Mitsubishi Electronics Research Laboratory in the United States. It focuses on challenging remote problems in the field of speech research. This year, it has been held for the seventh time. Participating teams in previous CHiME competitions include the University of Cambridge in the United Kingdom, Carnegie Mellon University in the United States, Johns Hopkins University, NTT in Japan, Hitachi Academia Sinica and other internationally renowned universities and research institutions, as well as Tsinghua University, University of Chinese Academy of Sciences, Chinese Academy of Sciences Institute of Acoustics, NPU, iFlytek and other top domestic universities and research institutes. This article will introduce the core scenario problems and technical solutions solved by these four papers,share the Volcano Engine streaming audio team’s progress in speech enhancement, based on AI encoder, echo cancellation and unsupervised adaptive speech enhancement Thinking and practice in the field.
Lightweight speech harmonic enhancement method based on learnable comb filterPaper address: https://www.isca-speech.org/archive/interspeech_2023/ le23_interspeech.htmlBackgroundLimited by delay and computing resources, speech enhancement in real-time audio and video communication scenarios usually uses input features based on filter banks. Through filter banks such as Mel and ERB, the original spectrum is compressed into lower-dimensional sub-bands. In the sub-band domain, the output of the deep learning-based speech enhancement model is the speech gain of the sub-band, which represents the proportion of the target speech energy. However, the enhanced audio over the compressed sub-band domain is blurry due to loss of spectral detail, often requiring post-processing to enhance harmonics. RNNoise and PercepNet use comb filters to enhance harmonics, but due to fundamental frequency estimation and comb filter gain calculation and model decoupling, they cannot be optimized end-to-end; DeepFilterNet uses a time-frequency domain filter to suppress inter-harmonic noise , but does not explicitly utilize the fundamental frequency information of speech. In response to the above problems, the team proposed a speech harmonic enhancement method based on a learnable comb filter. This method combines fundamental frequency estimation and comb filtering, and the gain of the comb filter can be optimized end-to-end. Experiments show that this method can achieve better harmonic enhancement with a similar amount of calculation as existing methods. Model framework structureFundamental frequency estimator (F0 Estimator)In order to reduce the difficulty of fundamental frequency estimation and enable the entire link to run end-to-end, the to-be-estimated The target fundamental frequency range is discretized into N discrete fundamental frequencies and estimated using a classifier. 1 dimension is added to represent non-voiced frames, and the final model output is N 1-dimensional probabilities. Consistent with CREPE, the team uses Gaussian smooth features as the training target and Binary Cross Entropy as the loss function:
Use a two-dimensional convolution layer (Conv2D) to simultaneously calculate the filtering results of all discrete fundamental frequencies during training. The weight of the two-dimensional convolution can be expressed as the matrix in the figure below. The matrix has N 1 dimensions, and each dimension is Initialize using the above filter:
The filter result corresponding to the fundamental frequency of each frame is obtained by multiplying the one-hot label of the target fundamental frequency and the output of the two-dimensional convolution:
The harmonic enhanced audio will be weighted and added to the original audio, and multiplied by the sub-band gain to get the final output:
During inference, each frame only needs to calculate the filtering result of one fundamental frequency, so the calculation cost of this method is low.
Model structure
The team uses Dual-Path Convolutional Recurrent Network (DPCRN) as the backbone of the speech enhancement model, and adds fundamental frequency estimator. The Encoder and Decoder use depth-separable convolution to form a symmetric structure. The Decoder has two parallel branches that output the sub-band gain G and the weighting coefficient R respectively. The input to the fundamental frequency estimator is the output of the DPRNN module and the linear spectrum. The calculation amount of this model is about 300 M MACs, of which the comb filtering calculation amount is about 0.53M MACs.
Model training
In the experiment, the VCTK-DEMAND and DNS4 challenge datasets were used for training, and the loss functions of speech enhancement and fundamental frequency estimation were used for multi-task learning.
Experimental results
The streaming audio team combined the proposed learnable comb filtering model with comb filtering using PercepNet and DeepFilterNet. The filtering algorithm models are compared, which are called DPCRN-CF, DPCRN-PN and DPCRN-DF respectively. On the VCTK test set, the method proposed in this article shows advantages over existing methods.
At the same time, the team conducted ablation experiments on fundamental frequency estimation and learnable filters. Experimental results show that end-to-end learning produces better results than using signal processing-based fundamental frequency estimation algorithms and filter weights.
End-to-end neural network audio encoder based on Intra-BRNN and GB-RVQ
Paper address: https://www.isca-speech .org/archive/pdfs/interspeech_2023/xu23_interspeech.pdf
Background
In recent years, many neural network models have been used for low-bitrate speech coding tasks, however some end-to-end models failed Make full use of intra-frame related information, and the introduced quantizer has a large quantization error, resulting in low audio quality after encoding. In order to improve the quality of the end-to-end neural network audio encoder, the streaming audio team proposed an end-to-end neural speech codec, namely CBRC (Convolutional and Bidirectional Recurrent neural Codec). CBRC uses an interleaved structure of 1D-CNN (one-dimensional convolution) and Intra-BRNN (intra-frame bidirectional recurrent neural network) to more effectively utilize intra-frame correlation. In addition, the team uses the Group-wise and Beam-search Residual Vector Quantizer (GB-RVQ) in CBRC to reduce quantization noise. CBRC encodes 16kHz audio with a 20ms frame length, without additional system delay, and is suitable for real-time communication scenarios. Experimental results show that the voice quality of CBRC encoding with a bit rate of 3kbps is better than that of Opus with 12kbps.
Model framework structure
CBRC overall structure
Encoder and Decoder network structure
Encoder uses 4 cascaded CBRNBlocks to extract audio features. Each CBRNBlock consists of three ResidualUnits for extracting features and a one-dimensional convolution that controls the downsampling rate. Each time the features in the Encoder are downsampled, the number of feature channels is doubled. ResidualUnit is composed of a residual convolution module and a residual bidirectional recurrent network, in which the convolution layer uses causal convolution, while the bidirectional GRU structure in Intra-BRNN only processes 20ms intra-frame audio features. The Decoder network is the mirror structure of the Encoder, using one-dimensional transposed convolution for upsampling. The interleaved structure of 1D-CNN and Intra-BRNN enables the Encoder and Decoder to make full use of the 20ms audio intra-frame correlation without introducing additional delay.
CBRNBlock structure
Group and beam search residual vector quantizer GB-RVQ
CBRC uses residual The Residual Vector Quantizer (RVQ) quantizes and compresses the output features of the encoding network to a specified bit rate. RVQ uses a multi-layer vector quantizer (VQ) cascade to compress features. Each layer of VQ quantizes the quantization residual of the previous layer of VQ, which can significantly reduce the amount of codebook parameters of a single layer of VQ at the same bit rate. The team proposed two better quantizer structures in CBRC, namely group-wise RVQ and beam-search residual vector quantizer (Beam-search RVQ).
Group Residual Vector Quantizer Group-wise RVQ |
Beam-search residual vector quantizer Beam-search RVQ |
|
|
|
#Beam-search RVQ algorithm brief process: |
##Objective score |
Subjective listening experience Score |
The above is the detailed content of Interspeech 2023 | Volcano Engine Streaming Audio Technology Speech Enhancement and AI Audio Coding. For more information, please follow other related articles on the PHP Chinese website!
![[Ghibli-style images with AI] Introducing how to create free images with ChatGPT and copyright](https://img.php.cn/upload/article/001/242/473/174707263295098.jpg?x-oss-process=image/resize,p_40)
The latest model GPT-4o released by OpenAI not only can generate text, but also has image generation functions, which has attracted widespread attention. The most eye-catching feature is the generation of "Ghibli-style illustrations". Simply upload the photo to ChatGPT and give simple instructions to generate a dreamy image like a work in Studio Ghibli. This article will explain in detail the actual operation process, the effect experience, as well as the errors and copyright issues that need to be paid attention to. For details of the latest model "o3" released by OpenAI, please click here⬇️ Detailed explanation of OpenAI o3 (ChatGPT o3): Features, pricing system and o4-mini introduction Please click here for the English version of Ghibli-style article⬇️ Create Ji with ChatGPT
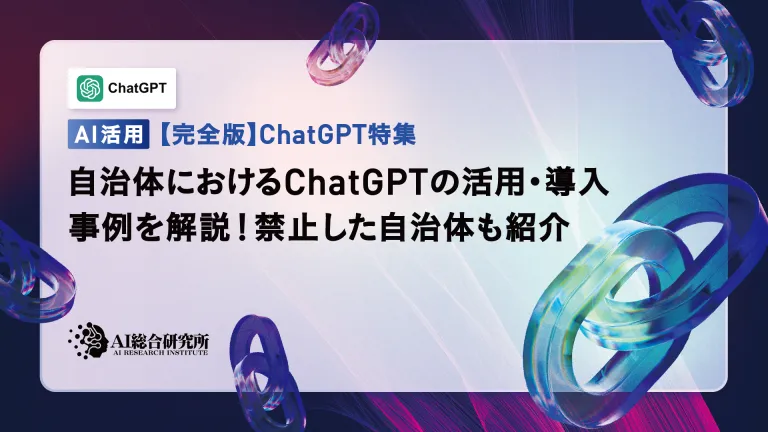
As a new communication method, the use and introduction of ChatGPT in local governments is attracting attention. While this trend is progressing in a wide range of areas, some local governments have declined to use ChatGPT. In this article, we will introduce examples of ChatGPT implementation in local governments. We will explore how we are achieving quality and efficiency improvements in local government services through a variety of reform examples, including supporting document creation and dialogue with citizens. Not only local government officials who aim to reduce staff workload and improve convenience for citizens, but also all interested in advanced use cases.
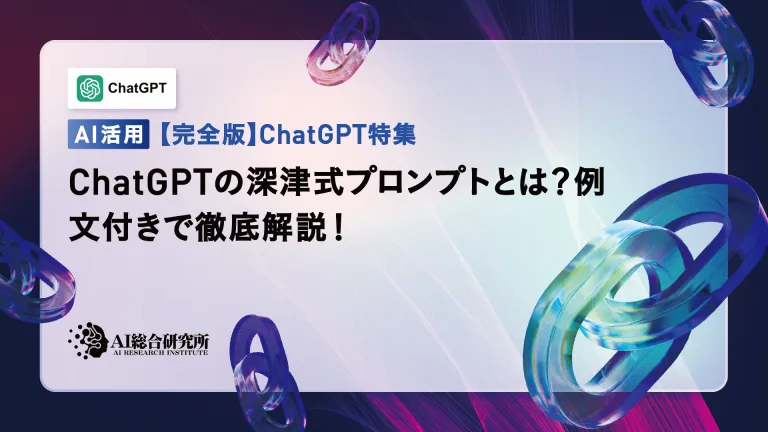
Have you heard of a framework called the "Fukatsu Prompt System"? Language models such as ChatGPT are extremely excellent, but appropriate prompts are essential to maximize their potential. Fukatsu prompts are one of the most popular prompt techniques designed to improve output accuracy. This article explains the principles and characteristics of Fukatsu-style prompts, including specific usage methods and examples. Furthermore, we have introduced other well-known prompt templates and useful techniques for prompt design, so based on these, we will introduce C.
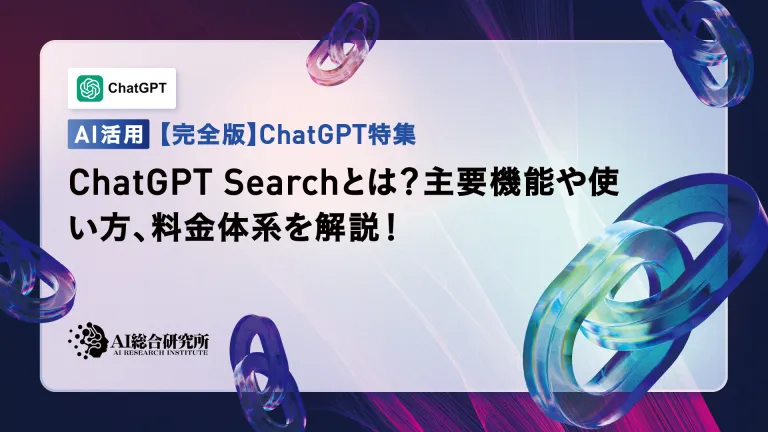
ChatGPT Search: Get the latest information efficiently with an innovative AI search engine! In this article, we will thoroughly explain the new ChatGPT feature "ChatGPT Search," provided by OpenAI. Let's take a closer look at the features, usage, and how this tool can help you improve your information collection efficiency with reliable answers based on real-time web information and intuitive ease of use. ChatGPT Search provides a conversational interactive search experience that answers user questions in a comfortable, hidden environment that hides advertisements
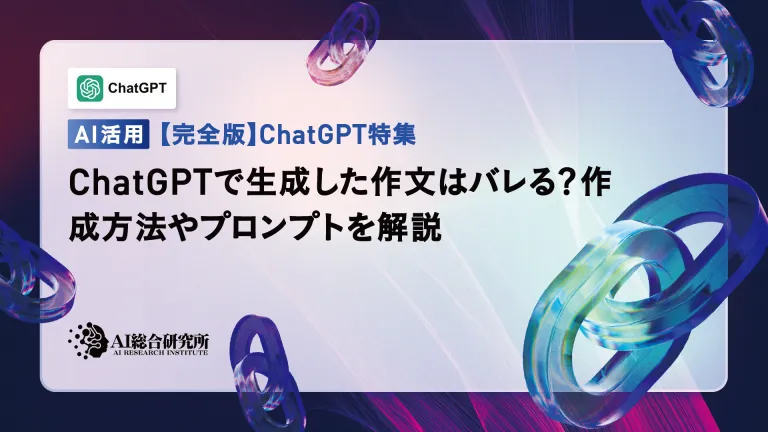
In a modern society with information explosion, it is not easy to create compelling articles. How to use creativity to write articles that attract readers within a limited time and energy requires superb skills and rich experience. At this time, as a revolutionary writing aid, ChatGPT attracted much attention. ChatGPT uses huge data to train language generation models to generate natural, smooth and refined articles. This article will introduce how to effectively use ChatGPT and efficiently create high-quality articles. We will gradually explain the writing process of using ChatGPT, and combine specific cases to elaborate on its advantages and disadvantages, applicable scenarios, and safe use precautions. ChatGPT will be a writer to overcome various obstacles,
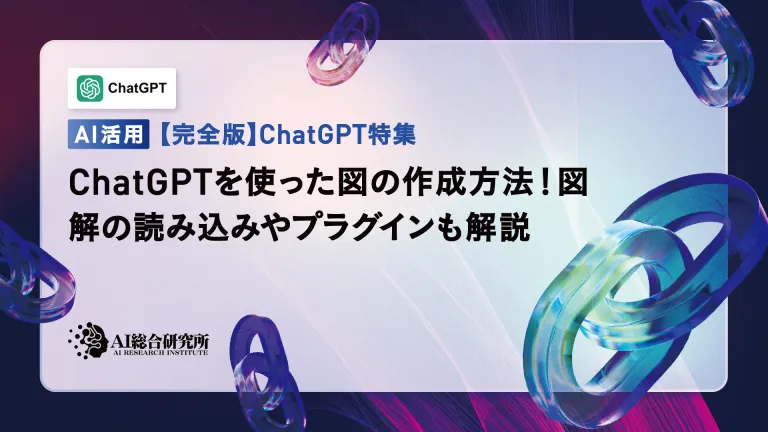
An efficient guide to creating charts using AI Visual materials are essential to effectively conveying information, but creating it takes a lot of time and effort. However, the chart creation process is changing dramatically due to the rise of AI technologies such as ChatGPT and DALL-E 3. This article provides detailed explanations on efficient and attractive diagram creation methods using these cutting-edge tools. It covers everything from ideas to completion, and includes a wealth of information useful for creating diagrams, from specific steps, tips, plugins and APIs that can be used, and how to use the image generation AI "DALL-E 3."
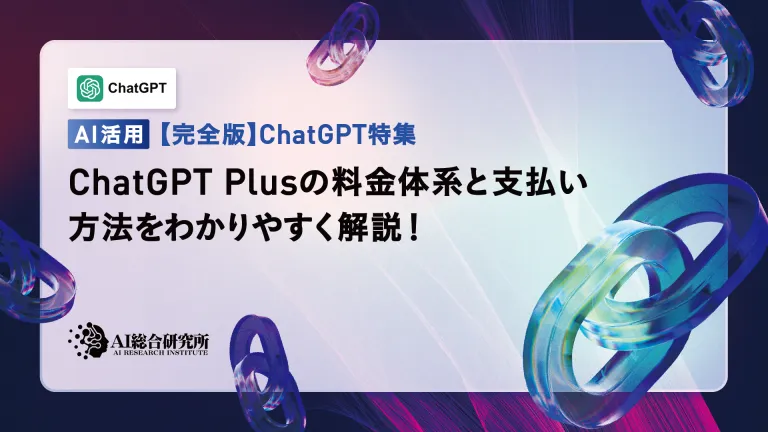
Unlock ChatGPT Plus: Fees, Payment Methods and Upgrade Guide ChatGPT, a world-renowned generative AI, has been widely used in daily life and business fields. Although ChatGPT is basically free, the paid version of ChatGPT Plus provides a variety of value-added services, such as plug-ins, image recognition, etc., which significantly improves work efficiency. This article will explain in detail the charging standards, payment methods and upgrade processes of ChatGPT Plus. For details of OpenAI's latest image generation technology "GPT-4o image generation" please click: Detailed explanation of GPT-4o image generation: usage methods, prompt word examples, commercial applications and differences from other AIs Table of contents ChatGPT Plus Fees Ch
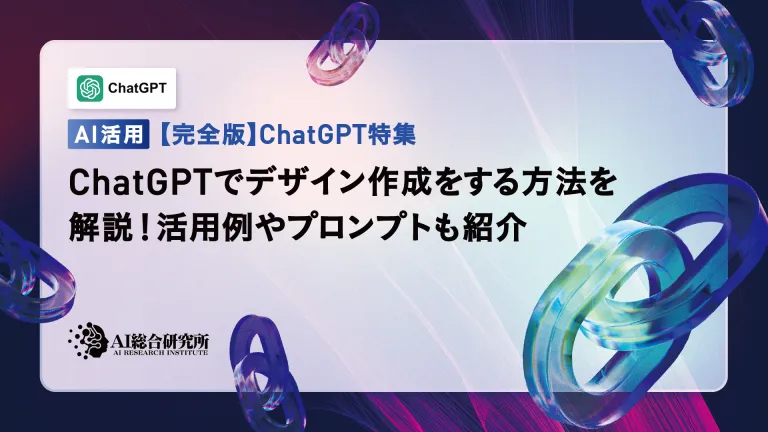
How to use ChatGPT to streamline your design work and increase creativity This article will explain in detail how to create a design using ChatGPT. We will introduce examples of using ChatGPT in various design fields, such as ideas, text generation, and web design. We will also introduce points that will help you improve the efficiency and quality of a variety of creative work, such as graphic design, illustration, and logo design. Please take a look at how AI can greatly expand your design possibilities. table of contents ChatGPT: A powerful tool for design creation


Hot AI Tools

Undresser.AI Undress
AI-powered app for creating realistic nude photos

AI Clothes Remover
Online AI tool for removing clothes from photos.

Undress AI Tool
Undress images for free

Clothoff.io
AI clothes remover

Video Face Swap
Swap faces in any video effortlessly with our completely free AI face swap tool!

Hot Article

Hot Tools
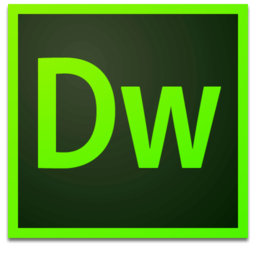
Dreamweaver Mac version
Visual web development tools
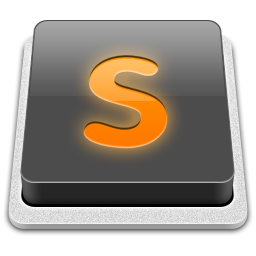
SublimeText3 Mac version
God-level code editing software (SublimeText3)
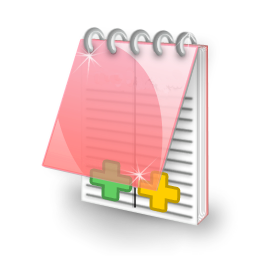
EditPlus Chinese cracked version
Small size, syntax highlighting, does not support code prompt function

MinGW - Minimalist GNU for Windows
This project is in the process of being migrated to osdn.net/projects/mingw, you can continue to follow us there. MinGW: A native Windows port of the GNU Compiler Collection (GCC), freely distributable import libraries and header files for building native Windows applications; includes extensions to the MSVC runtime to support C99 functionality. All MinGW software can run on 64-bit Windows platforms.

SecLists
SecLists is the ultimate security tester's companion. It is a collection of various types of lists that are frequently used during security assessments, all in one place. SecLists helps make security testing more efficient and productive by conveniently providing all the lists a security tester might need. List types include usernames, passwords, URLs, fuzzing payloads, sensitive data patterns, web shells, and more. The tester can simply pull this repository onto a new test machine and he will have access to every type of list he needs.
