


This article will introduce a method to accurately generate multi-view street view images through BEV Sketch layout
In the field of autonomous driving, image synthesis is widely used to improve downstream perception Task performance
In the field of computer vision, a long-standing research problem in improving the performance of perceptual models is to achieve it by synthesizing images. In vision-centric autonomous driving systems, using multi-view cameras, this problem becomes more prominent because some long-tail scenes can never be collected.
According to As shown in Figure 1(a), the existing generation method inputs the semantic segmentation-style BEV structure into the generation network and outputs reasonable multi-view images. When evaluated solely on scene-level metrics, existing methods appear to be capable of synthesizing photorealistic street view images. Once zoomed in, however, we found that it failed to produce accurate object-level detail. In the figure, we demonstrate a common mistake of state-of-the-art generation algorithms, which is that the generated vehicle is completely oriented in the opposite direction compared to the target 3D bounding box. Furthermore, editing semantic segmentation-style BEV structures is a difficult task that requires a lot of manpower. Therefore, we propose a two-stage method called BEVControl for providing more refined background and foreground geometries. control, as shown in Figure 1(b). BEVControl supports sketch-style BEV structure input, allowing for quick and easy editing. Additionally, our BEVControl decomposes visual consistency into two sub-goals: geometric consistency between street views and bird's-eye views via the Controller; appearance consistency between street views via the Coordinator
##Paper link:
BEVControl is a generated network of UNet structure, consisting of a series of modules. Each module has two elements, namely Controller and Coordinator.
- Input: BEV sketch, multi-view noise image and text prompt for easy editing;
- Output: generated multi-view image.
- Method details
Camera projection process of BEV sketch to camera condition. Input is a BEV sketch. The output is multi-view foreground conditions and background conditions.
Controller: Receives the foreground and background information of the camera view sketch in a self-attentional manner, and outputs geometric consistency with the BEV sketch Streetscape features.
- Coordinator: Utilizes a novel cross-view and cross-element attention mechanism to achieve cross-view contextual interaction and output street view features with appearance consistency.
- Proposed evaluation metrics
Recent street view image generation work only evaluates generation based on scene-level metrics (such as FID, road mIoU, etc.) quality.
- We found that it is impossible to evaluate the true generative ability of the generative network using only these metrics, as shown in the figure below. The reported qualitative and quantitative results show that both groups generate Street View images with similar FID scores but very different capabilities for fine-grained control over the foreground and background.
- Therefore, we propose a set of evaluation indicators for finely measuring the control capabilities of the generated network.
Quantitative results
Comparison of BEVControl and state-of-the-art methods on the proposed evaluation indicators.
- Apply BEVControl for data enhancement to improve target detection tasks.
Qualitative results
- Comparison of BEVControl and state-of-the-art methods on the NuScenes validation set.
##Demo effect
The above is the detailed content of More granular background and foreground control, faster editing: BEVControl's two-stage approach. For more information, please follow other related articles on the PHP Chinese website!
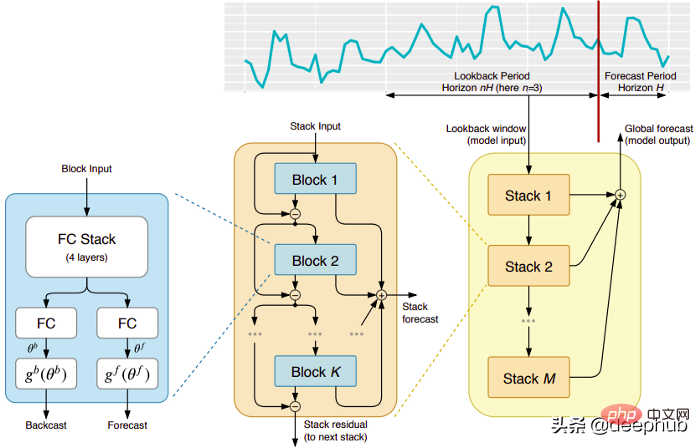
MakridakisM-Competitions系列(分别称为M4和M5)分别在2018年和2020年举办(M6也在今年举办了)。对于那些不了解的人来说,m系列得比赛可以被认为是时间序列生态系统的一种现有状态的总结,为当前得预测的理论和实践提供了经验和客观的证据。2018年M4的结果表明,纯粹的“ML”方法在很大程度上胜过传统的统计方法,这在当时是出乎意料的。在两年后的M5[1]中,最的高分是仅具有“ML”方法。并且所有前50名基本上都是基于ML的(大部分是树型模型)。这场比赛看到了LightG
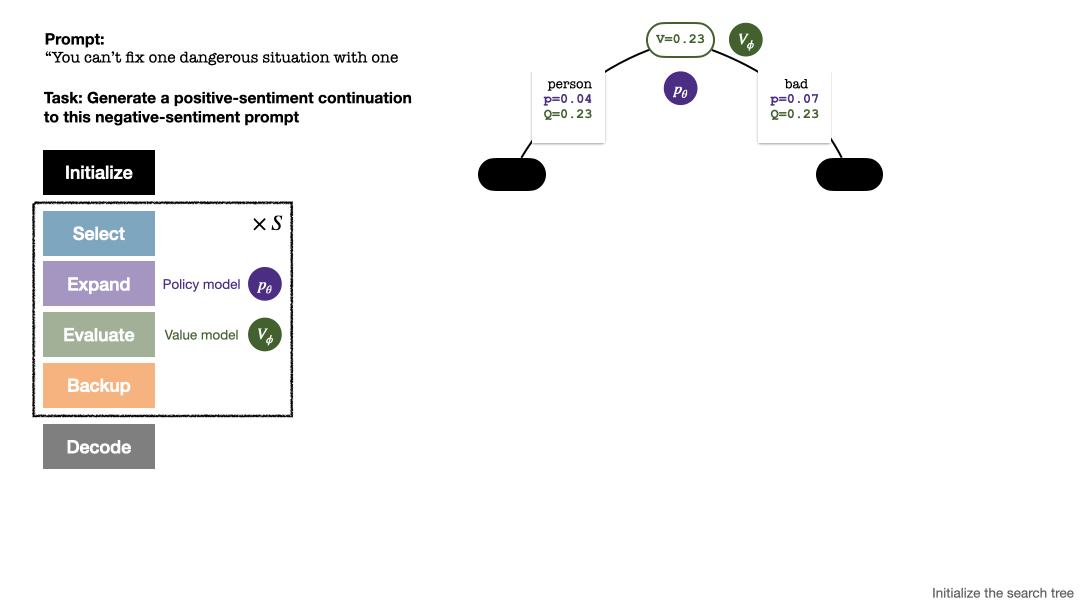
在一项最新的研究中,来自UW和Meta的研究者提出了一种新的解码算法,将AlphaGo采用的蒙特卡洛树搜索算法(Monte-CarloTreeSearch,MCTS)应用到经过近端策略优化(ProximalPolicyOptimization,PPO)训练的RLHF语言模型上,大幅提高了模型生成文本的质量。PPO-MCTS算法通过探索与评估若干条候选序列,搜索到更优的解码策略。通过PPO-MCTS生成的文本能更好满足任务要求。论文链接:https://arxiv.org/pdf/2309.150
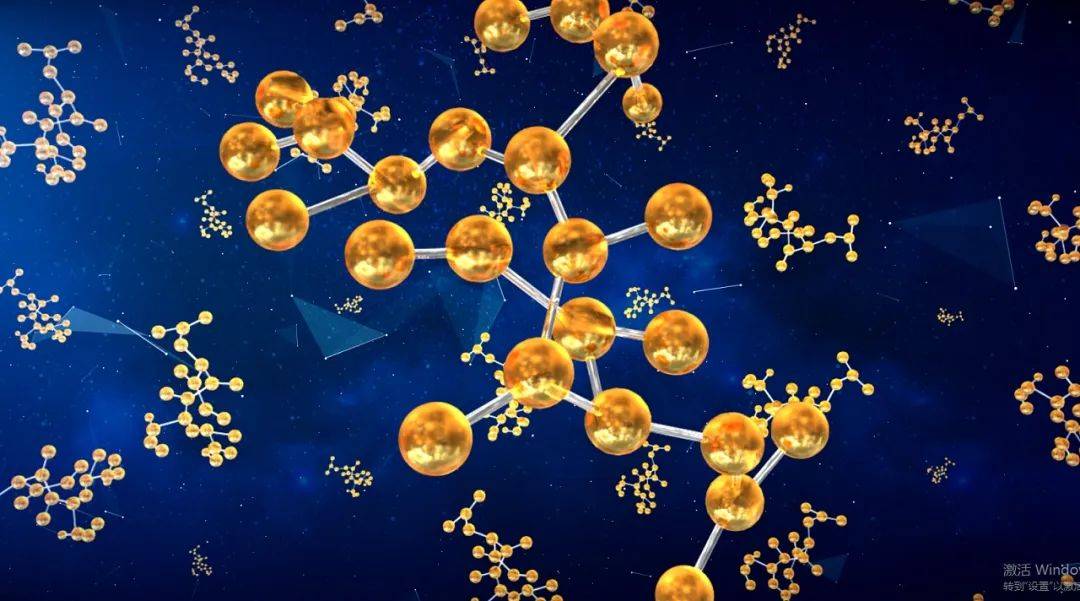
编辑|X传统意义上,发现所需特性的分子过程一直是由手动实验、化学家的直觉以及对机制和第一原理的理解推动的。随着化学家越来越多地使用自动化设备和预测合成算法,自主研究设备越来越接近实现。近日,来自MIT的研究人员开发了由集成机器学习工具驱动的闭环自主分子发现平台,以加速具有所需特性的分子的设计。无需手动实验即可探索化学空间并利用已知的化学结构。在两个案例研究中,该平台尝试了3000多个反应,其中1000多个产生了预测的反应产物,提出、合成并表征了303种未报道的染料样分子。该研究以《Autonom
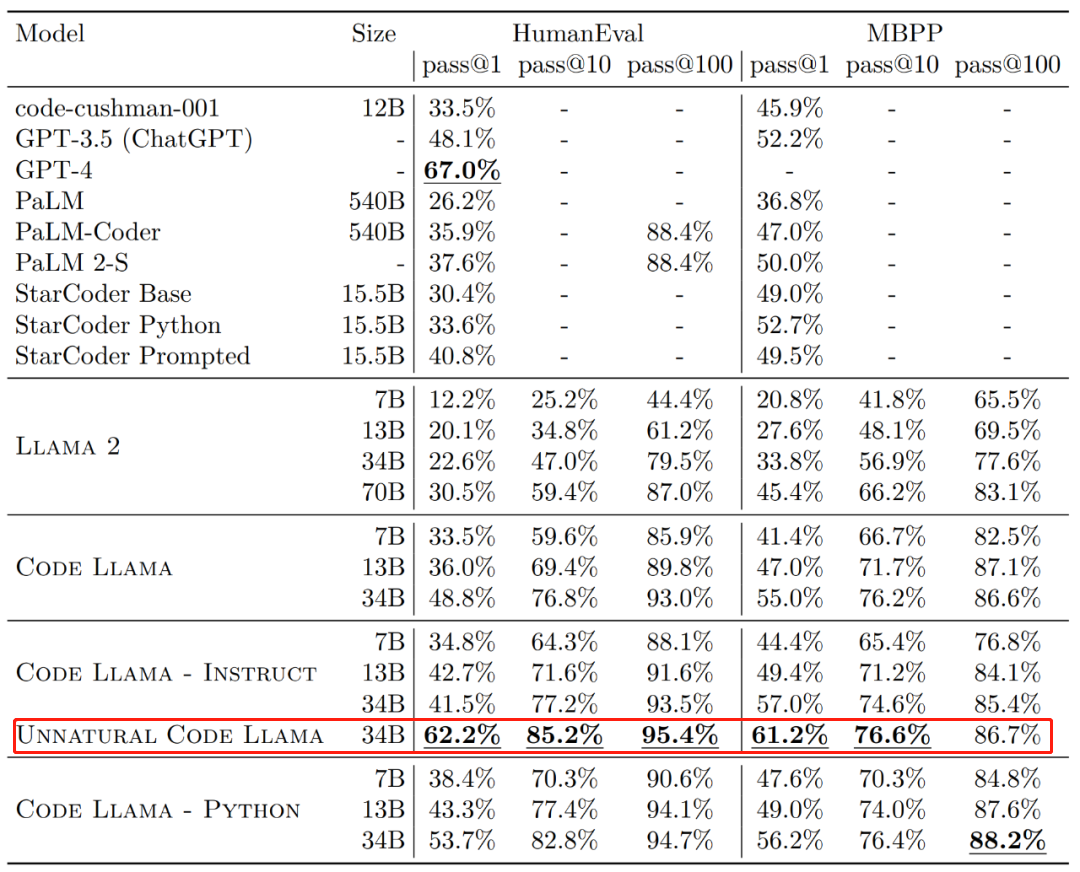
昨天,Meta开源专攻代码生成的基础模型CodeLlama,可免费用于研究以及商用目的。CodeLlama系列模型有三个参数版本,参数量分别为7B、13B和34B。并且支持多种编程语言,包括Python、C++、Java、PHP、Typescript(Javascript)、C#和Bash。Meta提供的CodeLlama版本包括:代码Llama,基础代码模型;代码羊-Python,Python微调版本;代码Llama-Instruct,自然语言指令微调版就其效果来说,CodeLlama的不同版
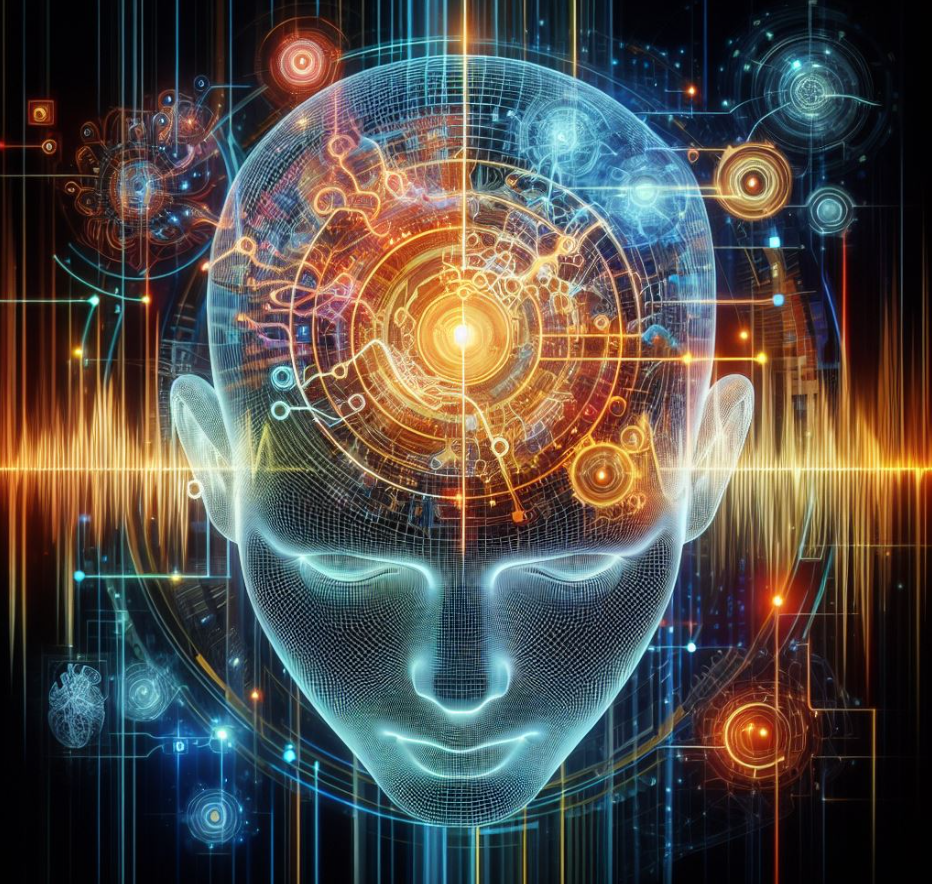
作者|陈旭鹏编辑|ScienceAI由于神经系统的缺陷导致的失语会导致严重的生活障碍,它可能会限制人们的职业和社交生活。近年来,深度学习和脑机接口(BCI)技术的飞速发展为开发能够帮助失语者沟通的神经语音假肢提供了可行性。然而,神经信号的语音解码面临挑战。近日,约旦大学VideoLab和FlinkerLab的研究者开发了一个新型的可微分语音合成器,可以利用一个轻型的卷积神经网络将语音编码为一系列可解释的语音参数(例如音高、响度、共振峰频率等),并通过可微分神经网络将这些参数合成为语音。这个合成器
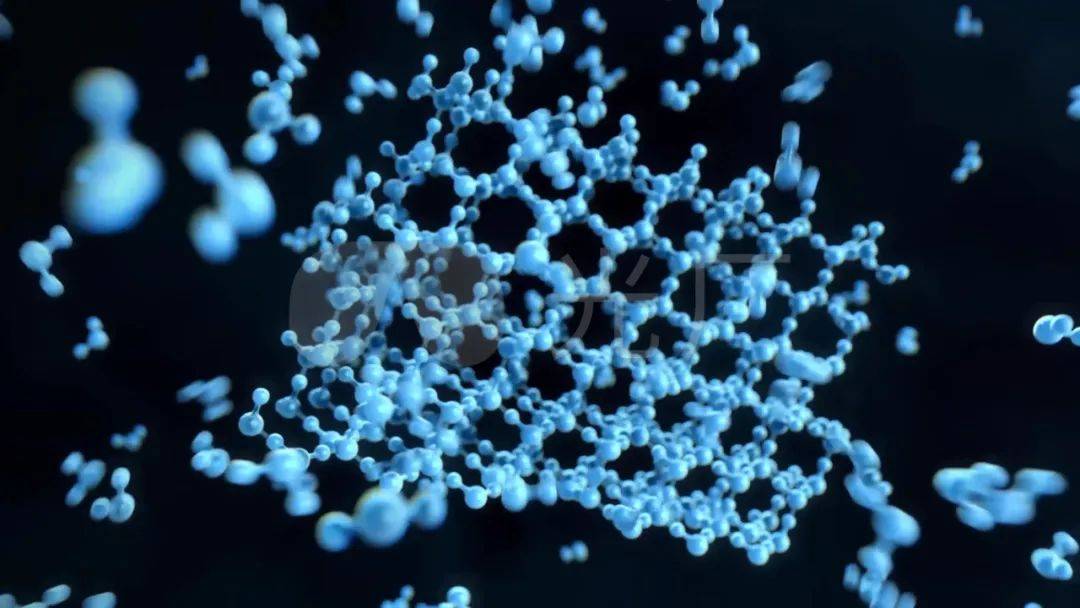
编辑|紫罗可合成分子的化学空间是非常广阔的。有效地探索这个领域需要依赖计算筛选技术,比如深度学习,以便快速地发现各种有趣的化合物。将分子结构转换为数字表示形式,并开发相应算法生成新的分子结构是进行化学发现的关键。最近,英国格拉斯哥大学的研究团队提出了一种基于电子密度训练的机器学习模型,用于生成主客体binders。这种模型能够以简化分子线性输入规范(SMILES)格式读取数据,准确率高达98%,从而实现对分子在二维空间的全面描述。通过变分自编码器生成主客体系统的电子密度和静电势的三维表示,然后通
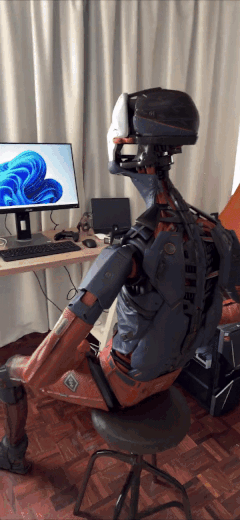
一个普通人用一台手机就能制作电影特效的时代已经来了。最近,一个名叫Simulon的3D技术公司发布了一系列特效视频,视频中的3D机器人与环境无缝融合,而且光影效果非常自然。呈现这些效果的APP也叫Simulon,它能让使用者通过手机摄像头的实时拍摄,直接渲染出CGI(计算机生成图像)特效,就跟打开美颜相机拍摄一样。在具体操作中,你要先上传一个3D模型(比如图中的机器人)。Simulon会将这个模型放置到你拍摄的现实世界中,并使用准确的照明、阴影和反射效果来渲染它们。整个过程不需要相机解算、HDR
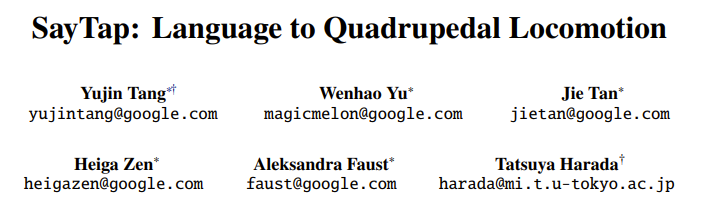
人类和四足机器人之间简单有效的交互是创造能干的智能助理机器人的途径,其昭示着这样一个未来:技术以超乎我们想象的方式改善我们的生活。对于这样的人类-机器人交互系统,关键是让四足机器人有能力响应自然语言指令。近来大型语言模型(LLM)发展迅速,已经展现出了执行高层规划的潜力。然而,对LLM来说,理解低层指令依然很难,比如关节角度目标或电机扭矩,尤其是对于本身就不稳定、必需高频控制信号的足式机器人。因此,大多数现有工作都会假设已为LLM提供了决定机器人行为的高层API,而这就从根本上限制了系统的表现能


Hot AI Tools

Undresser.AI Undress
AI-powered app for creating realistic nude photos

AI Clothes Remover
Online AI tool for removing clothes from photos.

Undress AI Tool
Undress images for free

Clothoff.io
AI clothes remover

AI Hentai Generator
Generate AI Hentai for free.

Hot Article

Hot Tools

SAP NetWeaver Server Adapter for Eclipse
Integrate Eclipse with SAP NetWeaver application server.

MinGW - Minimalist GNU for Windows
This project is in the process of being migrated to osdn.net/projects/mingw, you can continue to follow us there. MinGW: A native Windows port of the GNU Compiler Collection (GCC), freely distributable import libraries and header files for building native Windows applications; includes extensions to the MSVC runtime to support C99 functionality. All MinGW software can run on 64-bit Windows platforms.
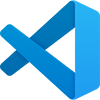
VSCode Windows 64-bit Download
A free and powerful IDE editor launched by Microsoft

MantisBT
Mantis is an easy-to-deploy web-based defect tracking tool designed to aid in product defect tracking. It requires PHP, MySQL and a web server. Check out our demo and hosting services.

mPDF
mPDF is a PHP library that can generate PDF files from UTF-8 encoded HTML. The original author, Ian Back, wrote mPDF to output PDF files "on the fly" from his website and handle different languages. It is slower than original scripts like HTML2FPDF and produces larger files when using Unicode fonts, but supports CSS styles etc. and has a lot of enhancements. Supports almost all languages, including RTL (Arabic and Hebrew) and CJK (Chinese, Japanese and Korean). Supports nested block-level elements (such as P, DIV),
