How to improve query performance in C++ big data development?
How to improve the query performance in C big data development?
In recent years, with the increasing amount of data and the continuous improvement of processing requirements, C big data development Plays an important role in various fields. However, when processing huge amounts of data, improving query performance becomes a very critical issue. In this article, we will explore some practical tips for improving query performance in C big data development and illustrate them with code examples.
1. Optimize data structure
In big data query, the selection and optimization of data structure are very important. An efficient data structure can reduce query time and improve query performance. The following are some commonly used optimization techniques:
- Use a hash table: A hash table is a fast search data structure that can achieve constant time complexity search operations. When working with large data collections, using hash tables can significantly speed up queries.
- Use index: Index is a data structure that sorts data and can speed up query operations. When processing large data collections, using indexes can reduce the number of data scans, thereby improving query performance.
- Use tree structure: Tree structure is a self-balancing data structure that can quickly locate data. When processing large data collections, using a tree structure can achieve fast range queries and maintain the orderliness of the data.
2. Reasonable use of parallel computing
In big data queries, parallel computing is an important means to improve performance. Proper use of multi-core processors and parallel programming technology can achieve parallel decomposition and parallel execution of query tasks. The following are some commonly used parallel computing techniques:
- Use multi-threading: Multi-threading is a common parallel computing technology that can perform multiple query tasks at the same time and improve query performance. In C, you can use multi-thread libraries such as std::thread or OpenMP to implement multi-thread parallel computing.
- Use a distributed computing framework: For the processing of massive data, single-machine computing may not be able to meet the needs. At this time, a distributed computing framework can be used to distribute the data on multiple machines for processing. Commonly used distributed computing frameworks include Hadoop, Spark, etc.
3. Optimizing query algorithm
In big data query, the optimization of query algorithm is very important. An efficient query algorithm can reduce unnecessary data scanning and calculations, thereby improving query performance. The following are some commonly used query algorithm optimization techniques:
- Binary search: For ordered data collections, you can use the binary search algorithm to quickly locate data. The time complexity of the binary search algorithm is O(logN), which is much lower than the complexity of linear search.
- Filtering and pruning: During the query process, data can be filtered through filter conditions to reduce unnecessary data scanning. For example, you can filter by date range, numerical range, etc. to reduce the amount of data that needs to be scanned when querying.
- Use the divide-and-conquer algorithm: The divide-and-conquer algorithm is an algorithm that decomposes a large problem into multiple small problems and solves them separately. In big data queries, the query task can be decomposed into multiple subtasks, queried separately and finally merged results, thereby reducing query time.
The following is a sample code that uses indexes to optimize queries:
#include <iostream> #include <vector> #include <algorithm> // 定义数据结构 struct Data { int id; std::string name; // 其他字段... }; // 定义索引 struct Index { int id; int index; }; // 查询函数 std::vector<Data> query(int queryId, const std::vector<Data>& data, const std::vector<Index>& index) { std::vector<Data> result; // 使用二分查找定位查询的数据 auto it = std::lower_bound(index.begin(), index.end(), queryId, [](const Index& index, int id) { return index.id < id; }); // 循环查询数据并存入结果 while (it != index.end() && it->id == queryId) { result.push_back(data[it->index]); it++; } return result; } int main() { // 构造测试数据 std::vector<Data> data = { {1, "Alice"}, {2, "Bob"}, {2, "Tom"}, // 其他数据... }; // 构造索引 std::vector<Index> index; for (int i = 0; i < data.size(); i++) { index.push_back({data[i].id, i}); } std::sort(index.begin(), index.end(), [](const Index& a, const Index& b) { return a.id < b.id; }); // 执行查询 int queryId = 2; std::vector<Data> result = query(queryId, data, index); // 输出查询结果 for (const auto& data : result) { std::cout << data.id << " " << data.name << std::endl; } return 0; }
By using indexes for queries, the number of data scans can be greatly reduced and query performance improved.
Summary: In C big data development, optimizing query performance is very important. By optimizing data structures, rationally utilizing parallel computing and optimizing query algorithms, query performance can be improved and program efficiency improved. I hope the introduction and sample code of this article will be helpful to you in improving query performance in C big data development.
The above is the detailed content of How to improve query performance in C++ big data development?. For more information, please follow other related articles on the PHP Chinese website!
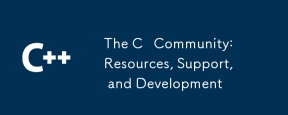
C Learners and developers can get resources and support from StackOverflow, Reddit's r/cpp community, Coursera and edX courses, open source projects on GitHub, professional consulting services, and CppCon. 1. StackOverflow provides answers to technical questions; 2. Reddit's r/cpp community shares the latest news; 3. Coursera and edX provide formal C courses; 4. Open source projects on GitHub such as LLVM and Boost improve skills; 5. Professional consulting services such as JetBrains and Perforce provide technical support; 6. CppCon and other conferences help careers

C# is suitable for projects that require high development efficiency and cross-platform support, while C is suitable for applications that require high performance and underlying control. 1) C# simplifies development, provides garbage collection and rich class libraries, suitable for enterprise-level applications. 2)C allows direct memory operation, suitable for game development and high-performance computing.
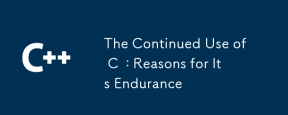
C Reasons for continuous use include its high performance, wide application and evolving characteristics. 1) High-efficiency performance: C performs excellently in system programming and high-performance computing by directly manipulating memory and hardware. 2) Widely used: shine in the fields of game development, embedded systems, etc. 3) Continuous evolution: Since its release in 1983, C has continued to add new features to maintain its competitiveness.
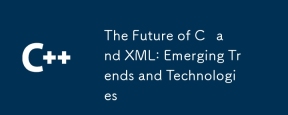
The future development trends of C and XML are: 1) C will introduce new features such as modules, concepts and coroutines through the C 20 and C 23 standards to improve programming efficiency and security; 2) XML will continue to occupy an important position in data exchange and configuration files, but will face the challenges of JSON and YAML, and will develop in a more concise and easy-to-parse direction, such as the improvements of XMLSchema1.1 and XPath3.1.
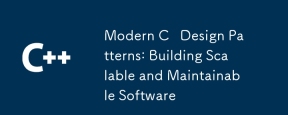
The modern C design model uses new features of C 11 and beyond to help build more flexible and efficient software. 1) Use lambda expressions and std::function to simplify observer pattern. 2) Optimize performance through mobile semantics and perfect forwarding. 3) Intelligent pointers ensure type safety and resource management.
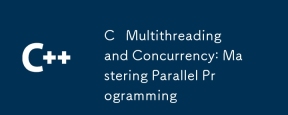
C The core concepts of multithreading and concurrent programming include thread creation and management, synchronization and mutual exclusion, conditional variables, thread pooling, asynchronous programming, common errors and debugging techniques, and performance optimization and best practices. 1) Create threads using the std::thread class. The example shows how to create and wait for the thread to complete. 2) Synchronize and mutual exclusion to use std::mutex and std::lock_guard to protect shared resources and avoid data competition. 3) Condition variables realize communication and synchronization between threads through std::condition_variable. 4) The thread pool example shows how to use the ThreadPool class to process tasks in parallel to improve efficiency. 5) Asynchronous programming uses std::as
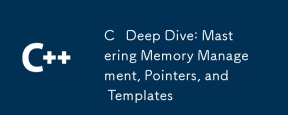
C's memory management, pointers and templates are core features. 1. Memory management manually allocates and releases memory through new and deletes, and pay attention to the difference between heap and stack. 2. Pointers allow direct operation of memory addresses, and use them with caution. Smart pointers can simplify management. 3. Template implements generic programming, improves code reusability and flexibility, and needs to understand type derivation and specialization.
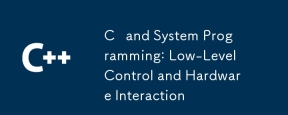
C is suitable for system programming and hardware interaction because it provides control capabilities close to hardware and powerful features of object-oriented programming. 1)C Through low-level features such as pointer, memory management and bit operation, efficient system-level operation can be achieved. 2) Hardware interaction is implemented through device drivers, and C can write these drivers to handle communication with hardware devices.


Hot AI Tools

Undresser.AI Undress
AI-powered app for creating realistic nude photos

AI Clothes Remover
Online AI tool for removing clothes from photos.

Undress AI Tool
Undress images for free

Clothoff.io
AI clothes remover

AI Hentai Generator
Generate AI Hentai for free.

Hot Article

Hot Tools

Atom editor mac version download
The most popular open source editor
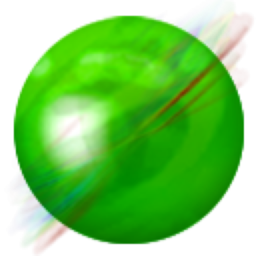
ZendStudio 13.5.1 Mac
Powerful PHP integrated development environment

SublimeText3 Chinese version
Chinese version, very easy to use
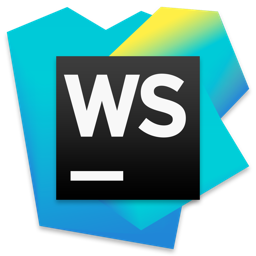
WebStorm Mac version
Useful JavaScript development tools
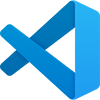
VSCode Windows 64-bit Download
A free and powerful IDE editor launched by Microsoft