How to implement machine vision algorithms and object recognition in C++?
How to implement machine vision algorithm and object recognition in C?
Introduction:
With the continuous development and application of artificial intelligence, machine vision technology has been widely used in various fields, such as autonomous driving, security monitoring, medical imaging, etc. Among them, C, as a widely used programming language, has the characteristics of high compilation efficiency and strong flexibility, and has gradually become the preferred language for the implementation of machine vision algorithms. This article will introduce how to implement machine vision algorithms and object recognition through C, and attach code examples, hoping to provide some help to readers.
1. Implementation of machine vision algorithm
1.1 Image processing
Image processing is an important part of the machine vision algorithm, mainly including image reading, display, saving and common image processing operations (Such as image binarization, filtering, edge detection, etc.). Next, we will introduce how to use C to implement machine vision algorithms through a simple image processing example.
#include <opencv2/core/core.hpp> #include <opencv2/highgui/highgui.hpp> #include <opencv2/imgproc/imgproc.hpp> int main() { // 读取图像 cv::Mat image = cv::imread("lena.jpg", cv::IMREAD_COLOR); // 图像二值化 cv::Mat grayImage; cv::cvtColor(image, grayImage, cv::COLOR_BGR2GRAY); cv::Mat binaryImage; cv::threshold(grayImage, binaryImage, 128, 255, cv::THRESH_BINARY); // 显示图像 cv::imshow("Binary Image", binaryImage); // 保存图像 cv::imwrite("binary.jpg", binaryImage); // 等待按键退出 cv::waitKey(0); return 0; }
In this example, we used the OpenCV library to read and process images. First, we read the image named "lena.jpg" through the cv::imread
function. Then, we convert the color image into a grayscale image and perform a binarization operation on the grayscale image through the cv::threshold function. Finally, we display the binarized image through the cv::imshow
function, and use the cv::imwrite
function to save the binary image to a file named "binary.jpg" in the file.
1.2 Feature extraction and description
Feature extraction and description is one of the core tasks in machine vision algorithms. It is the process of extracting representative features from images and describing them. In this section we will use the OpenCV library to implement an example of the SIFT (Scale Invariant Feature Transform) algorithm.
#include <opencv2/core/core.hpp> #include <opencv2/highgui/highgui.hpp> #include <opencv2/features2d/features2d.hpp> int main() { // 读取图像 cv::Mat image = cv::imread("lena.jpg", cv::IMREAD_COLOR); // 使用SIFT算法检测图像中的关键点 cv::Ptr<cv::SIFT> sift = cv::SIFT::create(); std::vector<cv::KeyPoint> keypoints; sift->detect(image, keypoints); // 绘制关键点 cv::Mat keypointImage; cv::drawKeypoints(image, keypoints, keypointImage, cv::Scalar::all(-1), cv::DrawMatchesFlags::DRAW_RICH_KEYPOINTS); // 显示图像 cv::imshow("Keypoints", keypointImage); // 等待按键退出 cv::waitKey(0); return 0; }
In this example, we use the cv::SIFT
class in the OpenCV library to implement the SIFT algorithm. First, we read the image named "lena.jpg" through the cv::imread
function. Then, we created a cv::SIFT
objectsift
and used the sift->detect
function to detect key points in the image. Next, we draw the key points on the image through the cv::drawKeypoints
function, and use the cv::imshow
function to display the results.
2. Implementation of object recognition
Object recognition is one of the important applications in machine vision. It completes the object recognition task by matching objects in images with pre-trained models. . In this section we will use the DNN (deep neural network) module in the OpenCV library to implement an example of object recognition.
#include <opencv2/core/utility.hpp> #include <opencv2/core/core.hpp> #include <opencv2/dnn/dnn.hpp> #include <opencv2/highgui/highgui.hpp> #include <opencv2/imgproc/imgproc.hpp> int main() { // 加载模型及相应的配置文件 std::string model = "MobileNetSSD_deploy.caffemodel"; std::string config = "MobileNetSSD_deploy.prototxt"; cv::dnn::Net net = cv::dnn::readNetFromCaffe(config, model); // 加载图像 cv::Mat image = cv::imread("person.jpg", cv::IMREAD_COLOR); // 对图像进行预处理 cv::Mat blob = cv::dnn::blobFromImage(image, 1.0, cv::Size(300, 300), cv::Scalar(127.5, 127.5, 127.5), true, false); // 将blob输入到网络中进行推理 net.setInput(blob); // 获取检测结果 cv::Mat detection = net.forward(); // 解析检测结果 cv::Mat detectionMat(detection.size[2], detection.size[3], CV_32F, detection.ptr<float>()); for (int i = 0; i < detectionMat.rows; i++) { float confidence = detectionMat.at<float>(i, 2); if (confidence > 0.5) { int x1 = static_cast<int>(detectionMat.at<float>(i, 3) * image.cols); int y1 = static_cast<int>(detectionMat.at<float>(i, 4) * image.rows); int x2 = static_cast<int>(detectionMat.at<float>(i, 5) * image.cols); int y2 = static_cast<int>(detectionMat.at<float>(i, 6) * image.rows); // 绘制边界框 cv::rectangle(image, cv::Point(x1, y1), cv::Point(x2, y2), cv::Scalar(0, 255, 0), 2); } } // 显示结果 cv::imshow("Detection", image); // 等待按键退出 cv::waitKey(0); return 0; }
In this example, we use the cv::dnn::Net
class in the OpenCV library to load the model and configuration file, and use cv::imread
The function reads the image named "person.jpg". Next, we preprocess the image through the cv::dnn::blobFromImage
function, and then input the processed data into the network for inference. Finally, we parse the detection results and draw the detected bounding box using the cv::rectangle
function.
Conclusion:
Through the introduction of this article, we have learned how to use C to implement machine vision algorithms and object recognition. From image processing to feature extraction and description, to object recognition, the C and OpenCV libraries provide a wealth of tools and functions to help us implement machine vision algorithms efficiently. I hope this article can provide readers with some help and inspiration in implementing machine vision algorithms and object recognition in C.
The above is the detailed content of How to implement machine vision algorithms and object recognition in C++?. For more information, please follow other related articles on the PHP Chinese website!
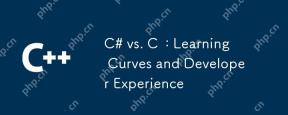
There are significant differences in the learning curves of C# and C and developer experience. 1) The learning curve of C# is relatively flat and is suitable for rapid development and enterprise-level applications. 2) The learning curve of C is steep and is suitable for high-performance and low-level control scenarios.
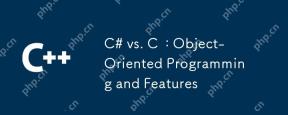
There are significant differences in how C# and C implement and features in object-oriented programming (OOP). 1) The class definition and syntax of C# are more concise and support advanced features such as LINQ. 2) C provides finer granular control, suitable for system programming and high performance needs. Both have their own advantages, and the choice should be based on the specific application scenario.
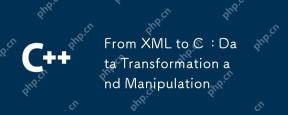
Converting from XML to C and performing data operations can be achieved through the following steps: 1) parsing XML files using tinyxml2 library, 2) mapping data into C's data structure, 3) using C standard library such as std::vector for data operations. Through these steps, data converted from XML can be processed and manipulated efficiently.
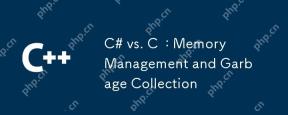
C# uses automatic garbage collection mechanism, while C uses manual memory management. 1. C#'s garbage collector automatically manages memory to reduce the risk of memory leakage, but may lead to performance degradation. 2.C provides flexible memory control, suitable for applications that require fine management, but should be handled with caution to avoid memory leakage.

C still has important relevance in modern programming. 1) High performance and direct hardware operation capabilities make it the first choice in the fields of game development, embedded systems and high-performance computing. 2) Rich programming paradigms and modern features such as smart pointers and template programming enhance its flexibility and efficiency. Although the learning curve is steep, its powerful capabilities make it still important in today's programming ecosystem.
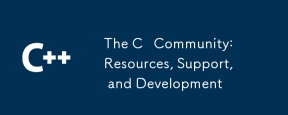
C Learners and developers can get resources and support from StackOverflow, Reddit's r/cpp community, Coursera and edX courses, open source projects on GitHub, professional consulting services, and CppCon. 1. StackOverflow provides answers to technical questions; 2. Reddit's r/cpp community shares the latest news; 3. Coursera and edX provide formal C courses; 4. Open source projects on GitHub such as LLVM and Boost improve skills; 5. Professional consulting services such as JetBrains and Perforce provide technical support; 6. CppCon and other conferences help careers

C# is suitable for projects that require high development efficiency and cross-platform support, while C is suitable for applications that require high performance and underlying control. 1) C# simplifies development, provides garbage collection and rich class libraries, suitable for enterprise-level applications. 2)C allows direct memory operation, suitable for game development and high-performance computing.
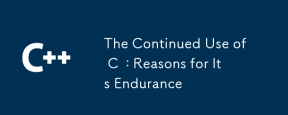
C Reasons for continuous use include its high performance, wide application and evolving characteristics. 1) High-efficiency performance: C performs excellently in system programming and high-performance computing by directly manipulating memory and hardware. 2) Widely used: shine in the fields of game development, embedded systems, etc. 3) Continuous evolution: Since its release in 1983, C has continued to add new features to maintain its competitiveness.


Hot AI Tools

Undresser.AI Undress
AI-powered app for creating realistic nude photos

AI Clothes Remover
Online AI tool for removing clothes from photos.

Undress AI Tool
Undress images for free

Clothoff.io
AI clothes remover

AI Hentai Generator
Generate AI Hentai for free.

Hot Article

Hot Tools

PhpStorm Mac version
The latest (2018.2.1) professional PHP integrated development tool

SAP NetWeaver Server Adapter for Eclipse
Integrate Eclipse with SAP NetWeaver application server.

SublimeText3 English version
Recommended: Win version, supports code prompts!

Atom editor mac version download
The most popular open source editor
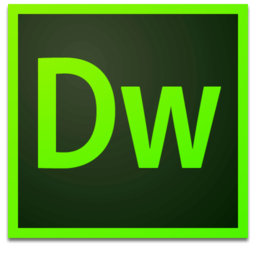
Dreamweaver Mac version
Visual web development tools