How to deal with data redundancy issues in C++ big data development?
How to deal with the data redundancy problem in C big data development?
Data redundancy refers to storing the same or similar data multiple times during the development process. This results in a waste of data storage space and seriously affects the performance and efficiency of the program. In big data development, the problem of data redundancy is particularly prominent. Therefore, solving the problem of data redundancy is an important task to improve the efficiency of big data development and reduce resource consumption.
This article will introduce how to use C language to deal with data redundancy issues in big data development, and provide corresponding code examples.
1. Use pointers to reduce data copy
When processing big data, data copy operations are often required, which consumes a lot of time and memory. To solve this problem, we can use pointers to reduce data copying. The following is a sample code:
#include <iostream> int main() { int* data = new int[1000000]; // 假设data为一个大数据数组 // 使用指针进行数据操作 int* temp = data; for (int i = 0; i < 1000000; i++) { *temp++ = i; // 数据赋值操作 } // 使用指针访问数据 temp = data; for (int i = 0; i < 1000000; i++) { std::cout << *temp++ << " "; // 数据读取操作 } delete[] data; // 释放内存 return 0; }
In the above code, we use the pointer temp to replace the copy operation, which can reduce the number of data copies and improve the execution efficiency of the code.
2. Use data compression technology to reduce storage space
Data redundancy leads to a waste of storage space. In order to solve this problem, we can use compression technology to reduce data storage space. Commonly used data compression algorithms include Huffman coding, LZW compression algorithm, etc. Following is the sample code for data compression using Huffman coding:
#include <iostream> #include <queue> #include <vector> #include <map> struct Node { int frequency; char data; Node* left; Node* right; Node(int freq, char d) { frequency = freq; data = d; left = nullptr; right = nullptr; } }; struct compare { bool operator()(Node* left, Node* right) { return (left->frequency > right->frequency); } }; void generateCodes(Node* root, std::string code, std::map<char, std::string>& codes) { if (root == nullptr) { return; } if (root->data != '') { codes[root->data] = code; } generateCodes(root->left, code + "0", codes); generateCodes(root->right, code + "1", codes); } std::string huffmanCompression(std::string text) { std::map<char, int> frequencies; for (char c : text) { frequencies[c]++; } std::priority_queue<Node*, std::vector<Node*>, compare> pq; for (auto p : frequencies) { pq.push(new Node(p.second, p.first)); } while (pq.size() > 1) { Node* left = pq.top(); pq.pop(); Node* right = pq.top(); pq.pop(); Node* newNode = new Node(left->frequency + right->frequency, ''); newNode->left = left; newNode->right = right; pq.push(newNode); } std::map<char, std::string> codes; generateCodes(pq.top(), "", codes); std::string compressedText = ""; for (char c : text) { compressedText += codes[c]; } return compressedText; } std::string huffmanDecompression(std::string compressedText, std::map<char, std::string>& codes) { Node* root = new Node(0, ''); Node* current = root; std::string decompressedText = ""; for (char c : compressedText) { if (c == '0') { current = current->left; } else { current = current->right; } if (current->data != '') { decompressedText += current->data; current = root; } } delete root; return decompressedText; } int main() { std::string text = "Hello, world!"; std::string compressedText = huffmanCompression(text); std::cout << "Compressed text: " << compressedText << std::endl; std::map<char, std::string> codes; generateCodes(compressedText, "", codes); std::string decompressedText = huffmanDecompression(compressedText, codes); std::cout << "Decompressed text: " << decompressedText << std::endl; return 0; }
In the above code, we are using Huffman coding to compress the text. First count the frequency of each character in the text, and then build a Huffman tree based on the frequency. Then the code of each character is generated, and 0 and 1 are used to represent the code to reduce the storage space occupied. Finally, the text is compressed and decompressed, and the results are output.
Summary:
By using pointers to reduce data copying and data compression technology to reduce storage space, we can effectively solve the data redundancy problem in big data development. In actual development, it is necessary to choose appropriate methods to deal with data redundancy according to specific circumstances to improve program performance and efficiency.
The above is the detailed content of How to deal with data redundancy issues in C++ big data development?. For more information, please follow other related articles on the PHP Chinese website!
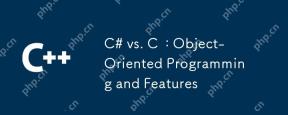
There are significant differences in how C# and C implement and features in object-oriented programming (OOP). 1) The class definition and syntax of C# are more concise and support advanced features such as LINQ. 2) C provides finer granular control, suitable for system programming and high performance needs. Both have their own advantages, and the choice should be based on the specific application scenario.
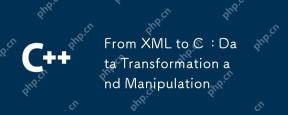
Converting from XML to C and performing data operations can be achieved through the following steps: 1) parsing XML files using tinyxml2 library, 2) mapping data into C's data structure, 3) using C standard library such as std::vector for data operations. Through these steps, data converted from XML can be processed and manipulated efficiently.
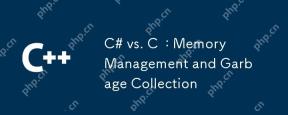
C# uses automatic garbage collection mechanism, while C uses manual memory management. 1. C#'s garbage collector automatically manages memory to reduce the risk of memory leakage, but may lead to performance degradation. 2.C provides flexible memory control, suitable for applications that require fine management, but should be handled with caution to avoid memory leakage.

C still has important relevance in modern programming. 1) High performance and direct hardware operation capabilities make it the first choice in the fields of game development, embedded systems and high-performance computing. 2) Rich programming paradigms and modern features such as smart pointers and template programming enhance its flexibility and efficiency. Although the learning curve is steep, its powerful capabilities make it still important in today's programming ecosystem.
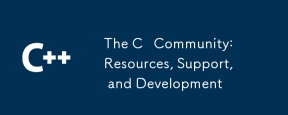
C Learners and developers can get resources and support from StackOverflow, Reddit's r/cpp community, Coursera and edX courses, open source projects on GitHub, professional consulting services, and CppCon. 1. StackOverflow provides answers to technical questions; 2. Reddit's r/cpp community shares the latest news; 3. Coursera and edX provide formal C courses; 4. Open source projects on GitHub such as LLVM and Boost improve skills; 5. Professional consulting services such as JetBrains and Perforce provide technical support; 6. CppCon and other conferences help careers

C# is suitable for projects that require high development efficiency and cross-platform support, while C is suitable for applications that require high performance and underlying control. 1) C# simplifies development, provides garbage collection and rich class libraries, suitable for enterprise-level applications. 2)C allows direct memory operation, suitable for game development and high-performance computing.
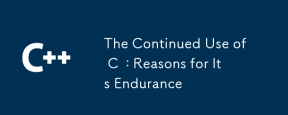
C Reasons for continuous use include its high performance, wide application and evolving characteristics. 1) High-efficiency performance: C performs excellently in system programming and high-performance computing by directly manipulating memory and hardware. 2) Widely used: shine in the fields of game development, embedded systems, etc. 3) Continuous evolution: Since its release in 1983, C has continued to add new features to maintain its competitiveness.
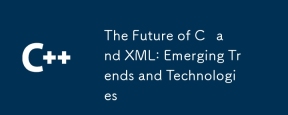
The future development trends of C and XML are: 1) C will introduce new features such as modules, concepts and coroutines through the C 20 and C 23 standards to improve programming efficiency and security; 2) XML will continue to occupy an important position in data exchange and configuration files, but will face the challenges of JSON and YAML, and will develop in a more concise and easy-to-parse direction, such as the improvements of XMLSchema1.1 and XPath3.1.


Hot AI Tools

Undresser.AI Undress
AI-powered app for creating realistic nude photos

AI Clothes Remover
Online AI tool for removing clothes from photos.

Undress AI Tool
Undress images for free

Clothoff.io
AI clothes remover

AI Hentai Generator
Generate AI Hentai for free.

Hot Article

Hot Tools

SecLists
SecLists is the ultimate security tester's companion. It is a collection of various types of lists that are frequently used during security assessments, all in one place. SecLists helps make security testing more efficient and productive by conveniently providing all the lists a security tester might need. List types include usernames, passwords, URLs, fuzzing payloads, sensitive data patterns, web shells, and more. The tester can simply pull this repository onto a new test machine and he will have access to every type of list he needs.

PhpStorm Mac version
The latest (2018.2.1) professional PHP integrated development tool

DVWA
Damn Vulnerable Web App (DVWA) is a PHP/MySQL web application that is very vulnerable. Its main goals are to be an aid for security professionals to test their skills and tools in a legal environment, to help web developers better understand the process of securing web applications, and to help teachers/students teach/learn in a classroom environment Web application security. The goal of DVWA is to practice some of the most common web vulnerabilities through a simple and straightforward interface, with varying degrees of difficulty. Please note that this software
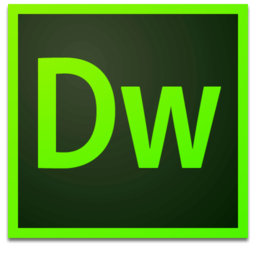
Dreamweaver Mac version
Visual web development tools
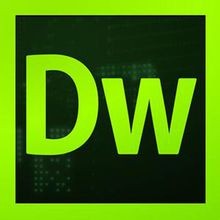
Dreamweaver CS6
Visual web development tools