


In recent years, image generation technology has made many key breakthroughs. Especially since the release of large models such as DALLE2 and Stable Diffusion, text generation image technology has gradually matured, and high-quality image generation has broad practical scenarios. However, detailed editing of existing images is still a difficult problem
On the one hand, due to the limitations of text descriptions, existing high-quality textual image models , only text can be used to edit pictures descriptively, and for some specific effects, text is difficult to describe; on the other hand, in actual application scenarios, image refinement editing tasks often only have a small number of reference pictures , This makes it difficult for many solutions that require a large amount of data for training to work when there is a small amount of data, especially when there is only one reference image.
Recently, researchers from NetEase Interactive Entertainment AI Lab proposed an image-to-image editing solution based on single image guidance. Given a single reference image, Transfer objects or styles in the reference image to the source image without changing the overall structure of the source image. The research paper has been accepted by ICCV 2023, and the relevant code has been open source.
- Paper address: https://arxiv.org/abs/2307.14352
- Code address: https://github.com/CrystalNeuro/visual-concept-translator
Let us first look at a set of pictures to feel its effect .
Thesis renderings: The upper left corner of each set of pictures is the source picture, the lower left corner is the reference picture, and the right side is the generated result picture
Main Body Framework
The author of the paper proposed an image editing framework based on Inversion-Fusion (Inversion-Fusion) - VCT (visual concept translator, visual concept converter). As shown in the figure below, the overall framework of VCT includes two processes: content-concept inversion process (Content-concept Inversion) and content-concept fusion process (Content-concept Fusion). The content-concept inversion process uses two different inversion algorithms to learn and represent the latent vectors of the structural information of the original image and the semantic information of the reference image respectively; the content-concept fusion process uses the latent vectors of the structural information and semantic information. Fusion to generate the final result.
The content that needs to be rewritten is: the main framework of the paper
It is worth mentioning that in In recent years, inversion methods have been widely used in the field of Generative Adversarial Networks (GAN) and have achieved remarkable results in many image generation tasks [1]. When GAN rewrites content, the original text needs to be rewritten into Chinese. The original sentence does not need to appear. A picture can be mapped to the hidden space of the trained GAN generator, and the purpose of editing can be achieved by controlling the hidden space. This inversion scheme can fully exploit the generative power of pre-trained generative models. This study actually rewrites the content with GAN. The original text needs to be rewritten into Chinese, and the original sentence does not need to appear. It is applied to image editing tasks based on image guidance with the diffusion model as a priori.
When rewriting the content, the original text needs to be rewritten into Chinese, and the original sentence does not need to appear
## Method introduction
Based on the idea of inversion, VCT designed a two-branch diffusion process, which includes a branch B* for content reconstruction and a main branch B for editing. They start from the same noise xT obtained from DDIM Inversion【2】, an algorithm that uses diffusion models to calculate noise from images. , respectively for content reconstruction and content editing. The pre-training model used in this paper is Latent Diffusion Models (LDM). The diffusion process occurs in the latent vector space z space. The double-branch process can be expressed as:
Double-branch diffusion process
Content reconstruction branch B* learns T content feature vectors , used to restore the structure of the original image information, and pass the structural information to the editing main branch B through the soft attention control scheme. The soft attention control scheme draws on Google's prompt2prompt[3] work. The formula is:
That is, when the number of steps of the diffusion model is within a certain range , replace the attention feature map of the editing main branch with the feature map of the content reconstruction branch to achieve structural control of the generated images. The editing main branch B combines the content feature vector learned from the original image and the concept feature vector
learned from the reference image to generate the edited picture.
Noise space (space) fusion
is diffusing At each step of the model, the fusion of feature vectors occurs in the noise space, which is a weighting of the noise predicted after the feature vectors are input into the diffusion model. The feature mixing of the content reconstruction branch occurs on the content feature vector and the empty text vector, which is consistent with the form of classifier-free diffusion guidance [4]:
The mixture of the edit main branch is a mixture of the content feature vector and the concept feature vector
, which is
At this point, the key to the research is how to obtain the feature vector of structural information from a single source image, and Obtain the feature vector of concept information
from a single reference image. The article achieves this purpose through two different inversion schemes.
In order to restore the source image, the article refers to the NULL-text[5] optimization scheme and learns the feature vectors of T stages to match the fitted source image. But unlike NULL-text, which optimizes the empty text vector to fit the DDIM path, this article directly fits the estimated clean feature vector by optimizing the source image feature vector. The fitting formula is:
Different from learning structural information, the concept information in the reference image needs to be represented by a single highly generalized feature vector. The T stages of the diffusion model share a concept feature vector . The article optimizes the existing inversion schemes Textual Inversion [6] and DreamArtist [7]. It uses a multi-concept feature vector to represent the content of the reference image. The loss function includes a noise estimation term of the diffusion model and an estimated reconstruction loss term in the latent vector space:
Experimental results
The article is on the subject replacement and stylization tasks Experiments were conducted to transform the content into the main body or style of the reference image while better maintaining the structural information of the source image.
Rewritten content: Paper on experimental effects
Compared with previous solutions, the VCT framework proposed in this article has the following advantages:
##(1) Application generalization:Compared with previous image editing tasks based on image guidance, VCT does not require a large amount of data for training, and has better generation quality and generalization. It is based on the idea of inversion and is based on high-quality Vincentian graph models pre-trained on open world data. In actual application, only one input image and one reference image are needed to achieve better image editing effects.
(2) Visual accuracy: Compared with recent text editing solutions for images, VCT uses pictures for reference guidance. Picture reference allows you to edit pictures more accurately than text descriptions. The following figure shows the comparison results between VCT and other solutions:
Comparison of the effects of the subject replacement task
Comparison effect of style transfer task
(3) No additional information is required: Comparison For some recent solutions that require adding additional control information (such as mask maps or depth maps) for guidance control, VCT directly learns structural information and semantic information from the source image and reference image for fusion generation. The following figure is Some comparison results. Among them, Paint-by-example replaces the corresponding objects with objects in the reference image by providing a mask map of the source image; Controlnet controls the generated results through line drawings, depth maps, etc.; and VCT directly draws from the source image. and reference images, learning structural information and content information to be fused into target images without additional restrictions.
Comparative effect of image editing scheme based on image guidance
NetEase Interactive Entertainment AI Lab
NetEase Interactive Entertainment AI Laboratory was established in 2017. It is affiliated to NetEase Interactive Entertainment Business Group and is the leading artificial intelligence laboratory in the game industry. The laboratory focuses on the research and application of computer vision, speech and natural language processing, and reinforcement learning in game scenarios. It aims to improve the technical level of NetEase Interactive Entertainment’s popular games and products through AI technology. At present, this technology has been used in many popular games, such as "Fantasy Westward Journey", "Harry Potter: Magic Awakening", "Onmyoji", "Westward Journey", etc.
The above is the detailed content of Various styles of VCT guidance, all with one picture, allowing you to easily implement it. For more information, please follow other related articles on the PHP Chinese website!
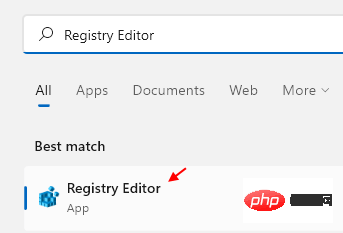
<p>Windows 11 改进了系统中的个性化功能,这使用户可以查看之前所做的桌面背景更改的近期历史记录。当您进入windows系统设置应用程序中的个性化部分时,您可以看到各种选项,更改背景壁纸也是其中之一。但是现在可以看到您系统上设置的背景壁纸的最新历史。如果您不喜欢看到此内容并想清除或删除此最近的历史记录,请继续阅读这篇文章,它将帮助您详细了解如何使用注册表编辑器进行操作。</p><h2>如何使用注册表编辑
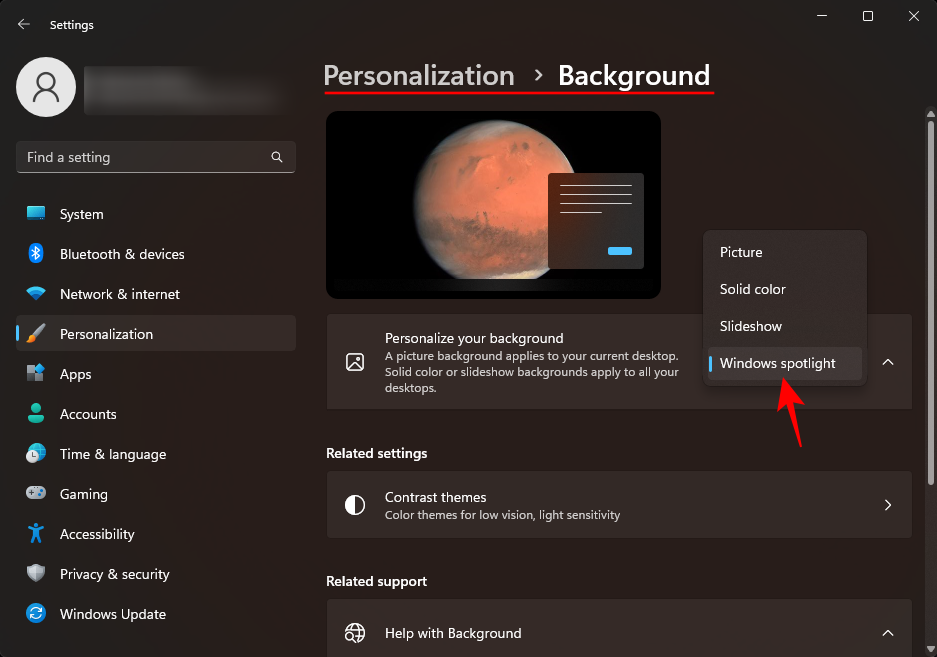
窗户从来不是一个忽视美学的人。从XP的田园绿场到Windows11的蓝色漩涡设计,默认桌面壁纸多年来一直是用户愉悦的源泉。借助WindowsSpotlight,您现在每天都可以直接访问锁屏和桌面壁纸的美丽、令人敬畏的图像。不幸的是,这些图像并没有闲逛。如果您爱上了Windows聚光灯图像之一,那么您将想知道如何下载它们,以便将它们作为背景保留一段时间。以下是您需要了解的所有信息。什么是WindowsSpotlight?窗口聚光灯是一个自动壁纸更新程序,可以从“设置”应用中的“个性化>
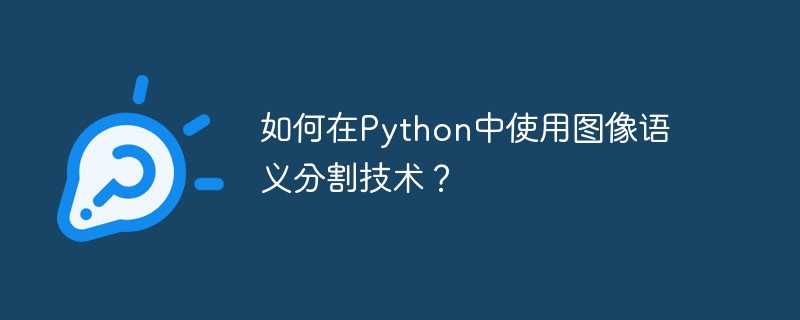
随着人工智能技术的不断发展,图像语义分割技术已经成为图像分析领域的热门研究方向。在图像语义分割中,我们将一张图像中的不同区域进行分割,并对每个区域进行分类,从而达到对这张图像的全面理解。Python是一种著名的编程语言,其强大的数据分析和数据可视化能力使其成为了人工智能技术研究领域的首选。本文将介绍如何在Python中使用图像语义分割技术。一、前置知识在深入
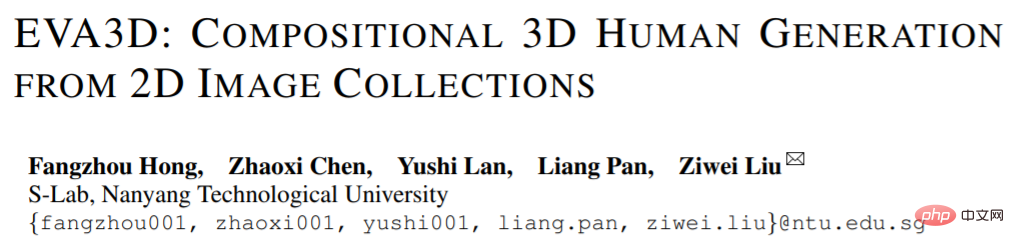
得益于 NeRF 提供的可微渲染,近期的三维生成模型已经在静止物体上达到了很惊艳的效果。但是在人体这种更加复杂且可形变的类别上,三维生成依旧有很大的挑战。本文提出了一个高效的组合的人体 NeRF 表达,实现了高分辨率(512x256)的三维人体生成,并且没有使用超分模型。EVA3D 在四个大型人体数据集上均大幅超越了已有方案,代码已开源。论文名称:EVA3D: Compositional 3D Human Generation from 2D image Collections论文地址:http
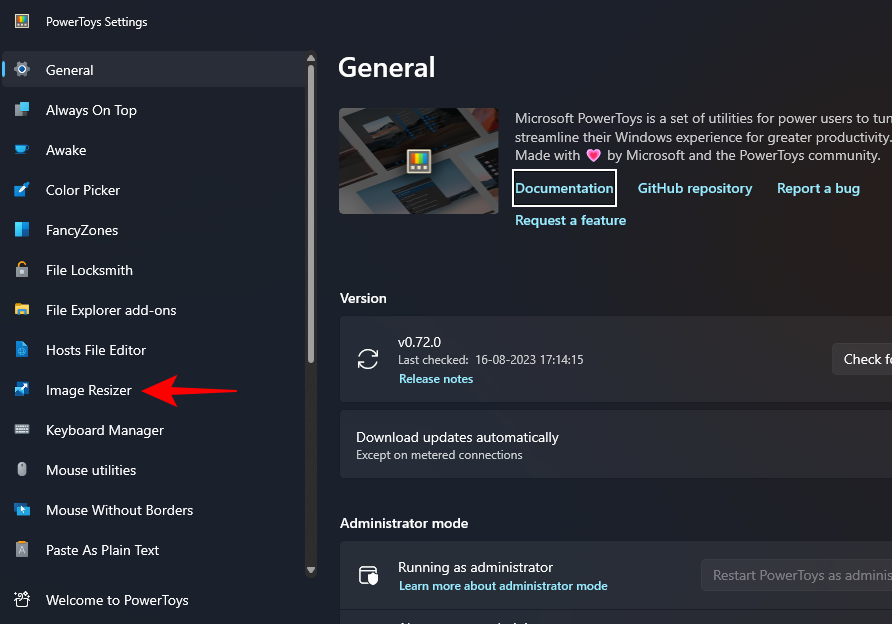
那些必须每天处理图像文件的人经常不得不调整它们的大小以适应他们的项目和工作的需求。但是,如果要处理的图像太多,则单独调整它们的大小会消耗大量时间和精力。在这种情况下,像PowerToys这样的工具可以派上用场,除其他外,可以使用其图像调整大小器实用程序批量调整图像文件的大小。以下是设置图像调整器设置并开始使用PowerToys批量调整图像大小的方法。如何使用PowerToys批量调整图像大小PowerToys是一个多合一的程序,具有各种实用程序和功能,可帮助您加快日常任务。它的实用程序之一是图像
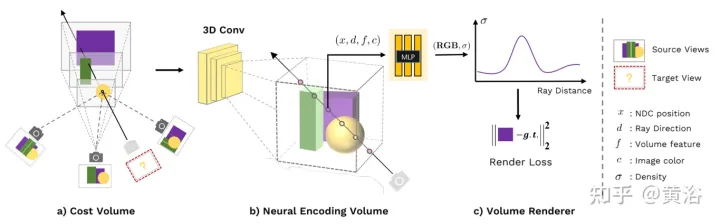
新视角图像生成(NVS)是计算机视觉的一个应用领域,在1998年SuperBowl的比赛,CMU的RI曾展示过给定多摄像头立体视觉(MVS)的NVS,当时这个技术曾转让给美国一家体育电视台,但最终没有商业化;英国BBC广播公司为此做过研发投入,但是没有真正产品化。在基于图像渲染(IBR)领域,NVS应用有一个分支,即基于深度图像的渲染(DBIR)。另外,在2010年曾很火的3D TV,也是需要从单目视频中得到双目立体,但是由于技术的不成熟,最终没有流行起来。当时基于机器学习的方法已经开始研究,比
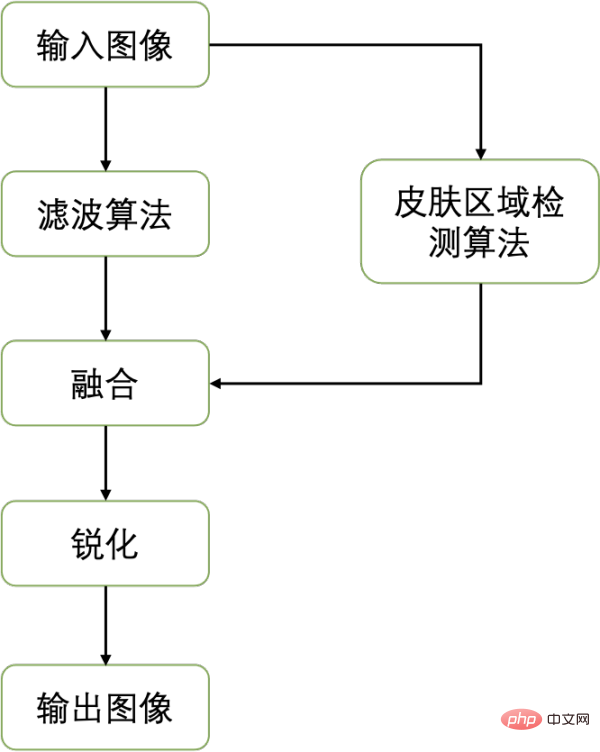
随着数字文化产业的蓬勃发展,人工智能技术开始广泛应用于图像编辑和美化领域。其中,人像美肤无疑是应用最广、需求最大的技术之一。传统美颜算法利用基于滤波的图像编辑技术,实现了自动化的磨皮去瑕疵效果,在社交、直播等场景取得了广泛的应用。然而,在门槛较高的专业摄影行业,由于对图像分辨率以及质量标准的较高要求,人工修图师还是作为人像美肤修图的主要生产力,完成包括匀肤、去瑕疵、美白等一系列工作。通常,一位专业修图师对一张高清人像进行美肤操作的平均处理时间为 1-2 分钟,在精度要求更高的广告、影视等领域,该
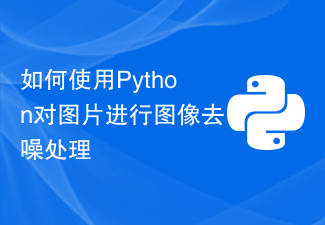
如何使用Python对图片进行图像去噪处理图像去噪是图像处理中的一项重要任务,它的目的是去除图像中的噪声,提高图像的质量和清晰度。Python是一种功能强大的编程语言,拥有丰富的图像处理库,如PIL、OpenCV等,可以帮助我们实现图像去噪的功能。本文将介绍如何使用Python对图片进行图像去噪处理,并给出相应的代码示例。导入所需的库首先,我们需要导入所需的


Hot AI Tools

Undresser.AI Undress
AI-powered app for creating realistic nude photos

AI Clothes Remover
Online AI tool for removing clothes from photos.

Undress AI Tool
Undress images for free

Clothoff.io
AI clothes remover

AI Hentai Generator
Generate AI Hentai for free.

Hot Article

Hot Tools

SublimeText3 Chinese version
Chinese version, very easy to use
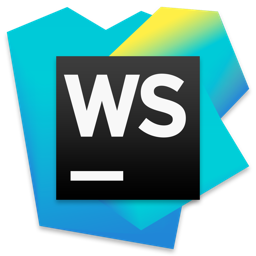
WebStorm Mac version
Useful JavaScript development tools

Zend Studio 13.0.1
Powerful PHP integrated development environment

SublimeText3 Linux new version
SublimeText3 Linux latest version

Safe Exam Browser
Safe Exam Browser is a secure browser environment for taking online exams securely. This software turns any computer into a secure workstation. It controls access to any utility and prevents students from using unauthorized resources.
