


Building a distributed blog system using Java and Redis: How to handle large amounts of article data
Building a distributed blog system using Java and Redis: How to process a large amount of article data
Introduction:
With the rapid development of Internet technology, blogs have become an important place for users to share knowledge, opinions and experiences. platform. Along with this comes a large amount of article data that needs to be stored and processed. To address this challenge, building a distributed blog system using Java and Redis is an effective solution. This article will introduce how to use Java and Redis to process large amounts of article data, and provide code examples.
1. Data model design
Before building a distributed blog system, we need to design the data model first. The key entity of the blog system is the article, and we can use a hash table to store the information of each article. The key of the hash table can be the unique identifier of the article (such as article ID), and the value can include information such as article title, author, publication time, content, etc. In addition to article information, we also need to consider ancillary information such as article classification, tags, and comments. This information can be stored using data structures such as ordered sets, lists, and hash tables.
2. Use Java to operate Redis
Java is a powerful programming language that can interact well with Redis. The following are some common Java example codes for operating Redis:
-
Connecting to the Redis server
Jedis jedis = new Jedis("localhost", 6379);
-
Storing article information
Map<String, String> article = new HashMap<>(); article.put("title", "Java与Redis构建分布式博客系统"); article.put("author", "John"); article.put("content", "..."); jedis.hmset("article:1", article);
-
Get article information
Map<String, String> article = jedis.hgetAll("article:1"); System.out.println(article.get("title")); System.out.println(article.get("author")); System.out.println(article.get("content"));
-
Add article category
jedis.zadd("categories", 1, "技术"); jedis.zadd("categories", 2, "生活");
-
Get the article list under the category
Set<String> articles = jedis.zrangeByScore("categories", 1, 1); for(String articleId : articles){ Map<String, String> article = jedis.hgetAll("article:" + articleId); System.out.println(article.get("title")); }
3. Distributed processing of large amounts of article data
When building a distributed blog system, we need to consider how to process large amounts of article data. A common method is to use sharding technology to disperse and store data in multiple Redis instances. Each instance is responsible for a part of the article data and provides corresponding read and write interfaces.
The following is a simple sample code to show how to use sharding technology to achieve distributed processing of large amounts of article data:
-
Create a Redis instance
List<Jedis> shards = new ArrayList<>(); shards.add(new Jedis("node1", 6379)); shards.add(new Jedis("node2", 6379)); shards.add(new Jedis("node3", 6379));
-
Storing article information
int shardIndex = calculateShardIndex(articleId); Jedis shard = shards.get(shardIndex); shard.hmset("article:" + articleId, article);
-
Getting article information
int shardIndex = calculateShardIndex(articleId); Jedis shard = shards.get(shardIndex); Map<String, String> article = shard.hgetAll("article:" + articleId);
-
Shard calculation method
private int calculateShardIndex(String articleId){ // 根据文章ID计算分片索引 int shardCount = shards.size(); return Math.abs(articleId.hashCode() % shardCount); }
4. Optimization of high-performance read and write operations
In order to improve the read and write performance of the distributed blog system, we can use the following optimization techniques:
- Use connection pool: Added to the Redis client to avoid frequent creation and destruction of connections.
- Batch operation: Use the pipelining mechanism to package multiple read and write operations and send them to the Redis server to reduce network overhead.
- Data caching: Use caching technology (such as Redis' caching function) to store popular article data in memory to reduce database load.
5. Summary
This article introduces how to use Java and Redis to build a distributed blog system and how to process a large amount of article data. Through reasonable data model design, Java operation of Redis and distributed processing technology, we can build a high-performance blog system. At the same time, the performance of the system can be further improved through read and write operation optimization technology. I hope this article helps you understand how to build distributed systems that handle large amounts of data.
(Total word count: 829 words)
The above is the detailed content of Building a distributed blog system using Java and Redis: How to handle large amounts of article data. For more information, please follow other related articles on the PHP Chinese website!
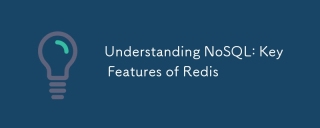
Key features of Redis include speed, flexibility and rich data structure support. 1) Speed: Redis is an in-memory database, and read and write operations are almost instantaneous, suitable for cache and session management. 2) Flexibility: Supports multiple data structures, such as strings, lists, collections, etc., which are suitable for complex data processing. 3) Data structure support: provides strings, lists, collections, hash tables, etc., which are suitable for different business needs.
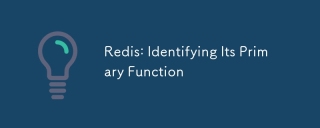
The core function of Redis is a high-performance in-memory data storage and processing system. 1) High-speed data access: Redis stores data in memory and provides microsecond-level read and write speed. 2) Rich data structure: supports strings, lists, collections, etc., and adapts to a variety of application scenarios. 3) Persistence: Persist data to disk through RDB and AOF. 4) Publish subscription: Can be used in message queues or real-time communication systems.

Redis supports a variety of data structures, including: 1. String, suitable for storing single-value data; 2. List, suitable for queues and stacks; 3. Set, used for storing non-duplicate data; 4. Ordered Set, suitable for ranking lists and priority queues; 5. Hash table, suitable for storing object or structured data.
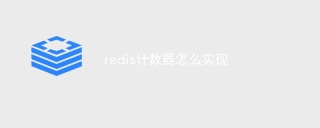
Redis counter is a mechanism that uses Redis key-value pair storage to implement counting operations, including the following steps: creating counter keys, increasing counts, decreasing counts, resetting counts, and obtaining counts. The advantages of Redis counters include fast speed, high concurrency, durability and simplicity and ease of use. It can be used in scenarios such as user access counting, real-time metric tracking, game scores and rankings, and order processing counting.
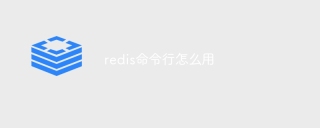
Use the Redis command line tool (redis-cli) to manage and operate Redis through the following steps: Connect to the server, specify the address and port. Send commands to the server using the command name and parameters. Use the HELP command to view help information for a specific command. Use the QUIT command to exit the command line tool.
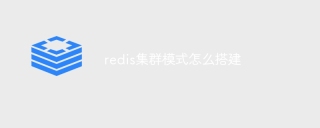
Redis cluster mode deploys Redis instances to multiple servers through sharding, improving scalability and availability. The construction steps are as follows: Create odd Redis instances with different ports; Create 3 sentinel instances, monitor Redis instances and failover; configure sentinel configuration files, add monitoring Redis instance information and failover settings; configure Redis instance configuration files, enable cluster mode and specify the cluster information file path; create nodes.conf file, containing information of each Redis instance; start the cluster, execute the create command to create a cluster and specify the number of replicas; log in to the cluster to execute the CLUSTER INFO command to verify the cluster status; make
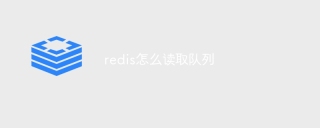
To read a queue from Redis, you need to get the queue name, read the elements using the LPOP command, and process the empty queue. The specific steps are as follows: Get the queue name: name it with the prefix of "queue:" such as "queue:my-queue". Use the LPOP command: Eject the element from the head of the queue and return its value, such as LPOP queue:my-queue. Processing empty queues: If the queue is empty, LPOP returns nil, and you can check whether the queue exists before reading the element.
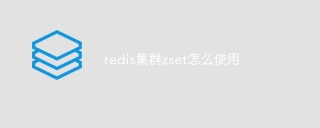
Use of zset in Redis cluster: zset is an ordered collection that associates elements with scores. Sharding strategy: a. Hash sharding: Distribute the hash value according to the zset key. b. Range sharding: divide into ranges according to element scores, and assign each range to different nodes. Read and write operations: a. Read operations: If the zset key belongs to the shard of the current node, it will be processed locally; otherwise, it will be routed to the corresponding shard. b. Write operation: Always routed to shards holding the zset key.


Hot AI Tools

Undresser.AI Undress
AI-powered app for creating realistic nude photos

AI Clothes Remover
Online AI tool for removing clothes from photos.

Undress AI Tool
Undress images for free

Clothoff.io
AI clothes remover

AI Hentai Generator
Generate AI Hentai for free.

Hot Article

Hot Tools

MinGW - Minimalist GNU for Windows
This project is in the process of being migrated to osdn.net/projects/mingw, you can continue to follow us there. MinGW: A native Windows port of the GNU Compiler Collection (GCC), freely distributable import libraries and header files for building native Windows applications; includes extensions to the MSVC runtime to support C99 functionality. All MinGW software can run on 64-bit Windows platforms.

DVWA
Damn Vulnerable Web App (DVWA) is a PHP/MySQL web application that is very vulnerable. Its main goals are to be an aid for security professionals to test their skills and tools in a legal environment, to help web developers better understand the process of securing web applications, and to help teachers/students teach/learn in a classroom environment Web application security. The goal of DVWA is to practice some of the most common web vulnerabilities through a simple and straightforward interface, with varying degrees of difficulty. Please note that this software
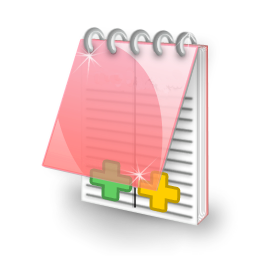
EditPlus Chinese cracked version
Small size, syntax highlighting, does not support code prompt function

SublimeText3 Linux new version
SublimeText3 Linux latest version

SublimeText3 Chinese version
Chinese version, very easy to use