


Building a recommendation system using Redis and Python: How to provide personalized recommendations
In the Internet era, recommendation systems have become one of the core functions of major platforms. By analyzing user behavior and personal preferences, recommendation systems can provide users with personalized content recommendations. This article will introduce how to use Redis and Python to build a simple recommendation system, and provide relevant code examples.
- Introduction to Redis
Redis is an open source, high-performance key-value storage system. It supports a variety of data structures, such as strings, lists, sets, ordered sets, etc., and provides a wealth of commands and functions suitable for various scenarios. In recommendation systems, Redis can be used to store user behavior data and recommendation results, and quickly perform data query and calculation.
- Building a user behavior recording module
The first step in the recommendation system is to collect and record user behavior data. We can use Redis's ordered set data structure to implement a user behavior recording module. The following is a simple example:
import redis # 连接Redis r = redis.Redis(host='localhost', port=6379, db=0) # 记录用户行为 def record_user_behavior(user_id, item_id): r.zincrby('user_behavior', 1, f'{user_id}:{item_id}') # 获取用户行为排行榜 def get_user_behavior_ranking(): return r.zrevrange('user_behavior', 0, -1, withscores=True)
In the above example, we record the user's behavior in the user_behavior
ordered collection through the zincrby
command, and use the user ID and item IDs are identified as members of an ordered set. The zincrby
command can perform an auto-increment operation on the specified member of the ordered set, making it convenient for us to count the number of user actions on different items.
- Build a recommendation model and calculate the recommendation results
The core of the recommendation system is the recommendation model and recommendation algorithm. In this article, we will use the collaborative filtering algorithm to implement a user-based recommendation system. The following is a simple example:
# 构建协同过滤推荐模型 def build_collaborative_filtering_model(): # 获取用户行为数据 behavior_data = get_user_behavior_ranking() # 构建用户相似度矩阵 similarity_matrix = {} for i in range(len(behavior_data)): user1, behavior1 = behavior_data[i] user1 = user1.split(':')[0] for j in range(i+1, len(behavior_data)): user2, behavior2 = behavior_data[j] user2 = user2.split(':')[0] # 计算用户相似度(这里简化为用户行为次数的比较) similarity = abs(int(behavior1) - int(behavior2)) # 更新用户相似度矩阵 if user1 not in similarity_matrix: similarity_matrix[user1] = {} similarity_matrix[user1][user2] = similarity if user2 not in similarity_matrix: similarity_matrix[user2] = {} similarity_matrix[user2][user1] = similarity return similarity_matrix # 根据用户行为和相似度矩阵进行推荐 def recommend_items(user_id, similarity_matrix): user_similarities = similarity_matrix[user_id] items = {} for user, similarity in user_similarities.items(): for item in r.zscan_iter(f'user_behavior', match=f'{user}:*'): item_id = item.decode().split(':')[1] items[item_id] = items.get(item_id, 0) + similarity sorted_items = sorted(items.items(), key=lambda x: x[1], reverse=True) return [item[0] for item in sorted_items[:5]]
In the above example, we build the collaborative filtering recommendation model through the build_collaborative_filtering_model
function, calculate the similarity between users, and use recommend_items
The function makes recommendations based on the similarity matrix. This simplifies the calculation of similarity and the acquisition of recommendation results, and can be optimized and improved according to specific needs in actual projects.
- Calling example
# 记录用户行为 record_user_behavior(1, 'item1') record_user_behavior(1, 'item2') record_user_behavior(2, 'item2') record_user_behavior(2, 'item3') # 构建推荐模型 similarity_matrix = build_collaborative_filtering_model() # 获取推荐结果 recommendations = recommend_items(1, similarity_matrix) print(recommendations)
In the calling example, we first recorded the behavior of two users, then built the recommendation model and obtained the recommendation results of user 1. The output will return a list of items that user 1 may be interested in.
Through the combination of Redis and Python, we can quickly build a simple personalized recommendation system. Of course, actual recommendation systems involve more complex algorithms and models, and this article only provides a basic framework and examples for reference. Readers can make further improvements and expansions based on actual needs.
The above is the detailed content of Building a recommendation system using Redis and Python: How to provide personalized recommendations. For more information, please follow other related articles on the PHP Chinese website!
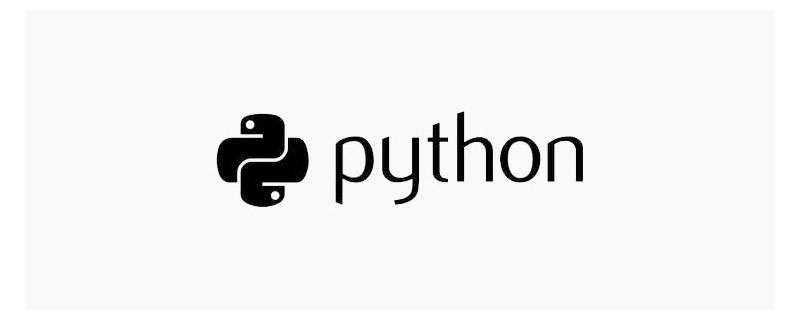
本篇文章给大家带来了关于Python的相关知识,其中主要介绍了关于Seaborn的相关问题,包括了数据可视化处理的散点图、折线图、条形图等等内容,下面一起来看一下,希望对大家有帮助。
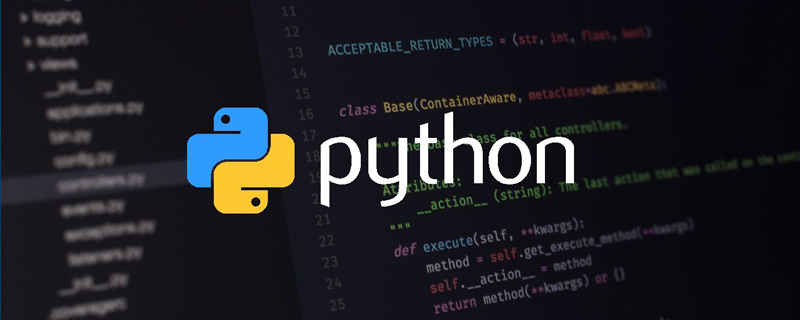
本篇文章给大家带来了关于Python的相关知识,其中主要介绍了关于进程池与进程锁的相关问题,包括进程池的创建模块,进程池函数等等内容,下面一起来看一下,希望对大家有帮助。
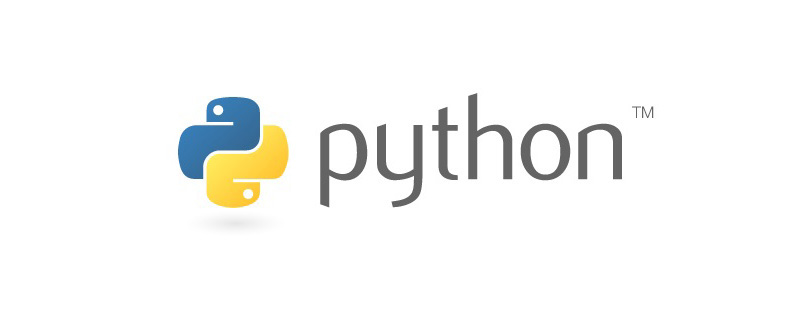
本篇文章给大家带来了关于Python的相关知识,其中主要介绍了关于简历筛选的相关问题,包括了定义 ReadDoc 类用以读取 word 文件以及定义 search_word 函数用以筛选的相关内容,下面一起来看一下,希望对大家有帮助。
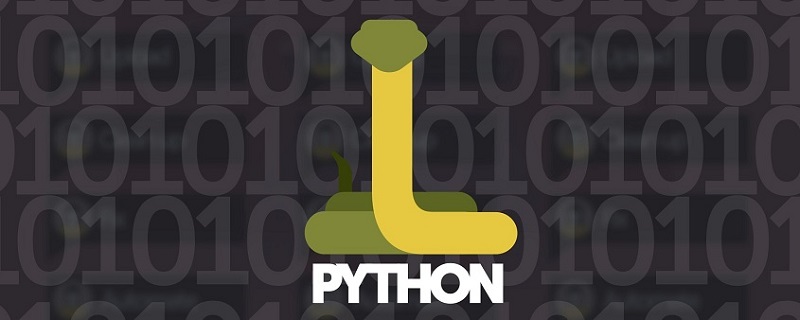
本篇文章给大家带来了关于Python的相关知识,其中主要介绍了关于数据类型之字符串、数字的相关问题,下面一起来看一下,希望对大家有帮助。
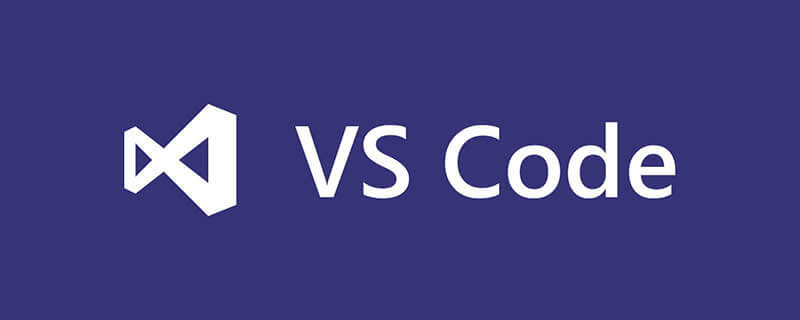
VS Code的确是一款非常热门、有强大用户基础的一款开发工具。本文给大家介绍一下10款高效、好用的插件,能够让原本单薄的VS Code如虎添翼,开发效率顿时提升到一个新的阶段。
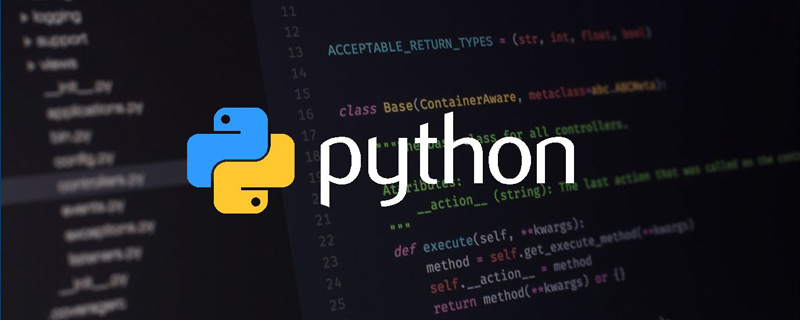
本篇文章给大家带来了关于Python的相关知识,其中主要介绍了关于numpy模块的相关问题,Numpy是Numerical Python extensions的缩写,字面意思是Python数值计算扩展,下面一起来看一下,希望对大家有帮助。
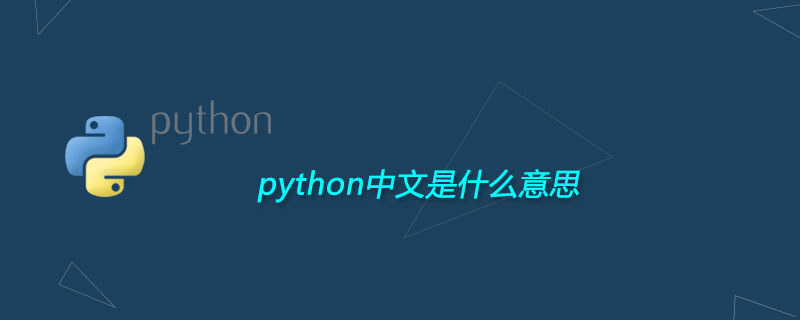
pythn的中文意思是巨蟒、蟒蛇。1989年圣诞节期间,Guido van Rossum在家闲的没事干,为了跟朋友庆祝圣诞节,决定发明一种全新的脚本语言。他很喜欢一个肥皂剧叫Monty Python,所以便把这门语言叫做python。


Hot AI Tools

Undresser.AI Undress
AI-powered app for creating realistic nude photos

AI Clothes Remover
Online AI tool for removing clothes from photos.

Undress AI Tool
Undress images for free

Clothoff.io
AI clothes remover

AI Hentai Generator
Generate AI Hentai for free.

Hot Article

Hot Tools
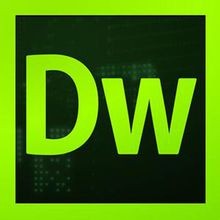
Dreamweaver CS6
Visual web development tools
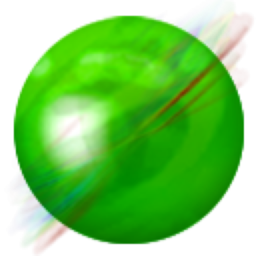
ZendStudio 13.5.1 Mac
Powerful PHP integrated development environment

MinGW - Minimalist GNU for Windows
This project is in the process of being migrated to osdn.net/projects/mingw, you can continue to follow us there. MinGW: A native Windows port of the GNU Compiler Collection (GCC), freely distributable import libraries and header files for building native Windows applications; includes extensions to the MSVC runtime to support C99 functionality. All MinGW software can run on 64-bit Windows platforms.
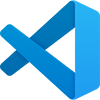
VSCode Windows 64-bit Download
A free and powerful IDE editor launched by Microsoft
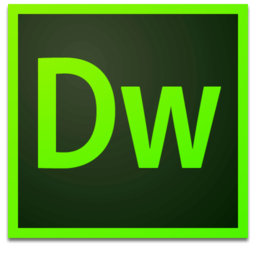
Dreamweaver Mac version
Visual web development tools
