


The perception and grasping of transparent objects in complex environments is a recognized problem in the field of robotics and computer vision. Recently, a team and collaborators from Tsinghua University Shenzhen International Graduate School proposed a visual-tactile fusion transparent object grasping framework, which is based on an RGB camera and a mechanical claw TaTa with tactile sensing capabilities, and uses sim2real To realize the grasping position detection of transparent objects. This framework can not only solve the problem of grasping irregular transparent objects such as glass fragments, but also solve the problems of grasping overlapping, stacked, uneven, sand piles and even highly dynamic underwater transparent objects.
Picture
Transparent objects are widely used in life due to their beauty, simplicity and other characteristics. For example, they can be found in kitchens, shops, and factories. Although transparent objects are common, grasping transparent objects is a very difficult problem for robots. There are three main reasons:
Picture
1. It does not have its own texture attributes. The information on the surface of transparent objects changes with the change of the environment, and the texture produced is mostly caused by light refraction and reflection, which brings great difficulties to the detection of transparent objects.
2. The annotation of transparent data sets is more difficult than the annotation of ordinary objects. In actual scenes, it is sometimes difficult for humans to distinguish transparent objects such as glass, let alone label images of transparent objects.
3. The surface of a transparent object is smooth, and even a small deviation in the grabbing position may lead to the failure of the grabbing task.
Therefore, how to solve the problem of grasping transparent objects in various complex scenes with as little cost as possible has become a very important issue in the field of transparent object research. Recently, the intelligent perception and robotics team from Tsinghua University Shenzhen International Graduate School proposed a transparent object grabbing framework based on visual and touch fusion. To realize the detection and grabbing of transparent objects. This method not only has a very high grabbing success rate, but can also be adapted to grabbing transparent objects in various complex scenes.
Picture
Please view the following paper link: https://ieeexplore.ieee.org/document/10175024
The corresponding author of the paper, Associate Professor Ding Wenbo of Shenzhen International Graduate School of Tsinghua University, said: "Robots have shown great application value in the field of home services, but most of the current robots focus on a single field and are general-purpose. The proposed robot grasping model will bring great impetus to the promotion and application of robot technology. Although we use transparent objects as the research object, this framework can be easily extended to the grasping tasks of common objects in life. "
The corresponding author of the paper and researcher Liu Houde from the Shenzhen International Graduate School of Tsinghua University said: "The unstructured environment in the home scene has brought great challenges to the practical application of robots. , we integrate vision and touch for perception, further simulating the perception process when humans interact with the outside world, and providing various guarantees for the stability of robot applications in complex scenarios. In addition to integrating vision and touch, the framework we propose It can also be extended to more modalities such as hearing."
Research Status
Grasping transparent objects is a challenge For this task, in addition to detecting the position of the object during the grabbing process, the grabbing position and angle should also be considered. Currently, most work on grasping transparent objects is performed on a plane with a simple background, but in real life, most scenes will not be as ideal as our experimental environment. Some special scenes, such as glass fragments, piles, overlaps, undulations, sand and underwater scenes, are more challenging.
- First of all, glass fragments are objects without a fixed model. Due to their random and changeable shapes, they place high demands on the versatility of the grasping network and grasping tools.
- Secondly, grabbing transparent objects on undulating planes is also challenging. As shown in the figure below, on the one hand, the depth information of transparent objects is difficult to obtain accurately, and on the other hand, the undulating scene has some shadows, overlaps, and reflection areas, which brings more challenges to the detection of transparent objects.
- Thirdly, due to the similar optical properties of water and transparent objects, grasping transparent objects in underwater scenes is also a challenge. Even with a depth camera, transparent objects cannot be accurately detected in water, and the situation gets worse when illuminated by light from different directions.
Picture
Algorithm Design
Picture
The design of the grasping algorithm is shown in the figure. In order to realize the grasping of transparent objects, we respectively proposed a transparent object grasping position detection algorithm, a tactile information extraction algorithm and a visual-tactile fusion classification. algorithm. In order to reduce the cost of labeling the dataset, we used Blender to create a multi-background transparent object grabbing synthetic dataset SimTrans12K, which contains 12,000 synthetic images and 160 real images. In addition to the dataset, we also propose a Gaussian-Mask annotation method for the unique optical properties of transparent objects. Since we use the Jamming gripper as the executor, we propose a specialized grasping network TGCNN for it, which can achieve good detection results after training on the synthetic data set.
Grasping framework
We have integrated the above algorithms to complete the capturing of transparent objects in different scenarios. Fetching, which constitutes the upper-level grabbing strategy of our visual-touch fusion framework. We decompose a grasping task into three subtasks, namely object classification, grasping position detection and grasping height detection. Each subtask can be accomplished by vision, touch, or a combination of vision and touch.
Similar to human grasping behavior, when vision can directly obtain the precise position of an object, we can control the hand to directly reach the object and complete the grasp, as shown in the figure below (A) Show. When vision cannot accurately obtain the position information of an object, after using vision to estimate the position of the object, we will use the tactile sensing function of the hand to slowly adjust the grasping position until the object is contacted and the appropriate grasping position is reached, as shown below (B) is shown. For object grasping under limited vision conditions, as shown in the figure below (C), we will use the rich tactile nerves of the hand to search within the possible range of the target until contact with the object is made. Although this is very inefficient, But it is an effective method to solve object grabbing in these special scenarios.
Picture
Inspired by human grasping strategies, we divide transparent object grasping tasks into three types: Flat surfaces with complex backgrounds, irregular scenes, and visually undetectable scenes, as shown in the figure below. In the first type, vision plays a key role, and we define the grasping method in this scenario as a vision-first grasping method. In the second type, vision and touch can work together, and we define the grasping method in this scenario as visual-tactile grasping. In the last type, vision may fail and touch becomes dominant in the task. We define the grasping method in this scenario as a touch-first grasping method.
Picture
The flow of the vision-first grabbing method is shown in the figure below. First, TGCNN is used to obtain the grabbing position and height. Then the tactile information is used to capture the position calibration, and finally the visual-tactile fusion algorithm is used for classification. The visual-tactile grasping is based on the previous one and adds the THS module, which can use the touch to obtain the height of the object. The haptic-first grasping approach has been joined by a TPE module that uses the sense of touch to obtain the position of transparent objects.
Experimental verification
In order to verify the effectiveness of our proposed framework and algorithm, we conducted a large number of verification experiments.
First, in order to test the effectiveness of our proposed transparent object dataset, annotation method and grasping position detection network, we conducted synthetic data detection experiments and under different backgrounds and brightness Transparent object grasping position detection experiment. Secondly, in order to verify the effectiveness of the visual-tactile fusion grasping framework, we designed a transparent object classification grasping experiment and a transparent fragment grasping experiment. Third, we designed a transparent object grasping experiment in irregular and visually restricted scenes to test the effectiveness of the framework after adding the THS module and TPE module.
Summary
To address the challenging problem of detecting, grasping, and classifying transparent objects, this study proposes a synthetic dataset-based A framework for visual-haptic fusion. First, the Blender simulation engine is used to render synthetic datasets instead of manually annotated datasets.
In addition, Gaussian-Mask is used instead of the traditional binary annotation method to make the generation of grab positions more accurate. In order to detect the grasping position of transparent objects, the author proposed an algorithm called TGCNN and conducted multiple comparative experiments. The results show that even if only synthetic data sets are used for training, the algorithm can perform well on different backgrounds. and lighting conditions to achieve good detection.
Considering the difficulty in grasping caused by the limitations of visual detection, this study proposes a tactile calibration method combined with the soft gripper TaTa, by adjusting the grasp with tactile information location to improve the crawling success rate. Compared with pure visual grasping, this method improves the grasping success rate by 36.7%.
In order to solve the problem of classifying transparent objects in complex scenes, this study proposes a transparent object classification method based on vision-tactile fusion, which is compared with a classification based on vision alone. , the accuracy increased by 39.1%.
In addition, in order to achieve transparent object grabbing in irregular and visually undetectable scenes, this study proposes THS and TPE modules, which can compensate for the lack of visual information. The transparent object grabbing problem below. The researchers systematically designed a large number of experiments to verify the effectiveness of the proposed framework in complex scenes such as various superpositions, overlaps, undulations, sandy areas, and underwater scenes. The study believes that the proposed framework can also be applied to object detection in low-visibility environments, such as smoke and turbid water, where tactile perception can make up for the lack of visual detection and improve classification accuracy through visual-tactile fusion.
About the author
The instructor of the visual-touch fusion transparent object grasping project is Ding Wenbo. Currently, he is an associate professor at Shenzhen International Graduate School of Tsinghua University, where he leads the intelligent perception and robotics research group. His research interests mainly include signal processing, machine learning, wearable devices, flexible human-computer interaction and machine perception. He previously graduated with a bachelor's degree and a doctoral degree from the Department of Electronic Engineering of Tsinghua University, and served as a postdoctoral fellow at the Georgia Institute of Technology, where he studied under Academician Wang Zhonglin. He has won many awards such as the Tsinghua University Special Prize, the Gold Medal of the 47th International Exhibition of Inventions of Geneva, the IEEE Scott Helt Memorial Award, the Second Prize of the Natural Science Award of the China Electronics Society, etc., and has worked in Nature Communications, Science Advances, Energy and Environmental Science, Advanced Energy He has published more than 70 papers in authoritative journals in the fields of Materials, IEEE TRO/RAL and other fields, has been cited more than 6,000 times by Google Scholar, and has authorized more than 10 patents in China and the United States. He serves as the associate editor of the authoritative international signal processing journal Digital Signal Processing, the chief guest editor of the IEEE JSTSP Special Issue on Robot Perception, and the Applied Signal Processing Systems Technical Committee Member of the IEEE Signal Processing Society.
Homepage of the research group: http://ssr-group.net/.
From left to right: Shoujie Li,Haixin Yu,Houde Liu
The co-authors of the paper are Shoujie Li (Ph.D. student at Tsinghua University) and Haixin Yu (Master student at Tsinghua University). The corresponding authors are Wenbo Ding and Houde Liu. Other authors include Linqi Ye (Shanghai University ), Chongkun Xia (Tsinghua University), Xueqian Wang (Tsinghua University), Xiao-Ping Zhang (Tsinghua University). Among them, Shoujie Li's main research directions are robot grasping, tactile perception and deep learning. As the first author, he has published many papers in authoritative robotics and control journals and conferences such as Soft Robotics, TRO, RAL, ICRA, IROS, etc., and has authorized 10 invention patents. The remaining projects have won 10 provincial and ministerial competition awards. The relevant research results were selected as the first author in the "ICRA 2022 Outstanding Mechanisms and Design Paper Finalists". He has won honors such as Tsinghua University Future Scholar Scholarship and National Scholarship.
The above is the detailed content of It can grab glass fragments and underwater transparent objects. Tsinghua has proposed a universal transparent object grabbing framework with a very high success rate.. For more information, please follow other related articles on the PHP Chinese website!
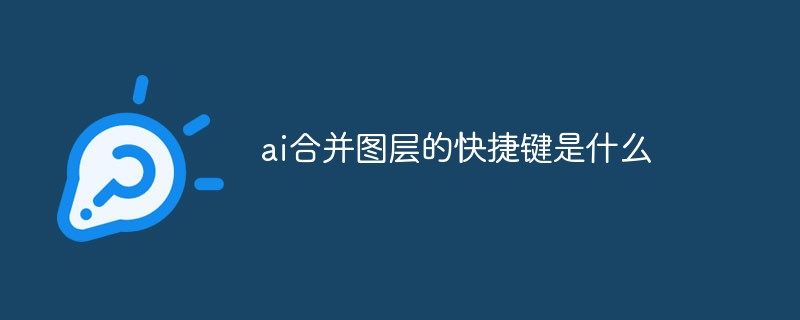
ai合并图层的快捷键是“Ctrl+Shift+E”,它的作用是把目前所有处在显示状态的图层合并,在隐藏状态的图层则不作变动。也可以选中要合并的图层,在菜单栏中依次点击“窗口”-“路径查找器”,点击“合并”按钮。
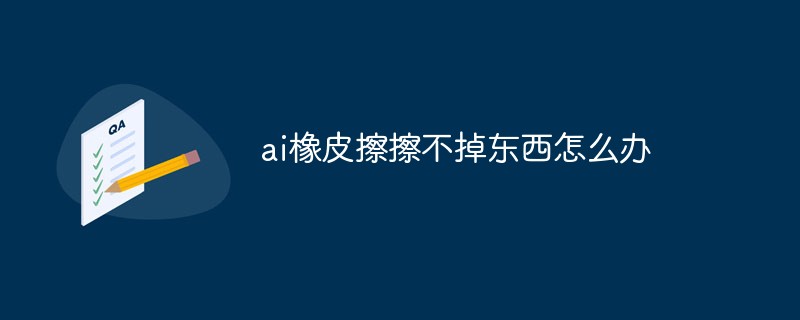
ai橡皮擦擦不掉东西是因为AI是矢量图软件,用橡皮擦不能擦位图的,其解决办法就是用蒙板工具以及钢笔勾好路径再建立蒙板即可实现擦掉东西。
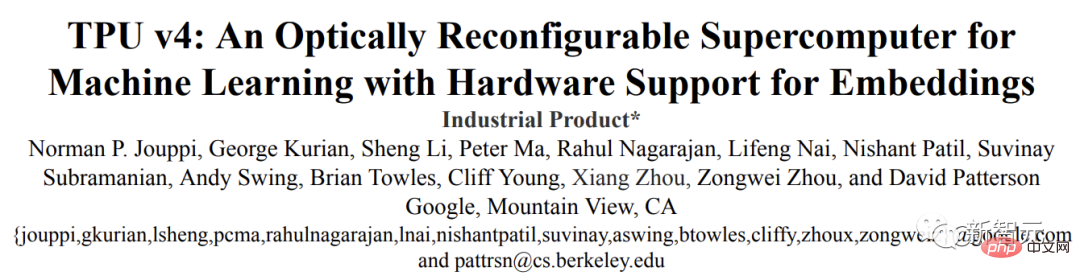
虽然谷歌早在2020年,就在自家的数据中心上部署了当时最强的AI芯片——TPU v4。但直到今年的4月4日,谷歌才首次公布了这台AI超算的技术细节。论文地址:https://arxiv.org/abs/2304.01433相比于TPU v3,TPU v4的性能要高出2.1倍,而在整合4096个芯片之后,超算的性能更是提升了10倍。另外,谷歌还声称,自家芯片要比英伟达A100更快、更节能。与A100对打,速度快1.7倍论文中,谷歌表示,对于规模相当的系统,TPU v4可以提供比英伟达A100强1.
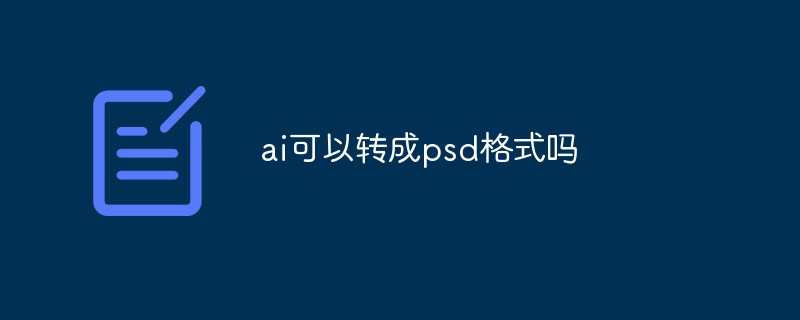
ai可以转成psd格式。转换方法:1、打开Adobe Illustrator软件,依次点击顶部菜单栏的“文件”-“打开”,选择所需的ai文件;2、点击右侧功能面板中的“图层”,点击三杠图标,在弹出的选项中选择“释放到图层(顺序)”;3、依次点击顶部菜单栏的“文件”-“导出”-“导出为”;4、在弹出的“导出”对话框中,将“保存类型”设置为“PSD格式”,点击“导出”即可;
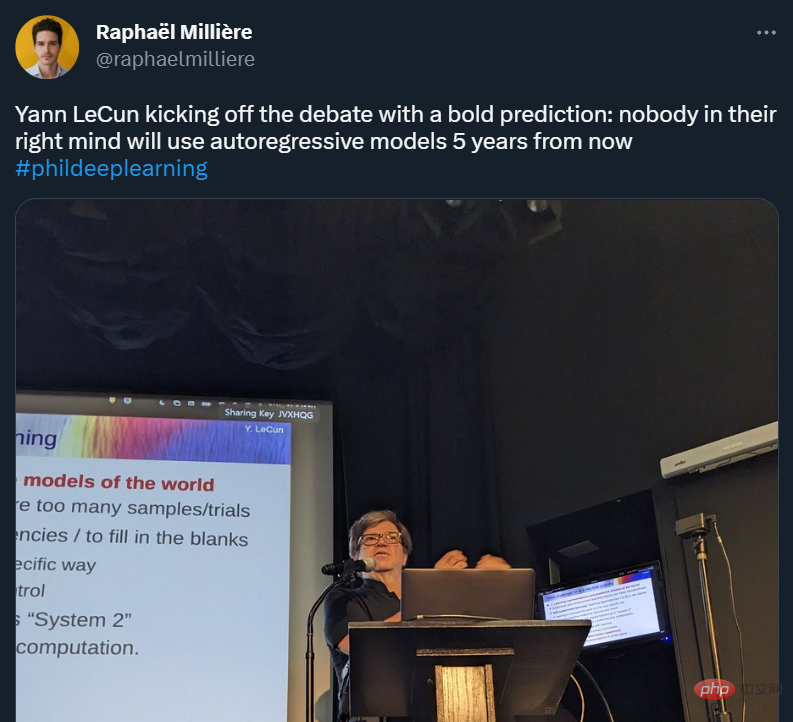
Yann LeCun 这个观点的确有些大胆。 「从现在起 5 年内,没有哪个头脑正常的人会使用自回归模型。」最近,图灵奖得主 Yann LeCun 给一场辩论做了个特别的开场。而他口中的自回归,正是当前爆红的 GPT 家族模型所依赖的学习范式。当然,被 Yann LeCun 指出问题的不只是自回归模型。在他看来,当前整个的机器学习领域都面临巨大挑战。这场辩论的主题为「Do large language models need sensory grounding for meaning and u
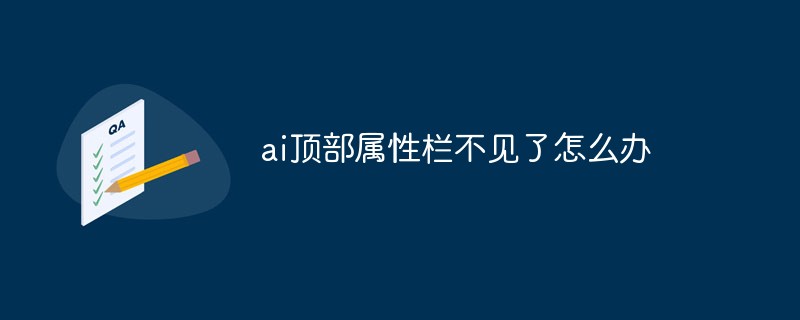
ai顶部属性栏不见了的解决办法:1、开启Ai新建画布,进入绘图页面;2、在Ai顶部菜单栏中点击“窗口”;3、在系统弹出的窗口菜单页面中点击“控制”,然后开启“控制”窗口即可显示出属性栏。
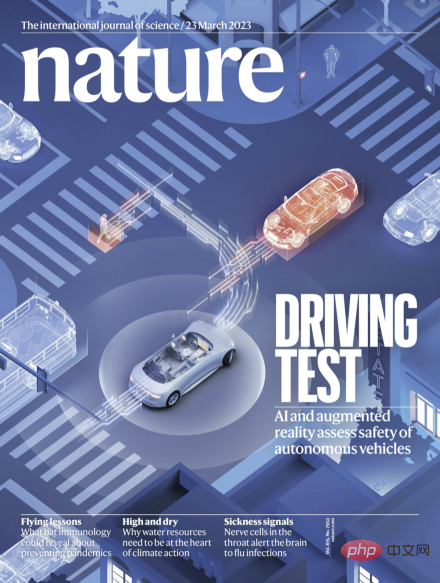
引入密集强化学习,用 AI 验证 AI。 自动驾驶汽车 (AV) 技术的快速发展,使得我们正处于交通革命的风口浪尖,其规模是自一个世纪前汽车问世以来从未见过的。自动驾驶技术具有显着提高交通安全性、机动性和可持续性的潜力,因此引起了工业界、政府机构、专业组织和学术机构的共同关注。过去 20 年里,自动驾驶汽车的发展取得了长足的进步,尤其是随着深度学习的出现更是如此。到 2015 年,开始有公司宣布他们将在 2020 之前量产 AV。不过到目前为止,并且没有 level 4 级别的 AV 可以在市场
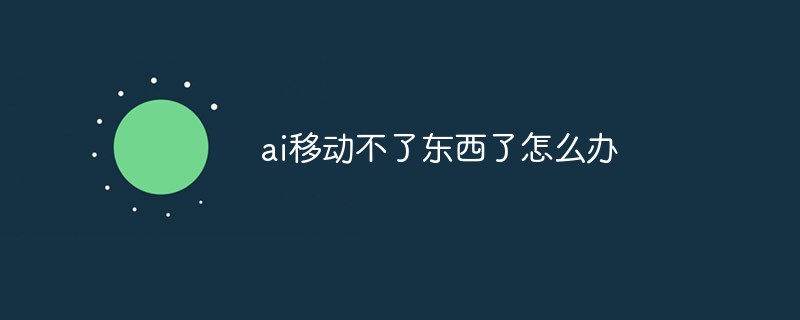
ai移动不了东西的解决办法:1、打开ai软件,打开空白文档;2、选择矩形工具,在文档中绘制矩形;3、点击选择工具,移动文档中的矩形;4、点击图层按钮,弹出图层面板对话框,解锁图层;5、点击选择工具,移动矩形即可。


Hot AI Tools

Undresser.AI Undress
AI-powered app for creating realistic nude photos

AI Clothes Remover
Online AI tool for removing clothes from photos.

Undress AI Tool
Undress images for free

Clothoff.io
AI clothes remover

AI Hentai Generator
Generate AI Hentai for free.

Hot Article

Hot Tools

mPDF
mPDF is a PHP library that can generate PDF files from UTF-8 encoded HTML. The original author, Ian Back, wrote mPDF to output PDF files "on the fly" from his website and handle different languages. It is slower than original scripts like HTML2FPDF and produces larger files when using Unicode fonts, but supports CSS styles etc. and has a lot of enhancements. Supports almost all languages, including RTL (Arabic and Hebrew) and CJK (Chinese, Japanese and Korean). Supports nested block-level elements (such as P, DIV),

Notepad++7.3.1
Easy-to-use and free code editor

SAP NetWeaver Server Adapter for Eclipse
Integrate Eclipse with SAP NetWeaver application server.
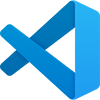
VSCode Windows 64-bit Download
A free and powerful IDE editor launched by Microsoft

DVWA
Damn Vulnerable Web App (DVWA) is a PHP/MySQL web application that is very vulnerable. Its main goals are to be an aid for security professionals to test their skills and tools in a legal environment, to help web developers better understand the process of securing web applications, and to help teachers/students teach/learn in a classroom environment Web application security. The goal of DVWA is to practice some of the most common web vulnerabilities through a simple and straightforward interface, with varying degrees of difficulty. Please note that this software
