


Application case sharing of PHP Bloom filter in large-scale data processing
Introduction:
With the rapid development of the Internet, the scale of data is becoming increasingly large. In the process of processing these large-scale data, we often face various challenges. One of the important issues is how to efficiently query and filter large-scale data to improve system performance and response speed. PHP Bloom filter is an effective tool to solve such problems. Its application will be introduced through a case sharing below.
Overview:
Bloom filter is a data structure that enables fast and efficient data search and filtering. It uses a combination of bit arrays and hash functions to efficiently determine whether an element exists while occupying a small memory space. Its principle is to hash each element through multiple hash functions to different positions in the bit array. As long as one position is 0, the element is considered not to exist.
Case background:
We assume that there is a very large email address database, which contains hundreds of millions of email addresses. Our task is to query whether an email address exists in this huge email address database. Due to the large amount of data, a simple traversal query method will consume a lot of time and resources. At this time, using Bloom filters can significantly improve the speed and efficiency of queries.
Case implementation:
First, we need to install the Bloom filter extension plug-in. It can be installed through the pecl
command:
$ pecl install bloom_filter
After the installation is completed, we can use the bloom_filter
extension in the PHP script. Here is a simple example code:
<?php $bf = new BloomFilter(1000000, 0.001); // 创建一个容量为1000000的布隆过滤器 // 将邮箱地址列表添加到布隆过滤器中 $emails = [/* 邮箱地址列表 */]; foreach ($emails as $email) { $bf->add($email); } // 查询是否存在某个邮箱地址 $emailToCheck = "example@example.com"; if ($bf->has($emailToCheck)) { echo "邮箱地址存在"; } else { echo "邮箱地址不存在"; } ?>
In the above example, we first create a Bloom filter with a capacity of 1000000. We then add the list of email addresses to the bloom filter one by one. Finally, we can query whether an email address exists through the has
method to get the query results.
Case results and reflections:
By using Bloom filters, we can greatly improve the query efficiency of large-scale data. In the above case, if we use the traditional traversal query method, it may take several seconds or minutes to query whether an email address exists. With Bloom filters, we can get accurate query results in a few milliseconds. However, it should be noted that although the Bloom filter can accurately determine the absence of an element, there is a certain misjudgment rate when determining the presence of an element. Therefore, in practical applications, we need to choose appropriate parameters based on specific needs and false positive rate limits.
Conclusion:
As an efficient data search and filtering tool, Bloom filter plays an important role in processing large-scale data. Its application can significantly improve system performance and response speed. Through the sharing of this case, we can better understand and apply Bloom filters.
Appendix: Bloom filter extension documentation and related resources:
- Extension plug-in:
bloom_filter
- https://pecl.php.net/package/ bloom_filter - Bloom filter Wikipedia: https://en.wikipedia.org/wiki/Bloom_filter
The above is the detailed content of Sharing of application cases of PHP Bloom filter in large-scale data processing. For more information, please follow other related articles on the PHP Chinese website!
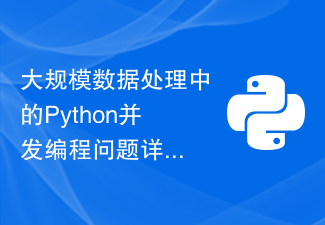
大规模数据处理中的Python并发编程问题详解在当今数据爆炸的时代,大规模数据处理成为了许多领域的重要任务。对于处理海量数据,提高处理效率是至关重要的。而在Python中,通过并发编程可以有效提高程序的执行速度,从而更高效地处理大规模数据。然而,并发编程也存在一些问题和挑战,特别是在大规模数据处理中。下面我们将分析并解决一些常见的Python并发编程问题,并
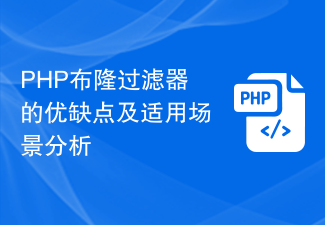
PHP布隆过滤器的优缺点及适用场景分析一、引言随着互联网的蓬勃发展,数据量的爆发式增长,如何高效地处理大规模数据成为了一个亟待解决的问题。在实际应用中,我们常常需要快速判断某个元素是否存在于一个大的数据集合中。这种需求下,布隆过滤器(BloomFilter)成为了一个非常有用的数据结构,它可以高效地判断一个元素是否属于一个集合。二、布隆过滤器的原理布隆过滤
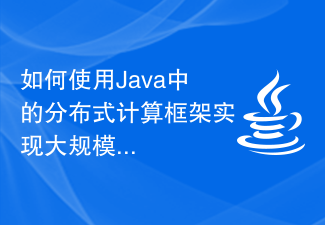
如何使用Java中的分布式计算框架实现大规模数据处理?引言:随着大数据时代的到来,我们需要处理越来越庞大的数据量。传统的单机计算已无法满足这一需求,因此分布式计算成为了解决大规模数据处理问题的一种有效手段。Java作为一种广泛使用的编程语言,提供了多种分布式计算框架,如Hadoop、Spark等。本文将介绍如何使用Java中的分布式计算框架实现大规模数据处理
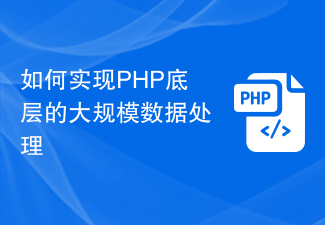
如何实现PHP底层的大规模数据处理,需要具体代码示例在现代的软件开发中,数据处理是一个非常重要而复杂的任务。对于大规模数据的处理,尤其需要考虑到性能和效率的因素。在PHP开发中,我们可以通过优化算法和采用适当的数据结构来实现大规模数据处理的底层操作。本文将介绍一些常见的技术和具体的代码示例,帮助读者实现PHP底层的大规模数据处理。一、使用高效的数据结构在处理
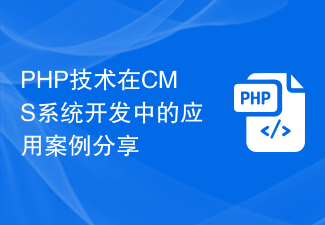
随着互联网的发展,内容管理系统(ContentManagementSystem,简称CMS)越来越暴露出其重要性。CMS作为一种通过互联网进行管理、发布和修改内容的解决方案,使得网站的开发、维护和更新都更加简单和高效。而PHP技术则是CMS系统的核心技术之一,在这篇文章中,我们将分享几个PHP技术在CMS系统开发中的应用案例。WordPressWordP
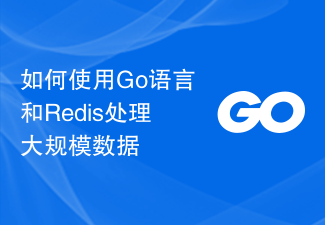
如何使用Go语言和Redis处理大规模数据在当今互联网时代,处理大规模数据成为了许多应用程序的核心需求。Go语言以其简洁、高效和并发性而著称,而Redis是一款高性能的键值存储系统。将它们结合使用,可以优化数据处理速度和资源利用率。本文将介绍如何使用Go语言和Redis处理大规模数据,并提供具体的代码示例。一、连接Redis首先,我们
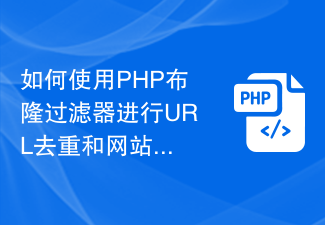
如何使用PHP布隆过滤器进行URL去重和网站爬取管理概述:在进行网站爬取时,一项重要的任务是去除重复的URL,以避免重复爬取相同页面,浪费资源和时间。布隆过滤器是一种高效的数据结构,适用于快速判断一个元素是否存在于一个大集合中。本文将介绍如何使用PHP布隆过滤器进行URL去重和网站爬取管理。安装布隆过滤器扩展首先,我们需要安装PHP的布隆过滤器扩展。可以通过
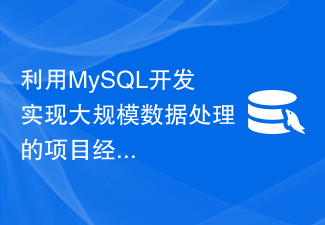
随着互联网的迅猛发展,数据量呈现几何倍数增长,这为数据库的管理和维护带来了极大的挑战。MySQL作为一款优秀的关系型数据库管理系统,随着其功能的不断完善和扩展,被越来越多的企业所接受和采用。本文将从项目实践的角度,分享在大规模数据处理领域利用MySQL开发所遇到的问题和解决方案,以及一些经验和技巧的总结。一、项目概述本项目是一款基于WEB的大数据处理系统,主


Hot AI Tools

Undresser.AI Undress
AI-powered app for creating realistic nude photos

AI Clothes Remover
Online AI tool for removing clothes from photos.

Undress AI Tool
Undress images for free

Clothoff.io
AI clothes remover

AI Hentai Generator
Generate AI Hentai for free.

Hot Article

Hot Tools
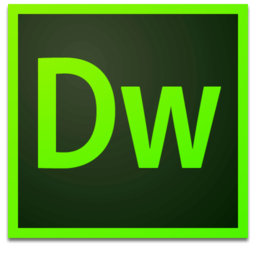
Dreamweaver Mac version
Visual web development tools

MantisBT
Mantis is an easy-to-deploy web-based defect tracking tool designed to aid in product defect tracking. It requires PHP, MySQL and a web server. Check out our demo and hosting services.

PhpStorm Mac version
The latest (2018.2.1) professional PHP integrated development tool

SublimeText3 Chinese version
Chinese version, very easy to use

mPDF
mPDF is a PHP library that can generate PDF files from UTF-8 encoded HTML. The original author, Ian Back, wrote mPDF to output PDF files "on the fly" from his website and handle different languages. It is slower than original scripts like HTML2FPDF and produces larger files when using Unicode fonts, but supports CSS styles etc. and has a lot of enhancements. Supports almost all languages, including RTL (Arabic and Hebrew) and CJK (Chinese, Japanese and Korean). Supports nested block-level elements (such as P, DIV),
