


NTU and Shanghai AI Lab compiled 300+ papers: the latest review of visual segmentation based on Transformer is released
SAM (Segment Anything), as a basic visual segmentation model, has attracted the attention and follow-up of many researchers in just 3 months. If you want to systematically understand the technology behind SAM, keep up with the pace of involution, and be able to make your own SAM model, then this Transformer-Based Segmentation Survey is not to be missed! Recently, several researchers from Nanyang Technological University and Shanghai Artificial Intelligence Laboratory wrote a review about Transformer-Based Segmentation, systematically reviewing the segmentation and detection models based on Transformer in recent years, and conducting research. The latest model is as of June this year! At the same time, the review also includes the latest papers in related fields and a large number of experimental analyzes and comparisons, and reveals a number of future research directions with broad prospects!
Visual segmentation aims to segment images, video frames or point clouds into multiple segments or groups. This technology has many real-world applications, such as autonomous driving, image editing, robot perception, and medical analysis. Over the past decade, deep learning-based methods have made significant progress in this field. Recently, Transformer has become a neural network based on a self-attention mechanism, originally designed for natural language processing, which significantly surpasses previous convolutional or recurrent methods in various visual processing tasks. Specifically, the Vision Transformer provides powerful, unified, and even simpler solutions for various segmentation tasks. This review provides a comprehensive overview of Transformer-based visual segmentation, summarizing recent advances. First, this article reviews the background, including problem definition, data sets, and previous convolution methods. Next, this paper summarizes a meta-architecture that unifies all recent Transformer-based methods. Based on this meta-architecture, this article studies various method designs, including modifications to this meta-architecture and related applications. In addition, this article also introduces several related settings, including 3D point cloud segmentation, basic model tuning, domain adaptive segmentation, efficient segmentation and medical segmentation. Furthermore, this paper compiles and re-evaluates these methods on several widely recognized datasets. Finally, the paper identifies open challenges in this field and proposes directions for future research. This article will continue and track the latest Transformer-based segmentation and detection methods.
Picture
Project address: https://github.com/lxtGH/Awesome-Segmentation-With-Transformer
Paper address: https://arxiv.org/pdf/2304.09854.pdf
Research motivation
- The emergence of ViT and DETR has made full progress in the field of segmentation and detection. Currently, the top-ranking methods on almost every data set benchmark are based on Transformer. For this reason, it is necessary to systematically summarize and compare the methods and technical characteristics of this direction.
- Recent large model architectures are all based on the Transformer structure, including multi-modal models and segmentation basic models (SAM), and various visual tasks are moving closer to unified model modeling.
- Segmentation and detection have derived many related downstream tasks, and many of these tasks are also solved using the Transformer structure.
Summary Features
- Systematic and readable. This article systematically reviews each task definition of segmentation, as well as related task definitions and evaluation indicators. And this article starts from the convolution method and summarizes a meta-architecture based on ViT and DETR. Based on this meta-architecture, this review summarizes and summarizes related methods, and systematically reviews recent methods. The specific technical review route is shown in Figure 1.
- Detailed classification from a technical perspective. Compared with previous Transformer reviews, this article’s classification of methods will be more detailed. This article brings together papers with similar ideas and compares their similarities and differences. For example, this article will classify methods that simultaneously modify the decoder side of the meta-architecture into image-based Cross Attention, and video-based spatio-temporal Cross Attention modeling.
- Comprehensiveness of the research question. This article will systematically review all directions of segmentation, including image, video, and point cloud segmentation tasks. At the same time, this article will also review related directions such as open set segmentation and detection models, unsupervised segmentation and weakly supervised segmentation.
Picture
Figure 1. Survey’s content roadmap
Figure 2. Summary of commonly used data sets and segmentation tasks
Summary of Transformer-Based segmentation and detection methods and Comparison
##Figure 3. General Meta-Architecture Framework
This article first summarizes a meta-architecture based on the DETR and MaskFormer frameworks. This model includes the following different modules:
- Backbone: Feature extractor, used to extract image features.
- Neck: Construct multi-scale features to handle multi-scale objects.
- Object Query: Query object, used to represent each entity in the scene, including foreground objects and background objects.
- Decoder: Decoder, used to gradually optimize Object Query and corresponding features.
- End-to-End Training: The design based on Object Query can achieve end-to-end optimization.
Based on this meta-architecture, existing methods can be divided into the following five different directions for optimization and adjustment according to tasks, as shown in Figure 4, each Directions have several different sub-directions.
Figure 4. Summary and comparison of Transformer-Based Segmentation methods
- Better feature expression learning, Representation Learning. Strong visual feature representation will always lead to better segmentation results. This article divides related work into three aspects: better visual Transformer design, hybrid CNN/Transformer/MLP, and self-supervised learning.
- Method design on the decoder side, Interaction Design in Decoder. This chapter reviews the new Transformer decoder design. This paper divides the decoder design into two groups: one is used to improve the cross-attention design in image segmentation, and the other is used to improve the spatio-temporal cross-attention design in video segmentation. The former focuses on designing a better decoder to improve upon the one in the original DETR. The latter extends query object-based object detectors and segmenters to the video domain for video object detection (VOD), video instance segmentation (VIS), and video pixel segmentation (VPS), focusing on modeling temporal consistency and correlation. sex.
- #Try to Optimizing Object Query from the perspective of query object optimization. Compared with Faster-RCNN, DETR requires a longer convergence timetable. Due to the key role of query objects, some existing methods have been studied to speed up training and improve performance. According to the method of object query, this paper divides the following literature into two aspects: adding location information and using additional supervision. Location information provides clues for fast training sampling of query features. Additional supervision focuses on designing specific loss functions in addition to the DETR default loss function.
- Use query objects to associate features and instances, Using Query For Association. Benefiting from the simplicity of query objects, multiple recent studies have used them as correlation tools to solve downstream tasks. There are two main usages: one is instance-level association, and the other is task-level association. The former uses the idea of instance discrimination to solve instance-level matching problems in videos, such as video segmentation and tracking. The latter uses query objects to bridge different subtasks to achieve efficient multi-task learning.
- Multi-modal conditional query object generation, Conditional Query Generation. This chapter mainly focuses on multi-modal segmentation tasks. Conditional query query objects are mainly used to handle cross-modal and cross-image feature matching tasks. Depending on the task input conditions, the decoder head uses different queries to obtain the corresponding segmentation masks. According to the sources of different inputs, this paper divides these works into two aspects: language features and image features. These methods are based on the strategy of fusing query objects with different model features, and have achieved good results in multiple multi-modal segmentation tasks and few-shot segmentation.
Figure 5 shows some representative work comparisons in these five different directions. For more specific method details and comparisons, please refer to the content of the paper.
Picture
Figure 5. Summary and comparison of Transformer-based segmentation and representativeness detection methods
Summary and comparison of methods in related research fields
This article also explores several related fields: 1. Point cloud segmentation method based on Transformer. 2. Vision and multi-modal large model tuning. 3. Research on domain-related segmentation models, including domain transfer learning and domain generalization learning. 4. Efficient semantic segmentation: unsupervised and weakly supervised segmentation models. 5. Class-independent segmentation and tracking. 6. Medical image segmentation.
Picture
Figure 6. Summary and comparison of Transformer-based methods in related research fields
Comparison of experimental results of different methods
Figure 7. Benchmark experiment of semantic segmentation data set
Figure 8. Benchmark experiment on panoramic segmentation data set
This article also uniformly uses the same experimental design conditions to compare the results of several representative works on panoramic segmentation and semantic segmentation on multiple data sets. It was found that when using the same training strategy and encoder, the gap between method performance will narrow.
In addition, this article also compares the results of recent Transformer-based segmentation methods on multiple different data sets and tasks. (Semantic segmentation, instance segmentation, panoramic segmentation, and corresponding video segmentation tasks)
Future Direction
In addition, this article also gives some Analysis of some possible future research directions. Three different directions are given here as examples.
- UpdateAdd a general and unified segmentation model. Using the Transformer structure to unify different segmentation tasks is a trend. Recent research uses query object-based Transformers to perform different segmentation tasks under one architecture. One possible research direction is to unify image and video segmentation tasks on various segmentation datasets through one model. These general models can achieve versatile and robust segmentation in various scenarios. For example, detecting and segmenting rare categories in various scenarios helps robots make better decisions.
- Segmentation model combined with visual reasoning. Visual reasoning requires the robot to understand the connections between objects in the scene, and this understanding plays a key role in motion planning. Previous research has explored using segmentation results as input to visual reasoning models for various applications such as object tracking and scene understanding. Joint segmentation and visual reasoning can be a promising direction, with mutually beneficial potential for both segmentation and relational classification. By incorporating visual reasoning into the segmentation process, researchers can leverage the power of reasoning to improve segmentation accuracy, while segmentation results can also provide better input for visual reasoning.
- Research on segmentation model of continuous learning. Existing segmentation methods are usually benchmarked on closed-world datasets with a predefined set of categories, i.e., it is assumed that the training and test samples have the same categories known in advance and feature space. However, real-world scenarios are often open-world and non-stable, and new categories of data may constantly emerge. For example, in autonomous vehicles and medical diagnostics, unanticipated situations may suddenly arise. There is a clear gap between the performance and capabilities of existing methods in real-world and closed-world scenarios. Therefore, it is hoped that new concepts can be gradually and continuously incorporated into the existing knowledge base of the segmentation model, so that the model can engage in lifelong learning.
For more research directions, please refer to the original paper.
The above is the detailed content of NTU and Shanghai AI Lab compiled 300+ papers: the latest review of visual segmentation based on Transformer is released. For more information, please follow other related articles on the PHP Chinese website!
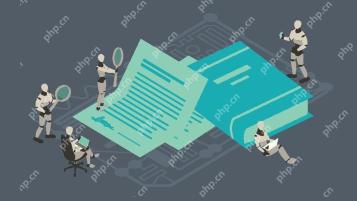
The legal tech revolution is gaining momentum, pushing legal professionals to actively embrace AI solutions. Passive resistance is no longer a viable option for those aiming to stay competitive. Why is Technology Adoption Crucial? Legal professional
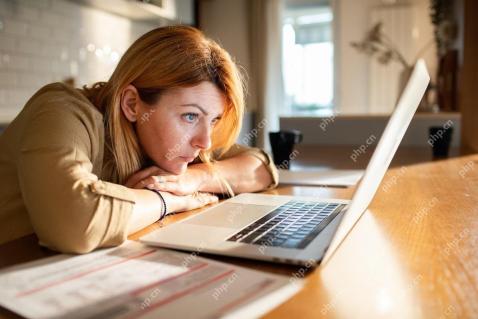
Many assume interactions with AI are anonymous, a stark contrast to human communication. However, AI actively profiles users during every chat. Every prompt, every word, is analyzed and categorized. Let's explore this critical aspect of the AI revo
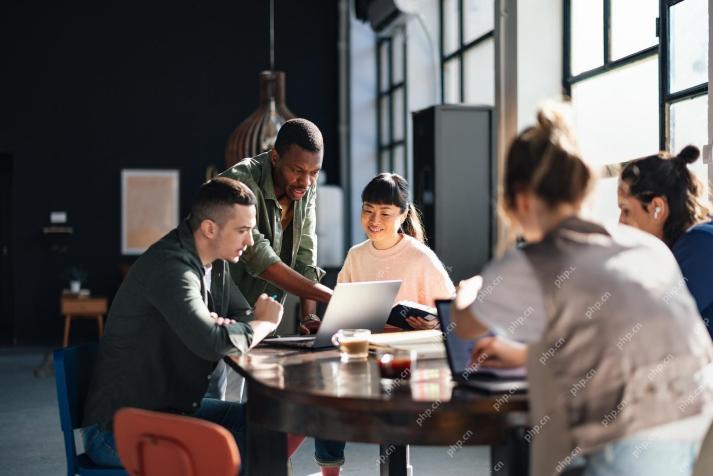
A successful artificial intelligence strategy cannot be separated from strong corporate culture support. As Peter Drucker said, business operations depend on people, and so does the success of artificial intelligence. For organizations that actively embrace artificial intelligence, building a corporate culture that adapts to AI is crucial, and it even determines the success or failure of AI strategies. West Monroe recently released a practical guide to building a thriving AI-friendly corporate culture, and here are some key points: 1. Clarify the success model of AI: First of all, we must have a clear vision of how AI can empower business. An ideal AI operation culture can achieve a natural integration of work processes between humans and AI systems. AI is good at certain tasks, while humans are good at creativity and judgment
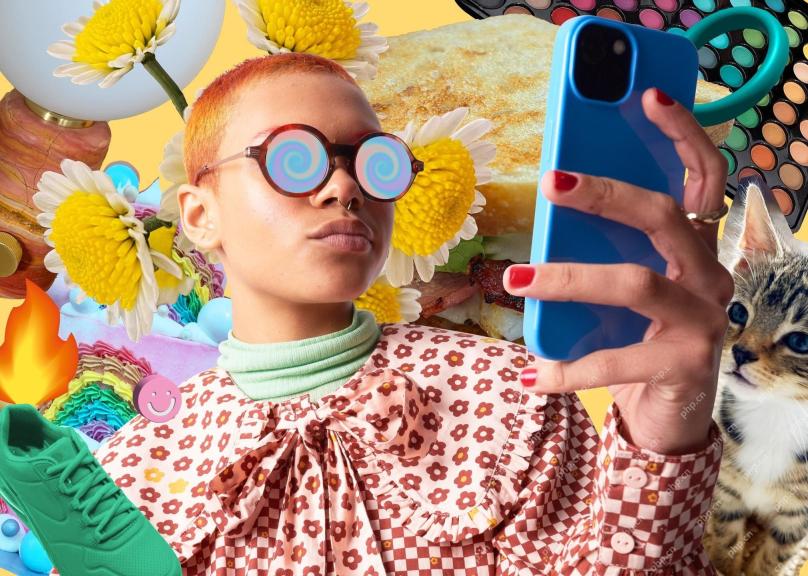
Meta upgrades AI assistant application, and the era of wearable AI is coming! The app, designed to compete with ChatGPT, offers standard AI features such as text, voice interaction, image generation and web search, but has now added geolocation capabilities for the first time. This means that Meta AI knows where you are and what you are viewing when answering your question. It uses your interests, location, profile and activity information to provide the latest situational information that was not possible before. The app also supports real-time translation, which completely changed the AI experience on Ray-Ban glasses and greatly improved its usefulness. The imposition of tariffs on foreign films is a naked exercise of power over the media and culture. If implemented, this will accelerate toward AI and virtual production
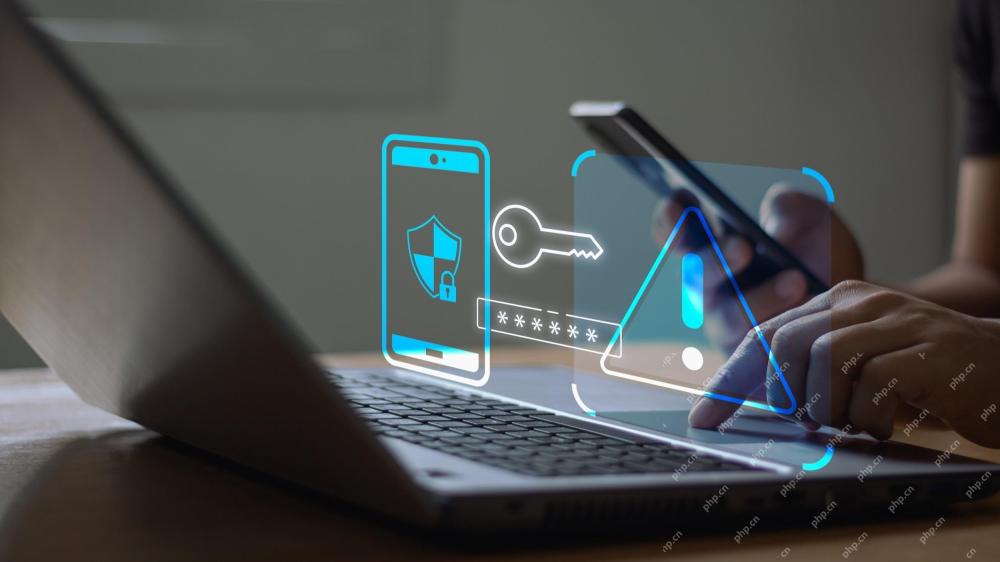
Artificial intelligence is revolutionizing the field of cybercrime, which forces us to learn new defensive skills. Cyber criminals are increasingly using powerful artificial intelligence technologies such as deep forgery and intelligent cyberattacks to fraud and destruction at an unprecedented scale. It is reported that 87% of global businesses have been targeted for AI cybercrime over the past year. So, how can we avoid becoming victims of this wave of smart crimes? Let’s explore how to identify risks and take protective measures at the individual and organizational level. How cybercriminals use artificial intelligence As technology advances, criminals are constantly looking for new ways to attack individuals, businesses and governments. The widespread use of artificial intelligence may be the latest aspect, but its potential harm is unprecedented. In particular, artificial intelligence
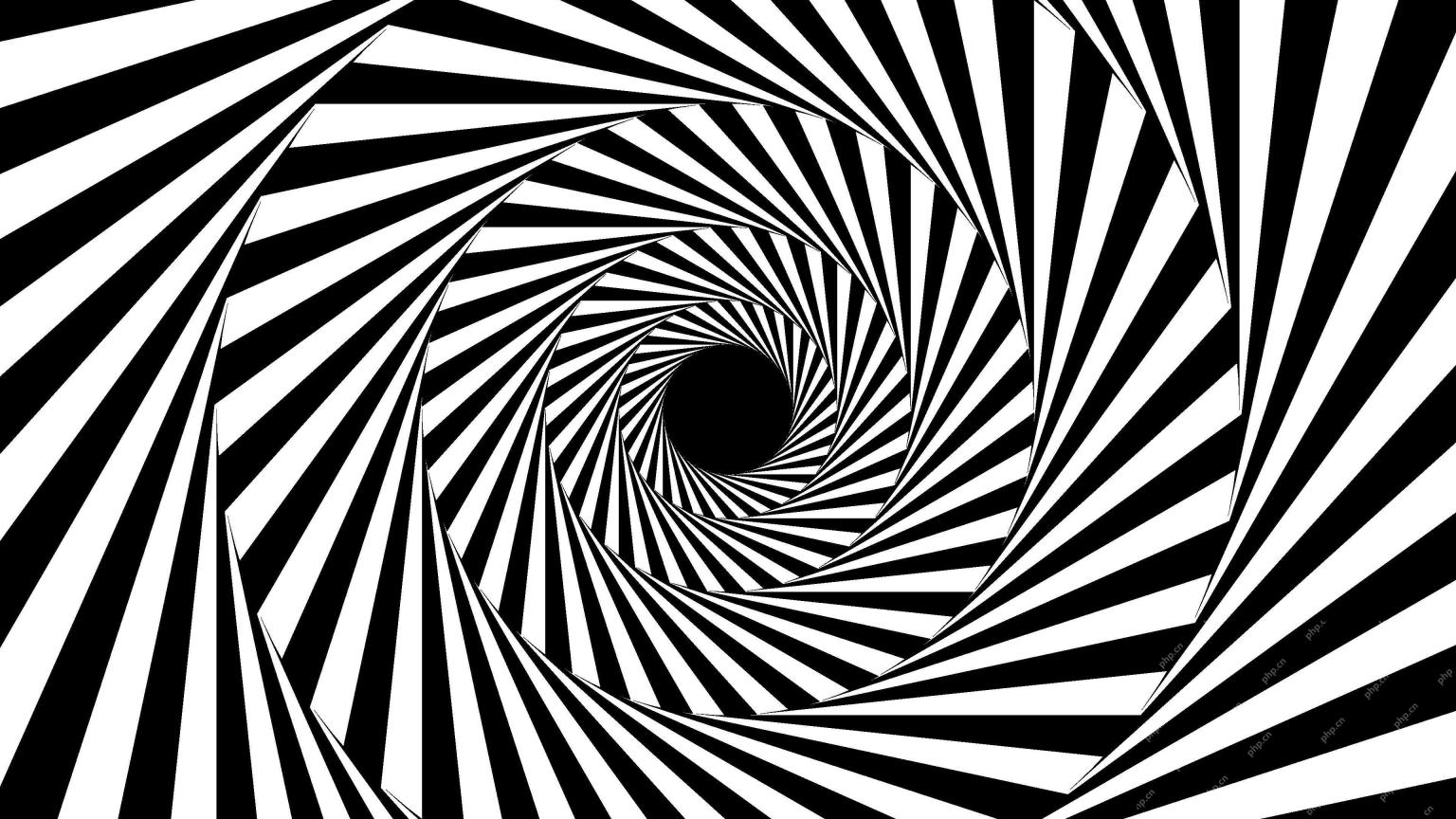
The intricate relationship between artificial intelligence (AI) and human intelligence (NI) is best understood as a feedback loop. Humans create AI, training it on data generated by human activity to enhance or replicate human capabilities. This AI
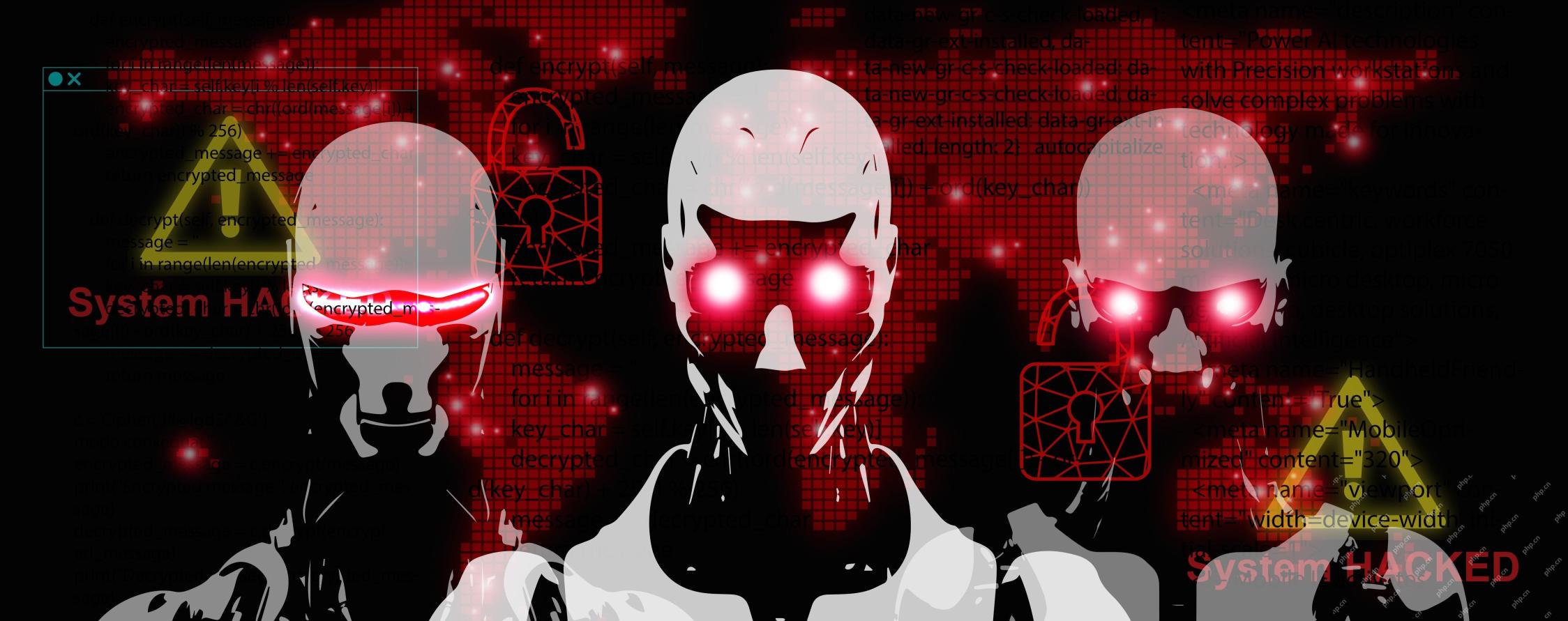
Anthropic's recent statement, highlighting the lack of understanding surrounding cutting-edge AI models, has sparked a heated debate among experts. Is this opacity a genuine technological crisis, or simply a temporary hurdle on the path to more soph
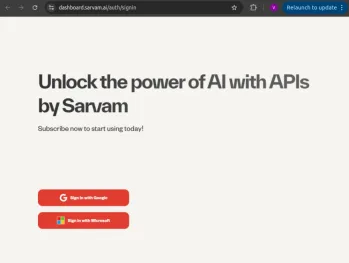
India is a diverse country with a rich tapestry of languages, making seamless communication across regions a persistent challenge. However, Sarvam’s Bulbul-V2 is helping to bridge this gap with its advanced text-to-speech (TTS) t


Hot AI Tools

Undresser.AI Undress
AI-powered app for creating realistic nude photos

AI Clothes Remover
Online AI tool for removing clothes from photos.

Undress AI Tool
Undress images for free

Clothoff.io
AI clothes remover

Video Face Swap
Swap faces in any video effortlessly with our completely free AI face swap tool!

Hot Article

Hot Tools

SAP NetWeaver Server Adapter for Eclipse
Integrate Eclipse with SAP NetWeaver application server.

DVWA
Damn Vulnerable Web App (DVWA) is a PHP/MySQL web application that is very vulnerable. Its main goals are to be an aid for security professionals to test their skills and tools in a legal environment, to help web developers better understand the process of securing web applications, and to help teachers/students teach/learn in a classroom environment Web application security. The goal of DVWA is to practice some of the most common web vulnerabilities through a simple and straightforward interface, with varying degrees of difficulty. Please note that this software

PhpStorm Mac version
The latest (2018.2.1) professional PHP integrated development tool

SublimeText3 Chinese version
Chinese version, very easy to use

Notepad++7.3.1
Easy-to-use and free code editor
