AI and ML are shifting from business terms to broader enterprise applications. Efforts around strategy and adoption are reminiscent of the cycles and inflection points in enterprise cloud strategy, when enterprises no longer had the choice to move to the cloud, only the question of when and how to move.
Implementation strategies for artificial intelligence and machine learning follow the same evolving pattern as companies build their approaches. In this article, we’ll discuss how to maximize the potential of artificial intelligence and machine learning.
According to a research report, nearly two-thirds of enterprise technology decision-makers have already, are currently, or plan to expand the application of artificial intelligence. This work and effort is driven by enterprise data lakes within enterprises that have largely sat idle due to compliance and low-cost storage, leveraging these rich repositories to allow AI to answer the questions we are not asking , or may not know what questions to ask.
Spending on AI-centric systems is expected to exceed $300 billion by 2026, and companies across industries will continue to adopt AI and machine learning technologies to transform their core processes and operations in the coming years. model to leverage machine learning systems to enhance operations and improve cost efficiencies. As business leaders begin to develop plans and strategies for how to make the most of this technology, they must remember that the path to adopting artificial intelligence and machine learning is a journey, not a race.
How to successfully implement artificial intelligence?
1. Clearly define use cases
For business leaders and their project managers, first take the time to clearly define and clarify what they want The specific problem or challenge that AI solves is important because the more specific the goals, the greater the chance of success in their implementation of AI.
2. Verify data availability
Once the use cases are clearly defined, the next step is to ensure that existing processes and systems can capture and track the data needed to perform the required analysis.
A lot of time and effort is spent on data ingestion and curation, so businesses must ensure they capture a sufficient amount of the right data, with the right variables or characteristics, such as age, gender or ethnicity. When organizations prioritize data governance programs, they should keep in mind the importance of data quality and quantity for successful outcomes.
3. Carry out basic data mining
It may be tempting for an enterprise to dive headfirst into a model building exercise, but it is crucial that it begins with a quick data exploration exercise. to validate their data assumptions and understanding. By leveraging an organization’s subject matter expertise and business insights, we can determine whether the data is telling the right story.
Such an exercise will also help businesses understand what important variable characteristics should or could be, and what kind of data classification should be created as input to any potential model.
4. Bring together a diverse and inclusive engineering team
To ensure the success of AI models, management teams need to bring together diverse ideas and perspectives. This requires hiring and including staff from as wide a cross-section of the population as possible, taking into account demographic and social factors such as gender, race and neurodiversity.
Skills gaps remain prominent across the tech industry and business, but recruiting and retaining employees of all backgrounds can mitigate this and ensure AI models are as inclusive and actionable as possible. Take the time to benchmark against industry and identify where more representation is needed.
5. Define the model building method
Instead of focusing on the ultimate goal that the hypothesis should achieve, it is better to focus on the hypothesis itself. Running tests to determine which variables or features are most important will validate assumptions and improve their execution.
Involving diverse business and domain experts is critical as their ongoing feedback plays an important role in validating and ensuring consensus among all stakeholders. In fact, since the success of any machine learning model depends on successful feature engineering, subject matter experts are always more valuable than algorithms when it comes to obtaining better features.
6. Define model verification methods
By defining performance indicators, the results of different algorithms can be evaluated, compared, and analyzed to further improve a specific model. For example, classification accuracy would be a good performance measure when dealing with classification use cases.
To train and evaluate the algorithm, the data needs to be divided into a training set and a test set. Depending on the complexity of the algorithm, this may be as simple as choosing a random split of the data, such as 60% for training and 40% for testing, or it may involve a more complex sampling process.
As with testing hypotheses, business and domain experts should be involved to validate the findings and ensure everything is moving in the right direction.
7. Automation and production promotion
After the model is built and verified, it must be put into production. Starting with a limited rollout over a few weeks or months, business users can provide ongoing feedback on model behavior and results, which can then be rolled out to a wider audience.
To disseminate results to the appropriate audience, appropriate tools and platforms should be selected to automate data collection and corresponding systems should be established. The platform should provide multiple interfaces to meet the different levels of knowledge needs of enterprise end users. For example, a business analyst may want to perform further analysis based on model results, while a casual end user may only want to interact with the data through dashboards and visualizations.
8. Continue to update the model
Once a model is released and deployed for use, it must be continuously monitored, because by understanding its effectiveness, the enterprise will be able to update the model as needed.
Models can become outdated for a number of reasons. Changes in the market may lead to changes in the company itself and its business model. Models are built on historical data in order to predict future outcomes, but as market dynamics deviate from the way a company has always done business, the model's performance can deteriorate. Therefore, it is important to remember what processes must be followed to ensure that the model remains up to date.
Artificial intelligence is rapidly moving from hype to reality in the enterprise space, with a significant impact on business operations and efficiency. Taking the time to develop an implementation plan now will put the business in a better position to reap further benefits.
The above is the detailed content of How to successfully implement artificial intelligence?. For more information, please follow other related articles on the PHP Chinese website!
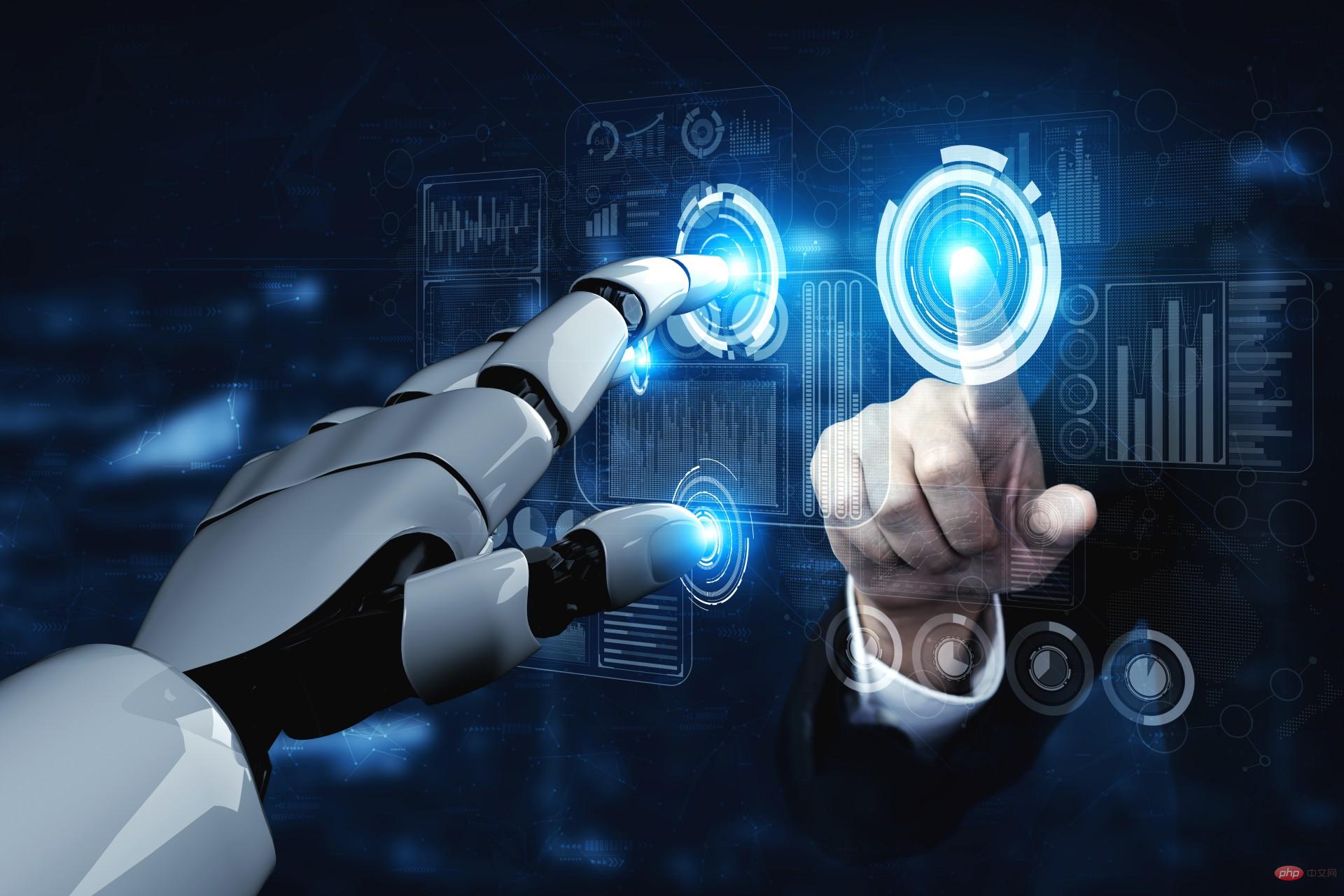
机器学习是一个不断发展的学科,一直在创造新的想法和技术。本文罗列了2023年机器学习的十大概念和技术。 本文罗列了2023年机器学习的十大概念和技术。2023年机器学习的十大概念和技术是一个教计算机从数据中学习的过程,无需明确的编程。机器学习是一个不断发展的学科,一直在创造新的想法和技术。为了保持领先,数据科学家应该关注其中一些网站,以跟上最新的发展。这将有助于了解机器学习中的技术如何在实践中使用,并为自己的业务或工作领域中的可能应用提供想法。2023年机器学习的十大概念和技术:1. 深度神经网
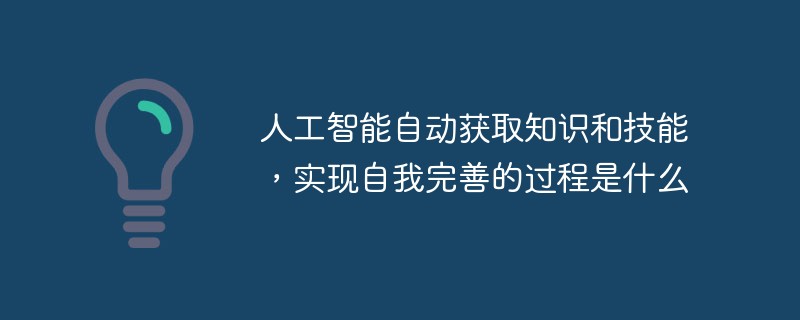
实现自我完善的过程是“机器学习”。机器学习是人工智能核心,是使计算机具有智能的根本途径;它使计算机能模拟人的学习行为,自动地通过学习来获取知识和技能,不断改善性能,实现自我完善。机器学习主要研究三方面问题:1、学习机理,人类获取知识、技能和抽象概念的天赋能力;2、学习方法,对生物学习机理进行简化的基础上,用计算的方法进行再现;3、学习系统,能够在一定程度上实现机器学习的系统。

本文将详细介绍用来提高机器学习效果的最常见的超参数优化方法。 译者 | 朱先忠审校 | 孙淑娟简介通常,在尝试改进机器学习模型时,人们首先想到的解决方案是添加更多的训练数据。额外的数据通常是有帮助(在某些情况下除外)的,但生成高质量的数据可能非常昂贵。通过使用现有数据获得最佳模型性能,超参数优化可以节省我们的时间和资源。顾名思义,超参数优化是为机器学习模型确定最佳超参数组合以满足优化函数(即,给定研究中的数据集,最大化模型的性能)的过程。换句话说,每个模型都会提供多个有关选项的调整“按钮
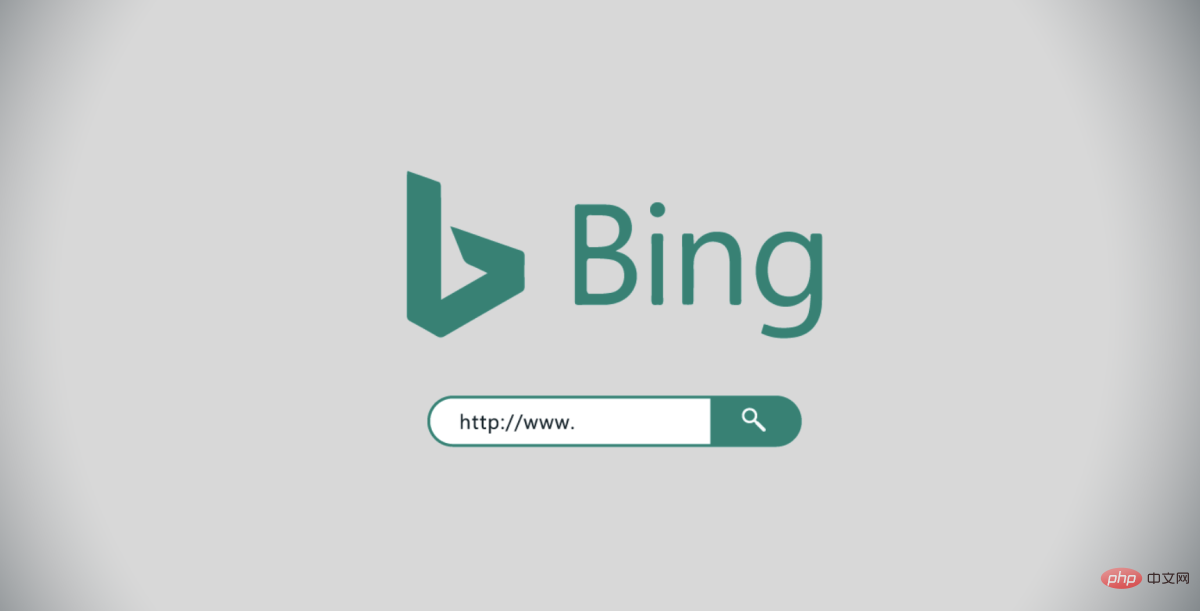
截至3月20日的数据显示,自微软2月7日推出其人工智能版本以来,必应搜索引擎的页面访问量增加了15.8%,而Alphabet旗下的谷歌搜索引擎则下降了近1%。 3月23日消息,外媒报道称,分析公司Similarweb的数据显示,在整合了OpenAI的技术后,微软旗下的必应在页面访问量方面实现了更多的增长。截至3月20日的数据显示,自微软2月7日推出其人工智能版本以来,必应搜索引擎的页面访问量增加了15.8%,而Alphabet旗下的谷歌搜索引擎则下降了近1%。这些数据是微软在与谷歌争夺生
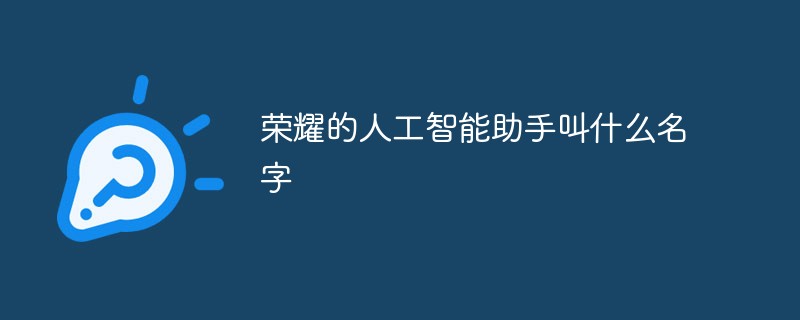
荣耀的人工智能助手叫“YOYO”,也即悠悠;YOYO除了能够实现语音操控等基本功能之外,还拥有智慧视觉、智慧识屏、情景智能、智慧搜索等功能,可以在系统设置页面中的智慧助手里进行相关的设置。
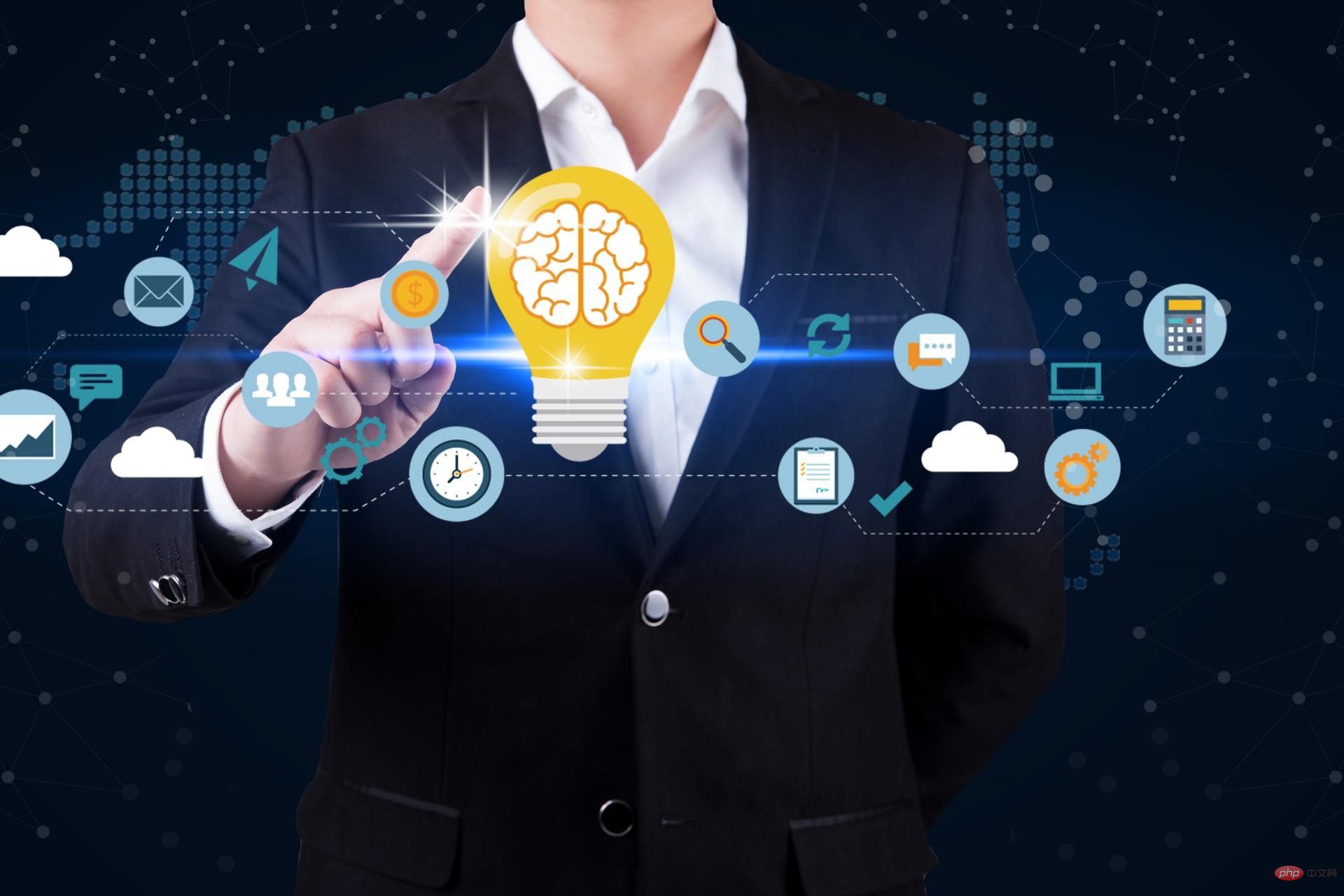
阅读论文可以说是我们的日常工作之一,论文的数量太多,我们如何快速阅读归纳呢?自从ChatGPT出现以后,有很多阅读论文的服务可以使用。其实使用ChatGPT API非常简单,我们只用30行python代码就可以在本地搭建一个自己的应用。 阅读论文可以说是我们的日常工作之一,论文的数量太多,我们如何快速阅读归纳呢?自从ChatGPT出现以后,有很多阅读论文的服务可以使用。其实使用ChatGPT API非常简单,我们只用30行python代码就可以在本地搭建一个自己的应用。使用 Python 和 C
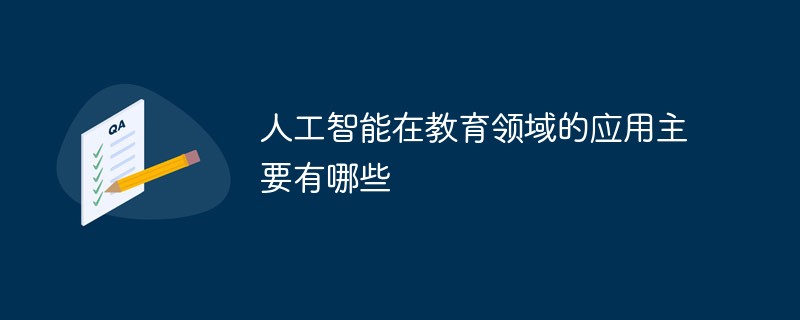
人工智能在教育领域的应用主要有个性化学习、虚拟导师、教育机器人和场景式教育。人工智能在教育领域的应用目前还处于早期探索阶段,但是潜力却是巨大的。
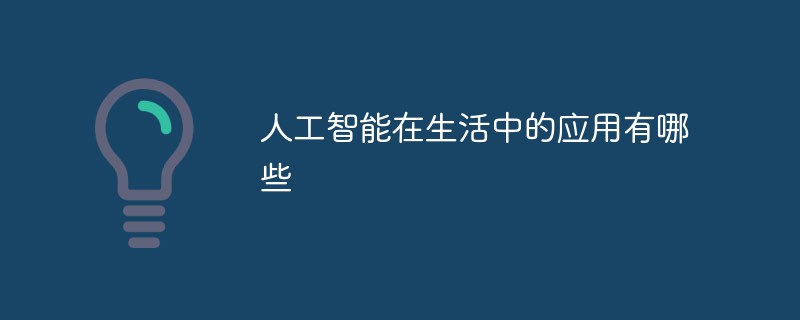
人工智能在生活中的应用有:1、虚拟个人助理,使用者可通过声控、文字输入的方式,来完成一些日常生活的小事;2、语音评测,利用云计算技术,将自动口语评测服务放在云端,并开放API接口供客户远程使用;3、无人汽车,主要依靠车内的以计算机系统为主的智能驾驶仪来实现无人驾驶的目标;4、天气预测,通过手机GPRS系统,定位到用户所处的位置,在利用算法,对覆盖全国的雷达图进行数据分析并预测。


Hot AI Tools

Undresser.AI Undress
AI-powered app for creating realistic nude photos

AI Clothes Remover
Online AI tool for removing clothes from photos.

Undress AI Tool
Undress images for free

Clothoff.io
AI clothes remover

AI Hentai Generator
Generate AI Hentai for free.

Hot Article

Hot Tools
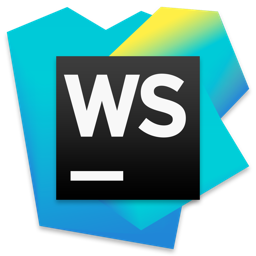
WebStorm Mac version
Useful JavaScript development tools

SAP NetWeaver Server Adapter for Eclipse
Integrate Eclipse with SAP NetWeaver application server.

MantisBT
Mantis is an easy-to-deploy web-based defect tracking tool designed to aid in product defect tracking. It requires PHP, MySQL and a web server. Check out our demo and hosting services.

SublimeText3 Chinese version
Chinese version, very easy to use
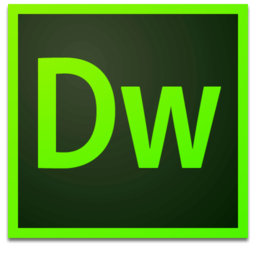
Dreamweaver Mac version
Visual web development tools