


A US$38 billion data giant wants to launch an 'AI' revolution in enterprises
Author | Wan Chen, Li Yuan
Editor | Jingyu
On June 28, local time, Databricks, a well-known American data platform, held its own annual conference, the Data and Artificial Intelligence Summit. At the meeting, Databricks announced a series of important new products such as LakehouseIQ, Lakehouse AI, Databricks Marketplace, and Lakehouse Apps.
Whether it is the name of the summit or the naming of new products, it can be seen that this well-known data platform is taking advantage of the big language model to accelerate its transformation to AI.
Databricks CEO Ali Ghodsi said the inclusiveness of data and AI|Databricks
"What Databricks wants to achieve is "data inclusiveness" and AI inclusiveness. The former allows data to reach every employee, and the latter allows AI to enter every product. Databricks CEO Ali Ghodsi announced the team's mission in his speech .
Just before the conference started, Databricks had just announced the acquisition of MosaicML, a new force in the AI field, for US$1.3 billion, setting a current acquisition record in the AI field, which shows the company's strength and determination in AI transformation.
Liu Qi, founder and CEO of PingCAP, who is participating in the meeting ahead, told Geek Park that the Databricks platform has just launched enterprise-level AI applications, and more than 1,500 companies have already trained models on it. "The numbers exceed expectations." At the same time, he believes that Databricks’ previous accumulation in data AI allowed the company to quickly add new products based on the previous platform when AI became popular, and to quickly provide services related to large models.
"The most critical thing is speed." Liu Qi said that in the era of large models, how to integrate large models with existing products faster and solve users' pain points may be the biggest challenge for all data companies at the moment. It is also the biggest opportunity.
Talking points
- Through the upgrade of the interactive interface, ordinary people who are not data analysts can directly use natural language to query and analyze data.
- It will become easier and easier for enterprises to deploy large models to cloud databases, and it will also become easier to directly use finished large model tools to analyze data.
- With the advancement of AI, the value of data will become higher and higher, and the potential of data will be further released.
Database welcomes natural language interaction
Databricks released a new LakehouseIQ tool at the conference, which was hailed as an "artifact". LakehouseIQ carries one of Databricks' biggest recent efforts - the universalization of data analysis. That is, ordinary people who do not master Python and SQL can easily access company data and conduct data analysis using natural language.
To achieve this goal, LakehouseIQ is designed as a collection of functions that can be used by both ordinary end users and developers, with different functions designed for different users.
LakehouseIQ Product Picture|Databricks
For developers, LakehouseIQ in Notebooks has been released. In this feature, LakehouseIQ can use large language models to help developers complete, generate and interpret code, as well as perform code repair, debugging and report generation.
For ordinary non-programmers, Databricks provides an interface that can be directly interacted with natural language. It is driven by a large language model and can directly use natural language to search and query data. At the same time, this function is integrated with Unity Catalog, allowing companies to control access to data searches and queries, and only return data that the questioner is authorized to view.
Since the launch of large models, using natural language to query and analyze data has actually been a hot topic in the direction of data analysis, and many companies have made plans in this direction. Including Databricks’ old rival Snowflake, the just-announced Document AI feature also focuses on this direction.
LakehouseIQ Natural Language Query Interface|Databricks
However, Databricks claims that LakehouseIQ is functionally superior. It points out that general purpose big language models have limitations in understanding specific customer data, internal terminology and usage patterns. Databricks’ technology leverages customers’ own schemas, documents, queries, popularity, threads, notebooks and business intelligence dashboards to gain intelligence and answer more queries.
There is another difference between the functions of Databricks and Snowflake. The Document AI function of the Snowflake platform is limited to querying unstructured data in documents, while LakehouseIQ is suitable for structured Lakehouse data and code.
02
From machine learning to AI
The similarities between Databricks and Snowflake at the launch don’t end there.
In this conference, Databricks released Databricks Marketplace and Lakehouse AI, which are completely consistent with the focus of Snowflake's two-day conference. Both focus on deploying large language models into database environments.
In Databricks’ vision, Databricks can not only assist customers in deploying large models in the future, but also provide finished large model tools.
Databricks used to have the Databricks Machine Learning brand. At this press conference, Databricks fully repositioned its brand and upgraded it to Lakehouse AI, focusing on assisting customers in deploying large models.
Databricks Marketplace is now available on Databricks. In the Databricks Marketplace, users can access a large, screened collection of open source language models, including MPT-7B, Falcon-7B, and Stable Diffusion, and can also discover and obtain data sets and data assets. Lakehouse AI also provides some large language model operations (LLMOps) functions.
Lakehouse AI Architecture Diagram|Databricks
Snowflake is also actively deploying this, and its similar functions are provided by Nvidia NeMo, Nvidia AI Enterprise, Dataiku and John Snow Labs (cooperation with Nvidia is one of the highlights of the Snowflake conference, see Geek Park's report) .
Snowflake and Databricks have differences in helping customers deploy large models. Snowflake has chosen to actively engage with partners, while Databricks has sought to add the functionality as a native feature of its core platform.
In terms of providing finished tools, Databricks announced that the Databricks Marketplace will also provide Lakehouse Apps in the future. Lakehouse Apps will run directly on customers' Databricks instances, where they can integrate with customers' data, consume and extend Databricks services, and enable users to interact through a single sign-on experience. Data never needs to leave the customer's instance, and there are no data movement and security/access issues.
This is completely consistent with Snowflake’s products in terms of naming and functionality. Snowflake's similar Snowflake Marketplace and Snowflake Native App are already online and are one of the highlights of its launch. Bloomberg announced a Data License Plus (DL) APP provided by Bloomberg at the Snowflake conference, which allows customers to configure a ready-to-use environment in the cloud in minutes, with fully modeled Bloomberg subscription data. and ESG content from multiple vendors.
03
Data platform welcomes new changes
At the opening keynote speech, Databricks announced a number: in the past 30 days, more than 1,500 customers have trained Transformer models on the Databricks platform.
When talking about this impressive number, PingCAP Liu Qi believes that this shows that enterprises are applying AI much faster than expected. “The application model does not necessarily have to train the model, so if the trained model There are 1,500 companies, so the number of applications must be much larger than this (number)."
Another point of view is that this shows that Databricks’ strategic layout in the field of AI is quite comprehensive. It is now more than just a data warehouse or data lake. Now it also provides: AI training, AI serving, model management, etc. 』
Ali Ghodsi uses the revolution of computing and the Internet to compare the transformation of large models in machine learning|Databricks
In other words, the underlying model can be trained on the Databricks platform, and the lowest-level model can be trained by simply adjusting the parameters. For the AI services required on top of this model, Databricks has also laid out the corresponding infrastructure - today it released vector search and feature store.
Databricks is fully upgraded to large models.
In the past, Databricks has accumulated a lot in AI, such as using small models to improve efficiency and reduce latency in building indexes, querying data, and predicting workloads. However, the ability to make up for large models at such a fast pace still surprises many people.
Before the AI layout fully displayed at today’s summit, Databricks acquired Okera (AI data governance), launched its own open source large model Dolly 2.0, and acquired MosaicML for US$1.3 billion. A series of actions were completed in one go.
In this regard, Howie, a teacher from Silicon Valley, believes that it can be clearly seen from the two conferences of Databricks and Snowflake: the founders of the two companies believe that the actions they have taken based on databases and data lakes will face them next. Fundamental changes. The way they were doing it a year ago won't work in the next few years.
Correspondingly, the ability to quickly complete large models also means that the incremental market brought by large models can be obtained.
Liu Qi believes that the emergence of large models has triggered many new demands that did not exist before there were no large models. Without data support, the model will not be able to function, especially in terms of differentiation. If everyone is a big model, then there may be no difference between you and others. 』
But compared to large models, the audience at the summit seemed to pay more attention to small models because of several advantages of small models: speed, cost, and safety. Liu Qi said that based on his own unique data, he can make differentiated models. The model must be small enough to meet these three requirements: cheap enough, fast enough, and safe enough.
It is worth noting that both Databricks and Snowflake recently announced their revenue data, and the annual revenue growth of the platform is more than 60%. This growth rate is reflected in the growing focus on data against the backdrop of a slowdown in software spending across the market. With the emergence of large-scale models, the value of data was highlighted at this Databricks Summit with the theme of data plus AI.
With the introduction of large-scale models, automatic generation of data becomes possible, and the amount of data is expected to increase exponentially. How to easily access data, how to support different data formats, and how to mine the value behind the data will become increasingly frequent needs.
On the other hand, many companies today are still exploring and waiting to integrate large models into enterprise software, but considering security, privacy, and cost, there are still very few who dare to use it directly. Once large models are deployed directly to enterprise data without moving data, the threshold for deploying large models will be further lowered, and the amount and speed of data consumption will be further released.
The above is the detailed content of A US$38 billion data giant wants to launch an 'AI' revolution in enterprises. For more information, please follow other related articles on the PHP Chinese website!
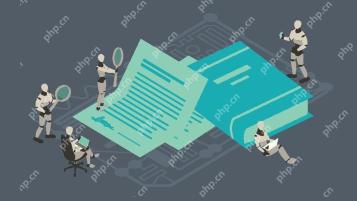
The legal tech revolution is gaining momentum, pushing legal professionals to actively embrace AI solutions. Passive resistance is no longer a viable option for those aiming to stay competitive. Why is Technology Adoption Crucial? Legal professional
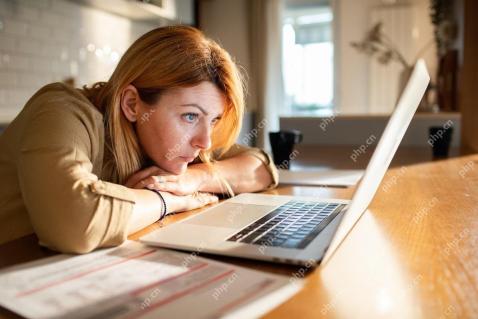
Many assume interactions with AI are anonymous, a stark contrast to human communication. However, AI actively profiles users during every chat. Every prompt, every word, is analyzed and categorized. Let's explore this critical aspect of the AI revo
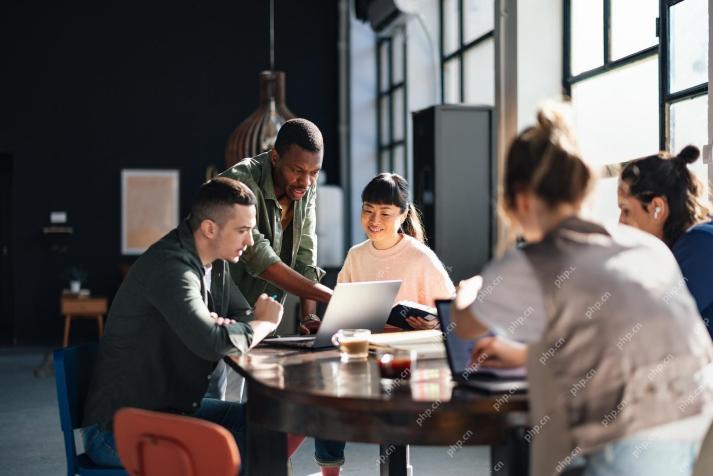
A successful artificial intelligence strategy cannot be separated from strong corporate culture support. As Peter Drucker said, business operations depend on people, and so does the success of artificial intelligence. For organizations that actively embrace artificial intelligence, building a corporate culture that adapts to AI is crucial, and it even determines the success or failure of AI strategies. West Monroe recently released a practical guide to building a thriving AI-friendly corporate culture, and here are some key points: 1. Clarify the success model of AI: First of all, we must have a clear vision of how AI can empower business. An ideal AI operation culture can achieve a natural integration of work processes between humans and AI systems. AI is good at certain tasks, while humans are good at creativity and judgment
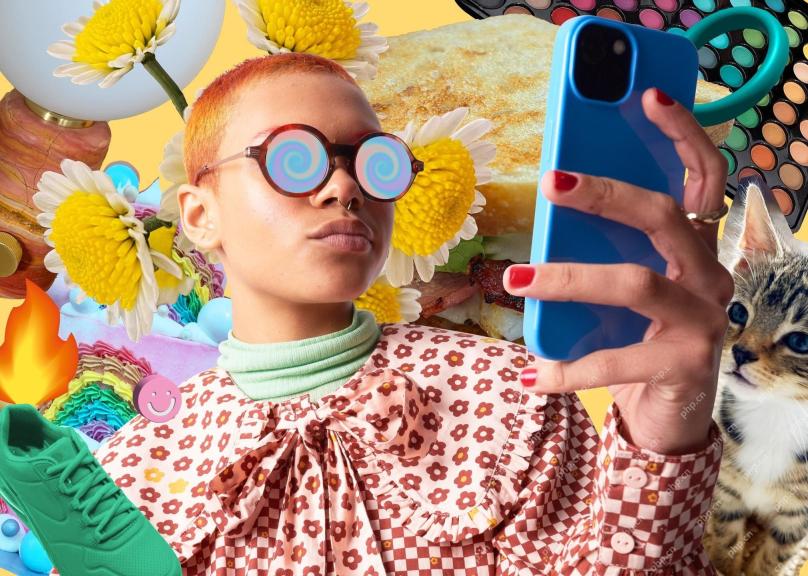
Meta upgrades AI assistant application, and the era of wearable AI is coming! The app, designed to compete with ChatGPT, offers standard AI features such as text, voice interaction, image generation and web search, but has now added geolocation capabilities for the first time. This means that Meta AI knows where you are and what you are viewing when answering your question. It uses your interests, location, profile and activity information to provide the latest situational information that was not possible before. The app also supports real-time translation, which completely changed the AI experience on Ray-Ban glasses and greatly improved its usefulness. The imposition of tariffs on foreign films is a naked exercise of power over the media and culture. If implemented, this will accelerate toward AI and virtual production
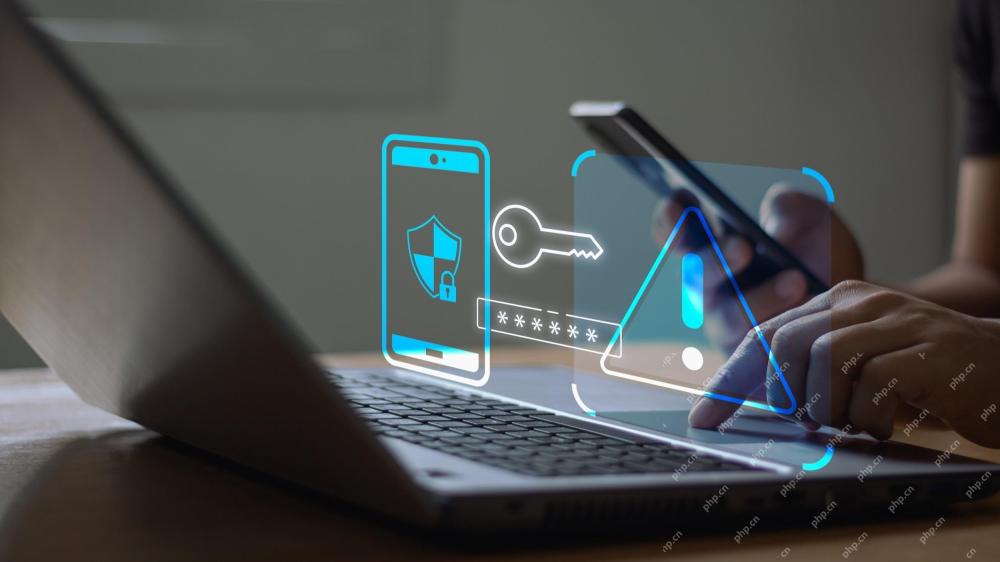
Artificial intelligence is revolutionizing the field of cybercrime, which forces us to learn new defensive skills. Cyber criminals are increasingly using powerful artificial intelligence technologies such as deep forgery and intelligent cyberattacks to fraud and destruction at an unprecedented scale. It is reported that 87% of global businesses have been targeted for AI cybercrime over the past year. So, how can we avoid becoming victims of this wave of smart crimes? Let’s explore how to identify risks and take protective measures at the individual and organizational level. How cybercriminals use artificial intelligence As technology advances, criminals are constantly looking for new ways to attack individuals, businesses and governments. The widespread use of artificial intelligence may be the latest aspect, but its potential harm is unprecedented. In particular, artificial intelligence
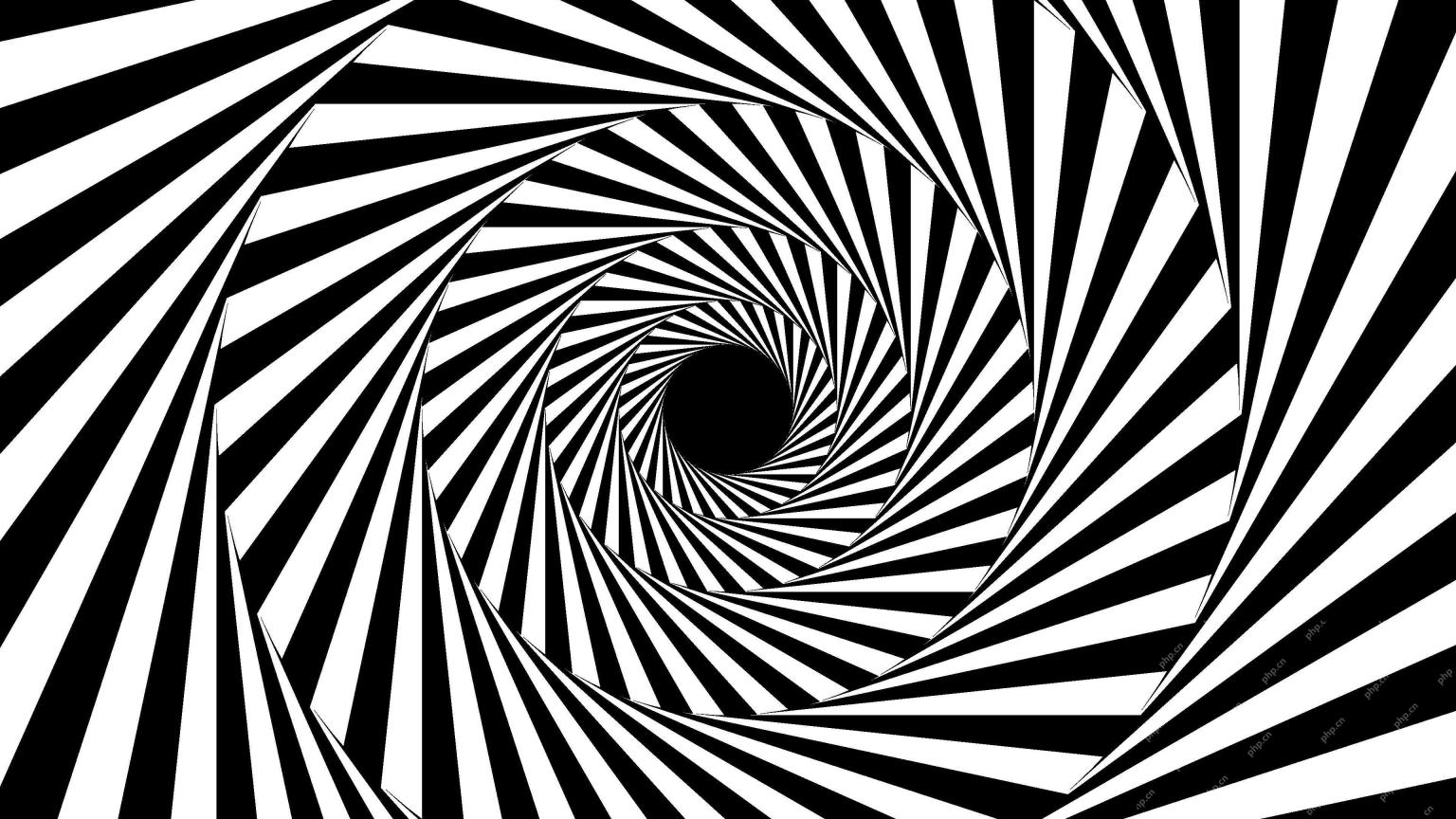
The intricate relationship between artificial intelligence (AI) and human intelligence (NI) is best understood as a feedback loop. Humans create AI, training it on data generated by human activity to enhance or replicate human capabilities. This AI
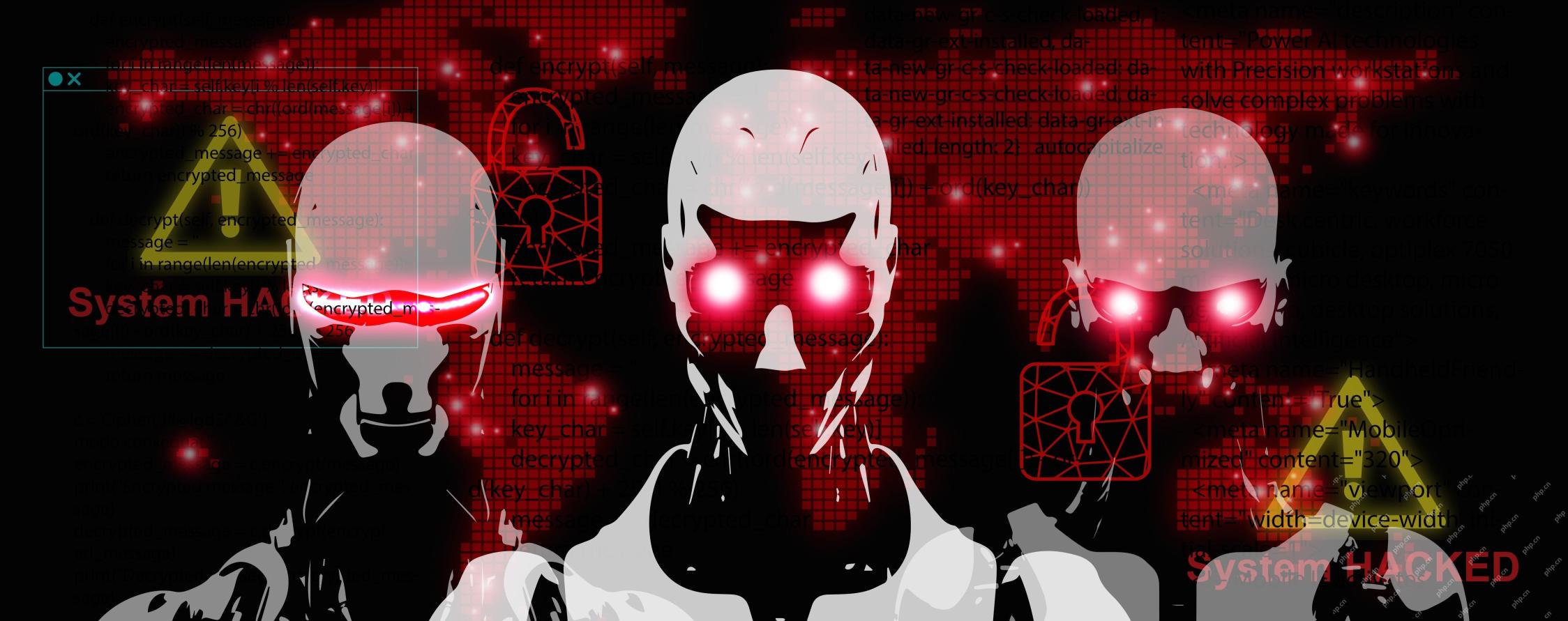
Anthropic's recent statement, highlighting the lack of understanding surrounding cutting-edge AI models, has sparked a heated debate among experts. Is this opacity a genuine technological crisis, or simply a temporary hurdle on the path to more soph
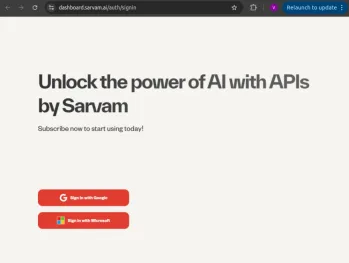
India is a diverse country with a rich tapestry of languages, making seamless communication across regions a persistent challenge. However, Sarvam’s Bulbul-V2 is helping to bridge this gap with its advanced text-to-speech (TTS) t


Hot AI Tools

Undresser.AI Undress
AI-powered app for creating realistic nude photos

AI Clothes Remover
Online AI tool for removing clothes from photos.

Undress AI Tool
Undress images for free

Clothoff.io
AI clothes remover

Video Face Swap
Swap faces in any video effortlessly with our completely free AI face swap tool!

Hot Article

Hot Tools

Safe Exam Browser
Safe Exam Browser is a secure browser environment for taking online exams securely. This software turns any computer into a secure workstation. It controls access to any utility and prevents students from using unauthorized resources.

SublimeText3 Linux new version
SublimeText3 Linux latest version

DVWA
Damn Vulnerable Web App (DVWA) is a PHP/MySQL web application that is very vulnerable. Its main goals are to be an aid for security professionals to test their skills and tools in a legal environment, to help web developers better understand the process of securing web applications, and to help teachers/students teach/learn in a classroom environment Web application security. The goal of DVWA is to practice some of the most common web vulnerabilities through a simple and straightforward interface, with varying degrees of difficulty. Please note that this software

SublimeText3 English version
Recommended: Win version, supports code prompts!
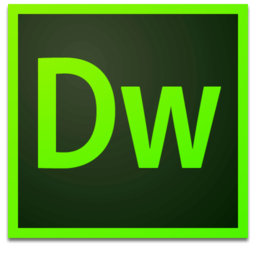
Dreamweaver Mac version
Visual web development tools
