


Based on information theory calibration technology, CML makes multi-modal machine learning more reliable
Multimodal machine learning has made impressive progress in various scenarios. However, the reliability of multimodal learning models lacks in-depth research. "Information is the elimination of uncertainty." The original intention of multi-modal machine learning is consistent with this - added modalities can make predictions more accurate and reliable. However, the paper "Calibrating Multimodal Learning" recently published in ICML2023 found that current multimodal learning methods violate this reliability assumption, and made detailed analysis and corrections.
Picture
- ##Paper Arxiv: https:// arxiv.org/abs/2306.01265
- Code GitHub: https://github.com/QingyangZhang/CML
The current multi-modal classification method has unreliable confidence, that is, when some modes are removed, the model may produce higher confidence, which violates the information theory "Information is the elimination of uncertainty" is the basic principle. To address this problem, this article proposes the Calibrating Multimodal Learning method. This method can be deployed in different multi-modal learning paradigms to improve the rationality and credibility of multi-modal learning models.
Picture
This work points out that current multi-modal learning methods have unreliable prediction confidence problems. Modal machine learning models tend to rely on partial modalities to estimate confidence. In particular, the study found that the confidence of current model estimates increases when certain modes are damaged. To solve this unreasonable problem, the authors propose an intuitive multi-modal learning principle: when the modality is removed, the model prediction confidence should not increase. However, current models tend to believe and be influenced by a subset of modalities, rather than considering all modalities fairly. This further affects the robustness of the model, i.e. the model is easily affected when certain modes are damaged.
To solve the above problems, some current methods adopt existing uncertainty calibration methods, such as Temperature Scaling or Bayesian learning methods. These methods can construct more accurate confidence estimates than traditional training/inference methods. However, these methods only match the confidence estimate of the final fusion result with the accuracy, and do not explicitly consider the relationship between the modal information amount and confidence. Therefore, they cannot essentially improve the credibility of the multi-modal learning model.
The author proposes a new regularization technique called "Calibrating Multimodal Learning (CML)". This technique enforces the matching relationship between model prediction confidence and information content by adding a penalty term to achieve consistency between prediction confidence and information content. This technique is based on the natural intuition that when a modality is removed, prediction confidence should decrease (at least it should not increase), which can inherently improve confidence calibration. Specifically, a simple regularization term is proposed to force the model to learn intuitive ordering relationships by adding a penalty to those samples whose prediction confidence increases when a modality is removed:
The above constraints are regular losses, which appear as penalties when modal information is removed and confidence increases.
Experimental results show that CML regularization can significantly improve the reliability of prediction confidence of existing multi-modal learning methods. Additionally, CML can improve classification accuracy and improve model robustness.
Multimodal machine learning has made significant progress in various scenarios, but the reliability of multimodal machine learning models is still a problem that needs to be solved. Through extensive empirical research, this paper finds that current multimodal classification methods have the problem of unreliable prediction confidence and violate the principles of information theory. To address this issue, researchers proposed the CML regularization technique, which can be flexibly deployed to existing models and improve performance in terms of confidence calibration, classification accuracy, and model robustness. It is believed that this new technology will play an important role in future multi-modal learning and improve the reliability and practicality of machine learning.
The above is the detailed content of Based on information theory calibration technology, CML makes multi-modal machine learning more reliable. For more information, please follow other related articles on the PHP Chinese website!
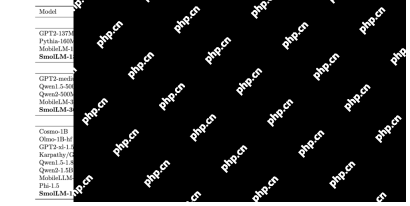
Harness the Power of On-Device AI: Building a Personal Chatbot CLI In the recent past, the concept of a personal AI assistant seemed like science fiction. Imagine Alex, a tech enthusiast, dreaming of a smart, local AI companion—one that doesn't rely
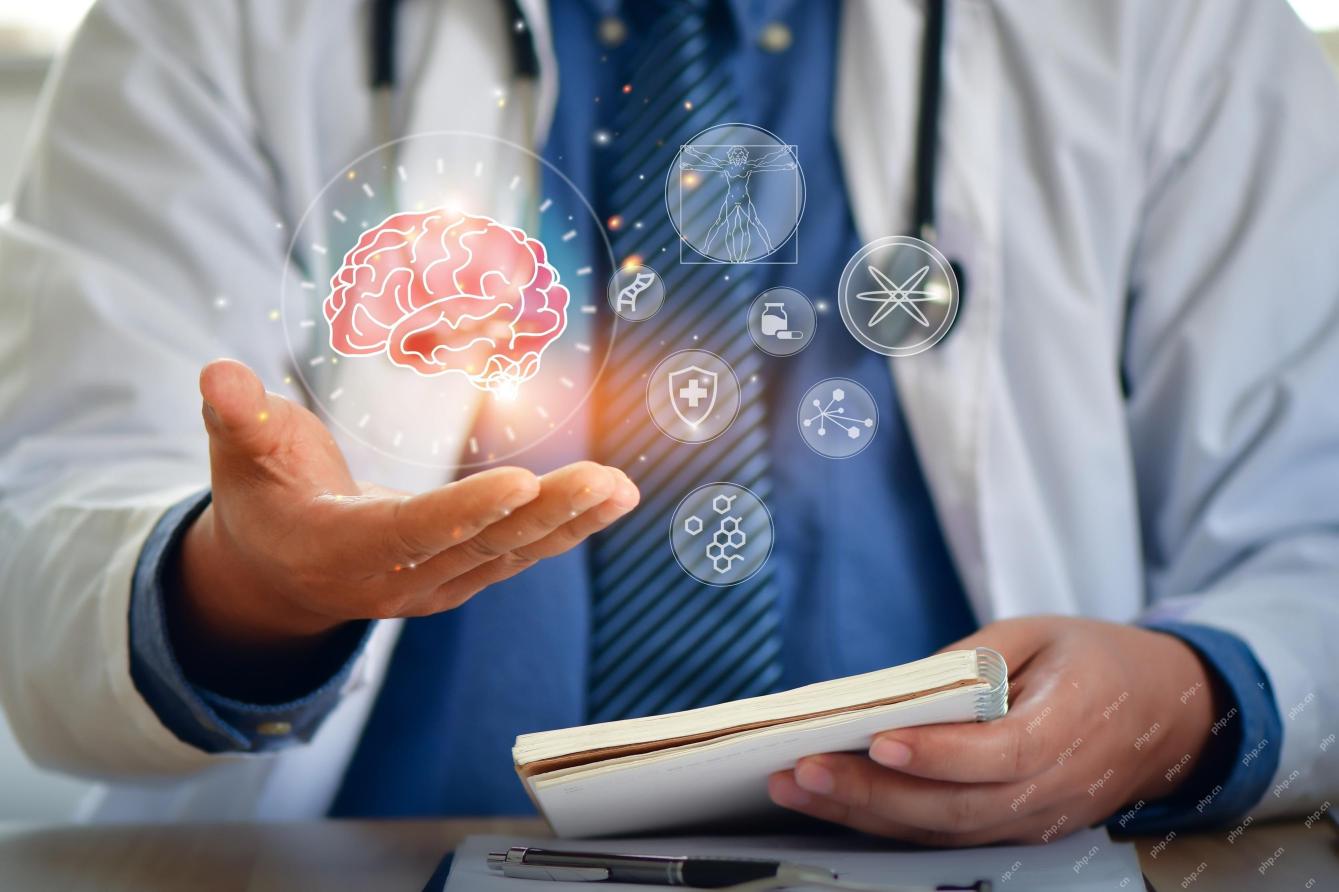
Their inaugural launch of AI4MH took place on April 15, 2025, and luminary Dr. Tom Insel, M.D., famed psychiatrist and neuroscientist, served as the kick-off speaker. Dr. Insel is renowned for his outstanding work in mental health research and techno
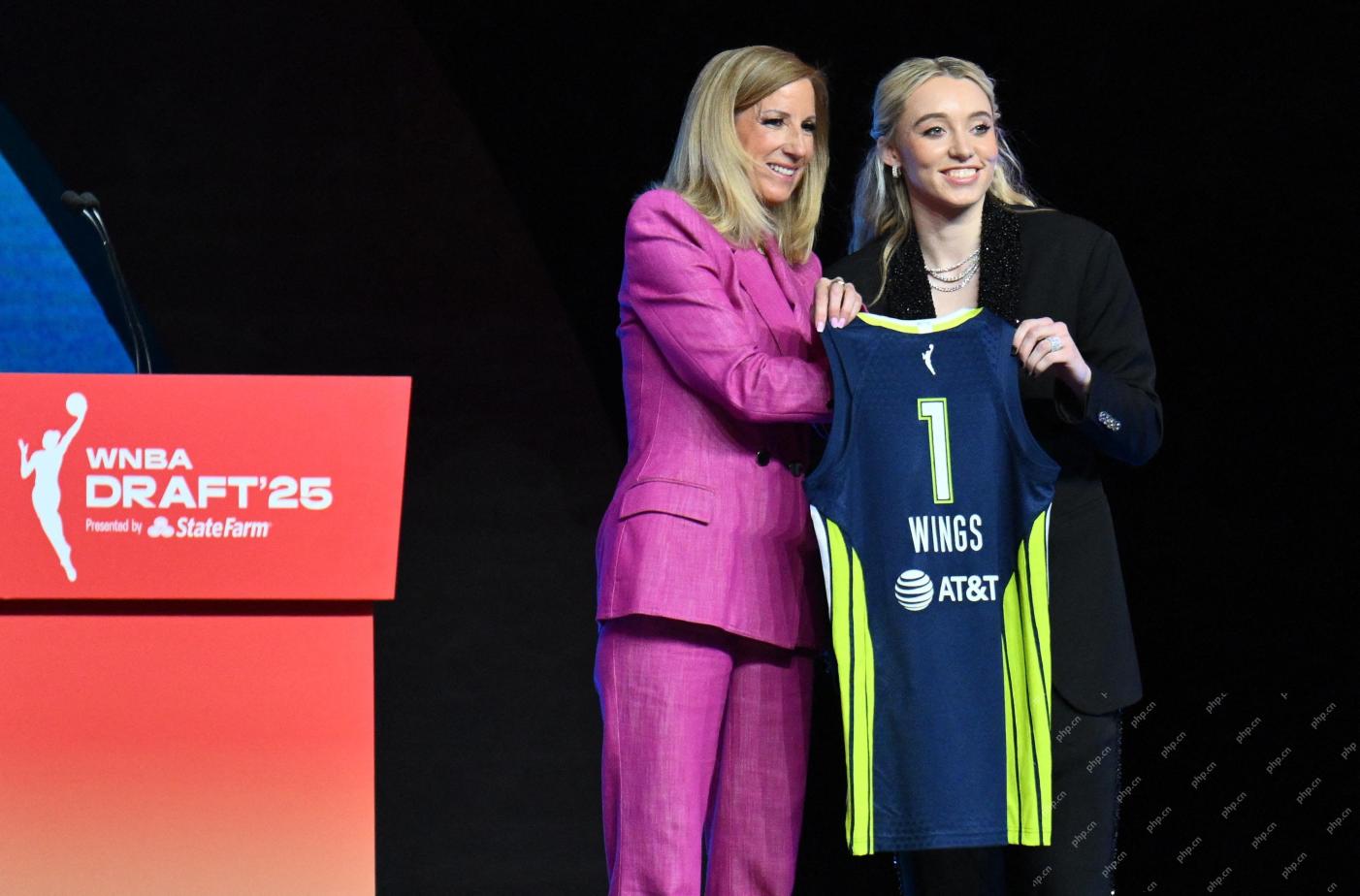
"We want to ensure that the WNBA remains a space where everyone, players, fans and corporate partners, feel safe, valued and empowered," Engelbert stated, addressing what has become one of women's sports' most damaging challenges. The anno
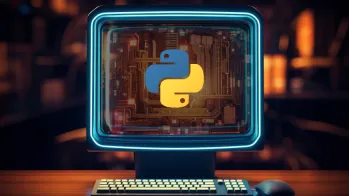
Introduction Python excels as a programming language, particularly in data science and generative AI. Efficient data manipulation (storage, management, and access) is crucial when dealing with large datasets. We've previously covered numbers and st
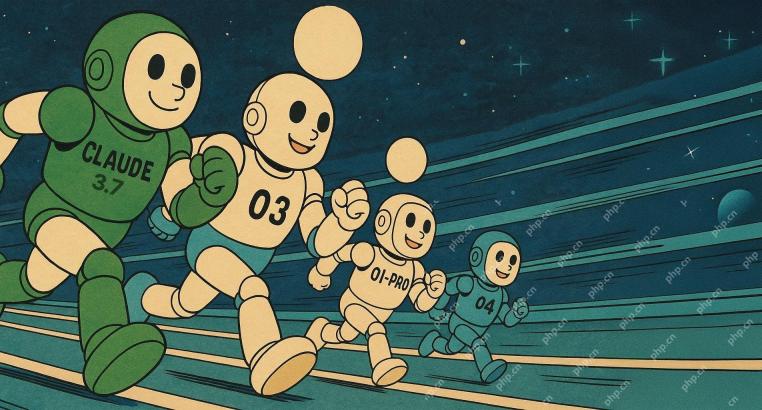
Before diving in, an important caveat: AI performance is non-deterministic and highly use-case specific. In simpler terms, Your Mileage May Vary. Don't take this (or any other) article as the final word—instead, test these models on your own scenario
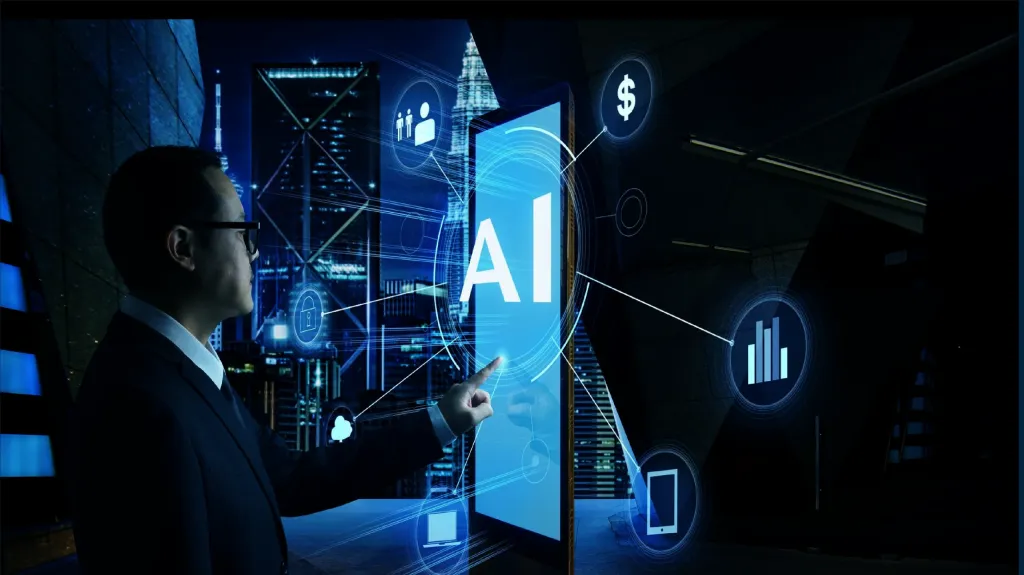
Building a Standout AI/ML Portfolio: A Guide for Beginners and Professionals Creating a compelling portfolio is crucial for securing roles in artificial intelligence (AI) and machine learning (ML). This guide provides advice for building a portfolio
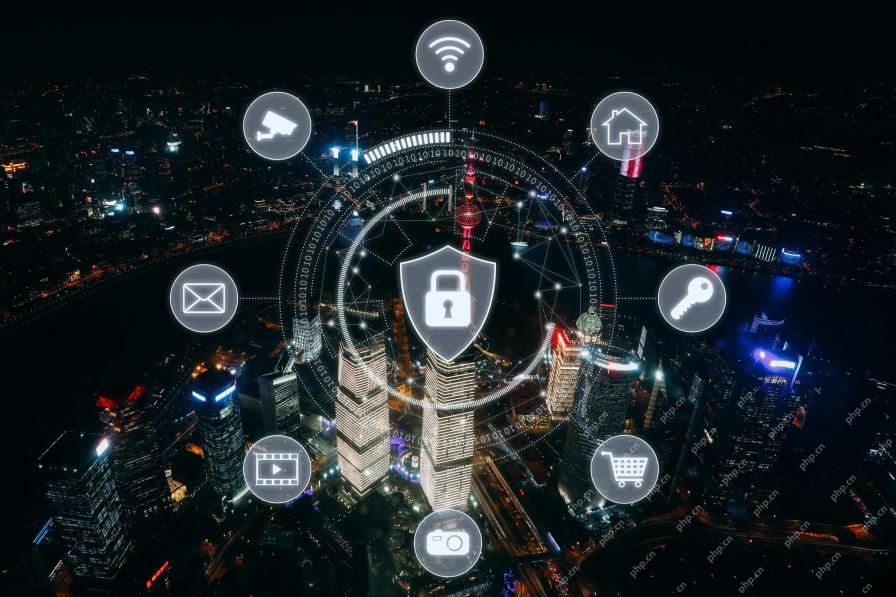
The result? Burnout, inefficiency, and a widening gap between detection and action. None of this should come as a shock to anyone who works in cybersecurity. The promise of agentic AI has emerged as a potential turning point, though. This new class
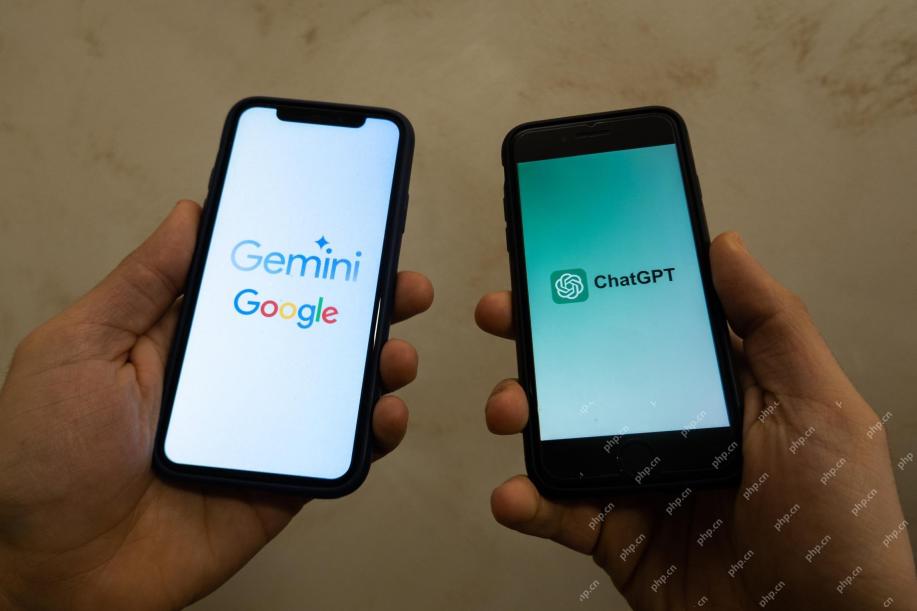
Immediate Impact versus Long-Term Partnership? Two weeks ago OpenAI stepped forward with a powerful short-term offer, granting U.S. and Canadian college students free access to ChatGPT Plus through the end of May 2025. This tool includes GPT‑4o, an a


Hot AI Tools

Undresser.AI Undress
AI-powered app for creating realistic nude photos

AI Clothes Remover
Online AI tool for removing clothes from photos.

Undress AI Tool
Undress images for free

Clothoff.io
AI clothes remover

AI Hentai Generator
Generate AI Hentai for free.

Hot Article

Hot Tools

MantisBT
Mantis is an easy-to-deploy web-based defect tracking tool designed to aid in product defect tracking. It requires PHP, MySQL and a web server. Check out our demo and hosting services.

SublimeText3 Linux new version
SublimeText3 Linux latest version

SublimeText3 Chinese version
Chinese version, very easy to use

Atom editor mac version download
The most popular open source editor
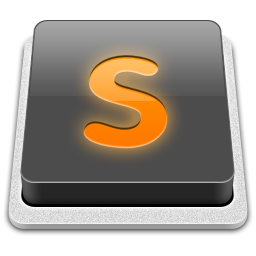
SublimeText3 Mac version
God-level code editing software (SublimeText3)