


Adaptive learning and multi-task learning technologies and applications in deep learning implemented using Java
Deep Learning is a method of Machine Learning, which allows computers to independently learn the characteristics of data by establishing multi-layer neural networks, thereby achieving the ability to learn skills and tasks. In order to make deep learning more efficient and flexible in practical applications, deep learning has been more widely used with the support of adaptive learning and multi-task learning technologies.
Java language is increasingly used in the field of deep learning, thanks to the convenient and easy-to-use development environment and excellent performance provided by the Java platform. Below we will introduce how to use Java to implement adaptive learning and multi-task learning technologies in deep learning, and illustrate their applications through practical cases.
1. Adaptive learning technology
Adaptive learning technology means that deep learning neural networks can learn new features and knowledge independently, and can adapt to new environments and tasks. Specifically, adaptive learning techniques include: unsupervised learning, incremental learning, and transfer learning. Let’s introduce them separately below.
(1) Unsupervised learning
Unsupervised learning means that the neural network can autonomously learn the characteristics and knowledge of the data without label data. In the Java language, we can use the DL4J (Deep Learning for Java) framework to implement unsupervised learning. The DL4J framework provides some commonly used unsupervised learning algorithms, such as AutoEncoder and Restricted Boltzmann Machines (RBM), etc. These algorithms can be used to extract features and reduce dimensionality of data. .
For example, we can use the DL4J framework to implement a simple autoencoder for unsupervised learning. The following is the Java code:
// 导入相关库 import org.nd4j.linalg.factory.Nd4j; import org.deeplearning4j.nn.api.Layer; import org.deeplearning4j.nn.conf.ComputationGraphConfiguration; import org.deeplearning4j.nn.conf.NeuralNetConfiguration; import org.deeplearning4j.nn.conf.layers.AutoEncoder; import org.deeplearning4j.nn.graph.ComputationGraph; import org.nd4j.linalg.api.ndarray.INDArray; // 构建自编码器 ComputationGraphConfiguration conf = new NeuralNetConfiguration.Builder() .learningRate(0.01) .graphBuilder() .addInputs("input") .addLayer("encoder", new AutoEncoder.Builder() .nIn(inputSize) .nOut(encodingSize) .build(), "input") .addLayer("decoder", new AutoEncoder.Builder() .nIn(encodingSize) .nOut(inputSize) .build(), "encoder") .setOutputs("decoder") .build(); ComputationGraph ae = new ComputationGraph(conf); ae.init(); // 训练自编码器 INDArray input = Nd4j.rand(batchSize, inputSize); ae.fit(new INDArray[]{input}, new INDArray[]{input});
The above code defines an autoencoder neural network and is trained using data generated by random numbers. During the training process, the autoencoder will autonomously learn the features of the data and use the learned features to reconstruct the input data.
(2) Incremental learning
Incremental learning means that the neural network can continuously update its own characteristics and knowledge while continuously receiving new data, and can quickly adapt to the new environment. and tasks. In the Java language, we can use the DL4J framework to implement incremental learning. The DL4J framework provides some commonly used incremental learning algorithms, such as Stochastic Gradient Descent (SGD for short) and Adaptive Moment Estimation (Adam for short).
For example, we can use the DL4J framework to implement a simple neural network for incremental learning. The following is the Java code:
// 导入相关库 import org.nd4j.linalg.factory.Nd4j; import org.deeplearning4j.nn.api.Layer; import org.deeplearning4j.nn.conf.ComputationGraphConfiguration; import org.deeplearning4j.nn.conf.NeuralNetConfiguration; import org.deeplearning4j.nn.api.Model; import org.deeplearning4j.nn.conf.layers.DenseLayer; import org.nd4j.linalg.dataset.DataSet; import org.nd4j.linalg.lossfunctions.LossFunctions.LossFunction; // 构建神经网络 NeuralNetConfiguration.Builder builder = new NeuralNetConfiguration.Builder() .updater(new Adam()) .seed(12345) .list() .layer(new DenseLayer.Builder().nIn(numInputs).nOut(numHiddenNodes) .activation(Activation.RELU) .build()) .layer(new OutputLayer.Builder().nIn(numHiddenNodes).nOut(numOutputs) .activation(Activation.SOFTMAX) .lossFunction(LossFunction.NEGATIVELOGLIKELIHOOD) .build()) .backpropType(BackpropType.Standard); // 初始化模型 Model model = new org.deeplearning4j.nn.multilayer.MultiLayerNetwork(builder.build()); model.init(); // 训练模型 DataSet dataSet = new DataSet(inputs, outputs); model.fit(dataSet);
The above code defines a simple neural network model and uses data generated by random numbers for training. During the training process, the neural network will receive new data and continuously update its own features and knowledge.
(3) Transfer learning
Transfer learning refers to using existing knowledge and models to learn and apply new knowledge and models on new tasks. In the Java language, we can use the DL4J framework to implement transfer learning. The DL4J framework provides some commonly used transfer learning algorithms, such as feedforward transfer learning and LSTM transfer learning.
For example, we can use the DL4J framework to implement a simple feed-forward transfer learning model to solve the image classification problem. The following is the Java code:
// 导入相关库 import org.deeplearning4j.nn.conf.ComputationGraphConfiguration; import org.deeplearning4j.nn.conf.inputs.InputType; import org.deeplearning4j.nn.conf.layers.DenseLayer; import org.deeplearning4j.nn.conf.layers.OutputLayer; import org.deeplearning4j.nn.transferlearning.FineTuneConfiguration; import org.deeplearning4j.nn.transferlearning.TransferLearning; import org.deeplearning4j.zoo.PretrainedType; import org.deeplearning4j.zoo.model.VGG16; import org.nd4j.linalg.dataset.api.iterator.DataSetIterator; import org.nd4j.linalg.lossfunctions.LossFunctions.LossFunction; // 载入VGG16模型 VGG16 vgg16 = (VGG16) PretrainedType.VGG16.pretrained(); ComputationGraph pretrained = vgg16.init(); System.out.println(pretrained.summary()); // 构建迁移学习模型 FineTuneConfiguration fineTuneConf = new FineTuneConfiguration.Builder() .learningRate(0.001) .build(); ComputationGraphConfiguration conf = new TransferLearning.GraphBuilder(pretrained) .fineTuneConfiguration(fineTuneConf) .setInputTypes(InputType.convolutional(224, 224, 3)) .removeVertexAndConnections("predictions") .addLayer("fc", new DenseLayer.Builder() .nIn(4096).nOut(numClasses).activation("softmax").build(), "fc7") .addLayer("predictions", new OutputLayer.Builder() .nIn(numClasses).nOut(numClasses).lossFunction(LossFunction.MCXENT).activation("softmax").build(), "fc") .build(); ComputationGraph model = new ComputationGraph(conf); model.init(); // 训练迁移学习模型 DataSetIterator trainData = getDataIterator("train"); DataSetIterator testData = getDataIterator("test"); for (int i = 0; i < numEpochs; i++) { model.fit(trainData); ... }
The above code first loads the pre-trained weights of the VGG16 model, and then uses the TransferLearning class to build a new transfer learning model. The model uses the first 7 convolutional layers of VGG16 as feature extractors, and then adds a fully connected layer and an output layer for image classification. During the training process, we used a data iterator to load and process training data and test data, and trained the model multiple iterations.
2. Multi-task learning technology
Multi-task learning technology means that the neural network can learn multiple tasks at the same time and can improve the learning effect by sharing and transferring knowledge. In the Java language, we can use the DL4J framework to implement multi-task learning. The DL4J framework provides some commonly used multi-task learning algorithms, such as joint learning (Multi-Task Learning, referred to as MTL) and transfer multi-task learning (Transfer Multi-Task Learning, referred to as TMTL).
For example, we can use the DL4J framework to implement a simple MTL model to solve robust regression and classification problems. The following is the Java code:
// 导入相关库 import org.deeplearning4j.nn.conf.ComputationGraphConfiguration; import org.deeplearning4j.nn.conf.inputs.InputType; import org.deeplearning4j.nn.conf.layers.DenseLayer; import org.deeplearning4j.nn.conf.layers.OutputLayer; import org.deeplearning4j.nn.multitask.MultiTaskNetwork; import org.nd4j.linalg.dataset.DataSet; import org.nd4j.linalg.dataset.api.iterator.DataSetIterator; import org.nd4j.linalg.lossfunctions.LossFunctions.LossFunction; // 构建MTL模型 ComputationGraphConfiguration.GraphBuilder builder = new NeuralNetConfiguration.Builder() .seed(12345) .updater(new Adam(0.0001)) .graphBuilder() .addInputs("input") .setInputTypes(InputType.feedForward(inputShape)) .addLayer("dense1", new DenseLayer.Builder() .nIn(inputSize) .nOut(hiddenSize) .activation(Activation.RELU) .build(), "input") .addLayer("output1", new OutputLayer.Builder() .nIn(hiddenSize) .nOut(outputSize1) .lossFunction(LossFunction.MSE) .build(), "dense1") .addLayer("output2", new OutputLayer.Builder() .nIn(hiddenSize) .nOut(outputSize2) .lossFunction(LossFunction.MCXENT) .activation(Activation.SOFTMAX) .build(), "dense1") .setOutputs("output1", "output2"); // 初始化MTL模型 MultiTaskNetwork model = new MultiTaskNetwork(builder.build()); model.init(); // 训练MTL模型 DataSetIterator dataSet = getDataSetIterator(); for (int i = 0; i < numEpochs; i++) { while(dataSet.hasNext()) { DataSet batch = dataSet.next(); model.fitMultiTask(batch); } ... }
The above code defines a simple MTL model. The model uses a shared hidden layer and two independent output layers for robust regression and classification tasks. During the training process, we used a data iterator to load and process training data, and trained the model for multiple iterations.
In summary, adaptive learning and multi-task learning technology are of great significance to the application of deep learning. Using the DL4J framework provided by the Java platform, we can easily implement these technologies and achieve better results in practical applications.
The above is the detailed content of Adaptive learning and multi-task learning technologies and applications in deep learning implemented using Java. For more information, please follow other related articles on the PHP Chinese website!
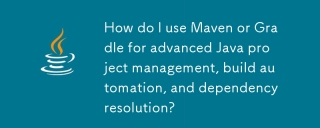
The article discusses using Maven and Gradle for Java project management, build automation, and dependency resolution, comparing their approaches and optimization strategies.
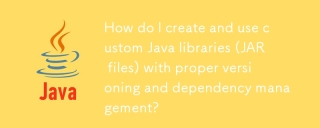
The article discusses creating and using custom Java libraries (JAR files) with proper versioning and dependency management, using tools like Maven and Gradle.
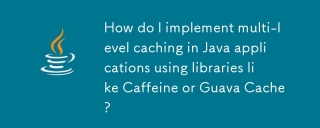
The article discusses implementing multi-level caching in Java using Caffeine and Guava Cache to enhance application performance. It covers setup, integration, and performance benefits, along with configuration and eviction policy management best pra
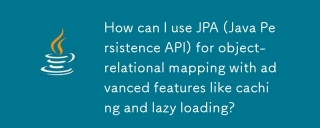
The article discusses using JPA for object-relational mapping with advanced features like caching and lazy loading. It covers setup, entity mapping, and best practices for optimizing performance while highlighting potential pitfalls.[159 characters]
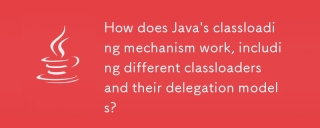
Java's classloading involves loading, linking, and initializing classes using a hierarchical system with Bootstrap, Extension, and Application classloaders. The parent delegation model ensures core classes are loaded first, affecting custom class loa


Hot AI Tools

Undresser.AI Undress
AI-powered app for creating realistic nude photos

AI Clothes Remover
Online AI tool for removing clothes from photos.

Undress AI Tool
Undress images for free

Clothoff.io
AI clothes remover

AI Hentai Generator
Generate AI Hentai for free.

Hot Article

Hot Tools
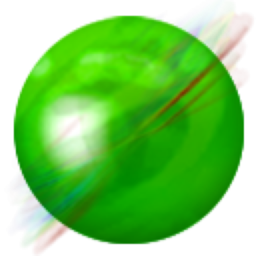
ZendStudio 13.5.1 Mac
Powerful PHP integrated development environment

PhpStorm Mac version
The latest (2018.2.1) professional PHP integrated development tool

SecLists
SecLists is the ultimate security tester's companion. It is a collection of various types of lists that are frequently used during security assessments, all in one place. SecLists helps make security testing more efficient and productive by conveniently providing all the lists a security tester might need. List types include usernames, passwords, URLs, fuzzing payloads, sensitive data patterns, web shells, and more. The tester can simply pull this repository onto a new test machine and he will have access to every type of list he needs.

DVWA
Damn Vulnerable Web App (DVWA) is a PHP/MySQL web application that is very vulnerable. Its main goals are to be an aid for security professionals to test their skills and tools in a legal environment, to help web developers better understand the process of securing web applications, and to help teachers/students teach/learn in a classroom environment Web application security. The goal of DVWA is to practice some of the most common web vulnerabilities through a simple and straightforward interface, with varying degrees of difficulty. Please note that this software
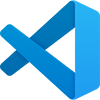
VSCode Windows 64-bit Download
A free and powerful IDE editor launched by Microsoft