Logistic regression algorithm example in Python
Logistic regression is a commonly used classification algorithm that is widely used in the fields of machine learning and data analysis. This article will introduce how to use the logistic regression algorithm for classification in Python. The specific implementation steps are as follows:
Step 1: Import the necessary Python libraries
Before starting to implement the logistic regression algorithm, we need Import the necessary Python libraries, such as: NumPy and pandas (for data processing and cleaning), sklearn and matplotlib (for model training and evaluation, and data visualization). The specific code is as follows:
import numpy as np import pandas as pd from sklearn.linear_model import LogisticRegression from sklearn.model_selection import train_test_split from sklearn.metrics import accuracy_score,confusion_matrix import matplotlib.pyplot as plt %matplotlib inline
Step 2: Load the data set
Next, we need to load the data set. Here we take the iris data set (iris) as an example. This data set contains 4 characteristics of 3 different species of iris (Setosa, Versicolour and Virginica): sepal length, sepal width, petal length and petal width. We can load the dataset by using pandas to read the data file in CSV format. The specific code is as follows:
data=pd.read_csv('iris.csv') print(data.head())
Step 3: Data processing and separation
Before inputting the data into the logistic regression model , we need to process and separate data. First, we need to separate the label column in the dataset as our target variable (y), while using the remaining feature columns as our independent variables (X). Secondly, we need to process the data, including: processing missing data, processing outliers, converting categorical variables, etc. Here, we can randomly split the data set into training data and test data by using the train_test_split function in the sklearn library. The specific code is as follows:
X=data.iloc[:,:-1] y=data.iloc[:,-1] X_train,X_test,y_train,y_test=train_test_split(X,y,test_size=0.3,random_state=42)
Step 4: Model training and evaluation
Now, we can use the logistic regression algorithm to fit the training data. We can create a logistic regression object by using the LogisticRegression class in the sklearn library, and then use the fit method to fit the training data. After training is completed, we can use the predict method to predict the test data, and use the accuracy_score and confusion_matrix functions in the sklearn library to evaluate the model. The specific code is as follows:
lr=LogisticRegression() lr.fit(X_train,y_train) y_pred=lr.predict(X_test) acc=accuracy_score(y_test,y_pred) cm=confusion_matrix(y_test,y_pred) print('Accuracy:', acc) print('Confusion Matrix: ', cm)
Step 5: Visualization of results
Finally, we can use the matplotlib library to visualize our results. For example, we can use scatter plots or histograms to show the characteristics of the data and logistic regression classification boundaries. The specific code is as follows:
colors=['blue','green','red'] markers=['o','s','^'] labels=['Setosa','Versicolour','Virginica'] for i, target in enumerate(set(data.iloc[:,-1])): plt.scatter(x=data.loc[data.iloc[:,-1]==target,'petal_length'], y=data.loc[data.iloc[:,-1]==target,'petal_width'], c=colors[i], marker=markers[i], label=labels[i]) x=np.linspace(0,8,1000) y=(-lr.coef_[0][0]*x-lr.intercept_)/lr.coef_[0][1] plt.plot(x,y,'k-',label='Decision Boundary') plt.xlabel('Petal Length') plt.ylabel('Petal Width') plt.legend(loc='lower right') plt.show()
In summary, the above are the basic steps to implement the logistic regression algorithm in Python, which can be adjusted and improved according to specific data sets and classification problems. Although the logistic regression algorithm is simple and easy to use, it also requires a full understanding of its principles and appropriate data processing and model optimization to achieve better classification results.
The above is the detailed content of Logistic regression algorithm example in Python. For more information, please follow other related articles on the PHP Chinese website!
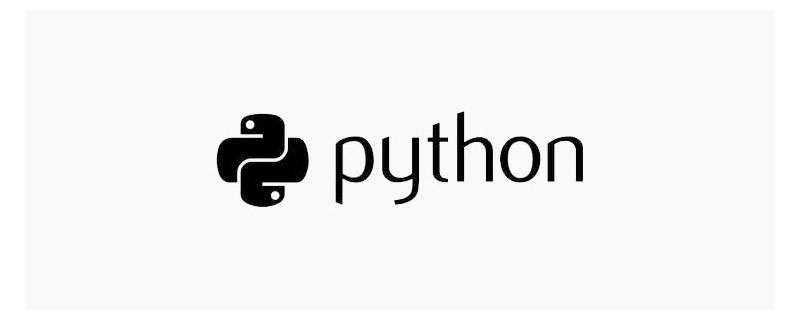
本篇文章给大家带来了关于Python的相关知识,其中主要介绍了关于Seaborn的相关问题,包括了数据可视化处理的散点图、折线图、条形图等等内容,下面一起来看一下,希望对大家有帮助。
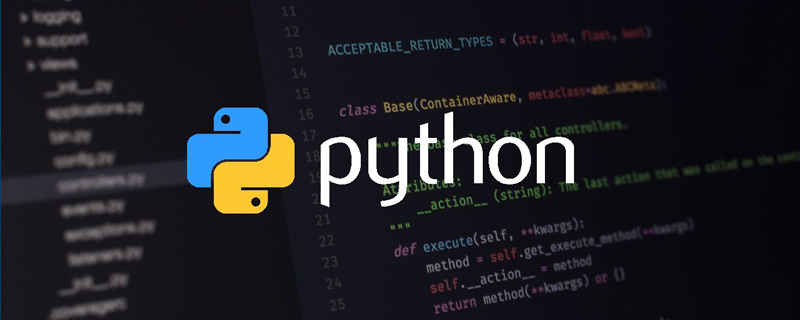
本篇文章给大家带来了关于Python的相关知识,其中主要介绍了关于进程池与进程锁的相关问题,包括进程池的创建模块,进程池函数等等内容,下面一起来看一下,希望对大家有帮助。
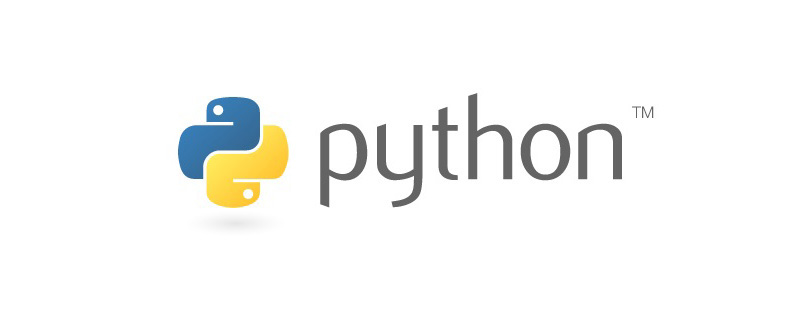
本篇文章给大家带来了关于Python的相关知识,其中主要介绍了关于简历筛选的相关问题,包括了定义 ReadDoc 类用以读取 word 文件以及定义 search_word 函数用以筛选的相关内容,下面一起来看一下,希望对大家有帮助。
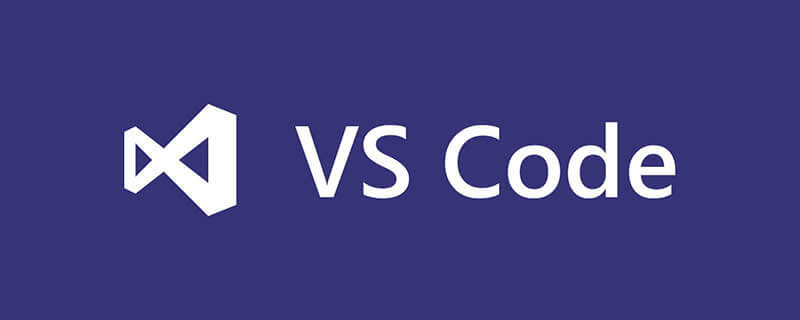
VS Code的确是一款非常热门、有强大用户基础的一款开发工具。本文给大家介绍一下10款高效、好用的插件,能够让原本单薄的VS Code如虎添翼,开发效率顿时提升到一个新的阶段。
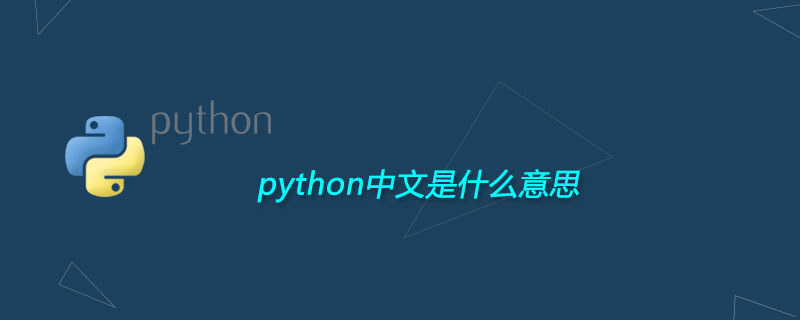
pythn的中文意思是巨蟒、蟒蛇。1989年圣诞节期间,Guido van Rossum在家闲的没事干,为了跟朋友庆祝圣诞节,决定发明一种全新的脚本语言。他很喜欢一个肥皂剧叫Monty Python,所以便把这门语言叫做python。
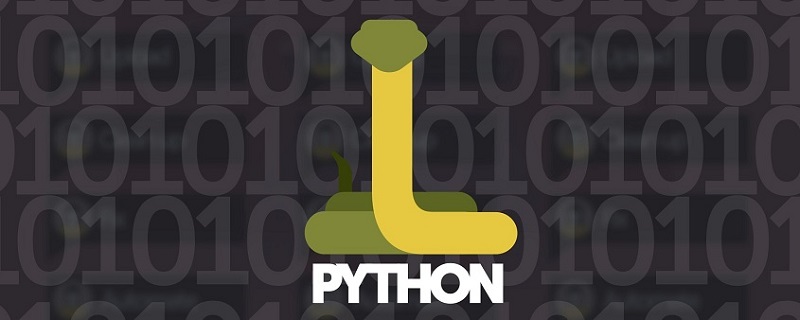
本篇文章给大家带来了关于Python的相关知识,其中主要介绍了关于数据类型之字符串、数字的相关问题,下面一起来看一下,希望对大家有帮助。
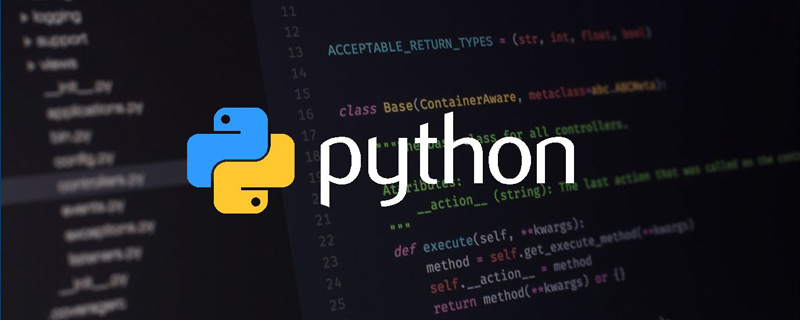
本篇文章给大家带来了关于Python的相关知识,其中主要介绍了关于numpy模块的相关问题,Numpy是Numerical Python extensions的缩写,字面意思是Python数值计算扩展,下面一起来看一下,希望对大家有帮助。


Hot AI Tools

Undresser.AI Undress
AI-powered app for creating realistic nude photos

AI Clothes Remover
Online AI tool for removing clothes from photos.

Undress AI Tool
Undress images for free

Clothoff.io
AI clothes remover

AI Hentai Generator
Generate AI Hentai for free.

Hot Article

Hot Tools

SublimeText3 Linux new version
SublimeText3 Linux latest version

Notepad++7.3.1
Easy-to-use and free code editor

Atom editor mac version download
The most popular open source editor
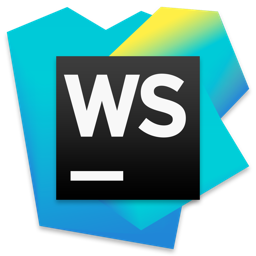
WebStorm Mac version
Useful JavaScript development tools
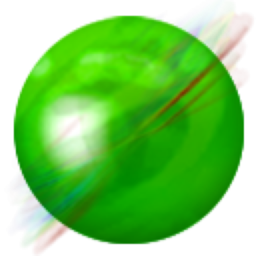
ZendStudio 13.5.1 Mac
Powerful PHP integrated development environment
