Random Forest (Random Forest) is an ensemble learning (Ensemble Learning) algorithm that improves accuracy and robustness by combining the prediction results of multiple decision trees. Random forests are widely used in various fields, such as finance, medical care, e-commerce, etc.
This article will introduce how to use Python to implement a random forest classifier and test it using the iris data set.
1. Iris Dataset
The Iris Dataset is a classic data set in machine learning. It contains 150 records, each record has 4 features and 1 category label. . The four characteristics are sepal length, sepal width, petal length and petal width, and the category label represents one of the three varieties of iris (Iris mountain, Iris versicolor, Iris virginia).
In Python, we can use scikit-learn, a powerful machine learning library, to load the iris data set. The specific operations are as follows:
from sklearn.datasets import load_iris iris = load_iris() X = iris.data y = iris.target
2. Build a random forest classifier
It is very simple to build a random forest classifier using scikit-learn. First, we need to import the RandomForestClassifier class from sklearn.ensemble and instantiate an object:
from sklearn.ensemble import RandomForestClassifier rfc = RandomForestClassifier(n_estimators=10)
Among them, the n_estimators parameter specifies the number of decision trees included in the random forest. Here, we set the number of decision trees in the random forest to 10.
Next, we need to divide the iris data set into training data and test data. Use the train_test_split function to randomly divide the data set into a training set and a test set:
from sklearn.model_selection import train_test_split X_train, X_test, y_train, y_test = train_test_split(X, y, test_size=0.3, random_state=42)
Among them, the test_size parameter specifies the proportion of the test set, and the random_state parameter specifies the seed of the pseudo-random number generator to ensure that each time Running the program gives the same result.
We can then use the training data to train the random forest classifier:
rfc.fit(X_train, y_train)
3. Test the random forest classifier
Once the classifier has been trained, we can use Test data to test its performance. Use the predict function to make predictions on the test set and the accuracy_score function to calculate the accuracy of the model:
from sklearn.metrics import accuracy_score y_pred = rfc.predict(X_test) accuracy = accuracy_score(y_test, y_pred) print("Accuracy:", accuracy)
Finally, we can use the matplotlib library to visualize the decision boundary of the classifier to better understand the behavior of the classifier :
import numpy as np import matplotlib.pyplot as plt from mpl_toolkits.mplot3d import Axes3D x_min, x_max = X[:, 0].min() - .5, X[:, 0].max() + .5 y_min, y_max = X[:, 1].min() - .5, X[:, 1].max() + .5 z_min, z_max = X[:, 2].min() - .5, X[:, 2].max() + .5 xx, yy, zz = np.meshgrid(np.arange(x_min, x_max, 0.2), np.arange(y_min, y_max, 0.2), np.arange(z_min, z_max, 0.2)) fig = plt.figure() ax = fig.add_subplot(111, projection='3d') Z = rfc.predict(np.c_[xx.ravel(), yy.ravel(), zz.ravel()]) Z = Z.reshape(xx.shape) ax.scatter(X[:, 0], X[:, 1], X[:, 2], c=y) ax.set_xlabel('Sepal length') ax.set_ylabel('Sepal width') ax.set_zlabel('Petal length') ax.set_title('Decision Boundary') ax.view_init(elev=30, azim=120) ax.plot_surface(xx, yy, zz, alpha=0.3, facecolors='blue') plt.show()
The above code will obtain a three-dimensional image, in which the color of the data points represents the variety of iris flowers, and the decision boundary is represented by a translucent blue surface.
4. Summary
This article introduces how to use Python to implement a random forest classifier and use the iris data set for testing. Due to the robustness and accuracy of the random forest algorithm, it has broad application prospects in practical applications. If you are interested in this algorithm, it is recommended to practice more and read relevant literature.
The above is the detailed content of Random forest algorithm example in Python. For more information, please follow other related articles on the PHP Chinese website!
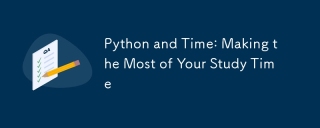
To maximize the efficiency of learning Python in a limited time, you can use Python's datetime, time, and schedule modules. 1. The datetime module is used to record and plan learning time. 2. The time module helps to set study and rest time. 3. The schedule module automatically arranges weekly learning tasks.
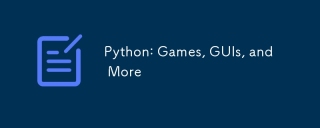
Python excels in gaming and GUI development. 1) Game development uses Pygame, providing drawing, audio and other functions, which are suitable for creating 2D games. 2) GUI development can choose Tkinter or PyQt. Tkinter is simple and easy to use, PyQt has rich functions and is suitable for professional development.
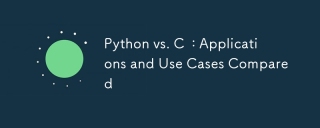
Python is suitable for data science, web development and automation tasks, while C is suitable for system programming, game development and embedded systems. Python is known for its simplicity and powerful ecosystem, while C is known for its high performance and underlying control capabilities.
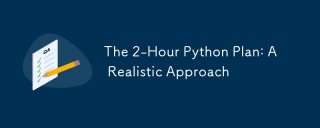
You can learn basic programming concepts and skills of Python within 2 hours. 1. Learn variables and data types, 2. Master control flow (conditional statements and loops), 3. Understand the definition and use of functions, 4. Quickly get started with Python programming through simple examples and code snippets.
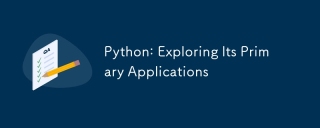
Python is widely used in the fields of web development, data science, machine learning, automation and scripting. 1) In web development, Django and Flask frameworks simplify the development process. 2) In the fields of data science and machine learning, NumPy, Pandas, Scikit-learn and TensorFlow libraries provide strong support. 3) In terms of automation and scripting, Python is suitable for tasks such as automated testing and system management.
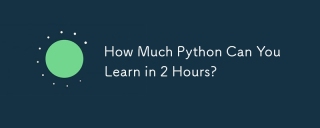
You can learn the basics of Python within two hours. 1. Learn variables and data types, 2. Master control structures such as if statements and loops, 3. Understand the definition and use of functions. These will help you start writing simple Python programs.
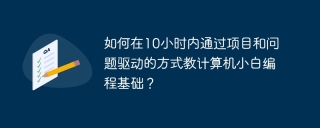
How to teach computer novice programming basics within 10 hours? If you only have 10 hours to teach computer novice some programming knowledge, what would you choose to teach...
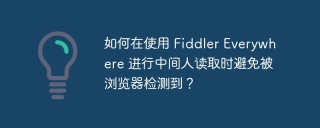
How to avoid being detected when using FiddlerEverywhere for man-in-the-middle readings When you use FiddlerEverywhere...


Hot AI Tools

Undresser.AI Undress
AI-powered app for creating realistic nude photos

AI Clothes Remover
Online AI tool for removing clothes from photos.

Undress AI Tool
Undress images for free

Clothoff.io
AI clothes remover

AI Hentai Generator
Generate AI Hentai for free.

Hot Article

Hot Tools

PhpStorm Mac version
The latest (2018.2.1) professional PHP integrated development tool

SublimeText3 English version
Recommended: Win version, supports code prompts!
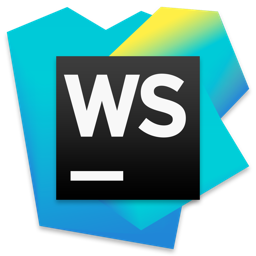
WebStorm Mac version
Useful JavaScript development tools

SAP NetWeaver Server Adapter for Eclipse
Integrate Eclipse with SAP NetWeaver application server.

Zend Studio 13.0.1
Powerful PHP integrated development environment