Detailed explanation of k-means clustering model in Python
Cluster analysis is a method used to discover similar objects in data. In fields such as data mining and machine learning, cluster analysis is widely used. k-means clustering is one of the more common clustering methods. It can divide the samples in the data set into k clusters, with the smallest internal difference in each cluster and the largest inter-cluster difference. This article will introduce the k-means clustering model in Python in detail.
- The principle of k-means clustering
The k-means clustering algorithm is an iterative clustering method. Its core steps include: initializing the center of mass, calculating distance, updating the center of mass, determining stopping conditions, etc.
First, you need to specify the number of clusters k. Then k data samples are randomly selected as the initial centroids, and for each remaining sample, it is assigned to the cluster with the nearest centroid. Next, the sum of the squared distances of all data points in each cluster from the centroid of the cluster is calculated as the error of the cluster. The centroid of each cluster is then updated, moving it to the center of all samples in that cluster. Repeat the above steps until the error is less than a certain threshold or the upper limit of the number of iterations is reached.
- Python implements k-means clustering
In Python, the sklearn library provides the k-means clustering function, which is the simplest way to use the k-means clustering algorithm. method. The following takes the iris data set as an example to show how to use Python to implement k-means clustering
from sklearn.cluster import KMeans from sklearn.datasets import load_iris iris = load_iris() X = iris.data[:, :2] # 为了便于可视化,只取前两个特征 y = iris.target kmeans = KMeans(n_clusters=3) # 聚成3类 kmeans.fit(X) centroids = kmeans.cluster_centers_ # 质心 labels = kmeans.labels_ # 样本分类 # 绘制图形 import matplotlib.pyplot as plt colors = ['red', 'green', 'blue'] for i in range(len(X)): plt.scatter(X[i][0], X[i][1], c=colors[labels[i]]) for c in centroids: plt.scatter(c[0], c[1], marker='x', s=300, linewidths=3, color='black') plt.show()
Execute the above code to generate an image similar to the following:
In the image, red, green and blue The color points represent different clusters, and the black "x" symbol represents the centroid of each cluster.
- How to choose the optimal k value
How to determine the optimal k value is one of the more difficult problems in the k-means clustering algorithm. Two common methods are introduced below: the elbow method and the contour coefficient method.
Elbow method: First, set the k value to a smaller integer and calculate the sum of squared errors (SSE) for each cluster. As the value of k increases, the sum of squared errors decreases. When the k value increases to a certain level, SSE no longer drops significantly. At this time, the relationship between the k value and SSE is drawn into a curve graph, which must present an elbow line segment. The line segment is at the "elbow" position here, and the corresponding k value is the optimal number of clusters.
Code example:
sse = [] for i in range(1, 11): kmeans = KMeans(n_clusters=i).fit(X) sse.append(kmeans.inertia_) # ineria_属性表示模型的误差平方和 plt.plot(range(1, 11), sse) plt.xlabel('K') plt.ylabel('SSE') plt.show()
Silhouette coefficient method: Silhouette coefficient combines the two factors of intra-cluster irrelevance and inter-cluster similarity. The larger the value of the silhouette coefficient, the better the clustering effect. The calculation process of the silhouette coefficient method is as follows:
For each sample, calculate its average distance from all samples in the same cluster (called a), and calculate its average distance from all samples in the nearest other clusters (called a) for b).
Calculate the silhouette coefficient s of each sample, $s = rac {b-a} {max(a, b)}$. The silhouette coefficient of the entire model is the average of the silhouette coefficients of all samples.
Code example:
from sklearn.metrics import silhouette_score sil_scores = [] for k in range(2, 11): kmeans = KMeans(n_clusters=k).fit(X) sil_score = silhouette_score(X, kmeans.labels_) # 计算轮廓系数 sil_scores.append(sil_score) plt.plot(range(2, 11), sil_scores) plt.xlabel('K') plt.ylabel('Silhouette Coefficient') plt.show()
- k-means clustering considerations
k-means clustering has the following considerations:
The initial value has a greater impact on the results. If the initial value is not good, you may get poor results.
The clustering results depend on the selected distance metric, such as Euclidean distance, Manhattan distance, etc. The choice should be made according to the actual situation.
Outliers in the data set are easily attracted to the wrong clusters, and removal of outliers should be considered.
When the sample class distribution is unbalanced, a common problem is to obtain clusters with extremely skewed attributes.
- Summary
k-means clustering is a widely used clustering algorithm. In Python, the KMeans function provided by the sklearn library can be used to quickly implement it. At the same time, the elbow method or the silhouette coefficient method can also be used to determine the optimal number of clusters. At the same time, attention should be paid to the selection of the k value and the setting of the initial centroid during application.
The above is the detailed content of Detailed explanation of k-means clustering model in Python. For more information, please follow other related articles on the PHP Chinese website!
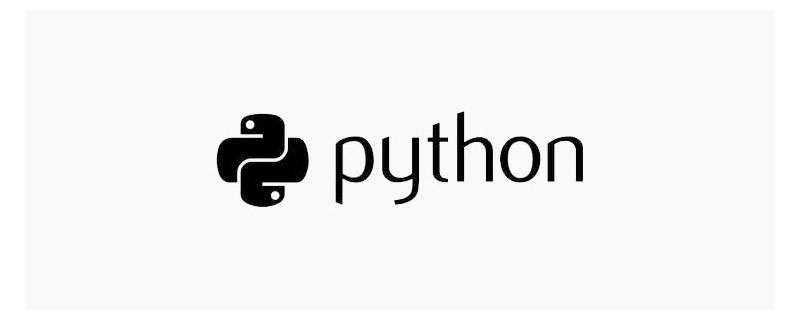
本篇文章给大家带来了关于Python的相关知识,其中主要介绍了关于Seaborn的相关问题,包括了数据可视化处理的散点图、折线图、条形图等等内容,下面一起来看一下,希望对大家有帮助。
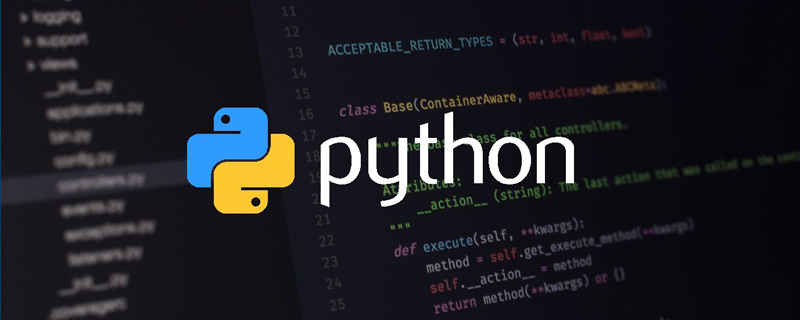
本篇文章给大家带来了关于Python的相关知识,其中主要介绍了关于进程池与进程锁的相关问题,包括进程池的创建模块,进程池函数等等内容,下面一起来看一下,希望对大家有帮助。
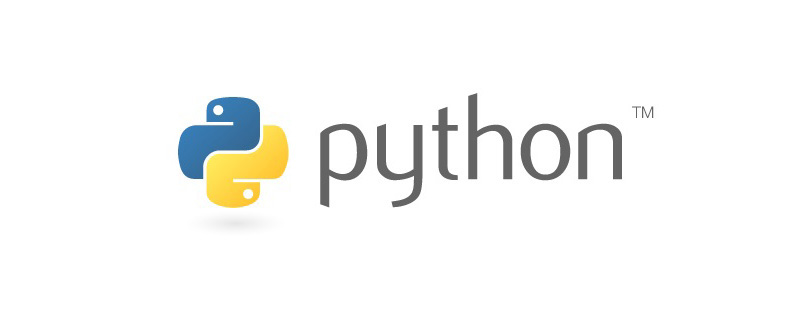
本篇文章给大家带来了关于Python的相关知识,其中主要介绍了关于简历筛选的相关问题,包括了定义 ReadDoc 类用以读取 word 文件以及定义 search_word 函数用以筛选的相关内容,下面一起来看一下,希望对大家有帮助。
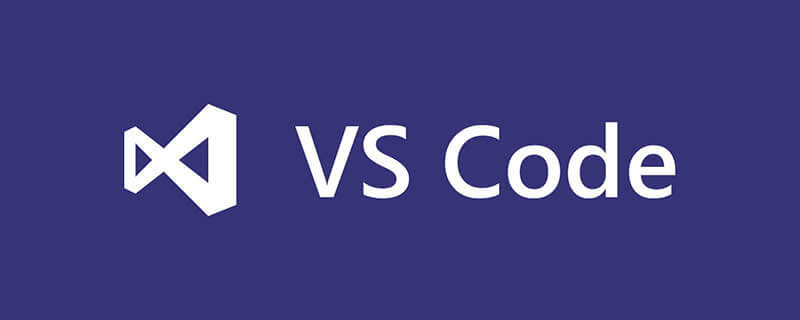
VS Code的确是一款非常热门、有强大用户基础的一款开发工具。本文给大家介绍一下10款高效、好用的插件,能够让原本单薄的VS Code如虎添翼,开发效率顿时提升到一个新的阶段。
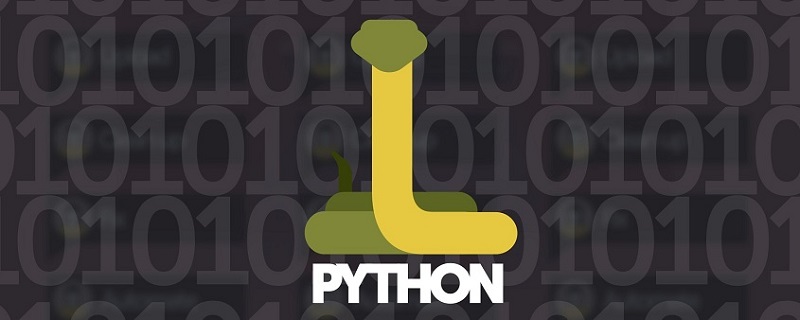
本篇文章给大家带来了关于Python的相关知识,其中主要介绍了关于数据类型之字符串、数字的相关问题,下面一起来看一下,希望对大家有帮助。
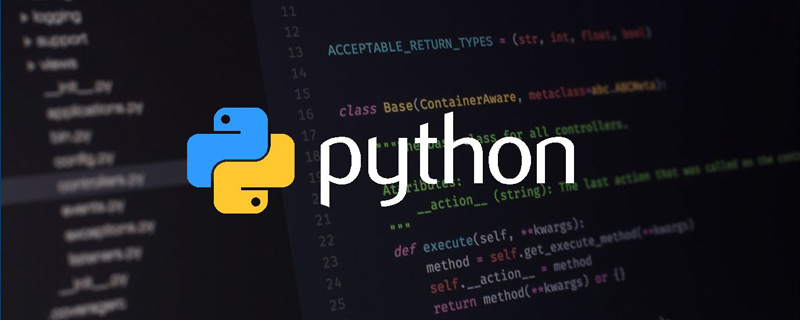
本篇文章给大家带来了关于Python的相关知识,其中主要介绍了关于numpy模块的相关问题,Numpy是Numerical Python extensions的缩写,字面意思是Python数值计算扩展,下面一起来看一下,希望对大家有帮助。
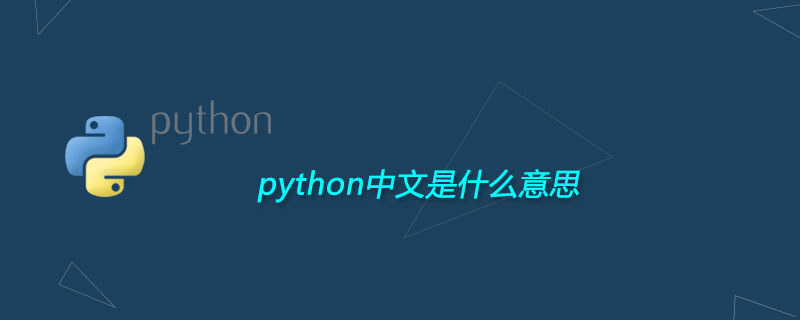
pythn的中文意思是巨蟒、蟒蛇。1989年圣诞节期间,Guido van Rossum在家闲的没事干,为了跟朋友庆祝圣诞节,决定发明一种全新的脚本语言。他很喜欢一个肥皂剧叫Monty Python,所以便把这门语言叫做python。


Hot AI Tools

Undresser.AI Undress
AI-powered app for creating realistic nude photos

AI Clothes Remover
Online AI tool for removing clothes from photos.

Undress AI Tool
Undress images for free

Clothoff.io
AI clothes remover

AI Hentai Generator
Generate AI Hentai for free.

Hot Article

Hot Tools

SAP NetWeaver Server Adapter for Eclipse
Integrate Eclipse with SAP NetWeaver application server.
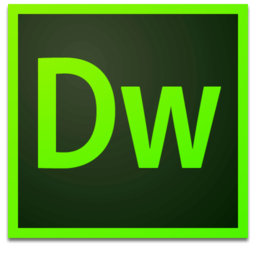
Dreamweaver Mac version
Visual web development tools
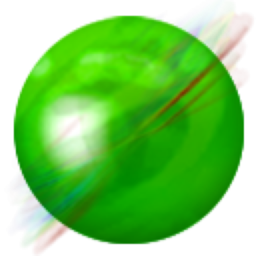
ZendStudio 13.5.1 Mac
Powerful PHP integrated development environment

Atom editor mac version download
The most popular open source editor

SublimeText3 Linux new version
SublimeText3 Linux latest version
