


Input the three-dimensional shapes of a rocking chair and a horse. What can you get?
##Wooden cart plus horse? Get a carriage and an electric horse; a banana and a sailboat? Get a banana sailboat; eggs plus deck chairs? Get the egg chair.
Researchers from UCSD, Shanghai Jiao Tong University, and Qualcomm teams have proposed the latest three-dimensional representation model OpenShape, making it possible to understand the open world of three-dimensional shapes.
- Paper address: https://arxiv.org/pdf/2305.10764.pdf
- Project homepage: https://colin97.github.io/OpenShape/
- Interactive demo: https://huggingface.co/spaces/OpenShape/openshape-demo
- Code address: https://github.com/Colin97/OpenShape_code
By learning a native encoder of 3D point clouds on multi-modal data (point cloud - text - image), OpenShape builds a representation space of 3D shapes and aligns it with CLIP's text and image spaces. Thanks to large-scale and diverse 3D pre-training, OpenShape achieves open-world understanding of 3D shapes for the first time, supporting zero-shot 3D shape classification, multi-modal 3D shape retrieval (text/image/point cloud input), and subtitles of 3D point clouds. Cross-modal tasks such as image generation and 3D point cloud-based image generation.
Three-dimensional shape zero-shot classificationOpenShape’s top3 and top5 accuracy on ModelNet40 reached 96.5% and 98.0% respectively.
Multimodal 3D shape retrieval
With OpenShape’s multimodal representation, users can perform 3D shape retrieval on image, text, or point cloud input. Study the retrieval of 3D shapes from integrated datasets by computing the cosine similarity between the input representation and the 3D shape representation and finding kNN.
The above figure shows the input image and two retrieved 3D shapes. Three-dimensional shape retrieval of text input The above image shows the input text and the retrieved three-dimensional shape. OpenShape learns a wide range of visual and semantic concepts, enabling fine-grained subcategory (first two lines) and attribute control (last two lines, such as color, shape, style, and their combinations). 3D shape retrieval of 3D point cloud input The above figure shows the input 3D point cloud and two retrieved 3D shapes.
#Double input three-dimensional shape retrieval
The above figure takes two 3D shapes as input and uses their OpenShape representations to retrieve the 3D shape that is closest to both inputs at the same time. The retrieved shape cleverly combines semantic and geometric elements from both input shapes.
Three-dimensional shape-based text and image generationBecause OpenShape's three-dimensional shape representation is aligned with CLIP's image and text representation space, they can be used with many based on Derived models from CLIP are combined to support a variety of cross-modal applications.
Subtitle generation of three-dimensional point cloud
By combining with the ready-made image subtitle model (ClipCap), OpenShape implements subtitle generation for 3D point clouds.
Image generation based on three-dimensional point cloud
By combining with the ready-made text-to-image diffusion model (Stable unCLIP), OpenShape implements image generation based on 3D point clouds (supporting optional text hints).
##More examples of image generation based on three-dimensional point clouds Training details
Multimodal representation alignment based on contrastive learning: OpenShape trains a 3D native encoder that will The 3D point cloud is used as input to extract the representation of the 3D shape. Following previous work, we exploit multimodal contrastive learning to align with CLIP's image and text representation spaces. Unlike previous work, OpenShape aims to learn a more general and scalable joint representation space. The focus of the research is mainly to expand the scale of 3D representation learning and address the corresponding challenges, so as to truly realize 3D shape understanding in the open world.
Integrating multiple 3D shape datasets: Since the scale and diversity of training data play a crucial role in learning large-scale 3D shape representations, the study integrated four Training on the currently largest publicly available 3D dataset. As shown in the figure below, the training data studied contains 876,000 training shapes. Among the four datasets, ShapeNetCore, 3D-FUTURE, and ABO contain high-quality human-verified 3D shapes, but only cover a limited number of shapes and dozens of categories. The Objaverse dataset is a recently released 3D dataset that contains significantly more 3D shapes and covers a more diverse object class. However, the shapes in the Objaverse are mainly uploaded by Internet users and have not been manually verified. Therefore, the quality is uneven and the distribution is extremely uneven, requiring further processing.
Text filtering and enrichment: Study found only between 3D shapes and 2D images Applying contrastive learning is insufficient to drive alignment of 3D shape and text spaces, even when trained on large-scale datasets. Research speculates that this is due to the inherent domain gap in CLIP's language and image representation spaces. Therefore, research needs to explicitly align 3D shapes with text. However, text annotations from original 3D data sets often face problems such as missing, wrong, or rough and single content. To this end, this paper proposes three strategies to filter and enrich text to improve the quality of text annotation: text filtering using GPT-4, subtitle generation and image retrieval of 2D renderings of 3D models.
#The study proposed three strategies to automatically Filter and enrich noisy text in raw datasets.
##Text filtering and enrichment examples
In each example, the left section shows the thumbnail, original shape name, and GPT-4 filtered results. The upper right part shows the image captions from the two captioning models, while the lower right part shows the retrieved image and its corresponding text.
Expand the three-dimensional backbone network. Since previous work on 3D point cloud learning mainly targeted small-scale 3D data sets like ShapeNet, these backbone networks may not be directly applicable to our large-scale 3D training, and the scale of the backbone network needs to be expanded accordingly. The study found that different 3D backbone networks exhibited different behaviors and scalability when trained on data sets of different sizes. Among them, PointBERT based on Transformer and SparseConv based on three-dimensional convolution show more powerful performance and scalability, so they were selected as the three-dimensional backbone network.
When scaling up the size of the 3D backbone model on the integrated dataset, the Performance and scalability comparison.
Difficult Negative Example Mining: The ensemble dataset of this study exhibits a high degree of class imbalance. Some common categories, like architecture, may occupy tens of thousands of shapes, while many other categories, like walruses and wallets, are underrepresented with only a few dozen or even fewer shapes. Therefore, when batches are randomly constructed for contrastive learning, shapes from two easily confused categories (e.g., apples and cherries) are unlikely to appear in the same batch to be contrasted. To this end, this paper proposes an offline difficult negative example mining strategy to improve training efficiency and performance.
Welcome to try the interactive demo on HuggingFace.The above is the detailed content of Open-world understanding of 3D point clouds, classification, retrieval, subtitles and image generation. For more information, please follow other related articles on the PHP Chinese website!
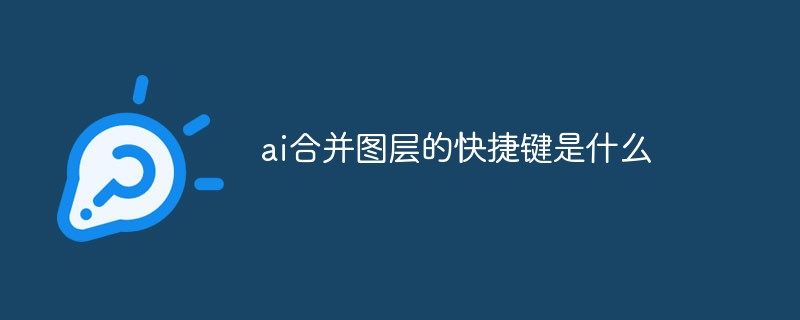
ai合并图层的快捷键是“Ctrl+Shift+E”,它的作用是把目前所有处在显示状态的图层合并,在隐藏状态的图层则不作变动。也可以选中要合并的图层,在菜单栏中依次点击“窗口”-“路径查找器”,点击“合并”按钮。
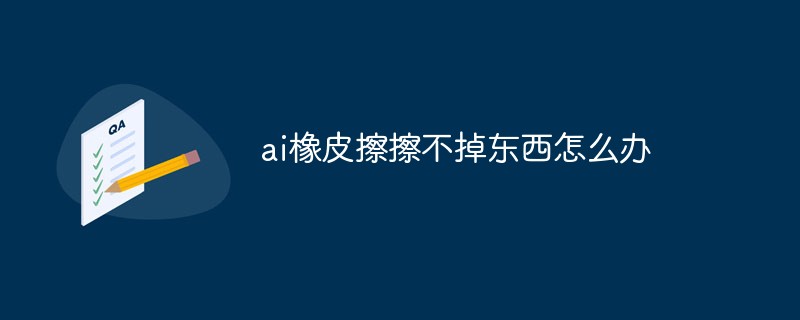
ai橡皮擦擦不掉东西是因为AI是矢量图软件,用橡皮擦不能擦位图的,其解决办法就是用蒙板工具以及钢笔勾好路径再建立蒙板即可实现擦掉东西。
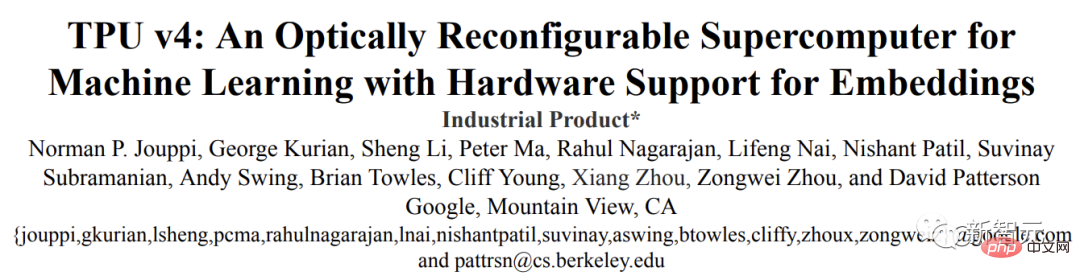
虽然谷歌早在2020年,就在自家的数据中心上部署了当时最强的AI芯片——TPU v4。但直到今年的4月4日,谷歌才首次公布了这台AI超算的技术细节。论文地址:https://arxiv.org/abs/2304.01433相比于TPU v3,TPU v4的性能要高出2.1倍,而在整合4096个芯片之后,超算的性能更是提升了10倍。另外,谷歌还声称,自家芯片要比英伟达A100更快、更节能。与A100对打,速度快1.7倍论文中,谷歌表示,对于规模相当的系统,TPU v4可以提供比英伟达A100强1.
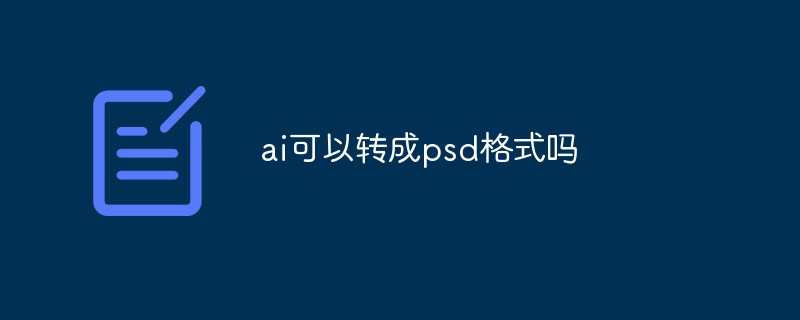
ai可以转成psd格式。转换方法:1、打开Adobe Illustrator软件,依次点击顶部菜单栏的“文件”-“打开”,选择所需的ai文件;2、点击右侧功能面板中的“图层”,点击三杠图标,在弹出的选项中选择“释放到图层(顺序)”;3、依次点击顶部菜单栏的“文件”-“导出”-“导出为”;4、在弹出的“导出”对话框中,将“保存类型”设置为“PSD格式”,点击“导出”即可;
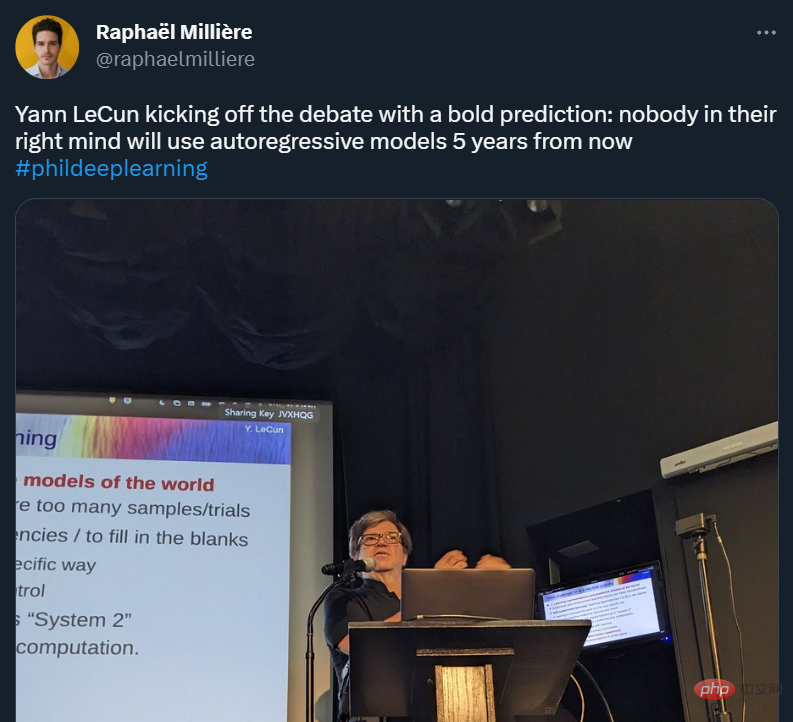
Yann LeCun 这个观点的确有些大胆。 「从现在起 5 年内,没有哪个头脑正常的人会使用自回归模型。」最近,图灵奖得主 Yann LeCun 给一场辩论做了个特别的开场。而他口中的自回归,正是当前爆红的 GPT 家族模型所依赖的学习范式。当然,被 Yann LeCun 指出问题的不只是自回归模型。在他看来,当前整个的机器学习领域都面临巨大挑战。这场辩论的主题为「Do large language models need sensory grounding for meaning and u
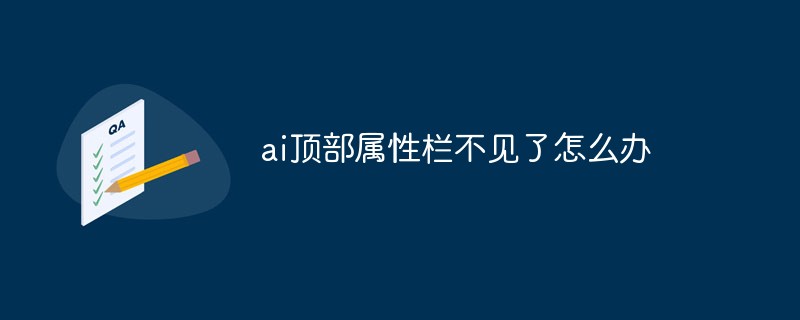
ai顶部属性栏不见了的解决办法:1、开启Ai新建画布,进入绘图页面;2、在Ai顶部菜单栏中点击“窗口”;3、在系统弹出的窗口菜单页面中点击“控制”,然后开启“控制”窗口即可显示出属性栏。
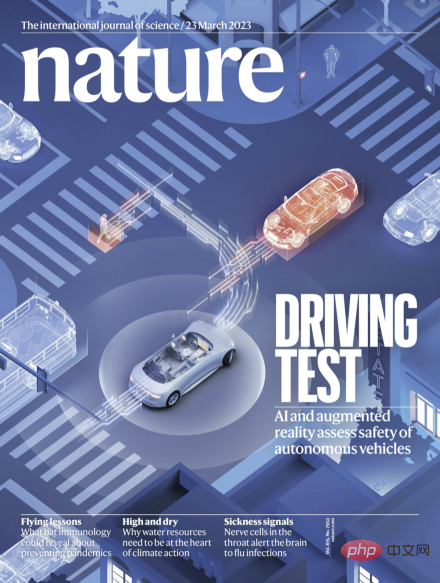
引入密集强化学习,用 AI 验证 AI。 自动驾驶汽车 (AV) 技术的快速发展,使得我们正处于交通革命的风口浪尖,其规模是自一个世纪前汽车问世以来从未见过的。自动驾驶技术具有显着提高交通安全性、机动性和可持续性的潜力,因此引起了工业界、政府机构、专业组织和学术机构的共同关注。过去 20 年里,自动驾驶汽车的发展取得了长足的进步,尤其是随着深度学习的出现更是如此。到 2015 年,开始有公司宣布他们将在 2020 之前量产 AV。不过到目前为止,并且没有 level 4 级别的 AV 可以在市场
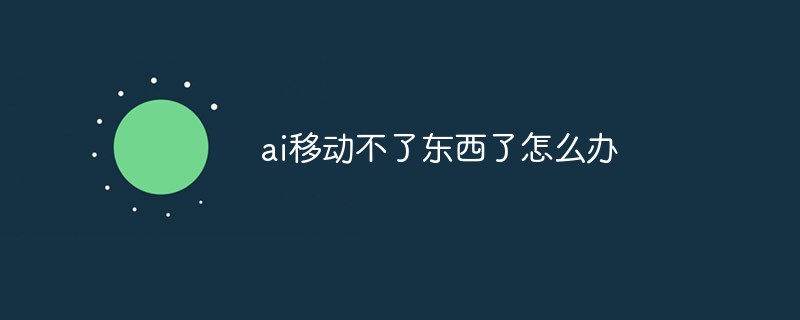
ai移动不了东西的解决办法:1、打开ai软件,打开空白文档;2、选择矩形工具,在文档中绘制矩形;3、点击选择工具,移动文档中的矩形;4、点击图层按钮,弹出图层面板对话框,解锁图层;5、点击选择工具,移动矩形即可。


Hot AI Tools

Undresser.AI Undress
AI-powered app for creating realistic nude photos

AI Clothes Remover
Online AI tool for removing clothes from photos.

Undress AI Tool
Undress images for free

Clothoff.io
AI clothes remover

AI Hentai Generator
Generate AI Hentai for free.

Hot Article

Hot Tools
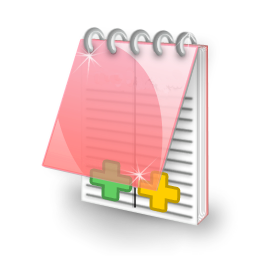
EditPlus Chinese cracked version
Small size, syntax highlighting, does not support code prompt function

SublimeText3 English version
Recommended: Win version, supports code prompts!

MinGW - Minimalist GNU for Windows
This project is in the process of being migrated to osdn.net/projects/mingw, you can continue to follow us there. MinGW: A native Windows port of the GNU Compiler Collection (GCC), freely distributable import libraries and header files for building native Windows applications; includes extensions to the MSVC runtime to support C99 functionality. All MinGW software can run on 64-bit Windows platforms.

SublimeText3 Linux new version
SublimeText3 Linux latest version

SAP NetWeaver Server Adapter for Eclipse
Integrate Eclipse with SAP NetWeaver application server.
