Python is one of the most popular programming languages currently, and its flexibility and scalability make it the tool of choice in the field of data analysis. Among them, Principal Component Analysis (PCA) is a commonly used data dimensionality reduction and feature extraction technology. The implementation and application of PCA in Python will be introduced in detail below.
PCA is a linear dimensionality reduction technique. Its basic idea is to project the original data into a low-dimensional space and retain the most data variance. The advantage of this is that it can reduce the dimensionality of the data, thereby reducing the computational complexity and improving the operating efficiency and generalization ability of the model. In practical applications, PCA is often used in data visualization, feature extraction, data compression and other fields.
Python provides a variety of library functions and toolkits to implement PCA, such as NumPy, SciPy, scikit-learn, etc. The following is a simple example code that shows how to use scikit-learn to perform PCA:
from sklearn.decomposition import PCA import numpy as np # 创建随机样本矩阵 np.random.seed(0) X = np.random.normal(size=(100, 5)) # 创建PCA实例 pca = PCA(n_components=2) # 训练模型并输出结果 X_pca = pca.fit_transform(X) print(X_pca)
The above code first generates a random matrix X with 100 rows and 5 columns, and then uses PCA to reduce its dimensionality are the two principal components, and finally output the dimensionally reduced result X_pca. Here, the core parameter of PCA is n_components, which represents the number of dimensions after dimensionality reduction.
Using PCA for data visualization is one of the important applications. High-dimensional data can usually be visualized as a two-dimensional or three-dimensional scatter plot by projecting the data onto the first two-dimensional principal components. The following is a simple visualization example, using the Iris data set to show the distribution of different types of iris flowers:
import matplotlib.pyplot as plt from sklearn import datasets # 加载Iris数据集 iris = datasets.load_iris() X = iris.data y = iris.target # 使用PCA降维到二维空间 pca = PCA(n_components=2) X_pca = pca.fit_transform(X) # 绘制二维散点图 colors = ['blue', 'red', 'green'] for i in range(len(colors)): plt.scatter(X_pca[y==i, 0], X_pca[y==i, 1], c=colors[i], label=iris.target_names[i]) plt.legend() plt.show()
The above code first loads the Iris data set, and then uses PCA to reduce it to a two-dimensional space. Finally, a scatter plot is used to visualize the distribution of different types of iris flowers in 2D space.
In addition to data visualization, PCA can also be used in fields such as feature extraction and data compression. For example, in image processing, PCA can be used to extract the subject information of an image, thereby reducing the amount of storage and calculation. In text processing, PCA can also be used to reduce the dimensionality of word vectors, thereby reducing the computational complexity of training and prediction models.
In general, PCA technology in Python is a very practical and powerful tool and is widely used in the fields of data analysis and machine learning. By reducing the dimensionality of data and extracting key feature information, it can help us better understand and deal with complex problems in the real world.
The above is the detailed content of What is the principal component analysis technique in Python?. For more information, please follow other related articles on the PHP Chinese website!
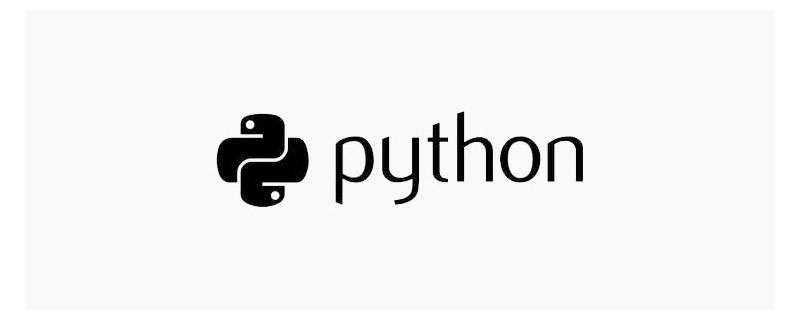
本篇文章给大家带来了关于Python的相关知识,其中主要介绍了关于Seaborn的相关问题,包括了数据可视化处理的散点图、折线图、条形图等等内容,下面一起来看一下,希望对大家有帮助。
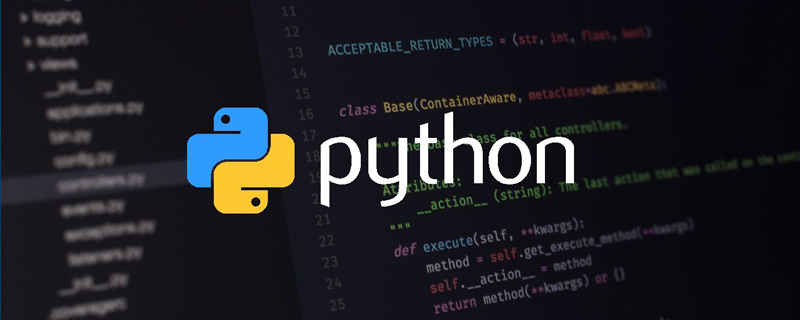
本篇文章给大家带来了关于Python的相关知识,其中主要介绍了关于进程池与进程锁的相关问题,包括进程池的创建模块,进程池函数等等内容,下面一起来看一下,希望对大家有帮助。
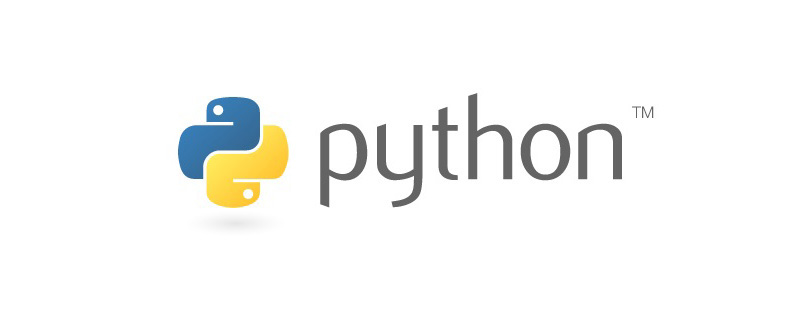
本篇文章给大家带来了关于Python的相关知识,其中主要介绍了关于简历筛选的相关问题,包括了定义 ReadDoc 类用以读取 word 文件以及定义 search_word 函数用以筛选的相关内容,下面一起来看一下,希望对大家有帮助。
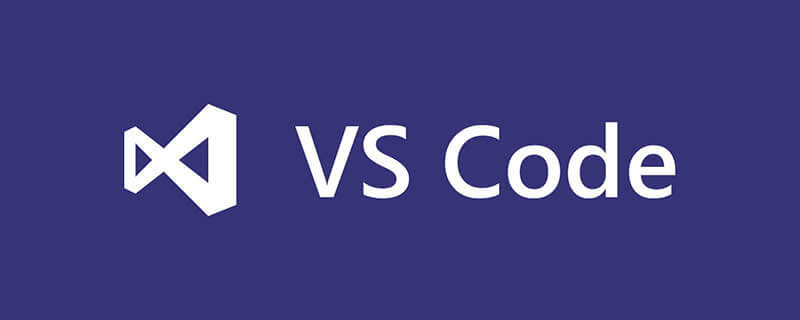
VS Code的确是一款非常热门、有强大用户基础的一款开发工具。本文给大家介绍一下10款高效、好用的插件,能够让原本单薄的VS Code如虎添翼,开发效率顿时提升到一个新的阶段。
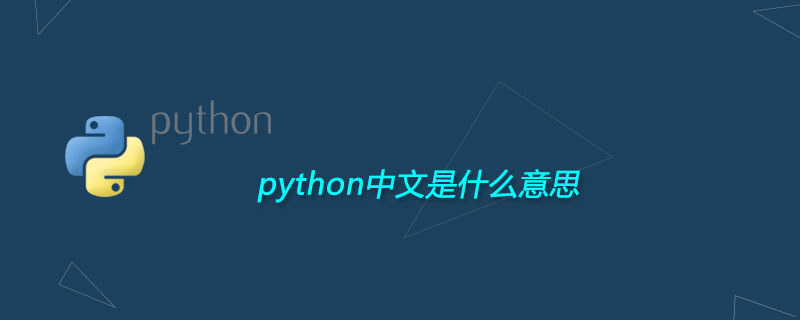
pythn的中文意思是巨蟒、蟒蛇。1989年圣诞节期间,Guido van Rossum在家闲的没事干,为了跟朋友庆祝圣诞节,决定发明一种全新的脚本语言。他很喜欢一个肥皂剧叫Monty Python,所以便把这门语言叫做python。
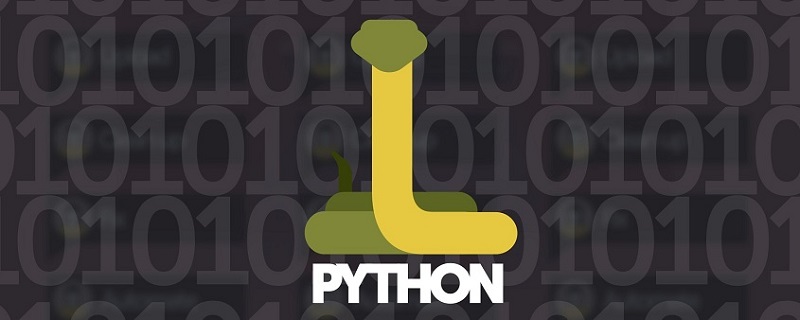
本篇文章给大家带来了关于Python的相关知识,其中主要介绍了关于数据类型之字符串、数字的相关问题,下面一起来看一下,希望对大家有帮助。
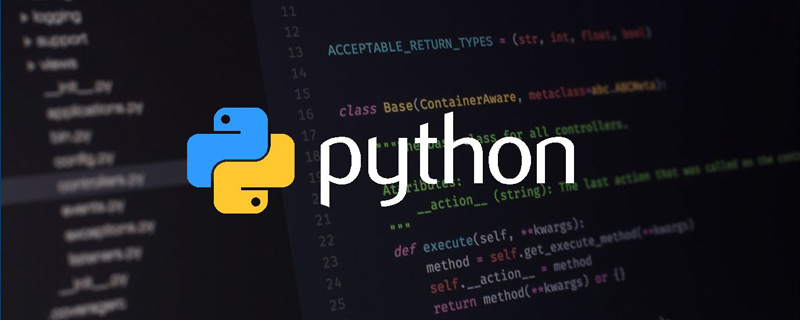
本篇文章给大家带来了关于Python的相关知识,其中主要介绍了关于numpy模块的相关问题,Numpy是Numerical Python extensions的缩写,字面意思是Python数值计算扩展,下面一起来看一下,希望对大家有帮助。


Hot AI Tools

Undresser.AI Undress
AI-powered app for creating realistic nude photos

AI Clothes Remover
Online AI tool for removing clothes from photos.

Undress AI Tool
Undress images for free

Clothoff.io
AI clothes remover

AI Hentai Generator
Generate AI Hentai for free.

Hot Article

Hot Tools
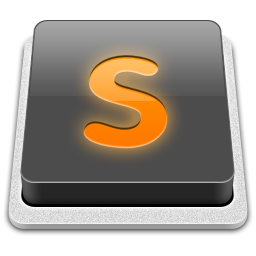
SublimeText3 Mac version
God-level code editing software (SublimeText3)

SublimeText3 Linux new version
SublimeText3 Linux latest version

SecLists
SecLists is the ultimate security tester's companion. It is a collection of various types of lists that are frequently used during security assessments, all in one place. SecLists helps make security testing more efficient and productive by conveniently providing all the lists a security tester might need. List types include usernames, passwords, URLs, fuzzing payloads, sensitive data patterns, web shells, and more. The tester can simply pull this repository onto a new test machine and he will have access to every type of list he needs.
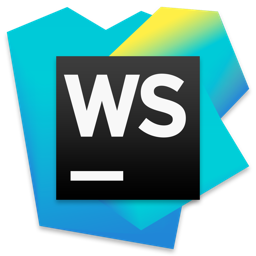
WebStorm Mac version
Useful JavaScript development tools

SublimeText3 English version
Recommended: Win version, supports code prompts!
