


Introduction
Node2vec is a method for graph embedding that can be used for tasks such as node classification, community discovery, and connection prediction.
Implementation process
Loading the dataset
First, let us load the required Python library and execute the following code to load the Cora dataset:
import networkx as nx import numpy as np import pandas as pd import matplotlib.pyplot as plt %matplotlib inline from sklearn.manifold import TSNE from node2vec import Node2Vec # 加载Cora数据集 cora = pd.read_csv('cora/cora.content', sep='\t', header=None) cited_in = pd.read_csv('cora/cora.cites', sep='\t', header=None, names=['target', 'source']) nodes, features = cora.iloc[:, :-1], cora.iloc[:, -1]
Among them, cora.content
contains all node feature information, with a total of 2708 nodes and 1433 features; and cora.cites
creates a node for each node through citation mapping. There are 5429 directed edge relationships between them. Next, we need to merge node features and reference information to build the graph structure.
# 定义函数:构造基于Cora数据集的图结构 def create_graph(nodes, features, cited_in): nodes.index = nodes.index.map(str) graph = nx.from_pandas_edgelist(cited_in, source='source', target='target') for index, row in nodes.iterrows(): node_id = str(row[0]) features = row.drop(labels=[0]) node_attrs = {f'attr_{i}': float(x) for i, x in enumerate(features)} if graph.has_node(node_id) == True: temp = graph.nodes[node_id] temp.update(node_attrs) graph.add_nodes_from([(node_id, temp)]) else: graph.add_nodes_from([(node_id, node_attrs)]) return graph # 构建图 graph = create_graph(nodes, features, cited_in)
This function integrates the node features in cora.content
with the directed edges of cora.cites
and labels them on the graph. Now we have built a graphical view that allows us to visualize our ideas.
Embedding data using Node2vec
In order to perform node feature classification, we need to extract some information from the network and pass it as input to the classifier. One example is to use the node 2 vector method to convert the extracted information into a vector expression so that each node has at least one dimension.
By randomly walking samples from the starting node to the target node, the Node2Vec model learns the vector representing each node. The node 2Vec model defines the transition probabilities between nodes during the random walk.
We will use the node2vec library to generate an embedding representation of the graph and employ a neural network for node classification.
# 定义函数:创建基于Cora数据集的嵌入 def create_embeddings(graph): # 初始化node2vec实例,指定相关超参数 n2v = Node2Vec(graph, dimensions=64, walk_length=30, num_walks=200, p=1, q=1, weight_key='attr_weight') # 基于指定参数训练得到嵌入向量表达式 model = n2v.fit(window=10, min_count=1, batch_words=4) # 获得所有图中节点的嵌入向量 embeddings = pd.DataFrame(model.wv.vectors) ids = list(map(str, model.wv.index2word)) # 将原有的特征和id与新获取到的嵌入向量按行合并 lookup_table = nodes.set_index(0).join(embeddings.set_index(embeddings.index)) return np.array(lookup_table.dropna().iloc[:, -64:]), np.array(list(range(1, lookup_table.shape[0] + 1))) # 创建嵌入向量 cora_embeddings, cora_labels = create_embeddings(graph)
Through the above code, we can obtain the 64-dimensional node embedding expression of each node.
Training classifiers
Next we will specify some classifiers and train them on the Cora dataset in order to perform accurate node classification operations based on embeddings.
from sklearn import svm, model_selection, metrics # 使用支持向量机作为示范的分类器 svm_model = svm.SVC(kernel='rbf', C=1, gamma=0.01) # 进行交叉验证和分类训练 scores = model_selection.cross_val_score( svm_model, cora_embeddings, cora_labels, cv=5) print(scores.mean())
In order to obtain better performance, when the support vector machine is used as a classifier, we also need to perform related parameter adjustment operations. Here, a 5-fold cross-validation method is used to evaluate its performance.
Visualized Node Embedding
In order to better understand, we need to reduce the dimensionality of the 64-dimensional feature expression that is difficult for humans to understand to achieve visualization. t-SNE is a method specifically designed to reduce the complexity of high-dimensional data, and we use it here. It generates a two-dimensional graph in which similar nodes are closely clustered together, and this graph is achieved by outputting a probability distribution vector containing only two elements.
# 定义函数:可视化Nodes2Vec的结果 def visualize_results(embeddings, labels): # 使用t-SNE对数据进行降维并绘图 tsne = TSNE(n_components=2, verbose=1, perplexity=40, n_iter=300) tsne_results = tsne.fit_transform(embeddings) plt.figure(figsize=(10, 5)) plt.scatter(tsne_results[:,0], tsne_results[:,1], c=labels) plt.colorbar() plt.show() # 可视化结果 visualize_results(cora_embeddings, cora_labels)
The embedding vector generated by Node2Vec will be input into t-SNE, where t-SNE reduces the dimensionality of the 64-dimensional vector expression and outputs a two-dimensional scatter plot that we can visualize using the matplotlib library. Whether most relevant nodes are tightly clustered can be checked in the graphical interface.
The above is the detailed content of How to implement node classification and visualization in python based on Node2Vec. For more information, please follow other related articles on the PHP Chinese website!
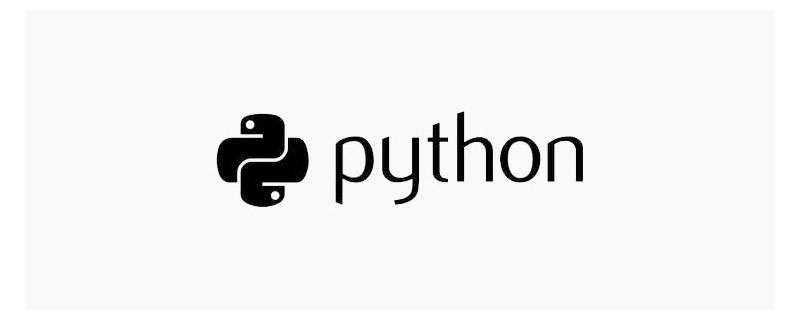
本篇文章给大家带来了关于Python的相关知识,其中主要介绍了关于Seaborn的相关问题,包括了数据可视化处理的散点图、折线图、条形图等等内容,下面一起来看一下,希望对大家有帮助。
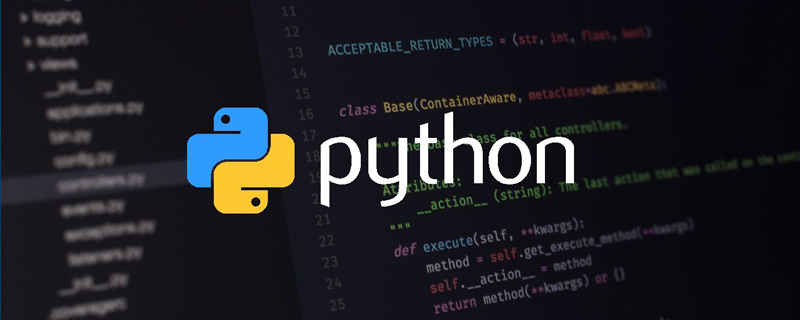
本篇文章给大家带来了关于Python的相关知识,其中主要介绍了关于进程池与进程锁的相关问题,包括进程池的创建模块,进程池函数等等内容,下面一起来看一下,希望对大家有帮助。
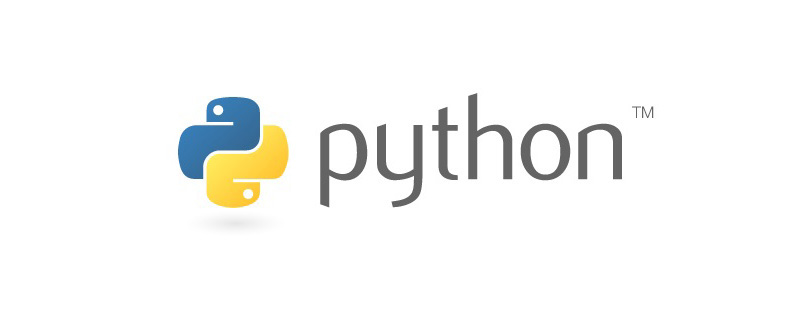
本篇文章给大家带来了关于Python的相关知识,其中主要介绍了关于简历筛选的相关问题,包括了定义 ReadDoc 类用以读取 word 文件以及定义 search_word 函数用以筛选的相关内容,下面一起来看一下,希望对大家有帮助。
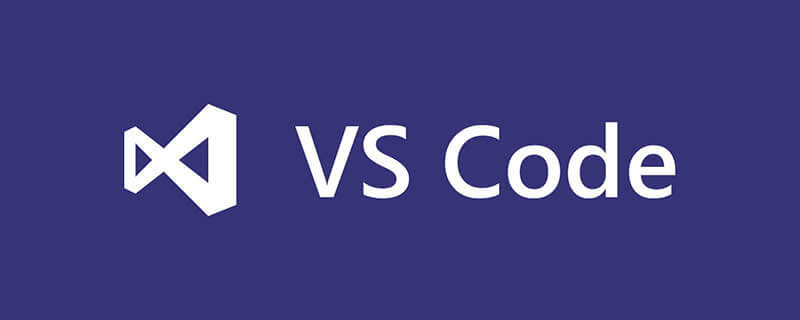
VS Code的确是一款非常热门、有强大用户基础的一款开发工具。本文给大家介绍一下10款高效、好用的插件,能够让原本单薄的VS Code如虎添翼,开发效率顿时提升到一个新的阶段。
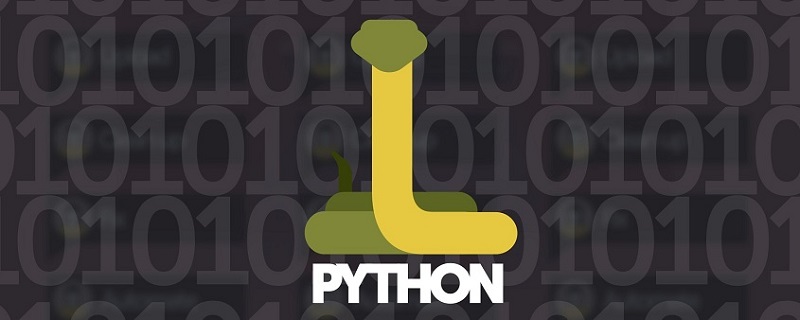
本篇文章给大家带来了关于Python的相关知识,其中主要介绍了关于数据类型之字符串、数字的相关问题,下面一起来看一下,希望对大家有帮助。
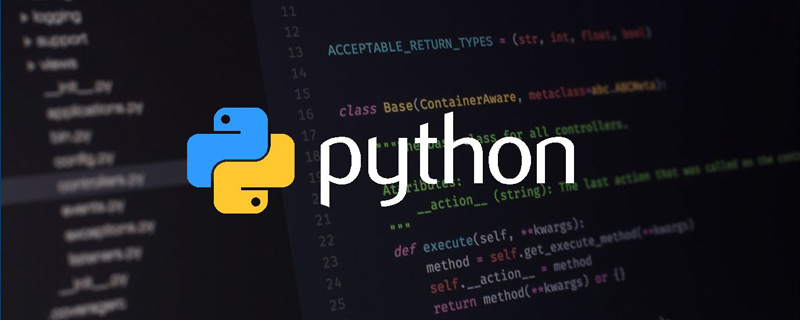
本篇文章给大家带来了关于Python的相关知识,其中主要介绍了关于numpy模块的相关问题,Numpy是Numerical Python extensions的缩写,字面意思是Python数值计算扩展,下面一起来看一下,希望对大家有帮助。
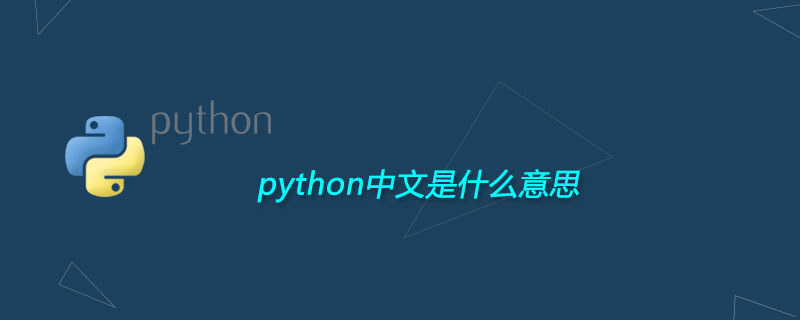
pythn的中文意思是巨蟒、蟒蛇。1989年圣诞节期间,Guido van Rossum在家闲的没事干,为了跟朋友庆祝圣诞节,决定发明一种全新的脚本语言。他很喜欢一个肥皂剧叫Monty Python,所以便把这门语言叫做python。


Hot AI Tools

Undresser.AI Undress
AI-powered app for creating realistic nude photos

AI Clothes Remover
Online AI tool for removing clothes from photos.

Undress AI Tool
Undress images for free

Clothoff.io
AI clothes remover

AI Hentai Generator
Generate AI Hentai for free.

Hot Article

Hot Tools
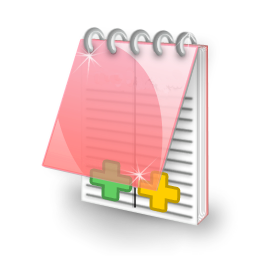
EditPlus Chinese cracked version
Small size, syntax highlighting, does not support code prompt function

MantisBT
Mantis is an easy-to-deploy web-based defect tracking tool designed to aid in product defect tracking. It requires PHP, MySQL and a web server. Check out our demo and hosting services.

Safe Exam Browser
Safe Exam Browser is a secure browser environment for taking online exams securely. This software turns any computer into a secure workstation. It controls access to any utility and prevents students from using unauthorized resources.
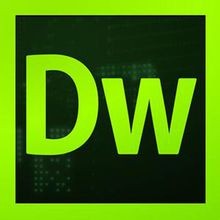
Dreamweaver CS6
Visual web development tools

PhpStorm Mac version
The latest (2018.2.1) professional PHP integrated development tool
