


How to invest in AI? 'Three major focus issues” faced by global first-tier VCs
At present, artificial intelligence is ushering in the "iPhone moment", and its spread is faster than any technological revolution in history.
However, Morgan Stanley pointed out in the report that it is undeniable that the uncertainty of AI development is high. VCs have keenly discovered this and pointed out the following "three major focus issues."
- AI is growing significantly and is in urgent need of modularization
The AI industry is developing rapidly, and the speed of "technology diffusion" exceeds that of the Internet revolution. Modularity is the key to achieving faster growth in AI.
"Tech Diffusion" (Tech Diffusion) is one of the most important themes in recent years. It is the process from when a technology is first commercialized, through vigorous promotion and widespread adoption, until it is finally eliminated due to backwardness.
What is unprecedented is the speed and spillover effect of the diffusion of artificial intelligence technology into non-technical adjacent fields. To illustrate this point more clearly, the chart below compares the technology diffusion speed of the electricity revolution after 1885, the Internet revolution after 2007, and the artificial intelligence revolution after 2022.
Among them, modularity (Modularity sub-modules that specialize in different aspects of tasks) is the key to achieving faster growth and disruption through the innovation stack. The continued growth of AI depends on widespread internet access, which in turn requires cheap electricity to make possible. These large models of artificial intelligence will be based on modular forms and are equally applicable to areas of rapid growth and disruption in the future.
As more and more different tasks are encountered, the performance of AI is getting worse and worse, because model training cannot cover all scenarios. This is also the reason why most mainstream AI products rely on prompt words to give relatively logical answers. "Modularization", that is, dividing modules into specialized tasks to handle different aspects, is one of the solutions to the generalization problem. )
For example, open source plug-ins for companies with large models, such as OpenAI's newly released data analysis tool "Code Interpreter", will benefit from this modular expansion method and will create greater breadth, depth and stickiness of use. However, the rapid pace of adoption relative to any technology in history also means that Generative AI’s S-curve will only take months, rather than the years or decades expected in the past.
- AI company valuations decline and extreme differentiation occurs
The 80/20 rule also exists in the financing and valuation of AI companies (80% of a company’s profits come from 20% of its projects). OpenAI recently raised another US$300 million, and its valuation is between US$27 billion and US$29 billion. . The company has raised a total of more than $11 billion in funding over the past seven rounds.
There is currently no competitor that can match OpenAI’s ChatGPT. Recent platform data shows that its number of monthly active users exceeds Reddit, Netflix and Linkedin, and is close to 2 billion.
However, on average, AI/ML company valuations are 60% lower than their valuation levels in January 2021, when AI/ML was in the midst of the hype cycle. Despite the clear growth in investment demand for AI (accounting for 10% of all venture capital investment), only a few private AI companies have passed the revaluation, and OpenAI is one such company.
- Open source large models may challenge financing models
The most heated question in recent weeks is how big is the moat of large model companies in the face of open source models?
As of 2023, investment funds in the AI field have exceeded US$12 billion, accounting for 10% of the total market venture capital. Despite re-achieving the Pareto point, 80% of the funding currently remains in the hands of large model owners rather than downstream APP manufacturers. The diffusion into non-tech industries has since accelerated.
Of course, there is a good reason - training larger and larger LLMs is expensive, and leveraging those models with APIs to create downstream applications is cheaper, and that seems to be the case now.
Will the emergence of open source LLM cause this capital deployment ratio to reverse at some point - whether in the public market or the private market, will financing be conducive to the emergence of low-cost open source LLM?
The above is the detailed content of How to invest in AI? 'Three major focus issues” faced by global first-tier VCs. For more information, please follow other related articles on the PHP Chinese website!
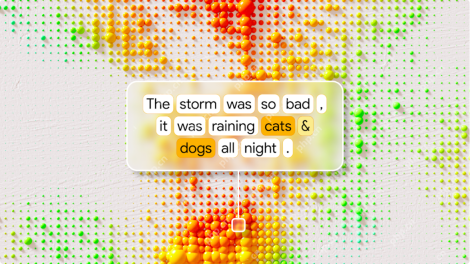
Exploring the Inner Workings of Language Models with Gemma Scope Understanding the complexities of AI language models is a significant challenge. Google's release of Gemma Scope, a comprehensive toolkit, offers researchers a powerful way to delve in
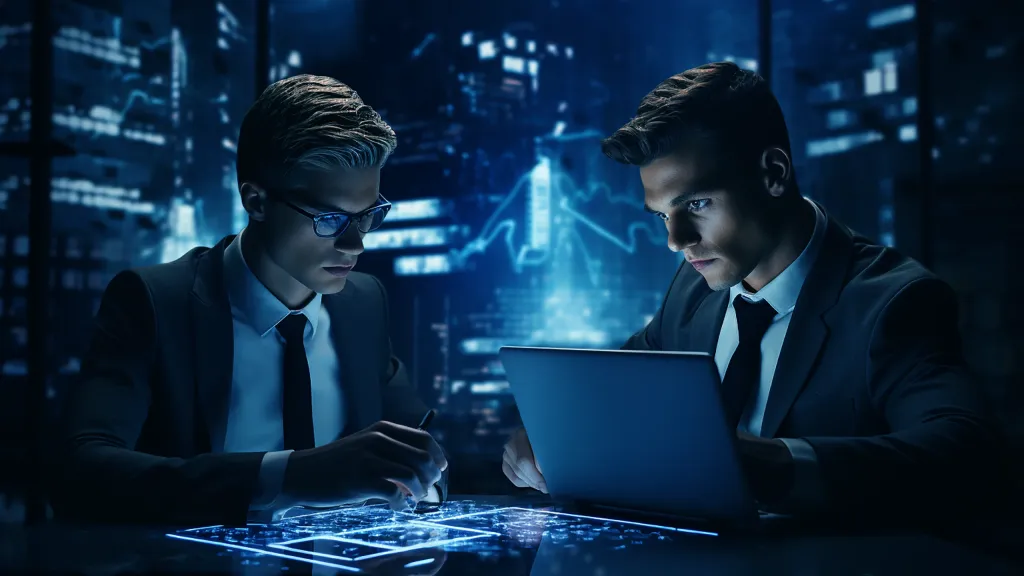
Unlocking Business Success: A Guide to Becoming a Business Intelligence Analyst Imagine transforming raw data into actionable insights that drive organizational growth. This is the power of a Business Intelligence (BI) Analyst – a crucial role in gu

SQL's ALTER TABLE Statement: Dynamically Adding Columns to Your Database In data management, SQL's adaptability is crucial. Need to adjust your database structure on the fly? The ALTER TABLE statement is your solution. This guide details adding colu
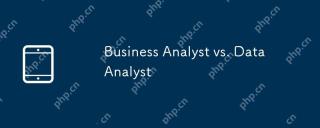
Introduction Imagine a bustling office where two professionals collaborate on a critical project. The business analyst focuses on the company's objectives, identifying areas for improvement, and ensuring strategic alignment with market trends. Simu
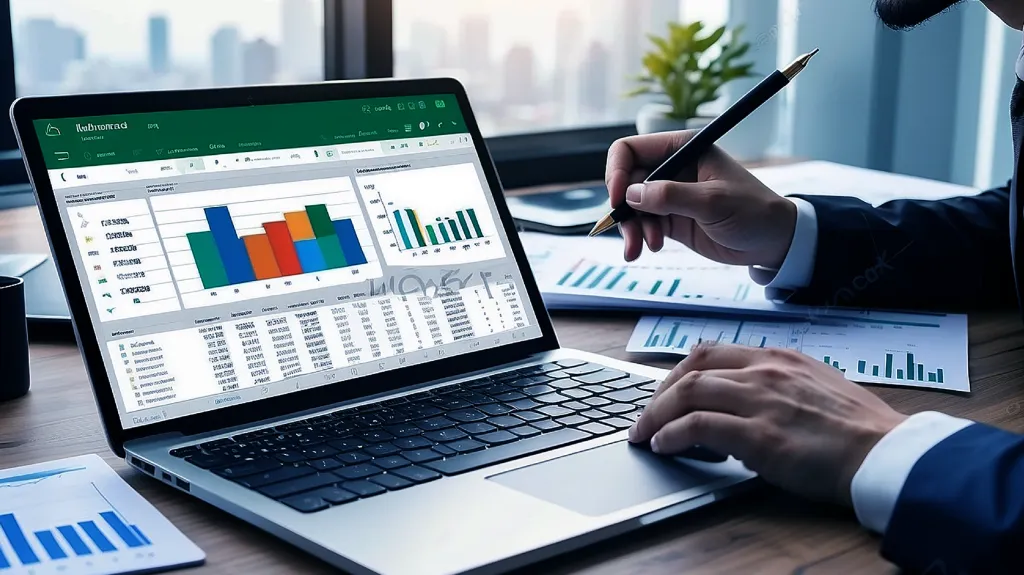
Excel data counting and analysis: detailed explanation of COUNT and COUNTA functions Accurate data counting and analysis are critical in Excel, especially when working with large data sets. Excel provides a variety of functions to achieve this, with the COUNT and COUNTA functions being key tools for counting the number of cells under different conditions. Although both functions are used to count cells, their design targets are targeted at different data types. Let's dig into the specific details of COUNT and COUNTA functions, highlight their unique features and differences, and learn how to apply them in data analysis. Overview of key points Understand COUNT and COU
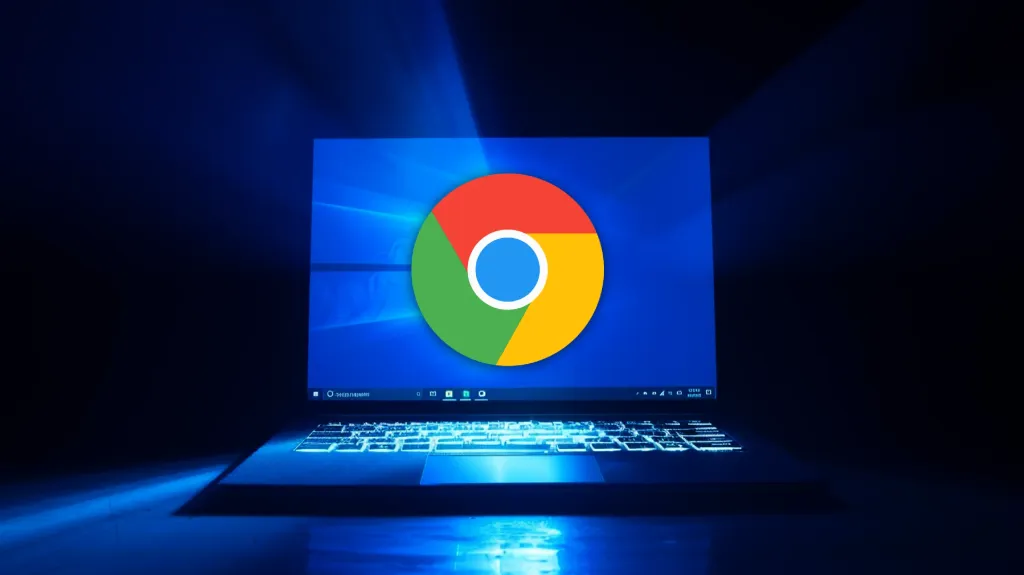
Google Chrome's AI Revolution: A Personalized and Efficient Browsing Experience Artificial Intelligence (AI) is rapidly transforming our daily lives, and Google Chrome is leading the charge in the web browsing arena. This article explores the exciti
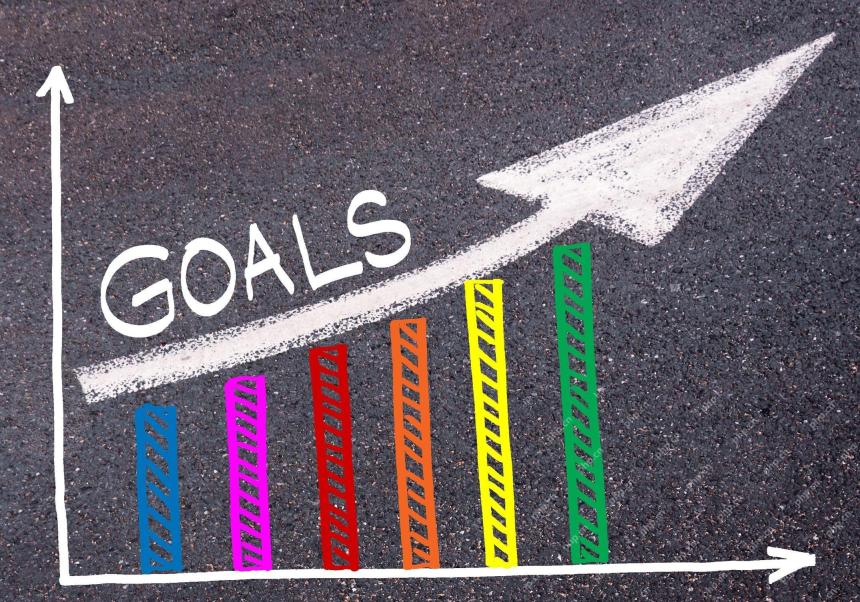
Reimagining Impact: The Quadruple Bottom Line For too long, the conversation has been dominated by a narrow view of AI’s impact, primarily focused on the bottom line of profit. However, a more holistic approach recognizes the interconnectedness of bu
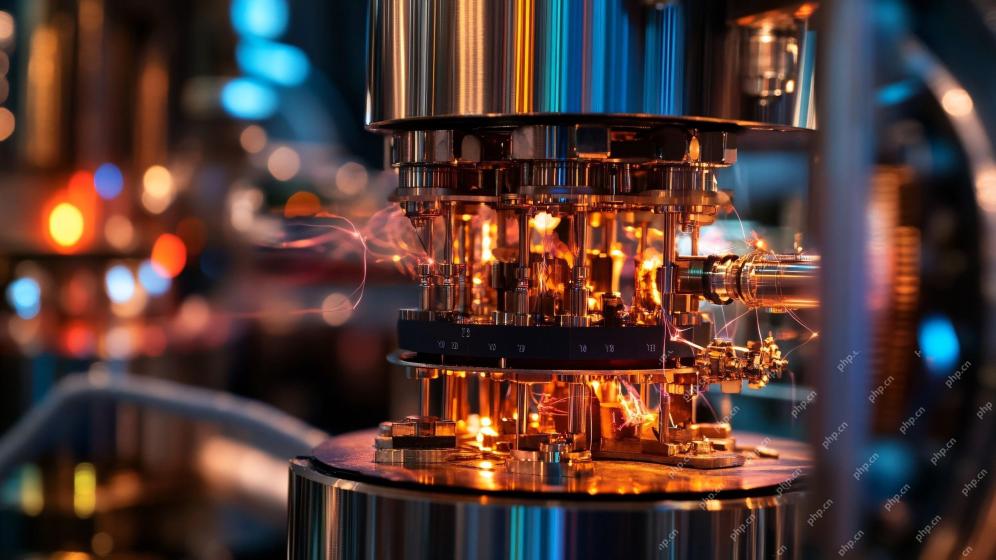
Things are moving steadily towards that point. The investment pouring into quantum service providers and startups shows that industry understands its significance. And a growing number of real-world use cases are emerging to demonstrate its value out


Hot AI Tools

Undresser.AI Undress
AI-powered app for creating realistic nude photos

AI Clothes Remover
Online AI tool for removing clothes from photos.

Undress AI Tool
Undress images for free

Clothoff.io
AI clothes remover

AI Hentai Generator
Generate AI Hentai for free.

Hot Article

Hot Tools

mPDF
mPDF is a PHP library that can generate PDF files from UTF-8 encoded HTML. The original author, Ian Back, wrote mPDF to output PDF files "on the fly" from his website and handle different languages. It is slower than original scripts like HTML2FPDF and produces larger files when using Unicode fonts, but supports CSS styles etc. and has a lot of enhancements. Supports almost all languages, including RTL (Arabic and Hebrew) and CJK (Chinese, Japanese and Korean). Supports nested block-level elements (such as P, DIV),

Safe Exam Browser
Safe Exam Browser is a secure browser environment for taking online exams securely. This software turns any computer into a secure workstation. It controls access to any utility and prevents students from using unauthorized resources.
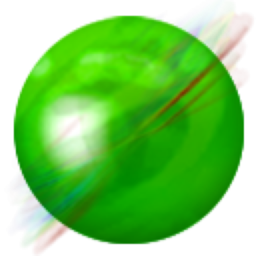
ZendStudio 13.5.1 Mac
Powerful PHP integrated development environment
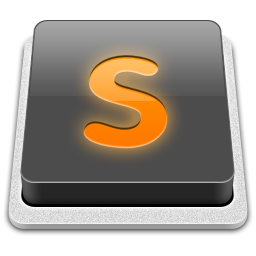
SublimeText3 Mac version
God-level code editing software (SublimeText3)
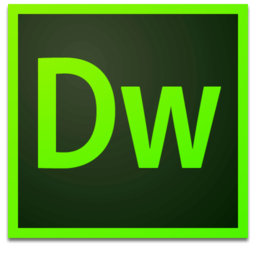
Dreamweaver Mac version
Visual web development tools