1. What is BitMap
Use a bit to represent the value or status of an element, and the element itself is the key. Bitmap can greatly save storage space, because we know that 8 bits can form a Byte. 2^32 power 4 billion data only requires 500M of memory, which is 8 times less memory
2. Introduction to the setbit command
setbit key offset value #设置bitmapkey为20220328 uid为100的用户已签到1 setbit 20220320 100 1 setbit 20220320 200 1 setbit 20220321 100 1 setbit 20220321 300 1 getbit 20220320 100 #返回1,说明这个用户已签到了 bitcount 20220320 #获取bitmap数量
bitmap pitfalls
127.0.0.1:6400> setbit bittest 100 1 #设置不存在的offset返回0 (integer) 0 127.0.0.1:6400> setbit bittest 100 1 #设置已存在的offset返回1 (integer) 1
setbit maxKey 4000000000 1 #Directly get you more than 600M memory
/** * 布隆过滤器bloom Filter * 1.百万分之一的概率哈希冲突,所以有存在的不一定存在,但是不存在的百分百不存在 * 2.不能删除,删除的时候不能简单的直接置为0,可能会影响其他元素的判断,其实问题不大一般生产数据也不会删除的,都是软删除 * 3.新增数据时候写入bloom Filter * 4.2^32次方40亿数据内存占用才600M,超级省内存,查找速度非常快,160M内存可以在千万级数据做到1%的误判 * 5.bitmap根据offset去申请内存的,所以要省内存的情况要限制offset值 */ public function bloomAction(){ $t1 = time(); for($i=0;$i<99;$i++){ $bl = new BloomFilter(); //$str = "1https://arnaud.le-blanc.net/php-rdkafka-doc/phpdoc/book.rdkafka.html?id=".time(); $str = "https://dasda.le-blanc.net/php-rdkafka-doc/phpdoc/book.rdkafka.html?id=".mt_rand(1,99999999); p($str); $res1 = $bl->JSHash($str);//两次哈希3s,md5哈希重复的概率是百万分之一 p($res1); } //p($res); $t2 = time(); echo $t2-$t1; } /** * 布隆过滤器初始化 bloom Filter 执行 php index.php "index/demo/loadDb2bloom" */ public function isExistBloomAction(){ $redis = redisCursor(); $email = input("email","","trim"); $tel = input("tel",""); $result = false; $msg = ""; if(filter_var($email,FILTER_VALIDATE_EMAIL)){ $key1 = "bloom_user_email"; $offset = BloomFilter::JSHash($email); $result = $redis->getbit($key1,$offset); $msg = $email; }elseif($tel){ $key2 = "bloom_user_telephone"; $offset = BloomFilter::JSHash($tel); $result = $redis->getbit($key2,$offset); $msg = $tel; } $result?apiSuccess($msg.",已存在"):apiError($msg.",不存在"); } /** * 布隆过滤器初始化 bloom Filter 执行 php index.php "index/demo/loadDb2bloom" */ public function loadDb2bloomAction(){ $time1 = time(); $redis = redisCursor(); $key1 = "bloom_user_email"; $key2 = "bloom_user_telephone"; //setbit() offset 必须是数字,value必须是1或0 //$redis->setbit($key,30,1); $table = "user"; $pkid = "id"; $field1 = "email"; $field2 = "telephone"; $maxid = Db::name($table)->max($pkid); $size = 5000; $page = ceil($maxid/$size); for($i=0;$i<$page;$i++){ $start = $i*$size; $where = " $pkid between ".$start." and ".($start+$size); $res = Db::name($table)->where($where)->field("$field1,$field2")->select(); if($res){//同步到bitmap foreach($res as $k=>$v){ //布隆过滤器 1.存在的不一定存在, 2.不存在的100%不存在(原因,哈希冲突可能用100W分之一的可能重复) //所以注册的时候判断不存在的,百分百可以注册,存在的可以查询一下数据库是否真的不存在 $value1 = BloomFilter::JSHash($v["$field1"]); $value2 = BloomFilter::JSHash($v["$field2"]); $redis->setbit($key1,$value1,1);//email去重 $redis->setbit($key2,$value2,1);//mobile去重 } } $time2 = time(); echo $where." 消耗时间 ".($time2-$time1).PHP_EOL; } $time3 = time(); echo " 总消耗时间 ".($time3-$time1).PHP_EOL; }
<?php class BloomFilter { /** * 下面的哈希函数随便用一个都行,都是把字符串转换成数字 */ /** * hash方法类 * 由Justin Sobel编写的按位散列函数 * update:Denny * 返回之前做了内存限制在160M,超过10亿的哈希后的数值,把它限制在10亿内,此时1000W的数据可做到1%误判,内存不差这600多M的话就别限制了 * 因为redis的bitmap申请内存是看offset申请内存的,setbit mykey 400000000 1,这样直接申请了600M内存 */ public static function JSHash($string, $limitMemory=true,$len = null) { $hash = 1315423911; $len || $len = strlen($string); for($i = 0; $i < $len; $i++) { $hash ^= (($hash << 5) + ord($string[$i]) + ($hash >> 2)); } $hashNum = ($hash % 0xFFFFFFFF) & 0xFFFFFFFF; //为了节省内存,超过10亿就对半拆,10亿,这时候大约是130M内存占用,千万级数据可以做到1%误判率,内存足够可以不用判断,直接生成就行了 //如果数据过4000W的话不用限制了,因为生成的数据最大也是2^32次方40多亿,此时内存占用大概在600M封顶了 if($limitMemory){ if($hashNum>4000000000){ $hashNum = intval($hashNum/5); }elseif($hashNum>3000000000){ $hashNum = intval($hashNum/4); }elseif($hashNum>2000000000){ $hashNum = intval($hashNum/3); } } return $hashNum; } }
The above is the detailed content of Analysis of bitmap examples in redis. For more information, please follow other related articles on the PHP Chinese website!
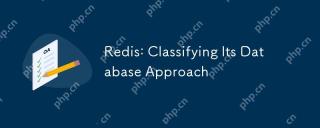
Redis's database methods include in-memory databases and key-value storage. 1) Redis stores data in memory, and reads and writes fast. 2) It uses key-value pairs to store data, supports complex data structures such as lists, collections, hash tables and ordered collections, suitable for caches and NoSQL databases.
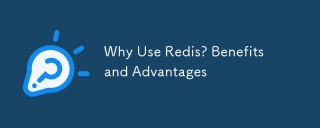
Redis is a powerful database solution because it provides fast performance, rich data structures, high availability and scalability, persistence capabilities, and a wide range of ecosystem support. 1) Extremely fast performance: Redis's data is stored in memory and has extremely fast read and write speeds, suitable for high concurrency and low latency applications. 2) Rich data structure: supports multiple data types, such as lists, collections, etc., which are suitable for a variety of scenarios. 3) High availability and scalability: supports master-slave replication and cluster mode to achieve high availability and horizontal scalability. 4) Persistence and data security: Data persistence is achieved through RDB and AOF to ensure data integrity and reliability. 5) Wide ecosystem and community support: with a huge ecosystem and active community,
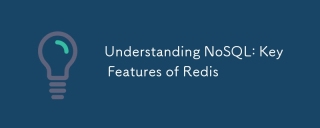
Key features of Redis include speed, flexibility and rich data structure support. 1) Speed: Redis is an in-memory database, and read and write operations are almost instantaneous, suitable for cache and session management. 2) Flexibility: Supports multiple data structures, such as strings, lists, collections, etc., which are suitable for complex data processing. 3) Data structure support: provides strings, lists, collections, hash tables, etc., which are suitable for different business needs.
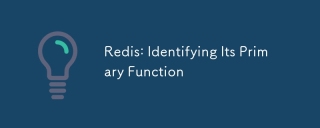
The core function of Redis is a high-performance in-memory data storage and processing system. 1) High-speed data access: Redis stores data in memory and provides microsecond-level read and write speed. 2) Rich data structure: supports strings, lists, collections, etc., and adapts to a variety of application scenarios. 3) Persistence: Persist data to disk through RDB and AOF. 4) Publish subscription: Can be used in message queues or real-time communication systems.

Redis supports a variety of data structures, including: 1. String, suitable for storing single-value data; 2. List, suitable for queues and stacks; 3. Set, used for storing non-duplicate data; 4. Ordered Set, suitable for ranking lists and priority queues; 5. Hash table, suitable for storing object or structured data.
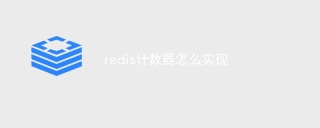
Redis counter is a mechanism that uses Redis key-value pair storage to implement counting operations, including the following steps: creating counter keys, increasing counts, decreasing counts, resetting counts, and obtaining counts. The advantages of Redis counters include fast speed, high concurrency, durability and simplicity and ease of use. It can be used in scenarios such as user access counting, real-time metric tracking, game scores and rankings, and order processing counting.
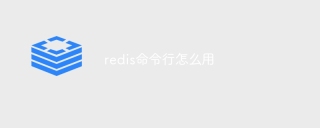
Use the Redis command line tool (redis-cli) to manage and operate Redis through the following steps: Connect to the server, specify the address and port. Send commands to the server using the command name and parameters. Use the HELP command to view help information for a specific command. Use the QUIT command to exit the command line tool.
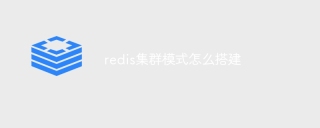
Redis cluster mode deploys Redis instances to multiple servers through sharding, improving scalability and availability. The construction steps are as follows: Create odd Redis instances with different ports; Create 3 sentinel instances, monitor Redis instances and failover; configure sentinel configuration files, add monitoring Redis instance information and failover settings; configure Redis instance configuration files, enable cluster mode and specify the cluster information file path; create nodes.conf file, containing information of each Redis instance; start the cluster, execute the create command to create a cluster and specify the number of replicas; log in to the cluster to execute the CLUSTER INFO command to verify the cluster status; make


Hot AI Tools

Undresser.AI Undress
AI-powered app for creating realistic nude photos

AI Clothes Remover
Online AI tool for removing clothes from photos.

Undress AI Tool
Undress images for free

Clothoff.io
AI clothes remover

AI Hentai Generator
Generate AI Hentai for free.

Hot Article

Hot Tools

PhpStorm Mac version
The latest (2018.2.1) professional PHP integrated development tool

SublimeText3 English version
Recommended: Win version, supports code prompts!
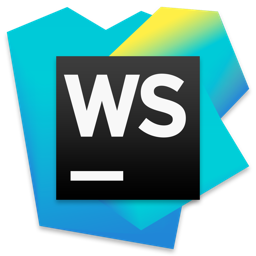
WebStorm Mac version
Useful JavaScript development tools

SAP NetWeaver Server Adapter for Eclipse
Integrate Eclipse with SAP NetWeaver application server.

Zend Studio 13.0.1
Powerful PHP integrated development environment