


Recently, a netizen compiled a list of "Numbers that every LLM developer should know" and explained why these numbers are important and how we should use them.
When he was at Google, there was a document compiled by legendary engineer Jeff Dean called "Numbers Every Engineer Should Know."
Jeff Dean: "Numbers Every Engineer Should Know"
For LLM (Large Language Model) developers, it is also very useful to have a similar set of numbers for rough estimation.
Prompt
##40-90%: Add "concise" to the prompt The subsequent cost savings
#You must know that you pay according to the token used by LLM during output.
This means that you can save a lot of money by letting your model be concise.
At the same time, this concept can be expanded to more places.
For example, you originally wanted to use GPT-4 to generate 10 alternatives, but now you may be able to ask it to provide 5 first, and then you can keep the other half of the money.
1.3: The average number of tokens per word
LLM operates in token units.
And a token is a word or a subpart of a word. For example, "eating" may be decomposed into two tokens "eat" and "ing".
Generally speaking, 750 English words will generate about 1000 tokens.
For languages other than English, the number of tokens per word will be increased, depending on their commonality in LLM’s embedding corpus.
Considering that the cost of using LLM is very high, the numbers related to the price are has become particularly important.
~50: Cost ratio of GPT-4 and GPT-3.5 Turbo
Using GPT-3.5-Turbo About 50 times cheaper than GPT-4. I say "approximately" because GPT-4 charges differently for prompts and generation.
So in actual application, it is best to confirm whether GPT-3.5-Turbo is enough to meet your needs.
For example, for tasks like summarizing, GPT-3.5-Turbo is more than sufficient.
##5: Use GPT- 3.5-Turbo vs. OpenAI Embedding Cost Ratio for Text Generation
This means that looking up something in a vector storage system is much cheaper than using generation with LLM. Specifically, searching in the neural information retrieval system costs about 5 times less than asking GPT-3.5-Turbo. Compared with GPT-4, the cost gap is as high as 250 times! 10: Cost Ratio of OpenAI Embeds vs. Self-Hosted Embeds Note: This number is very sensitive to load and embedding batch sizes are very sensitive, so consider them as approximations. With g4dn.4xlarge (on-demand price: $1.20/hour) we can leverage SentenceTransformers with HuggingFace (comparable to OpenAI’s embeddings) at ~9000 per second The speed of token embedding. Doing some basic calculations at this speed and node type shows that self-hosted embeds can be 10x cheaper. 6: Cost ratio of OpenAI basic model and fine-tuned model query On OpenAI, the cost of fine-tuned model 6 times that of the base model. This also means that it is more cost-effective to adjust the base model's prompts than to fine-tune a custom model. 1: Cost ratio of self-hosting base model vs. fine-tuned model query If you host the model yourself, then The cost of the fine-tuned model is almost the same as that of the base model: the number of parameters is the same for both models. ~$1 million: the cost of training a 13 billion parameter model on 1.4 trillion tokens Paper address: https://arxiv.org/pdf/2302.13971.pdf ##LLaMa’s The paper mentioned that it took them 21 days and used 2048 A100 80GB GPUs to train the LLaMa model. Assuming we train our model on the Red Pajama training set, assuming everything works fine, without any crashes, and it succeeds the first time, we will get the above numbers. In addition, this process also involves coordination between 2048 GPUs. Most companies do not have the conditions to do this. However, the most critical message is: it is possible to train our own LLM, but the process is not cheap. And every time it is run, it takes several days. In comparison, using a pre-trained model will be much cheaper. ##This number is a bit general, in general Generally speaking, the cost of fine-tuning is negligible. For example, you can fine-tune a 6B parameter model for about $7.
Training and fine-tuning
This means that if you want to fine-tune Shakespeare's entire work (about 1 million words), you only need to spend forty or fifty dollars.
However, fine-tuning is one thing, training from scratch is another...
GPU memory
If you are self-hosting the model, it is very important to understand the GPU memory, because LLM is pushing the GPU memory to the limit.The following statistics are used specifically for inference. If you want to do training or fine-tuning, you need quite a bit of video memory. V100: 16GB, A10G: 24GB, A100: 40/80GB: GPU memory capacity Understand the different types The amount of video memory your GPU has is important as this will limit the amount of parameters your LLM can have. Generally speaking, we like to use A10G because they are priced at $1.5 to $2 per hour on AWS on demand and have 24G of GPU memory, while each A100 The price is approximately $5/hour. 2x Parameter amount: Typical GPU memory requirements of LLM For example, when you have a 7 billion Parametric model requires approximately 14GB of GPU memory. This is because in most cases each argument requires a 16-bit floating point number (or 2 bytes). Usually you don't need more than 16 bits of precision, but most of the time the resolution starts to decrease when the precision reaches 8 bits (and in some cases this is acceptable). Of course, there are some projects that have improved this situation. For example, llama.cpp ran through a 13 billion parameter model by quantizing to 4 bits on a 6GB GPU (8 bits are also acceptable), but this is not common. ~1GB: Typical GPU memory requirements for embedding models Whenever you embed statements (clustering, semantics (which is often done for search and classification tasks), you need an embedding model like a sentence converter. OpenAI also has its own commercial embedding model.
Usually you don’t have to worry about how much video memory embedding takes up on the GPU, they are quite small and you can even embed LLM on the same GPU .
>10x: Improve throughput by batching LLM requests
##Latency of running LLM queries through GPU Very high: At a throughput of 0.2 queries per second, the latency may be 5 seconds.
Interestingly, if you run two tasks, the delay may only be 5.2 seconds.
This means that if you can bundle 25 queries together, you will need about 10 seconds of latency, and the throughput has been increased to 2.5 queries per second.
However, please read on.
~1 MB: GPU memory required for the 13 billion parameter model to output 1 token
What you need The amount of video memory is directly proportional to the maximum number of tokens you want to generate.
For example, generating output of up to 512 tokens (approximately 380 words) requires 512MB of video memory.
You might say, this is no big deal - I have 24GB of video memory, what is 512MB? However, if you want to run larger batches, this number starts to add up.
For example, if you want to do 16 batches, the video memory will be directly increased to 8GB.
The above is the detailed content of Imitating Jeff Dean's divine summary, a former Google engineer shared 'LLM development secrets': numbers that every developer should know!. For more information, please follow other related articles on the PHP Chinese website!
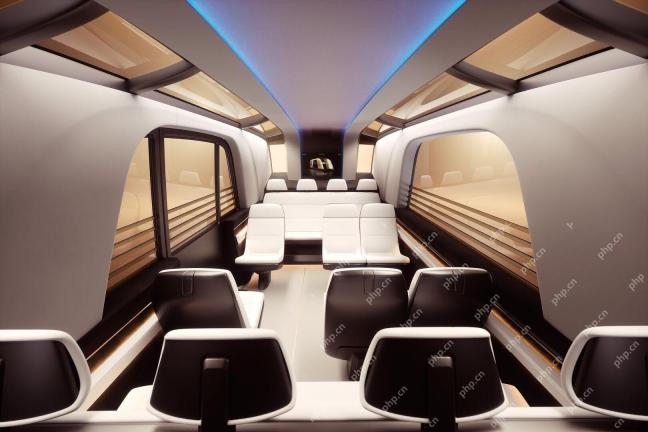
Since 2008, I've championed the shared-ride van—initially dubbed the "robotjitney," later the "vansit"—as the future of urban transportation. I foresee these vehicles as the 21st century's next-generation transit solution, surpas
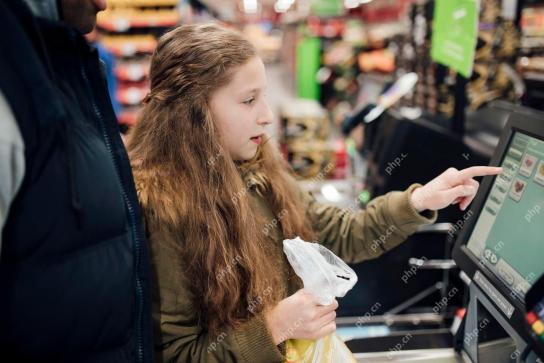
Revolutionizing the Checkout Experience Sam's Club's innovative "Just Go" system builds on its existing AI-powered "Scan & Go" technology, allowing members to scan purchases via the Sam's Club app during their shopping trip.
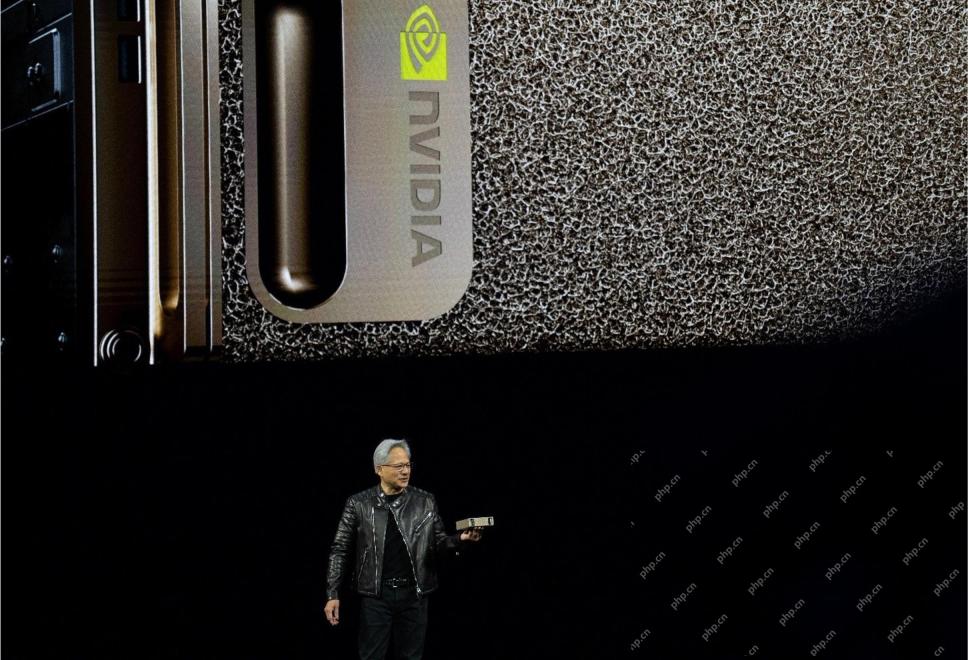
Nvidia's Enhanced Predictability and New Product Lineup at GTC 2025 Nvidia, a key player in AI infrastructure, is focusing on increased predictability for its clients. This involves consistent product delivery, meeting performance expectations, and
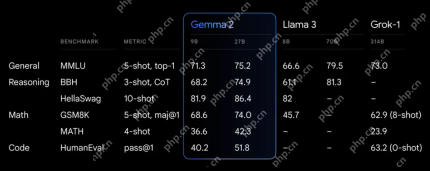
Google's Gemma 2: A Powerful, Efficient Language Model Google's Gemma family of language models, celebrated for efficiency and performance, has expanded with the arrival of Gemma 2. This latest release comprises two models: a 27-billion parameter ver
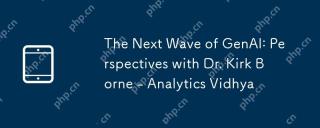
This Leading with Data episode features Dr. Kirk Borne, a leading data scientist, astrophysicist, and TEDx speaker. A renowned expert in big data, AI, and machine learning, Dr. Borne offers invaluable insights into the current state and future traje
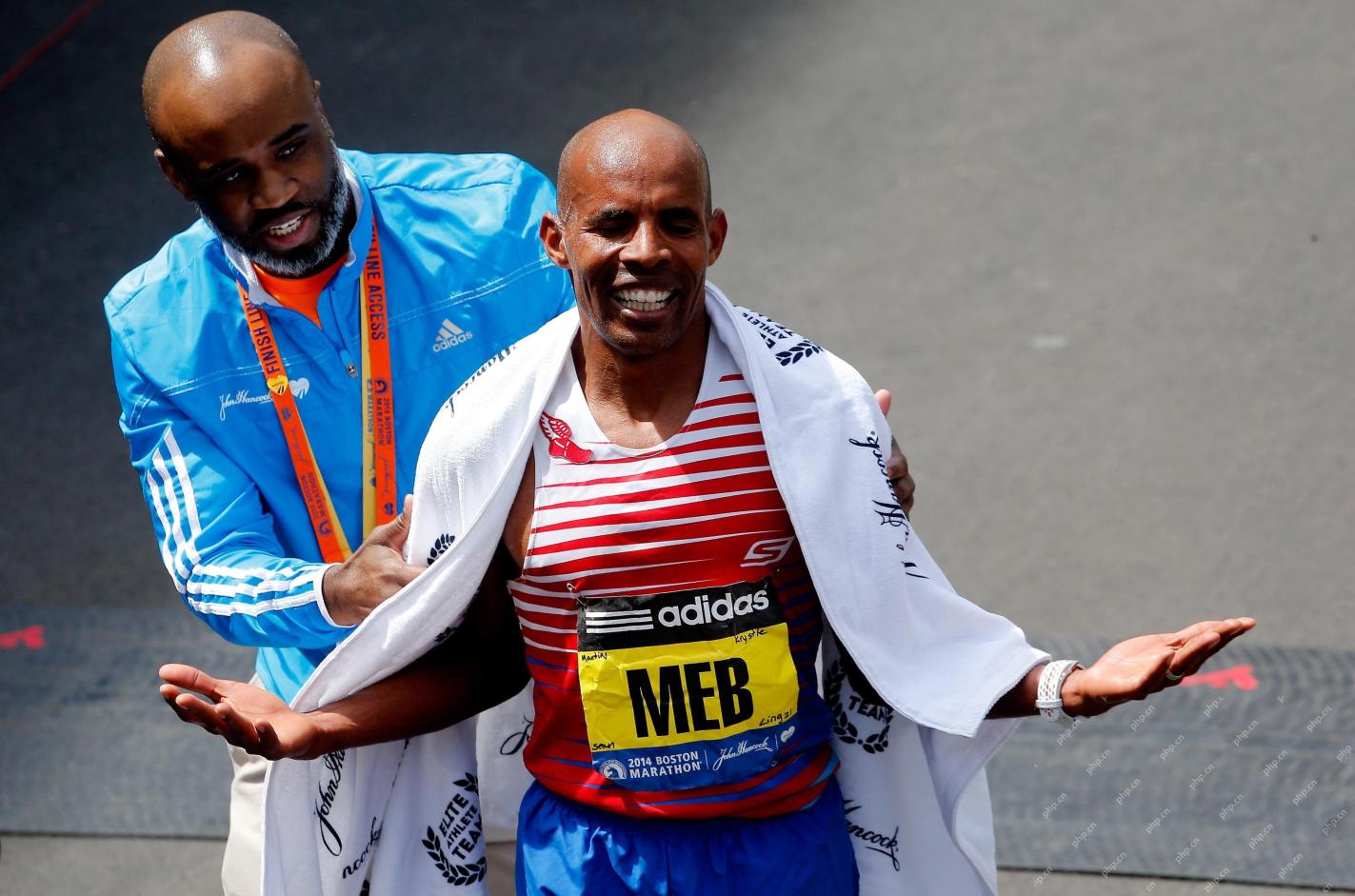
There were some very insightful perspectives in this speech—background information about engineering that showed us why artificial intelligence is so good at supporting people’s physical exercise. I will outline a core idea from each contributor’s perspective to demonstrate three design aspects that are an important part of our exploration of the application of artificial intelligence in sports. Edge devices and raw personal data This idea about artificial intelligence actually contains two components—one related to where we place large language models and the other is related to the differences between our human language and the language that our vital signs “express” when measured in real time. Alexander Amini knows a lot about running and tennis, but he still
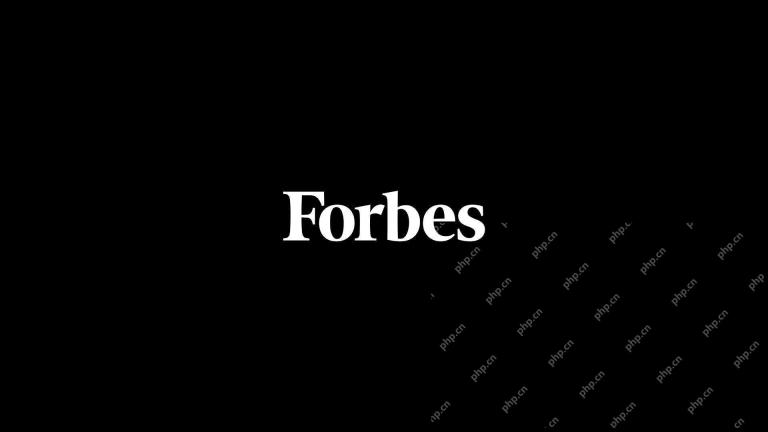
Caterpillar's Chief Information Officer and Senior Vice President of IT, Jamie Engstrom, leads a global team of over 2,200 IT professionals across 28 countries. With 26 years at Caterpillar, including four and a half years in her current role, Engst
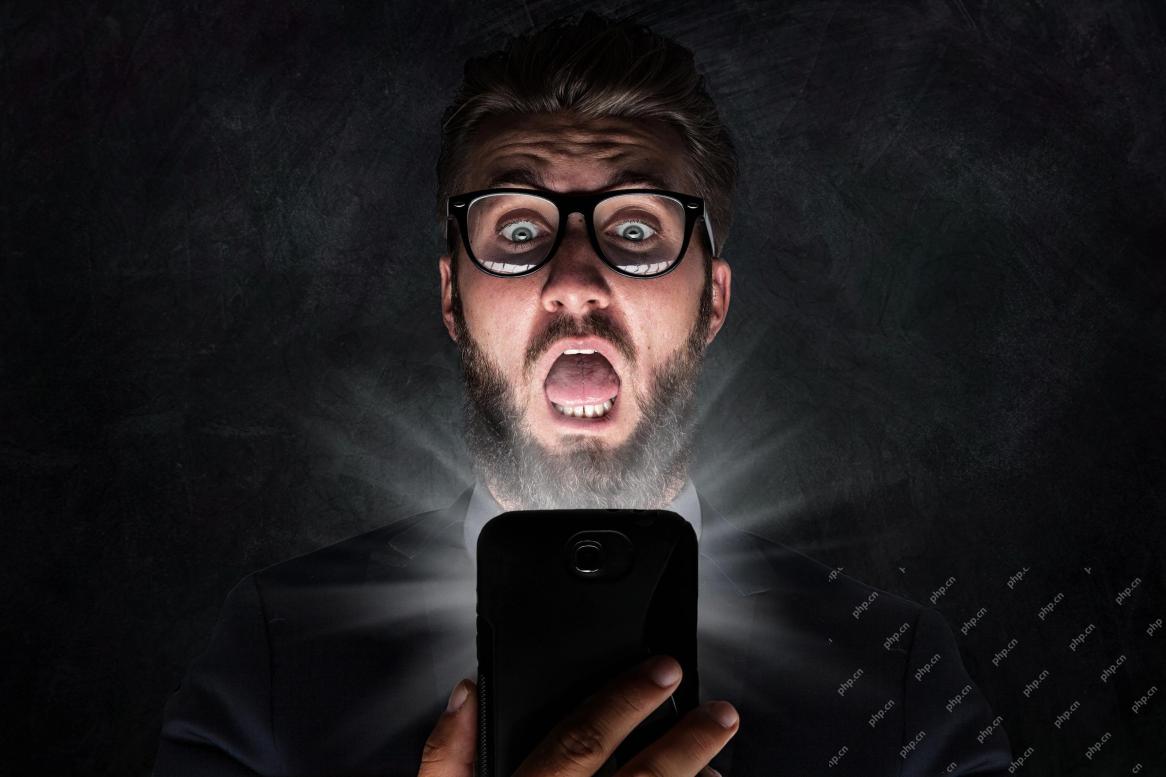
Google Photos' New Ultra HDR Tool: A Quick Guide Enhance your photos with Google Photos' new Ultra HDR tool, transforming standard images into vibrant, high-dynamic-range masterpieces. Ideal for social media, this tool boosts the impact of any photo,


Hot AI Tools

Undresser.AI Undress
AI-powered app for creating realistic nude photos

AI Clothes Remover
Online AI tool for removing clothes from photos.

Undress AI Tool
Undress images for free

Clothoff.io
AI clothes remover

Video Face Swap
Swap faces in any video effortlessly with our completely free AI face swap tool!

Hot Article

Hot Tools

Atom editor mac version download
The most popular open source editor

SublimeText3 Linux new version
SublimeText3 Linux latest version

mPDF
mPDF is a PHP library that can generate PDF files from UTF-8 encoded HTML. The original author, Ian Back, wrote mPDF to output PDF files "on the fly" from his website and handle different languages. It is slower than original scripts like HTML2FPDF and produces larger files when using Unicode fonts, but supports CSS styles etc. and has a lot of enhancements. Supports almost all languages, including RTL (Arabic and Hebrew) and CJK (Chinese, Japanese and Korean). Supports nested block-level elements (such as P, DIV),

Zend Studio 13.0.1
Powerful PHP integrated development environment

SecLists
SecLists is the ultimate security tester's companion. It is a collection of various types of lists that are frequently used during security assessments, all in one place. SecLists helps make security testing more efficient and productive by conveniently providing all the lists a security tester might need. List types include usernames, passwords, URLs, fuzzing payloads, sensitive data patterns, web shells, and more. The tester can simply pull this repository onto a new test machine and he will have access to every type of list he needs.