


After the release of ChatGPT, the ecosystem in the field of natural language processing has completely changed. Many problems that could not be solved before can be solved using ChatGPT.
However, it also brings a problem: the performance of large models is too strong, and it is difficult to evaluate the differences of each model with the naked eye.
For example, if several versions of the model are trained with different base models and hyperparameters, the performance may be similar from the examples, and the performance gap between the two models cannot be fully quantified.
Currentlythere are two main options for evaluating large language models:
1. Call OpenAI’s API interface for evaluation.ChatGPT can be used to evaluate the quality of the output of two models, but ChatGPT has been iteratively upgraded. The responses to the same question at different times may be different, and the evaluation results exist
Cannot reproduce problem.
2. Manual annotationIf you ask for manual annotation on the crowdsourcing platform,
the team with insufficient funds may Unable to afford it, there are also cases where third-party companiesleak data.
In order to solve such "large model evaluation problems", researchers from Peking University, Westlake University, North Carolina State University, Carnegie Mellon University, and MSRA collaborated to develop a PandaLM, a new language model evaluation framework, is committed to realizing a privacy-preserving, reliable, reproducible and cheap large model evaluation solution.
Provided with the same context, PandaLM can compare the response output of different LLMs and provide specific reasons.
To demonstrate the tool’s reliability and consistency, the researchers created a diverse human-annotated test dataset consisting of approximately 1,000 samples, in which PandaLM-7B’s accurate The rate reached 94% of
ChatGPT’s evaluation capability. Three lines of code using PandaLM
When two different large models produce different responses to the same instructions and context, PandaLM is designed to compare the two large models. The response quality of the model, and output comparison results, comparison reasons, and responses for reference.
There are three comparison results: response 1 is better, response 2 is better, and response 1 and response 2 have similar quality.
When comparing the performance of multiple large models, you only need to use PandaLM to compare them in pairs, and then summarize the results of the pairwise comparisons to rank or draw the performance of multiple large models. The model partial order relationship diagram can clearly and intuitively analyze the performance differences between different models.
PandaLM only needs to be "locally deployed" and "does not require human participation", so PandaLM's evaluation can protect privacy and is quite cheap.
In order to provide better interpretability, PandaLM can also explain its selections in natural language and generate an additional set of reference responses.
Considering that many existing models and frameworks are not open source or difficult to complete inference locally, PandaLM supports using specified model weights to generate text to be evaluated, or directly passing in a .json file containing the text to be evaluated.
Users can use PandaLM to evaluate user-defined models and input data by simply passing in a list containing the model name/HuggingFace model ID or .json file path. The following is a minimalist usage example:
In order to allow everyone to use PandaLM flexibly for free evaluation, researchers The model weights of PandaLM have also been published on the huggingface website. You can load the PandaLM-7B model through the following command:
Features of PandaLM
Reproducibility
Because the weights of PandaLM are public, even the output of the language model There is randomness. When the random seed is fixed, the evaluation results of PandaLM can always remain consistent.
The update of the model based on the online API is not transparent, its output may be very inconsistent at different times, and the old version of the model is no longer accessible, so the evaluation based on the online API is often not accessible. Reproducibility.
Automation, privacy protection and low overhead
Just deploy the PandaLM model locally and call the ready-made commands You can start to evaluate various large models without having to keep in constant communication with experts like hiring experts for annotation. There will also be no data leakage issues. At the same time, it does not involve any API fees or labor costs, making it very cheap.
Evaluation Level
To prove the reliability of PandaLM, the researchers hired three experts to conduct independent repeated annotations , a manually annotated test set was created.
The test set contains 50 different scenarios, and each scenario contains several tasks. This test set is diverse, reliable, and consistent with human preferences for text. Each sample of the test set consists of an instruction and context, and two responses generated by different large models, and the quality of the two responses is compared by humans.
Screen out samples with large differences between annotators to ensure that each annotator's IAA (Inter Annotator Agreement) on the final test set is close to 0.85. It is worth noting that the training set of PandaLM does not have any overlap with the manually annotated test set created.
These filtered samples require additional knowledge or difficult-to-obtain information to assist judgment, which makes it difficult for humans to Label them accurately.
The filtered test set contains 1000 samples, while the original unfiltered test set contains 2500 samples. The distribution of the test set is {0:105, 1:422, 2:472}, where 0 indicates that the two responses are of similar quality, 1 indicates that response 1 is better, and 2 indicates that response 2 is better. Taking the human test set as the benchmark, the performance comparison of PandaLM and gpt-3.5-turbo is as follows:
It can be seen that PandaLM-7B is in accuracy It has reached the level of 94% of gpt-3.5-turbo, and in terms of precision, recall, and F1 score, PandaLM-7B is almost the same as gpt-3.5-turbo.
Therefore, compared with gpt-3.5-turbo, it can be considered that PandaLM-7B already has considerable large model evaluation capabilities.
In addition to the accuracy, precision, recall, and F1 score on the test set, the results of comparisons between 5 large open source models of similar size are also provided.
First used the same training data to fine-tune the five models, and then used humans, gpt-3.5-turbo, and PandaLM to compare the five models separately.
The first tuple (72, 28, 11) in the first row of the table below indicates that there are 72 LLaMA-7B responses that are better than Bloom-7B, and there are 28 LLaMA The response of -7B is worse than that of Bloom-7B, with 11 responses of similar quality between the two models.
So in this example, humans think LLaMA-7B is better than Bloom-7B. The results in the following three tables show that humans, gpt-3.5-turbo and PandaLM-7B have completely consistent judgments on the relationship between the pros and cons of each model.
Summary
PandaLM provides a third article in addition to human evaluation and OpenAI API evaluation For solutions to evaluate large models, PandaLM not only has a high evaluation level, but also has reproducible evaluation results, automated evaluation processes, privacy protection and low overhead.
In the future, PandaLM will promote research on large models in academia and industry, so that more people can benefit from the development of large models.
The above is the detailed content of PandaLM, an open-source 'referee large model' from Peking University, West Lake University and others: three lines of code to fully automatically evaluate LLM, with an accuracy of 94% of ChatGPT. For more information, please follow other related articles on the PHP Chinese website!
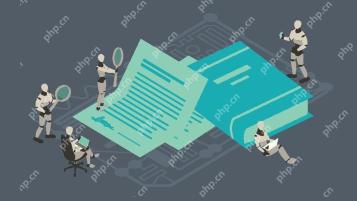
The legal tech revolution is gaining momentum, pushing legal professionals to actively embrace AI solutions. Passive resistance is no longer a viable option for those aiming to stay competitive. Why is Technology Adoption Crucial? Legal professional
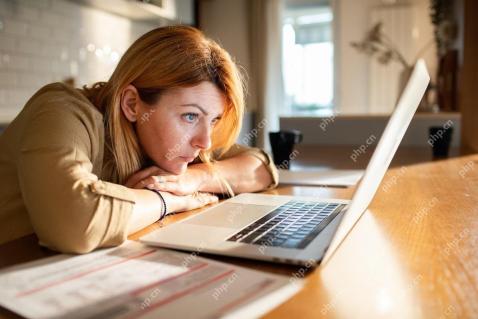
Many assume interactions with AI are anonymous, a stark contrast to human communication. However, AI actively profiles users during every chat. Every prompt, every word, is analyzed and categorized. Let's explore this critical aspect of the AI revo
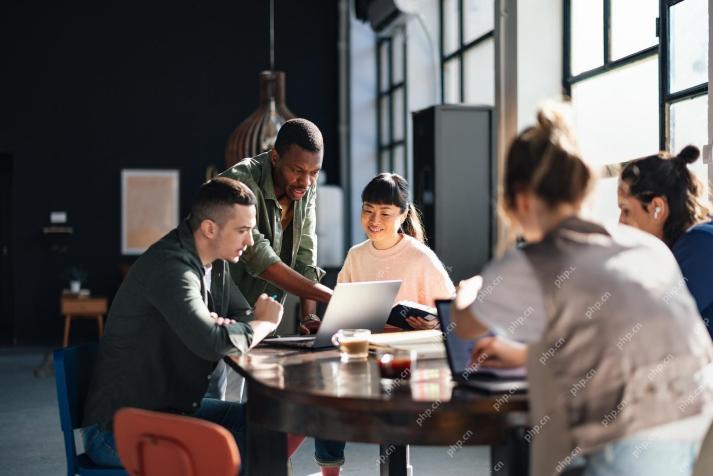
A successful artificial intelligence strategy cannot be separated from strong corporate culture support. As Peter Drucker said, business operations depend on people, and so does the success of artificial intelligence. For organizations that actively embrace artificial intelligence, building a corporate culture that adapts to AI is crucial, and it even determines the success or failure of AI strategies. West Monroe recently released a practical guide to building a thriving AI-friendly corporate culture, and here are some key points: 1. Clarify the success model of AI: First of all, we must have a clear vision of how AI can empower business. An ideal AI operation culture can achieve a natural integration of work processes between humans and AI systems. AI is good at certain tasks, while humans are good at creativity and judgment
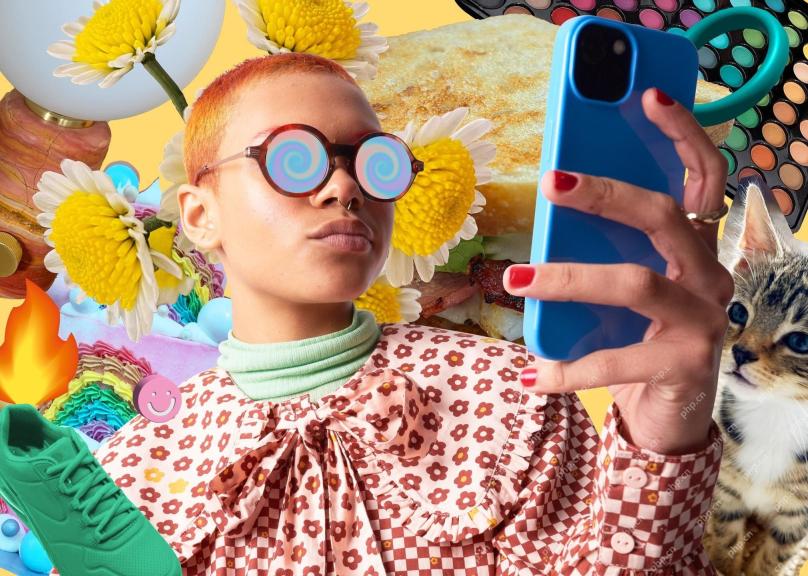
Meta upgrades AI assistant application, and the era of wearable AI is coming! The app, designed to compete with ChatGPT, offers standard AI features such as text, voice interaction, image generation and web search, but has now added geolocation capabilities for the first time. This means that Meta AI knows where you are and what you are viewing when answering your question. It uses your interests, location, profile and activity information to provide the latest situational information that was not possible before. The app also supports real-time translation, which completely changed the AI experience on Ray-Ban glasses and greatly improved its usefulness. The imposition of tariffs on foreign films is a naked exercise of power over the media and culture. If implemented, this will accelerate toward AI and virtual production
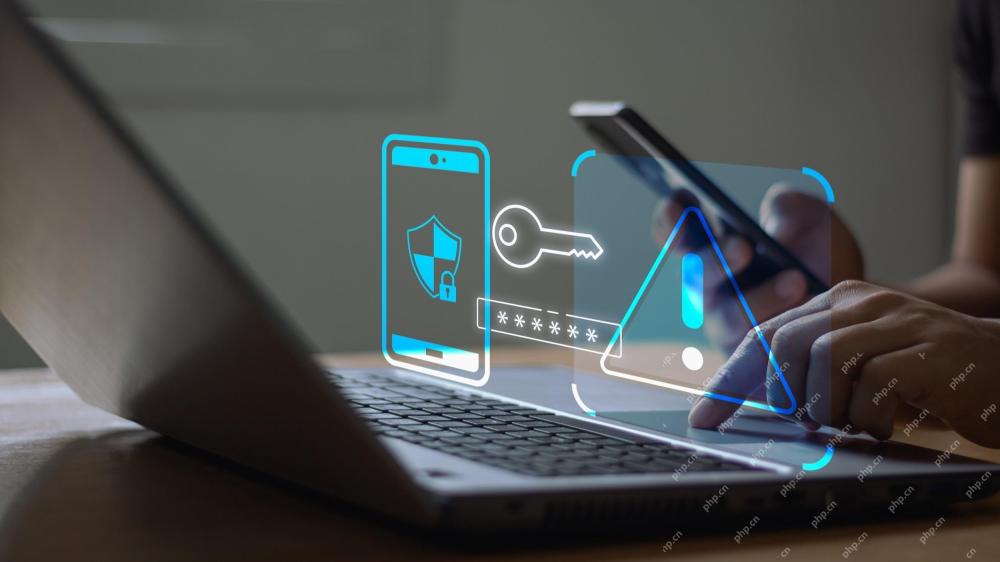
Artificial intelligence is revolutionizing the field of cybercrime, which forces us to learn new defensive skills. Cyber criminals are increasingly using powerful artificial intelligence technologies such as deep forgery and intelligent cyberattacks to fraud and destruction at an unprecedented scale. It is reported that 87% of global businesses have been targeted for AI cybercrime over the past year. So, how can we avoid becoming victims of this wave of smart crimes? Let’s explore how to identify risks and take protective measures at the individual and organizational level. How cybercriminals use artificial intelligence As technology advances, criminals are constantly looking for new ways to attack individuals, businesses and governments. The widespread use of artificial intelligence may be the latest aspect, but its potential harm is unprecedented. In particular, artificial intelligence
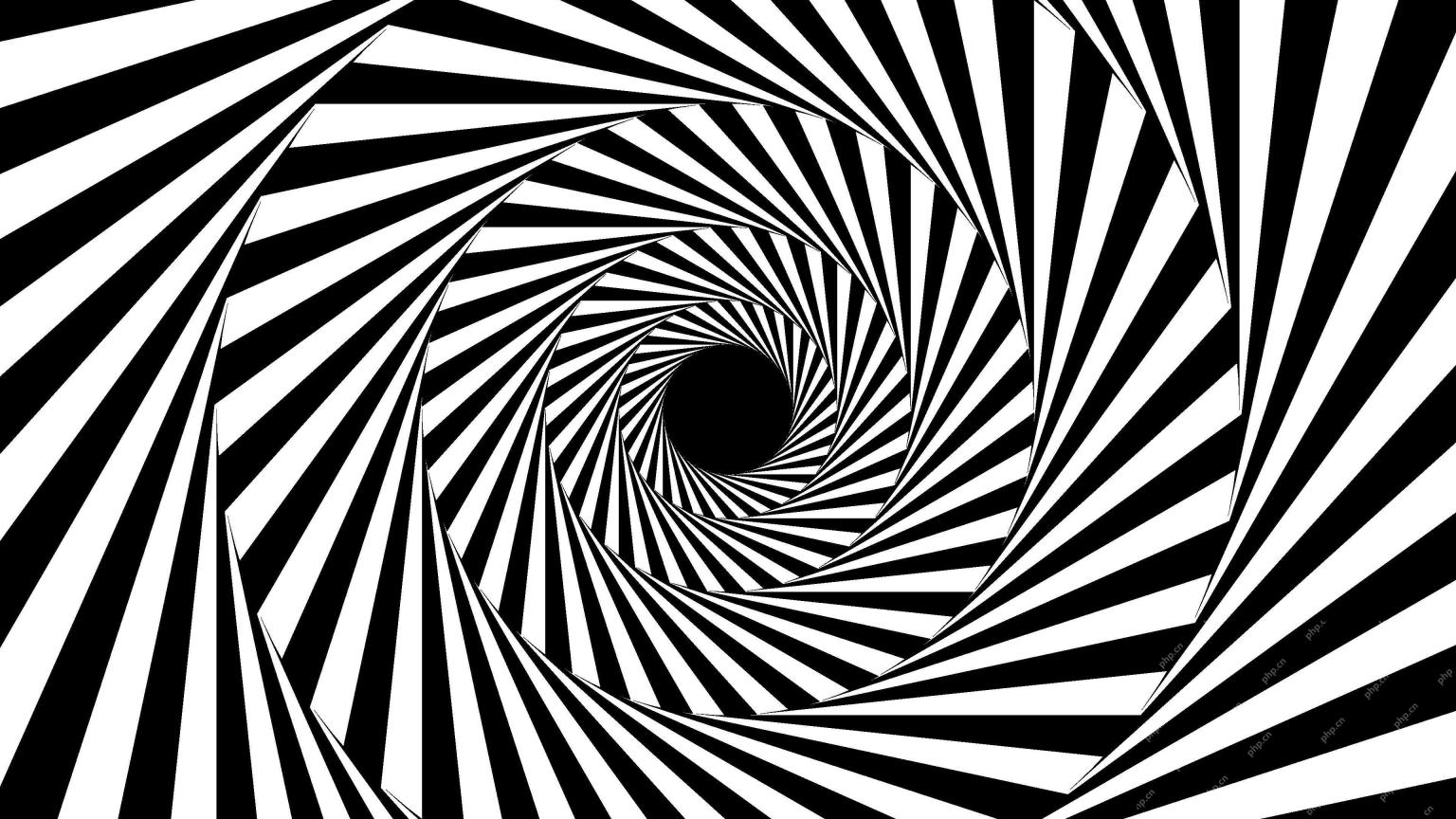
The intricate relationship between artificial intelligence (AI) and human intelligence (NI) is best understood as a feedback loop. Humans create AI, training it on data generated by human activity to enhance or replicate human capabilities. This AI
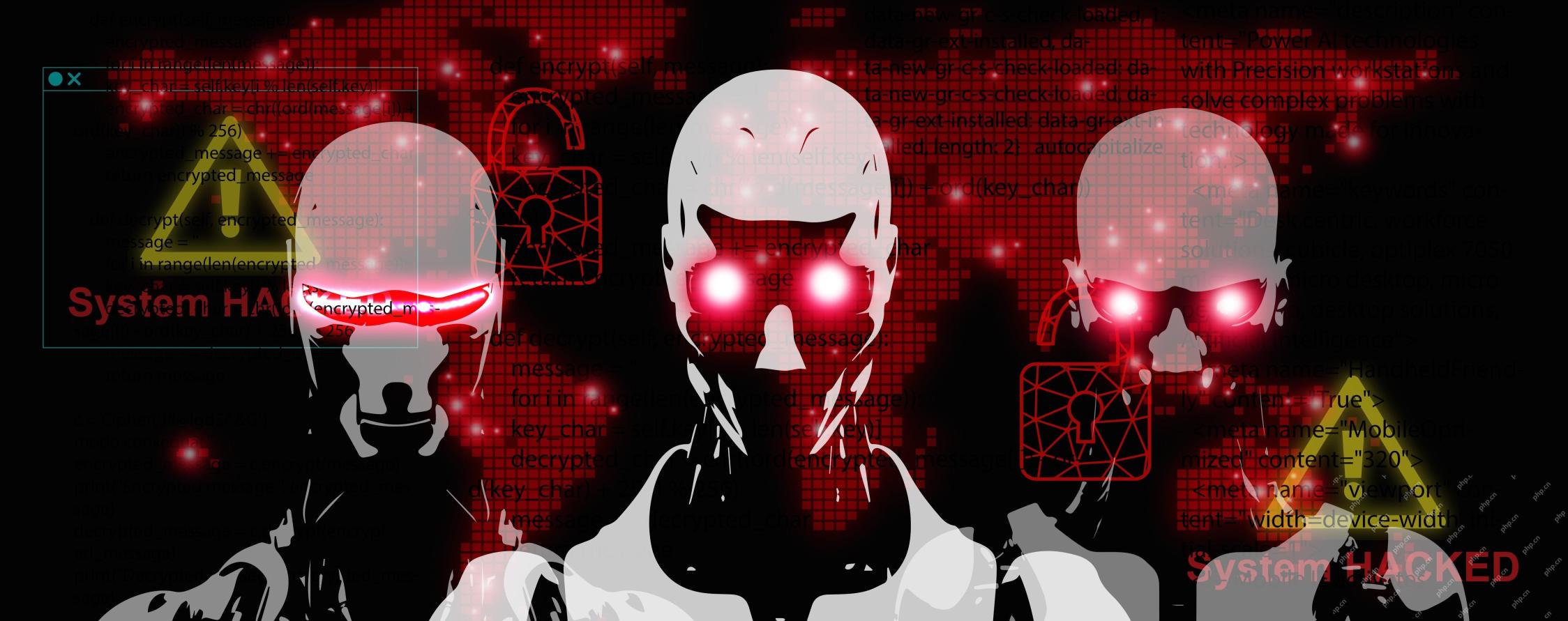
Anthropic's recent statement, highlighting the lack of understanding surrounding cutting-edge AI models, has sparked a heated debate among experts. Is this opacity a genuine technological crisis, or simply a temporary hurdle on the path to more soph
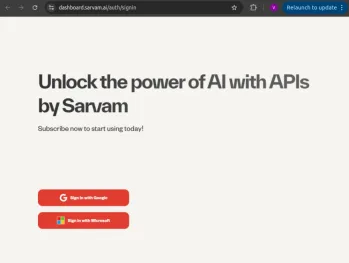
India is a diverse country with a rich tapestry of languages, making seamless communication across regions a persistent challenge. However, Sarvam’s Bulbul-V2 is helping to bridge this gap with its advanced text-to-speech (TTS) t


Hot AI Tools

Undresser.AI Undress
AI-powered app for creating realistic nude photos

AI Clothes Remover
Online AI tool for removing clothes from photos.

Undress AI Tool
Undress images for free

Clothoff.io
AI clothes remover

Video Face Swap
Swap faces in any video effortlessly with our completely free AI face swap tool!

Hot Article

Hot Tools

Safe Exam Browser
Safe Exam Browser is a secure browser environment for taking online exams securely. This software turns any computer into a secure workstation. It controls access to any utility and prevents students from using unauthorized resources.

SublimeText3 Linux new version
SublimeText3 Linux latest version

DVWA
Damn Vulnerable Web App (DVWA) is a PHP/MySQL web application that is very vulnerable. Its main goals are to be an aid for security professionals to test their skills and tools in a legal environment, to help web developers better understand the process of securing web applications, and to help teachers/students teach/learn in a classroom environment Web application security. The goal of DVWA is to practice some of the most common web vulnerabilities through a simple and straightforward interface, with varying degrees of difficulty. Please note that this software

SublimeText3 English version
Recommended: Win version, supports code prompts!
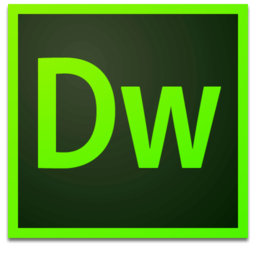
Dreamweaver Mac version
Visual web development tools
