


Distillation can also be Step-by-Step: the new method allows small models to be comparable to large models 2000 times the size
Although large-scale language models have amazing capabilities, due to their large scale, the costs required for their deployment are often huge. The University of Washington, together with the Google Cloud Computing Artificial Intelligence Research Institute and Google Research, further solved this problem and proposed the Distilling Step-by-Step paradigm to help model training. Compared with LLM, this method is more effective in training small models and applying them to specific tasks, and requires less training data than traditional fine-tuning and distillation. On a benchmark task, their 770M T5 model outperformed the 540B PaLM model. Impressively, their model only used 80% of the available data.
While large language models (LLMs) have demonstrated impressive Few-shot learning capability, but it is difficult to deploy such a large-scale model in real applications. Dedicated infrastructure serving a 175 billion parameter scale LLM requires at least 350GB of GPU memory. What's more, today's state-of-the-art LLM is composed of more than 500 billion parameters, which means it requires more memory and computing resources. Such computing requirements are out of reach for most manufacturers, let alone applications that require low latency.
In order to solve this problem of large models, deployers often use smaller specific models instead. These smaller models are trained using common paradigms - fine-tuning or distillation. Fine-tuning upgrades a small pre-trained model using downstream human annotated data. Distillation trains an equally smaller model using the labels produced by the larger LLM. Unfortunately, these paradigms come at a cost while reducing model size: to achieve comparable performance to LLM, fine-tuning requires expensive human labels, while distillation requires large amounts of unlabeled data that is difficult to obtain.
In a paper titled "Distilling Step-by-Step! Outperforming Larger Language Models with Less Training Data and Smaller Model Sizes", researchers from the University of Washington and Google A new simple mechanism, Distilling step-bystep, is introduced for training smaller models using less training data. This mechanism reduces the amount of training data required to fine-tune and distill the LLM, resulting in a smaller model size.
Paper link: https://arxiv.org/pdf/2305.02301 v1.pdf
#The core of this mechanism is to change the perspective and regard LLM as an agent that can reason, rather than as a source of noise labels. LLM can generate natural language rationales that can be used to explain and support the labels predicted by the model. For example, when asked "A gentleman carries golf equipment, what might he have? (a) clubs, (b) auditorium, (c) meditation center, (d) conference, (e) church" , LLM can answer "(a) club" through chain of thought (CoT) reasoning, and rationalize this label by explaining that "the answer must be something used to play golf." Of the above choices, only clubs are used for golf. We use these justifications as additional, richer information to train smaller models in a multi-task training setting and perform label prediction and justification prediction.
As shown in Figure 1, stepwise distillation can learn task-specific small models with less than 1/500 the number of parameters of LLM. Stepwise distillation also uses far fewer training examples than traditional fine-tuning or distillation.
Experimental results show that among the 4 NLP benchmarks, there are three promising experiments in conclusion.
- First, compared to fine-tuning and distillation, the stepwise distillation model achieves better performance on each data set, reducing the number of training instances by more than 50% on average (up to more than 85%) .
- Second, our model outperforms LLM when the model size is smaller (up to 2000 times smaller), greatly reducing the computational cost required for model deployment .
- Third, this research reduces the size of the model while also reducing the amount of data required to go beyond LLM. The researchers surpassed the performance of LLM with 540B parameters using a 770M T5 model. This smaller model uses only 80% of the labeled data set of existing fine-tuning methods.
When there is only unlabeled data, the performance of the small model is still better than that of LLM - only using a 11B T5 model exceeds The performance of PaLM of 540B has been improved.
The study further shows that when a smaller model performs worse than LLM, stepwise distillation can more effectively utilize additional unlabeled data than standard distillation methods. Make smaller models comparable to the performance of LLM.
Stepwise Distillation
The researchers proposed a new paradigm of stepwise distillation, which uses the reasoning ability of LLM to predict its predictions to train smaller models in a data-efficient manner. Model. The overall framework is shown in Figure 2.
The paradigm has two simple steps: first, given an LLM and an An unlabeled data set prompts LLM to generate an output label and a justification for the label. The rationale is explained in natural language and provides support for the label predicted by the model (see Figure 2). Justification is an emergent behavioral property of current self-supervised LLMs.
Then, in addition to task labels, use these reasons to train smaller downstream models. To put it bluntly, reasons can provide richer and more detailed information to explain why an input is mapped to a specific output label.
Experimental results
The researchers verified the effectiveness of stepwise distillation in the experiment. First, compared to standard fine-tuning and task distillation methods, stepwise distillation helps achieve better performance with a much smaller number of training examples, significantly improving the data efficiency of learning small task-specific models.
#Secondly, Studies show that the stepwise distillation method surpasses the performance of LLM with smaller model sizes, significantly reducing deployment costs compared to llm.
The above is the detailed content of Distillation can also be Step-by-Step: the new method allows small models to be comparable to large models 2000 times the size. For more information, please follow other related articles on the PHP Chinese website!
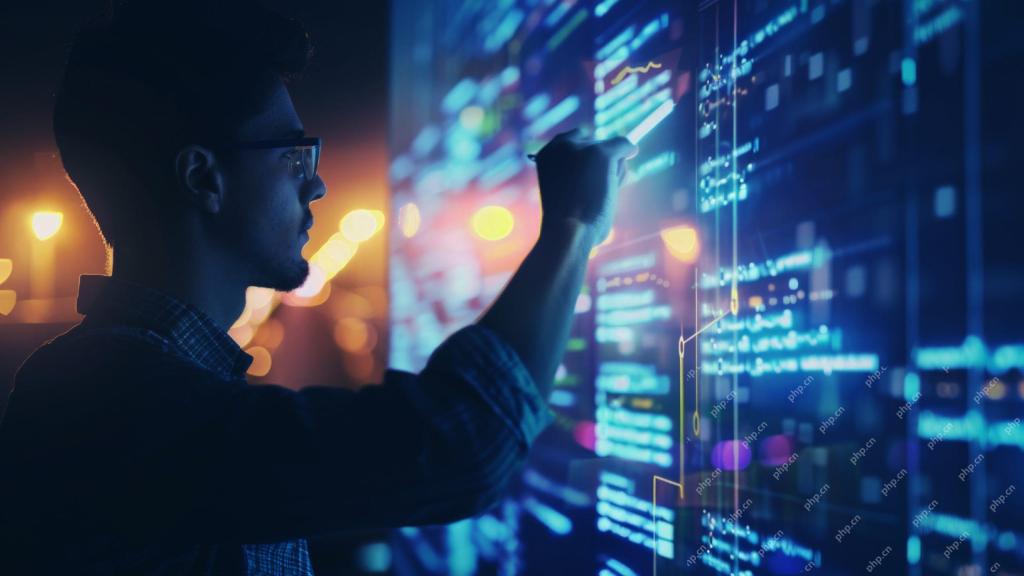
SQL alias: A tool to improve the readability of SQL queries Do you think there is still room for improvement in the readability of your SQL queries? Then try the SQL alias! Alias This convenient tool allows you to give temporary nicknames to tables and columns, making your queries clearer and easier to process. This article discusses all use cases for aliases clauses, such as renaming columns and tables, and combining multiple columns or subqueries. Overview SQL alias provides temporary nicknames for tables and columns to enhance the readability and manageability of queries. SQL aliases created with AS keywords simplify complex queries by allowing more intuitive table and column references. Examples include renaming columns in the result set, simplifying table names in the join, and combining multiple columns into one

Google's Gemini: Code Execution Capabilities of Large Language Models Large Language Models (LLMs), successors to Transformers, have revolutionized Natural Language Processing (NLP) and Natural Language Understanding (NLU). Initially replacing rule-

Unlocking AI's Potential: A Deep Dive into the Tree of Thoughts Technique Imagine navigating a dense forest, each path promising a different outcome, your goal: discovering hidden treasure. This analogy perfectly captures the essence of the Tree of
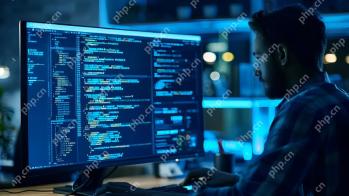
Introduction Imagine transforming a cluttered garage into a well-organized, brightly lit space where everything is easily accessible and neatly arranged. In the world of databases, this process is called normalization. Just as a tidy garage improve
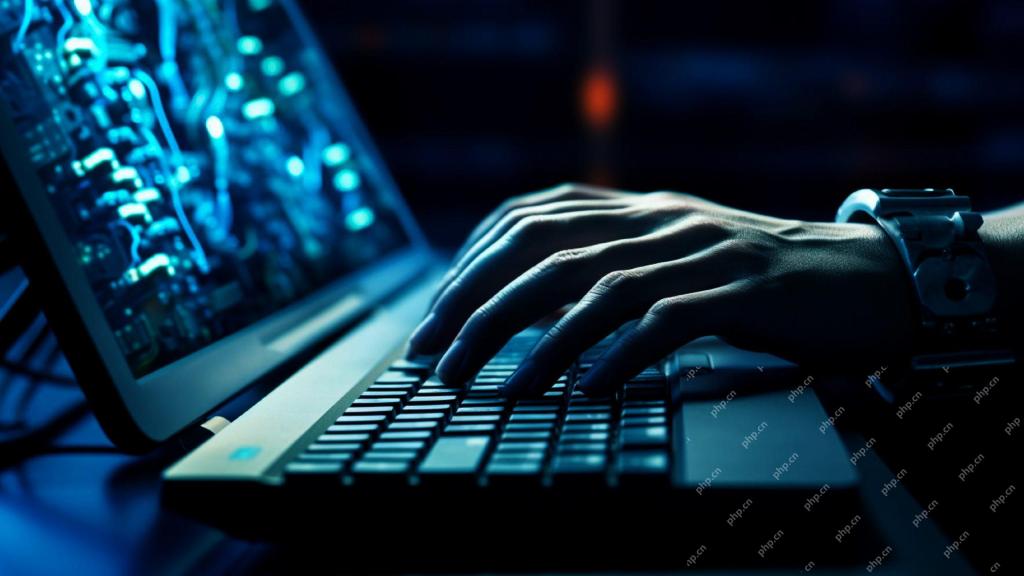
Prompt Engineering: Mastering Delimiters for Superior AI Results Imagine crafting a gourmet meal: each ingredient measured precisely, each step timed perfectly. Prompt engineering for AI is similar; delimiters are your essential tools. Just as pre
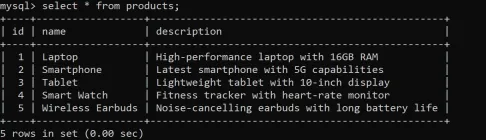
SQL REPLACE Functions: Efficient Data Cleaning and Text Operation Guide Have you ever needed to quickly fix large amounts of text in your database? SQL REPLACE functions can help a lot! It allows you to replace all instances of a specific substring with a new substring, making it easy to clean up data. Imagine that your data is scattered with typos—REPLACE can solve this problem immediately. Read on and I'll show you the syntax and some cool examples to get you started. Overview The SQL REPLACE function can efficiently clean up data by replacing specific substrings in text with other substrings. Use REPLACE(string, old
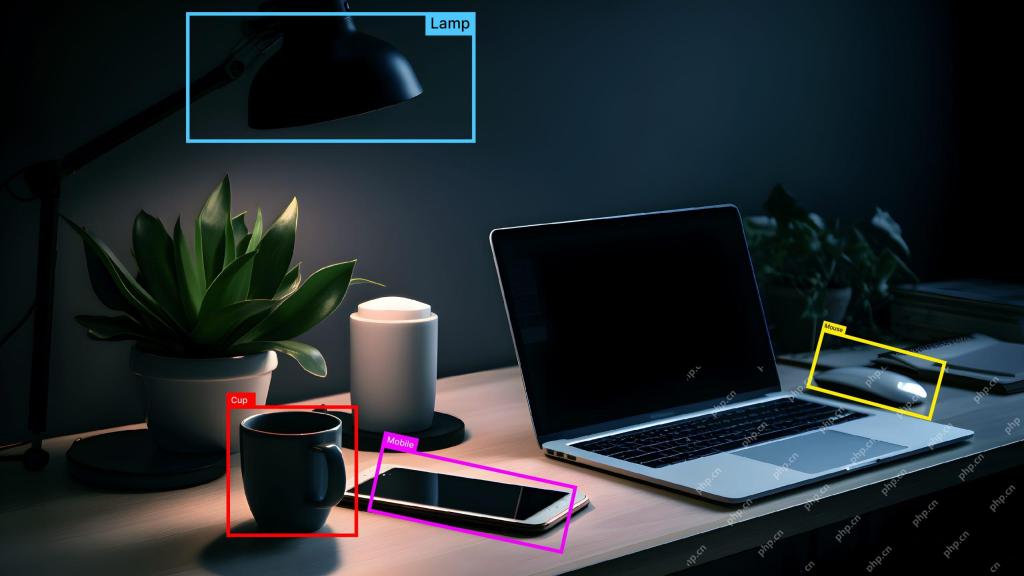
Object Detection: From R-CNN to YOLO – A Journey Through Computer Vision Imagine a computer not just seeing, but understanding images. This is the essence of object detection, a pivotal area in computer vision revolutionizing machine-world interactio
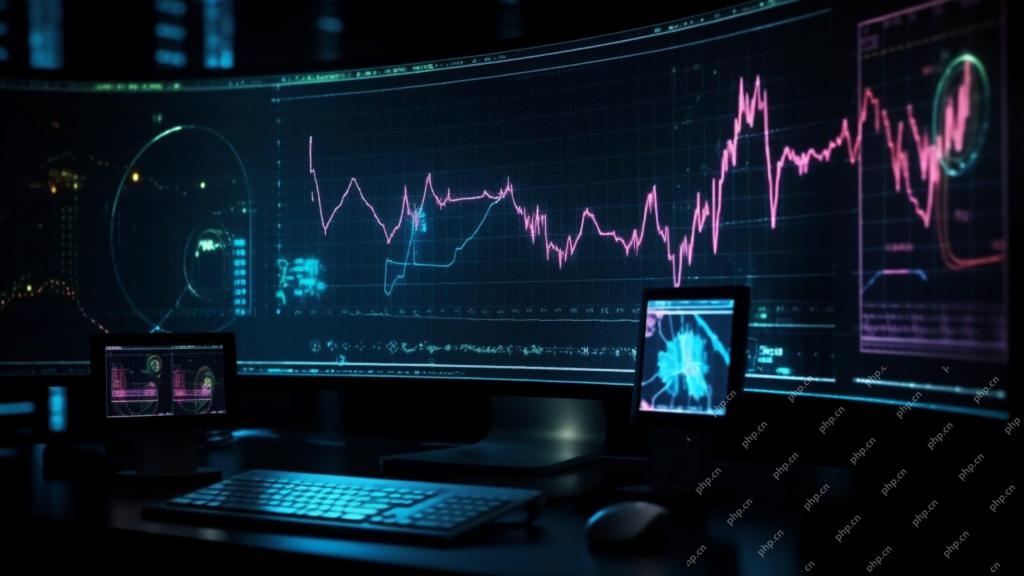
Kullback-Leibler (KL) Divergence: A Deep Dive into Relative Entropy Few mathematical concepts have as profoundly impacted modern machine learning and artificial intelligence as Kullback-Leibler (KL) divergence. This powerful metric, also known as re


Hot AI Tools

Undresser.AI Undress
AI-powered app for creating realistic nude photos

AI Clothes Remover
Online AI tool for removing clothes from photos.

Undress AI Tool
Undress images for free

Clothoff.io
AI clothes remover

Video Face Swap
Swap faces in any video effortlessly with our completely free AI face swap tool!

Hot Article

Hot Tools

MantisBT
Mantis is an easy-to-deploy web-based defect tracking tool designed to aid in product defect tracking. It requires PHP, MySQL and a web server. Check out our demo and hosting services.

SAP NetWeaver Server Adapter for Eclipse
Integrate Eclipse with SAP NetWeaver application server.

MinGW - Minimalist GNU for Windows
This project is in the process of being migrated to osdn.net/projects/mingw, you can continue to follow us there. MinGW: A native Windows port of the GNU Compiler Collection (GCC), freely distributable import libraries and header files for building native Windows applications; includes extensions to the MSVC runtime to support C99 functionality. All MinGW software can run on 64-bit Windows platforms.

PhpStorm Mac version
The latest (2018.2.1) professional PHP integrated development tool
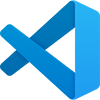
VSCode Windows 64-bit Download
A free and powerful IDE editor launched by Microsoft