By now, no one should dispute that most artificial intelligence is built on and currently uses biases that are problematic in some way. This is a challenge that has been observed and proven hundreds of times. The challenge for organizations is to root out AI bias, rather than just settling for better, unbiased data.
In a major revision to its publication, Towards Standards for Identifying and Managing Bias in Artificial Intelligence (NIST 1270 Special Publication), last year’s public Following the comment period, the National Institute of Standards and Technology (NIST) made a strong argument for looking beyond data and even ML processes to uncover and destroy AI bias.
Rather than blaming poorly collected or poorly labeled data, the authors say the next frontier of bias in AI is “human and systemic institutional and social factors” and push for a shift away from A socio-technical perspective looks for better answers.
“Context is everything,” said Reva Schwartz, NIST’s lead researcher on bias in artificial intelligence and one of the report’s authors. “AI systems do not operate in isolation. They help people make decisions that directly impact the lives of others. If we are to develop trustworthy AI systems, we need to consider all factors that could erode public trust in AI. Among these factors There are many that go beyond the technology itself and influence it, as highlighted by the comments we received from a variety of people and organizations."
Causing AI Bias What are human and systemic biases?
According to the NIST report, human beings are divided into two broad categories: individuals and groups, and there are many specific biases under each category.
Individual human biases include automation complacency, where people rely too much on automated skills; implicit bias, an unconscious belief, attitude, association, or stereotype that affects someone's decision-making; There is also confirmation bias, where people prefer information that is consistent or congruent with their existing beliefs.
Group Human foundations include groupthink, the phenomenon in which people make suboptimal decisions out of a desire to conform to the group or avoid disagreement; funding bias, when reporting is biased The results satisfy a funding agency or financial backer, which in turn may be subject to additional personal/group biases.
For systemic bias, the NIST report defines it as historical, social and institutional. Essentially, long-standing biases have been codified into society and institutions over time and are largely accepted as “facts” or “just the way things are.”
The reason these biases matter is because of how much impact AI deployment is having on the way organizations work today. Because of racially biased data, people are being denied mortgages, denying them the chance to own a home for the first time. Job seekers are being denied interviews because the AI is trained to make hiring decisions that historically favor men over women. Promising young students are denied interviews or admission to colleges because their last names don't match those of successful people from the past.
In other words: Biased AI creates as many locked doors as efficient openings. If organizations don’t actively work to eliminate bias in their deployments, they will quickly find themselves experiencing a severe lack of trust in how they think and operate.
What is the socio-technical perspective recommended by NIST?
At its core is the recognition that the results of any AI application are more than just mathematical and computational inputs. They are made by developers or data scientists, their positions and institutions vary, and they all have a certain level of burden.
NIST’s report reads: “A sociotechnical approach to AI considers the values and behaviors modeled from data sets, the humans who interact with them, and complex organizational factors. These factors are involved in their commissioning, design, development, and ultimate deployment."
NIST believes that through a sociotechnical lens, organizations can improve , privacy, reliability, robustness, security and security resiliency” to foster trust.
One of their recommendations was for organizations to implement or improve their test, evaluation, validation and verification (TEVV) processes. There should be ways to mathematically verify biases in a given data set or trained model. They also recommend creating more involvement from different fields and positions in AI development efforts, and having multiple stakeholders from different departments or outside the organization. In the “human-in-the-loop” model, individuals or groups continuously correct the basic ML output, which is also an effective tool for eliminating bias.
In addition to these and revised reports, there is NIST’s Artificial Intelligence Risk Management Framework (AI RMF), a consensus-driven set of recommendations for managing the risks involved in AI systems. Once completed, it will cover transparency, design and development, governance and testing of AI technologies and products. The initial comment period for the AI RMF has passed, but we still have many opportunities to learn about AI risks and mitigations.
The above is the detailed content of NIST: AI bias goes far beyond the data itself. For more information, please follow other related articles on the PHP Chinese website!
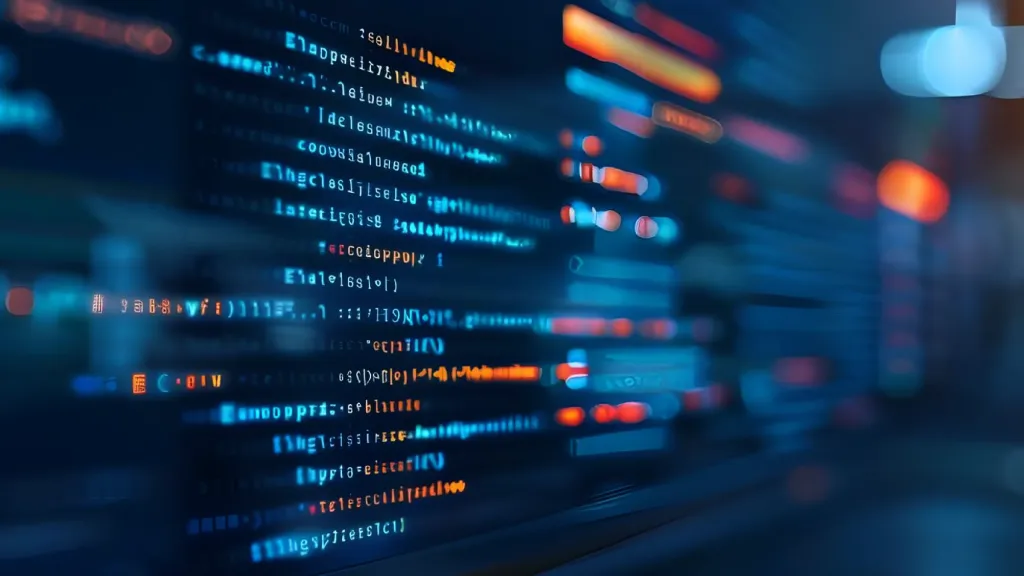
Running large language models at home with ease: LM Studio User Guide In recent years, advances in software and hardware have made it possible to run large language models (LLMs) on personal computers. LM Studio is an excellent tool to make this process easy and convenient. This article will dive into how to run LLM locally using LM Studio, covering key steps, potential challenges, and the benefits of having LLM locally. Whether you are a tech enthusiast or are curious about the latest AI technologies, this guide will provide valuable insights and practical tips. Let's get started! Overview Understand the basic requirements for running LLM locally. Set up LM Studi on your computer
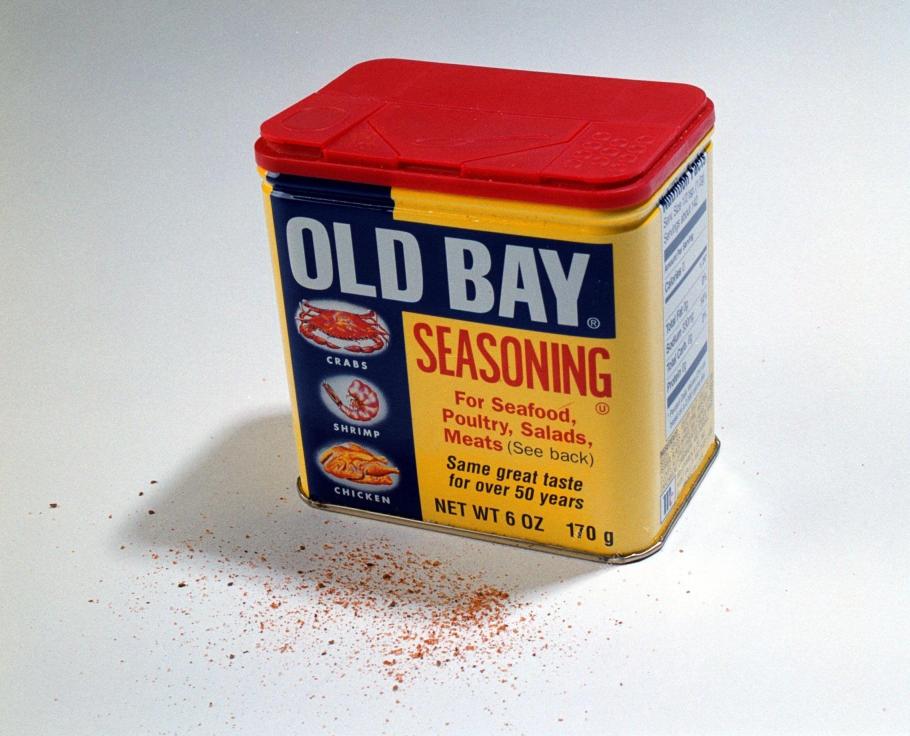
Guy Peri is McCormick’s Chief Information and Digital Officer. Though only seven months into his role, Peri is rapidly advancing a comprehensive transformation of the company’s digital capabilities. His career-long focus on data and analytics informs
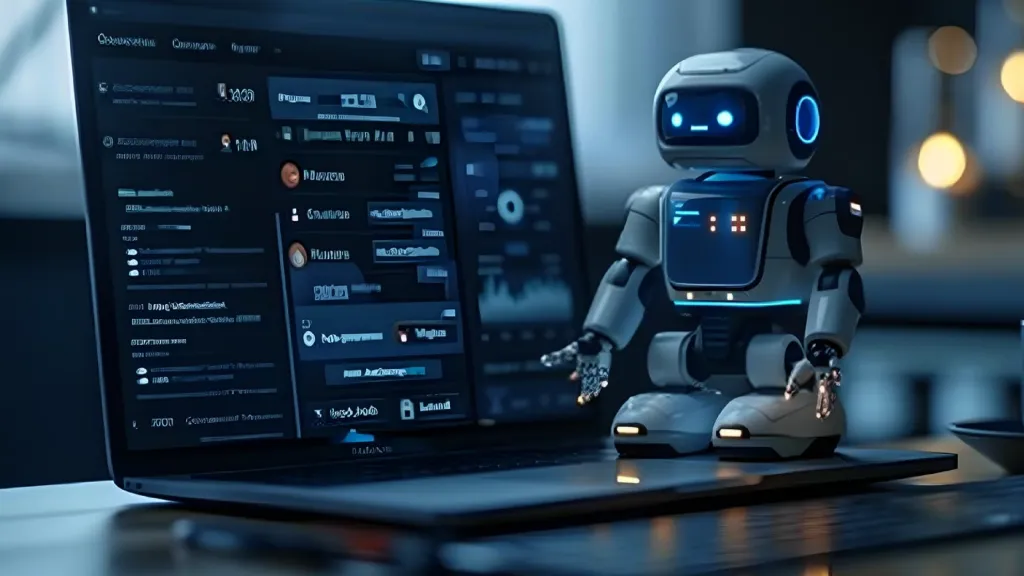
Introduction Artificial intelligence (AI) is evolving to understand not just words, but also emotions, responding with a human touch. This sophisticated interaction is crucial in the rapidly advancing field of AI and natural language processing. Th
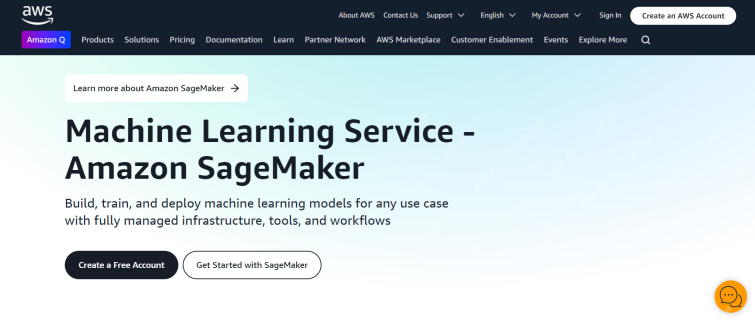
Introduction In today's data-centric world, leveraging advanced AI technologies is crucial for businesses seeking a competitive edge and enhanced efficiency. A range of powerful tools empowers data scientists, analysts, and developers to build, depl
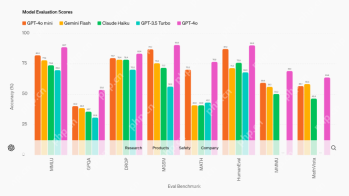
This week's AI landscape exploded with groundbreaking releases from industry giants like OpenAI, Mistral AI, NVIDIA, DeepSeek, and Hugging Face. These new models promise increased power, affordability, and accessibility, fueled by advancements in tr
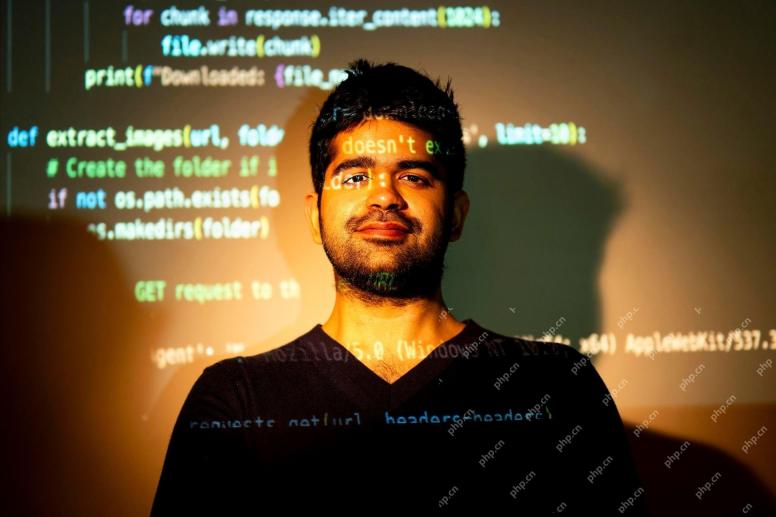
But the company’s Android app, which offers not only search capabilities but also acts as an AI assistant, is riddled with a host of security issues that could expose its users to data theft, account takeovers and impersonation attacks from malicious
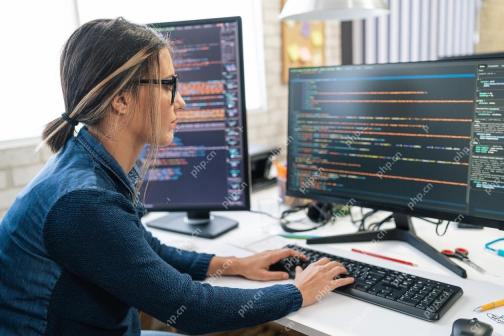
You can look at what’s happening in conferences and at trade shows. You can ask engineers what they’re doing, or consult with a CEO. Everywhere you look, things are changing at breakneck speed. Engineers, and Non-Engineers What’s the difference be
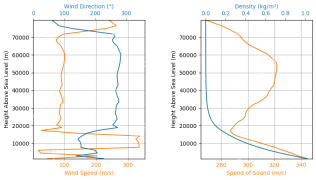
Simulate Rocket Launches with RocketPy: A Comprehensive Guide This article guides you through simulating high-power rocket launches using RocketPy, a powerful Python library. We'll cover everything from defining rocket components to analyzing simula


Hot AI Tools

Undresser.AI Undress
AI-powered app for creating realistic nude photos

AI Clothes Remover
Online AI tool for removing clothes from photos.

Undress AI Tool
Undress images for free

Clothoff.io
AI clothes remover

AI Hentai Generator
Generate AI Hentai for free.

Hot Article

Hot Tools

SecLists
SecLists is the ultimate security tester's companion. It is a collection of various types of lists that are frequently used during security assessments, all in one place. SecLists helps make security testing more efficient and productive by conveniently providing all the lists a security tester might need. List types include usernames, passwords, URLs, fuzzing payloads, sensitive data patterns, web shells, and more. The tester can simply pull this repository onto a new test machine and he will have access to every type of list he needs.
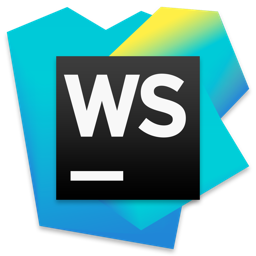
WebStorm Mac version
Useful JavaScript development tools
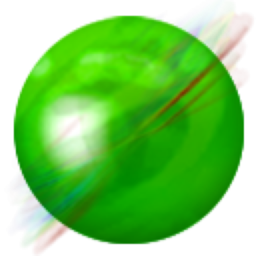
ZendStudio 13.5.1 Mac
Powerful PHP integrated development environment

Safe Exam Browser
Safe Exam Browser is a secure browser environment for taking online exams securely. This software turns any computer into a secure workstation. It controls access to any utility and prevents students from using unauthorized resources.

MinGW - Minimalist GNU for Windows
This project is in the process of being migrated to osdn.net/projects/mingw, you can continue to follow us there. MinGW: A native Windows port of the GNU Compiler Collection (GCC), freely distributable import libraries and header files for building native Windows applications; includes extensions to the MSVC runtime to support C99 functionality. All MinGW software can run on 64-bit Windows platforms.