Today’s on-the-job learning faces challenges. Sophisticated analytics, artificial intelligence, and robots are suddenly intruding into every aspect of the workplace, fundamentally upending this time-honored and effective way of learning. As technology increasingly automates jobs, tens of thousands of people leave or take jobs every year, and hundreds of millions of people must learn new skills and new ways of working. But there is broader evidence that companies deploying smart machines hinders this critical channel of learning: My colleagues and I have found that AI takes away learning opportunities from newcomers and reduces opportunities for veterans to practice, forcing both to master new methods at the same time. and old ways that overwhelm them.
So, can employees learn to work with these machines? Some of the previous observations were from learners who engaged in practices that challenged conventional practices, where these practices were not the focus and where tolerance for their consequences was high. I call this widespread and informal process “covert learning.”
Barriers to Learning
I discovered four common barriers to acquiring the skills you need that trigger covert learning.
1. Newbies are losing their “learning advantage”
In any job, training employees incurs costs and reduces quality because newbies are slow and prone to mistakes. As organizations embrace smart machines, they often have trainees reduce their involvement in risky and complex parts as a management strategy. As a result, trainees are denied the opportunity to stretch the boundaries of their abilities and grow from their mistakes with limited help—precisely the necessary conditions for learning new skills.
The same phenomenon occurs in investment banks. Callen Anthony of New York University discovered in an investment bank that partners used algorithms to assist companies in mergers and acquisitions and interpret valuations, causing junior analysts to become further and further apart from senior partners. The junior analyst's job is simply to extract raw reports from the system (a web-based collection of financial data on companies of interest) and pass them on to senior partners for analysis.
What is the implicit logic of this division of labor? First, to reduce the risk of junior staff making mistakes on complex client-facing work; and second, to maximize the effectiveness of senior partners: the less time junior staff have to explain their work to them, the more they can focus on higher-level analysis. This improves efficiency in the short term, but deprives junior analysts of the opportunity to challenge complex work, makes it more difficult for them to understand the entire valuation process, and weakens the company's future capabilities.
2. Experts are alienated from their work
Sometimes smart machines get caught between trainees and their work, and sometimes they prevent experts from doing important practical work. In robotic surgery, the surgeon cannot see the patient's body or the robot for most of the procedure, making it impossible to directly assess and manage critical aspects. For example, in traditional surgery, surgeons are acutely aware of how devices and instruments touch the patient's body and adjust accordingly. But in robotic surgery, surgeons must rely on others to alert them if a robotic arm hits a patient's head, or if a cleaning arm is about to replace an instrument. This has a twofold impact on learning: surgeons are unable to hone the skills needed to fully understand their own work, and surgeons must acquire such new skills through others.
3. Learners must master both old and new methods
Robotic surgery uses a new set of skills and techniques to achieve the effects that traditional surgery attempts to achieve. It promises greater precision and better ergonomics and is incorporated directly into the curriculum, with residents required to learn both robotics and traditional methods. But the course doesn't give them enough time to master both, which often leads to the worst result: mastering neither. I call this problem methodological overload.
4. Standard learning methods are defaulted to be effective
Decades of research and tradition have led trainee doctors to follow the “see one, do one, teach one” approach. But as we have seen, it is not adapted to robotic surgery. Still, the pressure to rely on old-school learning methods is strong, and there are few deviants: Surgical training studies, standard procedures, policies, and senior surgeons all continue to emphasize traditional learning methods, even when it is clearly inappropriate for robotic surgery.
Learning in the Shadows
Faced with the above obstacles, it’s no surprise that covert learners quietly bypass or break the rules to gain the guidance and experience they need. Nearly 100 years ago, sociologist Robert Merton discovered that extraordinary measures are used when legitimate means no longer work to achieve worthy goals. The same goes for professional knowledge (perhaps the ultimate goal of a career).
Given the barriers I describe, we should understand that people will learn key skills in other ways. These methods are generally flexible and effective, but they often impose costs on individuals and organizations: implicit learners may be punished, such as losing practice opportunities or status, or causing waste or even harm. But people still take risks again and again because their learning methods work when compliance methods fail. It would be wrong to imitate these extraordinary methods without discernment, but they do have organizational features worth learning from.
1. Continuous Learning
As smart technologies become more powerful, covert learning is also developing rapidly. New forms will emerge over time, providing new experiences. It is vital to remain cautious. Covert learners are often aware that what they are doing is unconventional, and they may be punished for what they are doing. (Imagine if a surgical resident made it known that he or she wanted to work with the least skilled attending.) Because it produces results, middle managers often turn a blind eye to these practices as long as the covert learner does not openly acknowledge them. When observers, especially senior managers, declare a desire to study how employees gain skills by breaking rules, learners and their managers may be reluctant to share their experiences. A better solution is to introduce a neutral third party that can ensure strict anonymity and compare practices in different cases. My informants came to know and trust me, and they realized that I was observing work in many work groups and facilities, so they felt confident that their identities would be protected. This is crucial to getting them to tell the truth.
2. Adapt the implicit learning practices you discover to fit the structure of the organization, work, and technology
Organizational approaches to intelligent machines often stop at letting individual experts control the work and reduce reliance on trainees. level. Robotic surgical systems allowed senior surgeons to operate with less help, and they did. The investment banking system allows senior partners to exclude junior analysts from complex valuation work, and they do. All stakeholders should insist that organization, technology and work design improve productivity and strengthen OJL. For example, in the Los Angeles Police Department, this will mean changing incentives for patrol officers, redesigning the PredPol user interface, creating new roles to connect police and software engineers, and building an annotated library of best practices cases initiated by police officers.
3. Make smart machines part of the solution
Artificial intelligence can help learners when they encounter difficulties, provide training to experts as mentors, and cleverly connect the two group. For example, Juho Kim built ToolScape and Lecture-Scape when he was a PhD student at MIT, which can crowdsource annotations for teaching videos and provide clarification and opportunities for users who had previously paused to find annotations. He calls it learner sourcing. On the hardware side, augmented reality systems are starting to bring expert guidance and annotation into workflows.
Existing apps use tablets or smart glasses to add guidance to work in real time. More sophisticated smart systems are expected soon. For example, such a system could overlay footage of a model welder in the factory over an apprentice welder's field of view, showing how the job was done, recording the apprentice's attempts for comparison, and connecting the apprentice to the model welder as needed. While much of the growing community of engineers in these fields is focused on formal training, the deeper crisis is OJL. We need to reallocate our efforts on OJL.
Over thousands of years, technological advances have driven the redesign of work processes, and apprentices have acquired necessary new skills from their mentors. But as we’ve seen, smart machines are now forcing us to disconnect apprentices from mentors and mentors from work in the name of productivity. Organizations often inadvertently choose productivity over employee engagement, so learning on the job becomes increasingly difficult. However, secret learners are looking for risky, out-of-the-box learning methods. Organizations that want to compete in a world of intelligent machines should pay close attention to these “non-conformists.” Their actions can provide insight into how best to get things done in the future when experts, apprentices, and smart machines work and learn together.
The above is the detailed content of Challenges and responses to working with artificial intelligence. For more information, please follow other related articles on the PHP Chinese website!
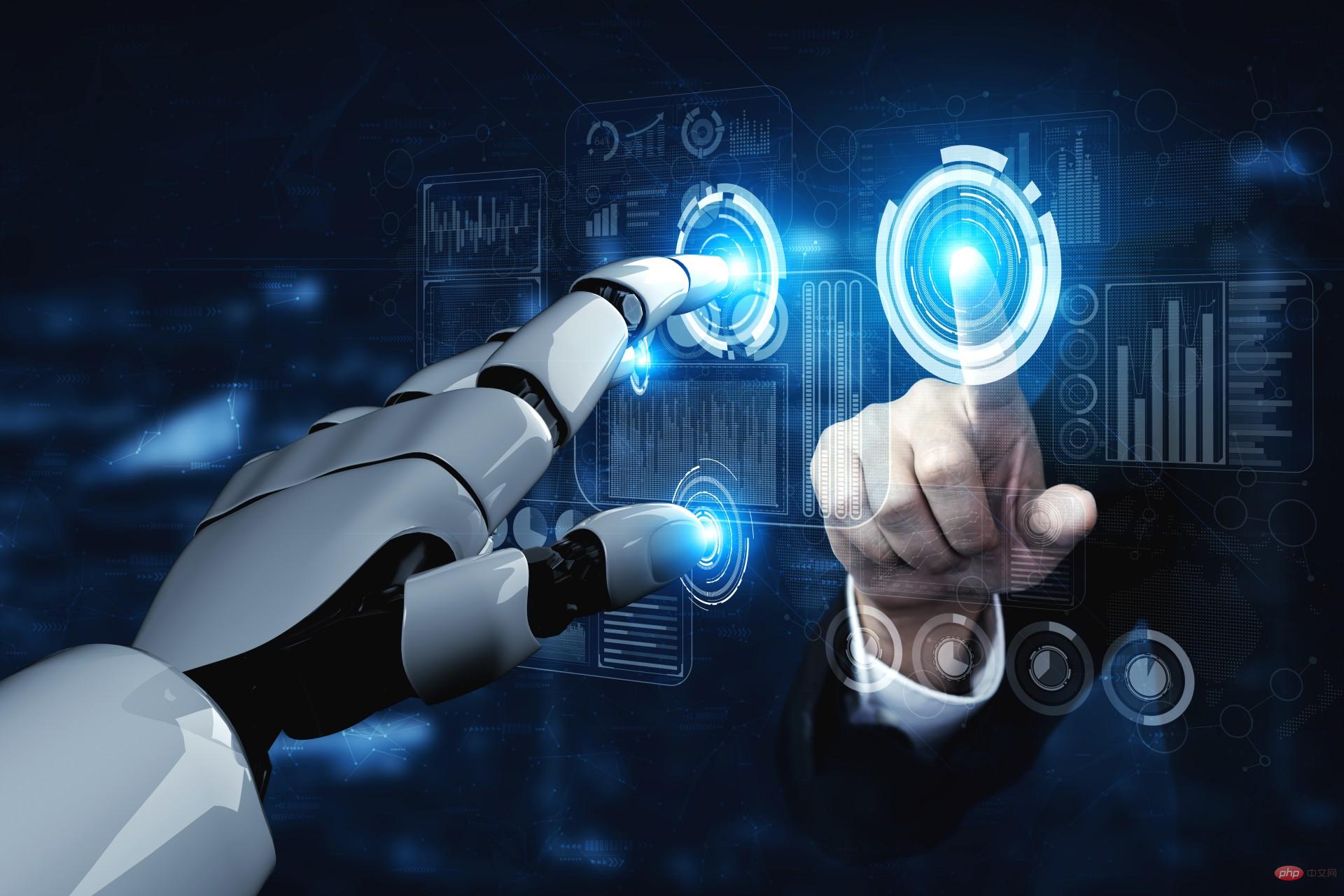
机器学习是一个不断发展的学科,一直在创造新的想法和技术。本文罗列了2023年机器学习的十大概念和技术。 本文罗列了2023年机器学习的十大概念和技术。2023年机器学习的十大概念和技术是一个教计算机从数据中学习的过程,无需明确的编程。机器学习是一个不断发展的学科,一直在创造新的想法和技术。为了保持领先,数据科学家应该关注其中一些网站,以跟上最新的发展。这将有助于了解机器学习中的技术如何在实践中使用,并为自己的业务或工作领域中的可能应用提供想法。2023年机器学习的十大概念和技术:1. 深度神经网

本文将详细介绍用来提高机器学习效果的最常见的超参数优化方法。 译者 | 朱先忠审校 | 孙淑娟简介通常,在尝试改进机器学习模型时,人们首先想到的解决方案是添加更多的训练数据。额外的数据通常是有帮助(在某些情况下除外)的,但生成高质量的数据可能非常昂贵。通过使用现有数据获得最佳模型性能,超参数优化可以节省我们的时间和资源。顾名思义,超参数优化是为机器学习模型确定最佳超参数组合以满足优化函数(即,给定研究中的数据集,最大化模型的性能)的过程。换句话说,每个模型都会提供多个有关选项的调整“按钮
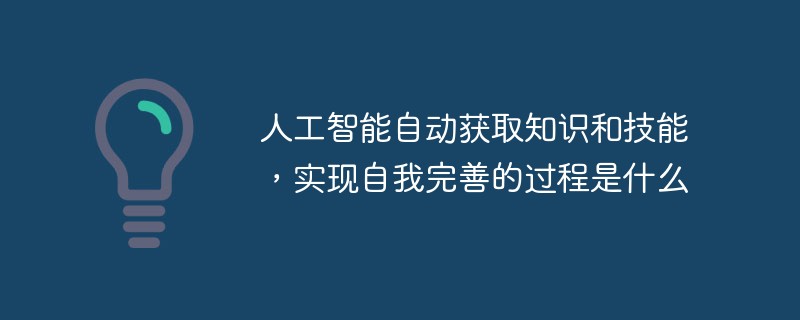
实现自我完善的过程是“机器学习”。机器学习是人工智能核心,是使计算机具有智能的根本途径;它使计算机能模拟人的学习行为,自动地通过学习来获取知识和技能,不断改善性能,实现自我完善。机器学习主要研究三方面问题:1、学习机理,人类获取知识、技能和抽象概念的天赋能力;2、学习方法,对生物学习机理进行简化的基础上,用计算的方法进行再现;3、学习系统,能够在一定程度上实现机器学习的系统。
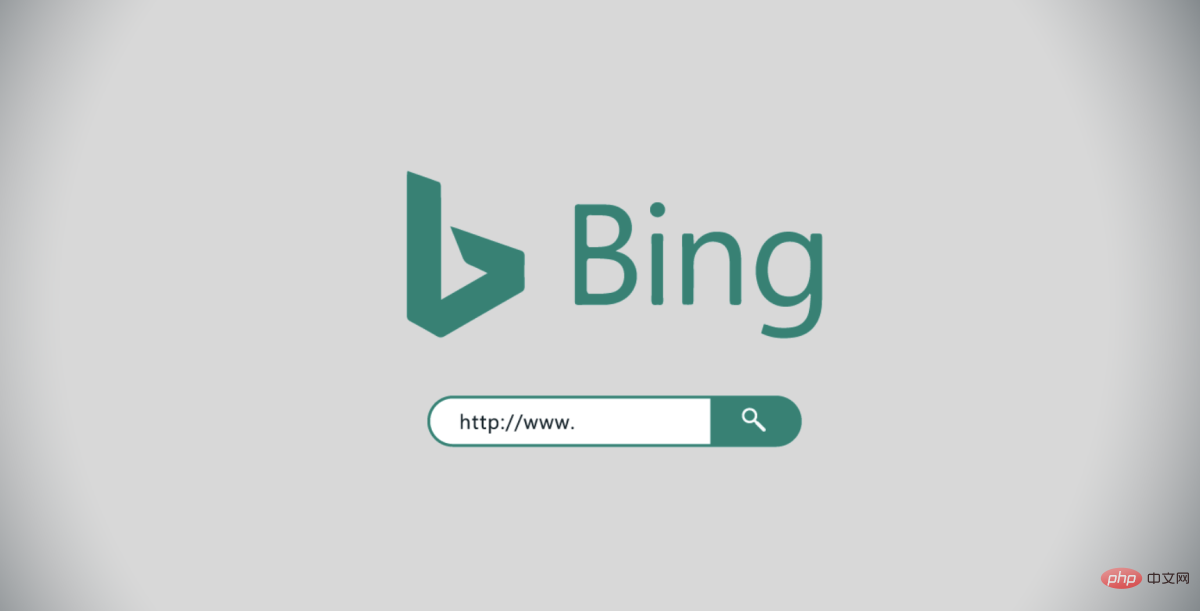
截至3月20日的数据显示,自微软2月7日推出其人工智能版本以来,必应搜索引擎的页面访问量增加了15.8%,而Alphabet旗下的谷歌搜索引擎则下降了近1%。 3月23日消息,外媒报道称,分析公司Similarweb的数据显示,在整合了OpenAI的技术后,微软旗下的必应在页面访问量方面实现了更多的增长。截至3月20日的数据显示,自微软2月7日推出其人工智能版本以来,必应搜索引擎的页面访问量增加了15.8%,而Alphabet旗下的谷歌搜索引擎则下降了近1%。这些数据是微软在与谷歌争夺生
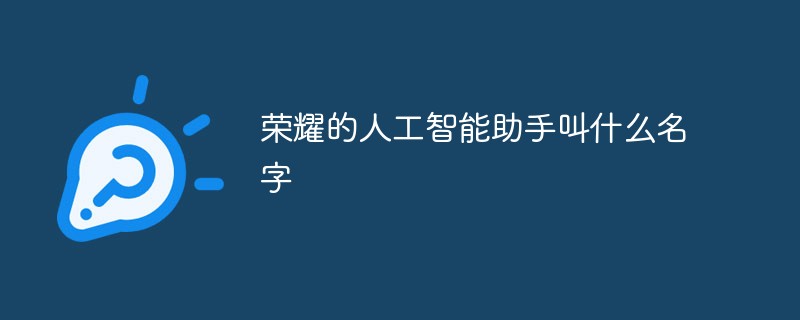
荣耀的人工智能助手叫“YOYO”,也即悠悠;YOYO除了能够实现语音操控等基本功能之外,还拥有智慧视觉、智慧识屏、情景智能、智慧搜索等功能,可以在系统设置页面中的智慧助手里进行相关的设置。
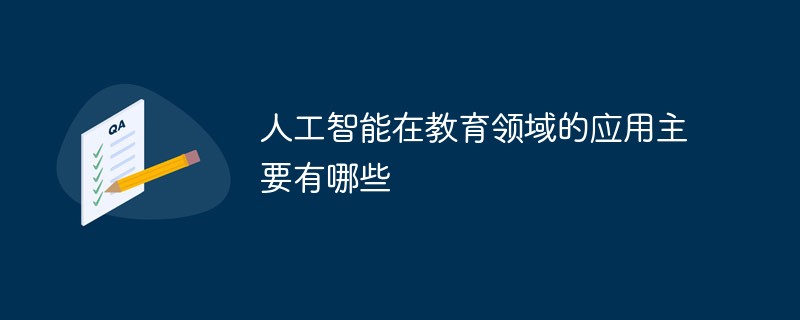
人工智能在教育领域的应用主要有个性化学习、虚拟导师、教育机器人和场景式教育。人工智能在教育领域的应用目前还处于早期探索阶段,但是潜力却是巨大的。
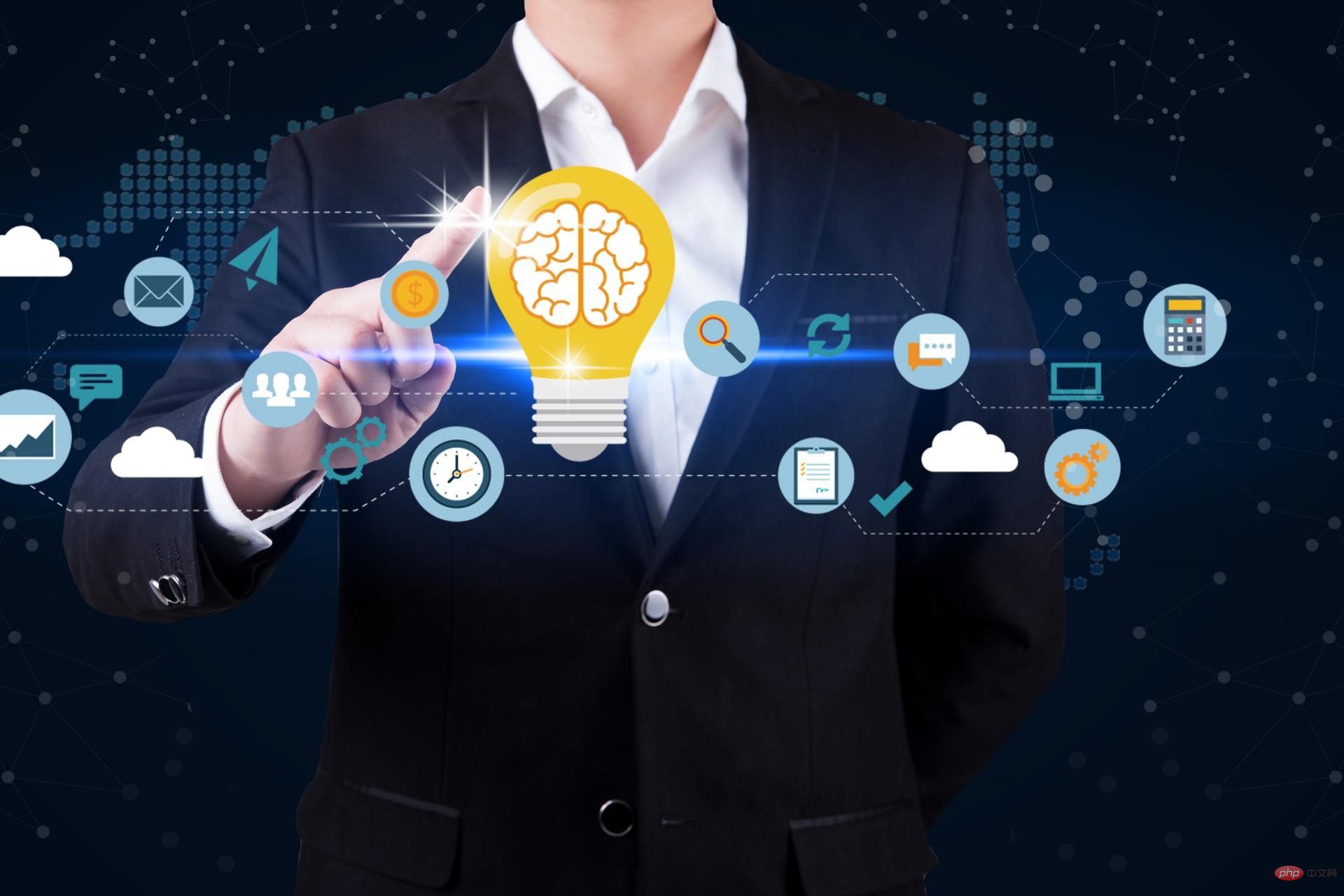
阅读论文可以说是我们的日常工作之一,论文的数量太多,我们如何快速阅读归纳呢?自从ChatGPT出现以后,有很多阅读论文的服务可以使用。其实使用ChatGPT API非常简单,我们只用30行python代码就可以在本地搭建一个自己的应用。 阅读论文可以说是我们的日常工作之一,论文的数量太多,我们如何快速阅读归纳呢?自从ChatGPT出现以后,有很多阅读论文的服务可以使用。其实使用ChatGPT API非常简单,我们只用30行python代码就可以在本地搭建一个自己的应用。使用 Python 和 C
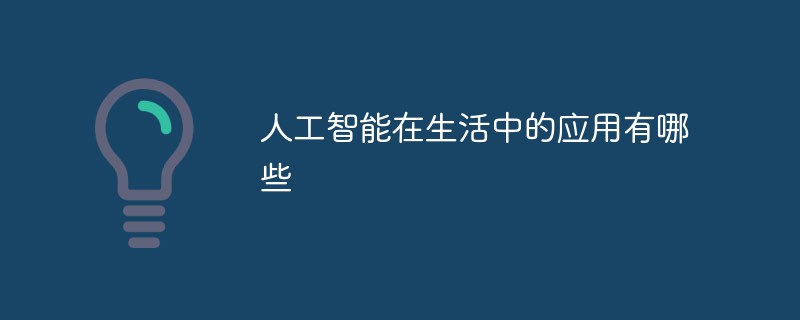
人工智能在生活中的应用有:1、虚拟个人助理,使用者可通过声控、文字输入的方式,来完成一些日常生活的小事;2、语音评测,利用云计算技术,将自动口语评测服务放在云端,并开放API接口供客户远程使用;3、无人汽车,主要依靠车内的以计算机系统为主的智能驾驶仪来实现无人驾驶的目标;4、天气预测,通过手机GPRS系统,定位到用户所处的位置,在利用算法,对覆盖全国的雷达图进行数据分析并预测。


Hot AI Tools

Undresser.AI Undress
AI-powered app for creating realistic nude photos

AI Clothes Remover
Online AI tool for removing clothes from photos.

Undress AI Tool
Undress images for free

Clothoff.io
AI clothes remover

AI Hentai Generator
Generate AI Hentai for free.

Hot Article

Hot Tools

SAP NetWeaver Server Adapter for Eclipse
Integrate Eclipse with SAP NetWeaver application server.

PhpStorm Mac version
The latest (2018.2.1) professional PHP integrated development tool

DVWA
Damn Vulnerable Web App (DVWA) is a PHP/MySQL web application that is very vulnerable. Its main goals are to be an aid for security professionals to test their skills and tools in a legal environment, to help web developers better understand the process of securing web applications, and to help teachers/students teach/learn in a classroom environment Web application security. The goal of DVWA is to practice some of the most common web vulnerabilities through a simple and straightforward interface, with varying degrees of difficulty. Please note that this software

SublimeText3 English version
Recommended: Win version, supports code prompts!
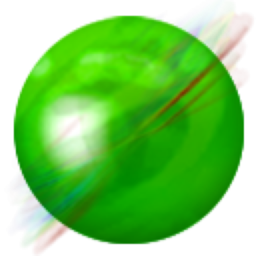
ZendStudio 13.5.1 Mac
Powerful PHP integrated development environment
