


Currently, the selection of non-English text and image generation models is limited, and users often have to translate the prompt into English before entering the model. This will not only cause additional operational burden, but also language and cultural errors in the translation process will affect the accuracy of the generated images.
Zhiyuan Research Institute’s FlagAI team pioneered an efficient training method, using a multi-language pre-training model combined with Stable Diffusion to train a multi-language text and image generation model - AltDiffusion-m18, supporting 18 types Language text-image generation.
Including Chinese, English, Japanese, Thai, Korean, Hindi, Ukrainian, Arabic, Turkish, Vietnamese, Polish, Dutch, Portuguese, Italian, Spanish, German, French, Russian.
Huggingface: https://huggingface.co/BAAI/AltDiffusion-m18
GitHub: https://github.com/FlagAI-Open/FlagAI/blob/master/examples/AltDiffusion -m18
AltDiffusion-m18 achieved Stable Diffusion 95~99% effect in the objective evaluation of FID, IS, CLIP score in English, reached the optimal level in Chinese and Japanese, and filled in the remaining 15 categories. The gap in the language text and picture generation model has greatly satisfied the industry's strong demand for multi-language text and picture generation. Special thanks go to the Stable Diffusion Research Team for providing advice on this work.
In addition, AltDiffusion-m18 related innovative technology report "AltCLIP: Altering the Language Encoder in CLIP for Extended Language Capabilities" has been accepted by Findings of ACL 2023.
Technical Highlights
1 New AltCLIP, efficient and low-cost construction of multi-language T2I model
AltDiffusion released last year -m9, based on Stable Diffusion v1.4, the Zhiyuan team innovatively replaced the language tower with the multi-language tower AltCLIP, and used multi-language data in nine languages for fine-tuning, extending the original Stable Diffusion that only supports English to support 9 different languages.
AltCLIP: https://github.com/FlagAI-Open/FlagAI/tree/master/examples/AltCLIP-m18
And AltDiffusion-m18 is based on Stable Diffusion v2.1 training. The new language tower of Stable Diffusion v2.1 is the inverted second layer of OpenCLIP. Therefore, the new AltCLIP uses the inverted second layer of OpenCLIP as the distillation target to retrain, and based on m9, it will only use the CrossAttention layer K and V matrices in Unet. Fine-tuning is expanded into a two-stage training method, as shown in the figure below:
- First stage: Earlier during the experiment of m9, it was discovered that fine-tuning the K and V matrices The main thing to learn is the conceptual alignment of text and pictures, so the first stage of m18 training continues to use the data of 18 languages to fine-tune the K and V matrices. In addition, experiments have proven that reducing the resolution of an image from 512*512 to 256*256 does not lose the semantic information of the image. Therefore, in the first stage of learning text-image concept alignment, the resolution of 256*256 is used for training, which speeds up the training.
- The second stage: In order to further improve the quality of the generated images, use the resolution of 512*512 to train the full parameters of Unet in the data of 18 languages. In addition, 10% of the text is discarded for unconditional training to serve classifier-free guidance inference.
- In addition, a classifier-free guided training technique is adopted to further improve the generation quality.
The latest evaluation results show that AltCLIP-m18 surpasses CLIP and reaches the optimal level in Chinese and English zero-shot (zero sample) retrieval tasks⬇️
On multi-language image classification benchmarks, AltCLIP-m9 (early version, supports 9 languages) and AltCLIP-m18 reach the optimal level ⬇️
Similarly, thanks to AltCLIP With the innovative idea of changing towers, AltDiffusion-m18 can also be seamlessly connected to all Stable Diffusion models and ecological tools built on the original CLIP. All tools that support Stable Diffusion such as Stable Diffusion WebUI, DreamBooth, etc. can be applied to AltDiffusion-m18. Painless to get started and great playability!
2 Multi-language generation effects are aligned, with superior performance and accurate details
With the blessing of the new AltCLIP, AltDiffusion-m18 has achieved 95~99% of the original Stable Diffusion effect in the English FID, IS, CLIP score evaluation, and has achieved the most advanced performance in 17 languages including Chinese and Japanese. The performance of AltDiffusion-m18 is shown in the following table:
The above is the detailed content of AltDiffusion-m18, a versatile tool for generating multilingual texts and images. For more information, please follow other related articles on the PHP Chinese website!
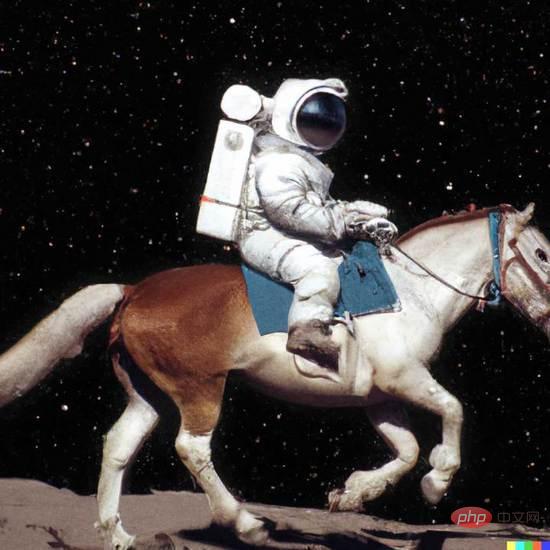
1 前言在发布DALL·E的15个月后,OpenAI在今年春天带了续作DALL·E 2,以其更加惊艳的效果和丰富的可玩性迅速占领了各大AI社区的头条。近年来,随着生成对抗网络(GAN)、变分自编码器(VAE)、扩散模型(Diffusion models)的出现,深度学习已向世人展现其强大的图像生成能力;加上GPT-3、BERT等NLP模型的成功,人类正逐步打破文本和图像的信息界限。在DALL·E 2中,只需输入简单的文本(prompt),它就可以生成多张1024*1024的高清图像。这些图像甚至
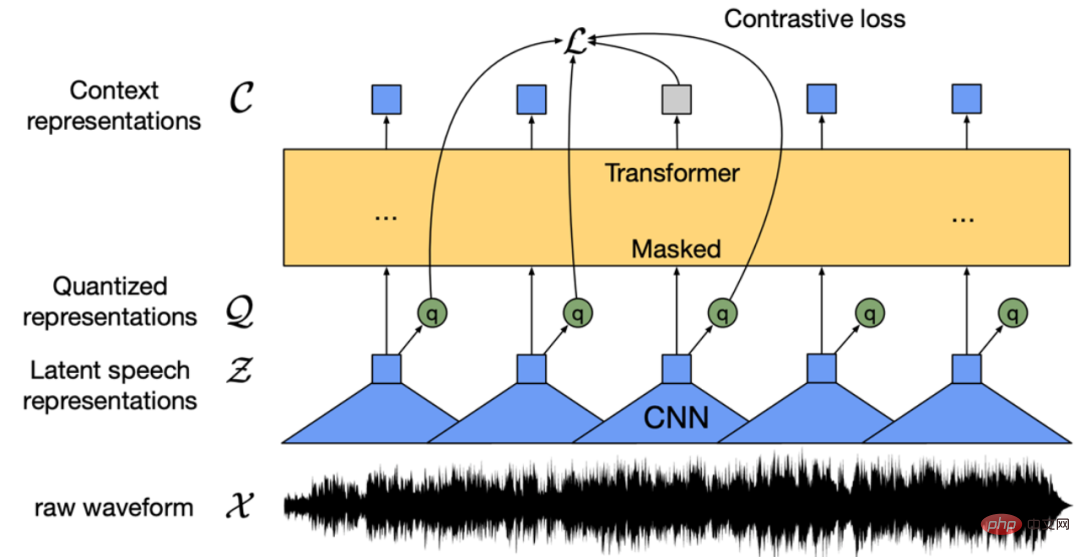
Wav2vec 2.0 [1],HuBERT [2] 和 WavLM [3] 等语音预训练模型,通过在多达上万小时的无标注语音数据(如 Libri-light )上的自监督学习,显著提升了自动语音识别(Automatic Speech Recognition, ASR),语音合成(Text-to-speech, TTS)和语音转换(Voice Conversation,VC)等语音下游任务的性能。然而这些模型都没有公开的中文版本,不便于应用在中文语音研究场景。 WenetSpeech [4] 是
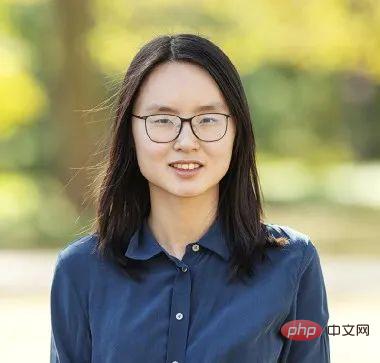
“Making large models smaller”这是很多语言模型研究人员的学术追求,针对大模型昂贵的环境和训练成本,陈丹琦在智源大会青源学术年会上做了题为“Making large models smaller”的特邀报告。报告中重点提及了基于记忆增强的TRIME算法和基于粗细粒度联合剪枝和逐层蒸馏的CofiPruning算法。前者能够在不改变模型结构的基础上兼顾语言模型困惑度和检索速度方面的优势;而后者可以在保证下游任务准确度的同时实现更快的处理速度,具有更小的模型结构。陈丹琦 普
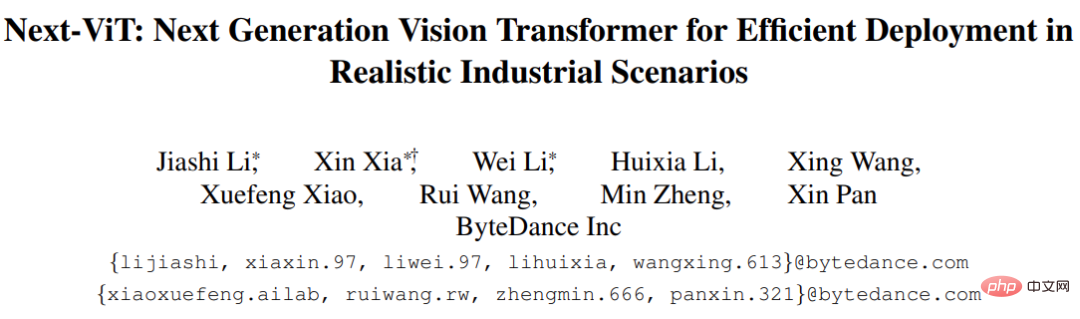
由于复杂的注意力机制和模型设计,大多数现有的视觉 Transformer(ViT)在现实的工业部署场景中不能像卷积神经网络(CNN)那样高效地执行。这就带来了一个问题:视觉神经网络能否像 CNN 一样快速推断并像 ViT 一样强大?近期一些工作试图设计 CNN-Transformer 混合架构来解决这个问题,但这些工作的整体性能远不能令人满意。基于此,来自字节跳动的研究者提出了一种能在现实工业场景中有效部署的下一代视觉 Transformer——Next-ViT。从延迟 / 准确性权衡的角度看,
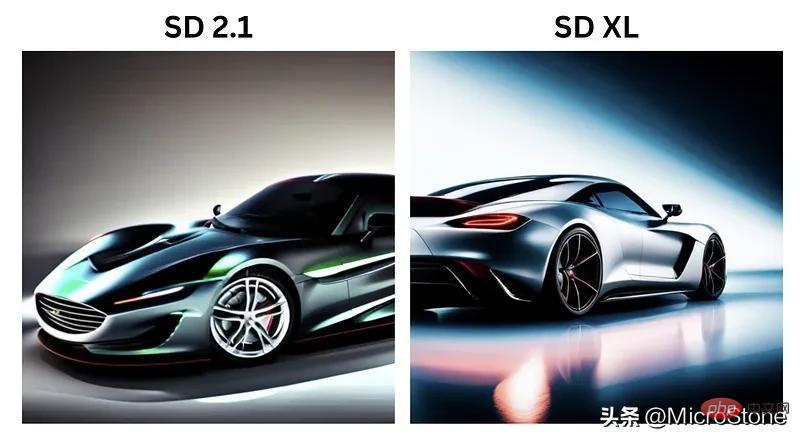
3月27号,Stability AI的创始人兼首席执行官Emad Mostaque在一条推文中宣布,Stable Diffusion XL 现已可用于公开测试。以下是一些事项:“XL”不是这个新的AI模型的官方名称。一旦发布稳定性AI公司的官方公告,名称将会更改。与先前版本相比,图像质量有所提高与先前版本相比,图像生成速度大大加快。示例图像让我们看看新旧AI模型在结果上的差异。Prompt: Luxury sports car with aerodynamic curves, shot in a
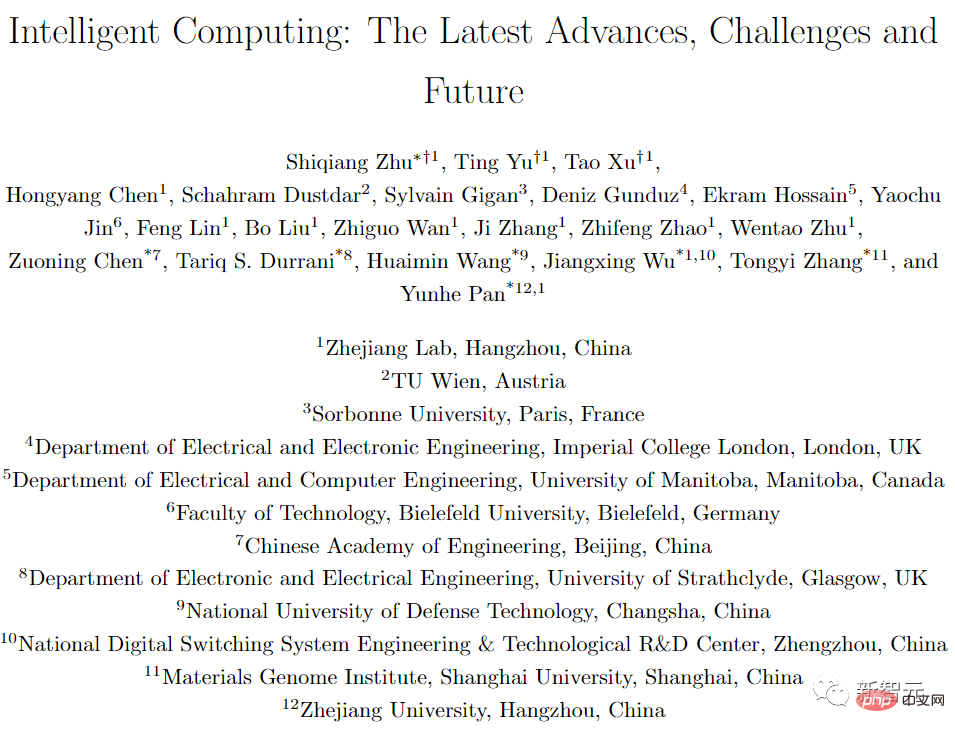
人工智能就是一个「拼财力」的行业,如果没有高性能计算设备,别说开发基础模型,就连微调模型都做不到。但如果只靠拼硬件,单靠当前计算性能的发展速度,迟早有一天无法满足日益膨胀的需求,所以还需要配套的软件来协调统筹计算能力,这时候就需要用到「智能计算」技术。最近,来自之江实验室、中国工程院、国防科技大学、浙江大学等多达十二个国内外研究机构共同发表了一篇论文,首次对智能计算领域进行了全面的调研,涵盖了理论基础、智能与计算的技术融合、重要应用、挑战和未来前景。论文链接:https://spj.scien
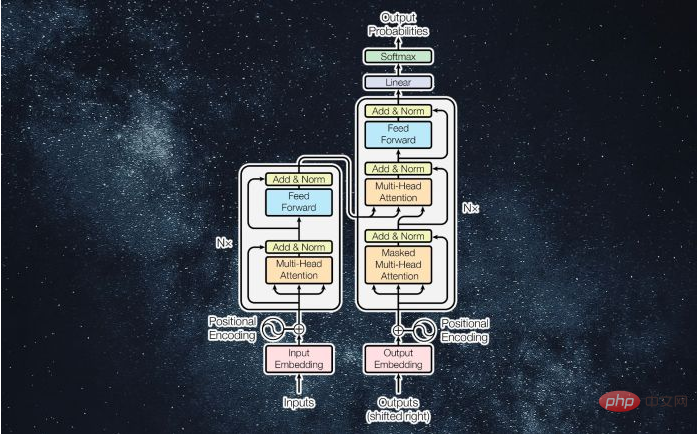
译者 | 李睿审校 | 孙淑娟近年来, Transformer 机器学习模型已经成为深度学习和深度神经网络技术进步的主要亮点之一。它主要用于自然语言处理中的高级应用。谷歌正在使用它来增强其搜索引擎结果。OpenAI 使用 Transformer 创建了著名的 GPT-2和 GPT-3模型。自从2017年首次亮相以来,Transformer 架构不断发展并扩展到多种不同的变体,从语言任务扩展到其他领域。它们已被用于时间序列预测。它们是 DeepMind 的蛋白质结构预测模型 AlphaFold
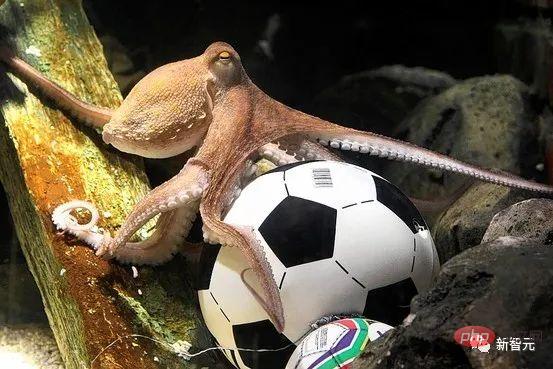
说起2010年南非世界杯的最大网红,一定非「章鱼保罗」莫属!这只位于德国海洋生物中心的神奇章鱼,不仅成功预测了德国队全部七场比赛的结果,还顺利地选出了最终的总冠军西班牙队。不幸的是,保罗已经永远地离开了我们,但它的「遗产」却在人们预测足球比赛结果的尝试中持续存在。在艾伦图灵研究所(The Alan Turing Institute),随着2022年卡塔尔世界杯的持续进行,三位研究员Nick Barlow、Jack Roberts和Ryan Chan决定用一种AI算法预测今年的冠军归属。预测模型图


Hot AI Tools

Undresser.AI Undress
AI-powered app for creating realistic nude photos

AI Clothes Remover
Online AI tool for removing clothes from photos.

Undress AI Tool
Undress images for free

Clothoff.io
AI clothes remover

AI Hentai Generator
Generate AI Hentai for free.

Hot Article

Hot Tools
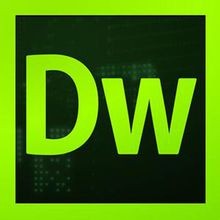
Dreamweaver CS6
Visual web development tools
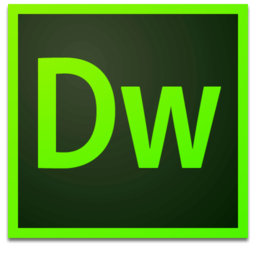
Dreamweaver Mac version
Visual web development tools

mPDF
mPDF is a PHP library that can generate PDF files from UTF-8 encoded HTML. The original author, Ian Back, wrote mPDF to output PDF files "on the fly" from his website and handle different languages. It is slower than original scripts like HTML2FPDF and produces larger files when using Unicode fonts, but supports CSS styles etc. and has a lot of enhancements. Supports almost all languages, including RTL (Arabic and Hebrew) and CJK (Chinese, Japanese and Korean). Supports nested block-level elements (such as P, DIV),

Notepad++7.3.1
Easy-to-use and free code editor

Zend Studio 13.0.1
Powerful PHP integrated development environment
